the Creative Commons Attribution 4.0 License.
the Creative Commons Attribution 4.0 License.
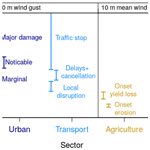
Review article: A European perspective on wind and storm damage – from the meteorological background to index-based approaches to assess impacts
Daniel Gliksman
Paul Averbeck
Nico Becker
Barry Gardiner
Valeri Goldberg
Jens Grieger
Dörthe Handorf
Karsten Haustein
Alexia Karwat
Florian Knutzen
Hilke S. Lentink
Rike Lorenz
Deborah Niermann
Joaquim G. Pinto
Ronald Queck
Astrid Ziemann
Christian L. E. Franzke
Wind and windstorms cause severe damage to natural and human-made environments. Thus, wind-related risk assessment is vital for the preparation and mitigation of calamities. However, the cascade of events leading to damage depends on many factors that are environment-specific and the available methods to address wind-related damage often require sophisticated analysis and specialization. Fortunately, simple indices and thresholds are as effective as complex mechanistic models for many applications. Nonetheless, the multitude of indices and thresholds available requires a careful selection process according to the target sector. Here, we first provide a basic background on wind and storm formation and characteristics, followed by a comprehensive collection of both indices and thresholds that can be used to predict the occurrence and magnitude of wind and storm damage. We focused on five key sectors: forests, urban areas, transport, agriculture and wind-based energy production. For each sector we described indices and thresholds relating to physical properties such as topography and land cover but also to economic aspects (e.g. disruptions in transportation or energy production). In the face of increased climatic variability, the promotion of more effective analysis of wind and storm damage could reduce the impact on society and the environment.
- Article
(1236 KB) -
Supplement
(456 KB) - BibTeX
- EndNote
Wind is a common feature of our day-to-day weather just like air temperature and precipitation. Wind is per definition a sustained air movement in the atmosphere, which can range from still conditions to extraordinary values, from very local to global spatial scales, and has a wide range of temporal scales from seconds to decades. Winds can have both a beneficial and detrimental effect on society, infrastructure and agriculture. On the one hand, storms, which have very strong winds, can lead to considerable damage in infrastructure and forestry, e.g. Storm Kyrill in 2007 (Fink et al., 2009), contribute to widespread forest fires, e.g. Australia 2020 (van Oldenborgh et al., 2021) or enhance evaporation, thus drying out the soil (Bittelli et al., 2008). We view damage as a disadvantageous change in the quantities, quality or function of an object. On the other hand, moderately strong winds can have positive effects on wind energy production and cause a stronger mixing in the boundary layer (cancelling detrimental thermal inversions to agriculture) or – in the case of nightly slope winds – alleviate summer heat conditions in valleys and cities (Ganbat et al., 2015).
The damage associated with strong winds is primarily due to short-term wind gusts and leads to a substantial increase in wind speed (Brasseur, 2001). Wind gusts are sudden increases in wind speed, which last typically less than 20 s, while strong winds refer to sustained wind speed over longer time periods. Strong wind gusts often lead to uprooting or breaking of trees, damage to crops in fields (Gardiner et al., 2016), lifting of roofs and damaging critical infrastructure like bridges and roads (Klawa and Ulbrich, 2003; Mitchell-Wallace et al., 2017). In coastal areas, strong winds and wind gusts may lead to storm surges and coastal flooding (Flather, 2001). The exact impacts of strong winds depend also on other factors besides wind speed thresholds. For example, damage to forests depends on many other factors like precipitation and topography (Gardiner, 2021). Thus, to predict damage or identify areas at risk of wind or storm damage, indices are a vital tool in assessing the likelihood and magnitude of damage in a given sector or environment. For example, Merz et al. (2020) explore in their review the current state of knowledge on skilful forecasts of impacts for many hazards, for which indices are very useful. With storm damage we refer to damage, mainly to properties and forests, caused by severe wind storms, while wind damage is more general and includes all adverse effects of wind, including storm damage. We define risk as the likelihood here that wind causes some damage, and their consequences and risk can be quantified as the function of hazard probability, exposure and vulnerability (e.g. Kelman, 2003; Hoeppe, 2016; Franzke, 2017).
For wind indices and wind impact models different wind parameters are in use. These are often derived from modelled data like reanalysis data sets. While these model parameters are strongly related to observed wind parameters, they are not the same and their definitions cannot be used interchangeably. Since observational data are rare and it is more common to work with modelled data, the following parameter definitions focus on parameters derived from models. It is often assumed that the maximum daily or hourly gust speed (metres per second) at 10 m height relates strongest to damage. The WMO defines a wind gust as the maximum of the wind averaged over 3 s intervals, which is in most cases shorter than the model time step. Thus, many models rely on parametrization for gust speed. For example, the ECMWF Integrated Forecasting System deduces the magnitude of a gust within each time step from the time-step-averaged surface stress, surface friction, wind shear and stability. Other common parameters in use are daily or hourly mean or maximum wind speeds at 10 m height, which express the mean or maximum values of all model time steps in an hour or a day. The parameterized gust speed as well as mean wind speeds in a model grid cell can deviate widely from local observations.
Indices can be used to predict damage caused directly by wind, or to quantify how the wind modulates the damage caused by another process such as fire or drought. Furthermore, the choice of indices depends also on land use as it influences the interaction between land surfaces and the wind; tree species and forest structures can have considerable influence on the damage probability (Gardiner, 2021). The understanding of wind and storm dynamics, and the ability to predict the damage they cause, requires an interdisciplinary approach. However, much of the relevant literature is in specialized journals. Here, we aim to bring these different disciplines together to provide an interdisciplinary synthesis of the topic. To bridge the gap between the different communities, within the ClimXtreme consortium, we created a work group and invited specialists from outside the consortium to broaden our research expertise. During regular joint meetings we identified the following sectors: forests, urban areas, transport, agriculture and energy as the most relevant terrestrial environments that could be impacted by wind and storm damage. We focused on literature resources stemming mainly from Europe, but in cases of relevance and to further expand the scope of the review we also incorporated examples from other regions.
We provide a basic background on wind and storm formation and intra-seasonal variability in Sect. 2. Section 3 focuses on the interactions between wind and surface structures which are prone to wind damage. Section 4 focuses on wind- and storm-related indices and thresholds. In particular, we cover the following sectors: forests, urban areas, transport, agriculture and energy. Additionally, we discuss compound indices and thresholds used by national weather services. Finally, in Sect. 5 we provide an outlook and discuss open research questions. Due to the location of the authors, we provide mainly a European perspective on this topic but believe our synthesis is more widely applicable.
2.1 The general circulation and wind generation
The general circulation of the atmosphere is driven by the differential heating of the Earth (Held, 2019); the equatorial regions receive more solar radiation than higher latitudes, while in the polar regions the atmosphere is losing heat into space. This differential heating of the Earths' surface causes pressure differences in the atmosphere. As a result, a pressure gradient force acts on the air masses, leading to a movement from high- to low-pressure centres to alleviate this pressure difference. Since the atmosphere moves toward an equilibrium, it causes a meridional heat transport towards the poles through the atmosphere and ocean, which takes place mainly through the movements of circulation systems and storms (Bjerknes, 1922; Schultz et al., 2019; Ma et al., 2021).
Mid-latitude weather systems include both cyclones and anticyclones, but strong wind situations are primarily associated with intense cyclones. The main paths that weather systems and storms take are called storm tracks (Hoskins and Valdez, 1990; Blender et al., 1997; Chang et al., 2002; Ulbrich et al., 2009). Storm tracks form over the major ocean basins of the Northern Hemisphere and Southern Hemisphere and are closely related to atmospheric jet streams, which are areas of maximum upper-level wind speed and determine the areas that are prone to storms as discussed below in Sect. 2.4. These regimes set the propensity with which weather systems take a more poleward or equatorward path on intra-seasonal timescales, thus offering potential predictability.
In its most basic form, atmospheric jet streams (Feldstein and Franzke, 2017) are a product of the pressure gradient force, induced by the above-mentioned latitudinal air temperature gradients, and the Coriolis force. For large-scale movements in the atmosphere, the wind is diverted to the right (left) in the Northern (Southern) Hemisphere due to the Coriolis force. The resulting winds in the free atmosphere, above the boundary layer, blow parallel to lines of equal pressure, in a balance between the pressure gradient and the Coriolis force, also called geostrophic wind. The strength of the dominant westerly winds over western Europe is determined by the pressure difference between the subpolar and subtropical regions over the eastern North Atlantic. The stronger the pressure difference, the stronger the mid-latitude westerlies.
Under hypothetical unperturbed conditions, the bands of maximum wind speed sit at 30 and 60∘ latitude in either hemisphere at upper levels of the troposphere, due to surface friction. However, differential diabatic heating over land and the ocean, or orographic surface features, such as mountains, do perturb the jet stream in multiple ways. As a result, in the extratropics of the Northern Hemisphere the jet stream is commonly split into a subtropical and mid-latitudinal branch. While the former is mainly driven by angular momentum transport by the thermally direct Hadley circulation (Held and Hou, 1980), the latter is primarily driven by the eddy momentum flux convergence provided by short waves that form in regions of enhanced baroclinicity (Held, 1975). Accordingly, the mid-latitudinal jet stream is referred to as an eddy-driven or polar jet stream due to its proximity to polar latitudes.
In the atmosphere unstable conditions are needed for weather systems to form (Holton and Hakim, 2012). So-called baroclinically unstable conditions occur where we find strong horizontal and vertical air temperature gradients. For example, the North Atlantic is an ideal source region for baroclinically unstable conditions as very cold polar air is advected over moderately warm ocean waters, leading to excessive air temperature gradients and, thus, pressure gradients, which – under the influence of the Coriolis force – generate enhanced baroclinicity.
In the boundary layer, the pressure gradient and Coriolis forces are not in balance, because the surface characteristics, local conditions, vertical stability, and other effects play crucial roles in modifying the winds. Under the influence of surface friction, the air movements are not parallel to the lines of equal pressure but have a tangential component from high- to low-pressure centres. On the regional to local scale, wind systems like the land–sea breeze and mountain-valley wind systems develop due to differential heating conditions within comparatively small distances, which vary between day- and nighttime.
2.2 How do cyclones form?
While anti-cyclones are primarily associated with low wind conditions in their centre and strong winds are only found around its edges (i.e. co-located with another pressure system), cyclones feature typically strong pressure gradients and are thus associated with strong winds and wind gusts. Many extratropical cyclones develop under the influence of the mid-latitude jet stream, its associated baroclinicity and upper-air flow divergence. Other cyclones develop as secondary cyclones in the trailing cold fronts of pre-existing systems and are more influenced by lower-level processes such as latent heat release (Parker, 1998; Dacre and Gray, 2009). Another large group of cyclones develop by the interaction of atmospheric waves with topography (McGinley, 1982; Radinovic, 1987). Focusing on the North Atlantic sector for a European perspective, baroclinically driven (primary) cyclones develop typically over the North Atlantic (Dacre and Gray, 2009), secondary cyclones develop further downstream often close to the eastern North Atlantic (Priestley et al., 2020b), and the orographically driven cyclones dominate in the Mediterranean basin (Trigo et al., 1999).
The most common conceptual models to describe extratropical cyclone development are the Norwegian and the Shapiro–Keyser models (Bjerknes, 1922; Schultz et al., 2019; Dacre, 2020). According to the Norwegian model, a stationary front forms between cold and warm air, initiating strong vertical wind shear within the troposphere. A front is a density discontinuity and, hence, separates cold and warm air masses. Typically triggered by an upper-level trough, a cyclone begins to grow along this front where it develops a warm and a cold front. As the cyclone deepens, both fronts become better defined and a warm sector develops. When the cold front catches up to the warm front, the so-called occlusion process starts. At this stage, the cyclone reaches its most intense period (Bjerknes, 1922), followed by cyclone decay. In the Shapiro–Keyser model, the initial development is similar, but the cold front does not overtake the warm front, but rather it builds a T-bone structure (see Fig. 16–24 of Schultz et al., 2019) instead of a narrowing warm sector during occlusion as in the classical model (Shapiro and Keyser, 1990).
Windstorms produce winds which are strong enough to cause damage; they typically have wind speeds in excess of 15 m s−1 (Wallace and Hobbs, 2006). In order to quantify the impact of windstorms, it is important to know the parts of a storm where the strongest wind speed typically occurs. There are three zones where strong winds can occur: the warm jet, the cold jet and the sting jet (Clark and Gray, 2018). Hewson and Neu (2015; see their Fig. 1) have developed a conceptual windstorm model to describe how strong winds may develop associated with the passage of a cyclone during different stages of its development. In most cases, the strongest winds are often associated with the passage of the cold jet at the cold front. However, Shapiro–Keyser cyclones may on occasion feature sting jets, which, if they reach the surface, may lead to even more damaging wind speed (Clark and Gray, 2018).
The potentially most damaging events affecting Europe are commonly assigned to slow movers, rapid developers or serial storms (Mailier et al., 2006). Slow-mover cyclones lead to large accumulations of precipitation in the same area, often triggering severe flooding (Grams et al., 2014). Rapid developers are fast deepening cyclones, often fulfilling the conditions for a “bomb” (Gyakum and Danielson, 2000). When occurring close to Europe, many of these are secondary cyclones. Finally, serial storms (also known as cyclone families) indicate that multiple and related cyclones affect the same area within a comparatively short period of time, leading, potentially, to severe cumulative losses (Mailier et al., 2006; Pinto et al., 2014). In these clustering periods, the passage of storms occurs more frequently than may be expected if they would occur independently from each other (Vitolo et al., 2009; Franzke, 2013; Blender et al., 2015). Two physical reasons are given in the literature (Economou et al., 2015; Dacre and Gray, 2020): (i) the steering through the large-scale flow, typically characterized by an intensified, quasi-stationary jet stream extending towards Europe and (ii) the occurrence of secondary cyclogenesis.
2.3 Spatial characteristics of storms
To analyse cyclones and storms, objective identification and tracking methods are needed (Ulbrich et al., 2009; Neu et al., 2013). This leads to a Lagrangian perspective where certain properties during the life cycle of the cyclone can be defined by, for example, radius, propagation speed, and spatial wind distribution. Various objective methods for the identification and tracking of extratropical cyclones have been used to investigate their characteristics (Neu et al., 2013; Priestley et al., 2020b).
In the North Atlantic–European region, cyclone track densities show maximum values over the western North Atlantic with a second maximum over the Mediterranean (Ulbrich et al., 2009; Pinto et al., 2005). North Atlantic cyclone activity shows a tilt towards the northern North Atlantic. While this can be found in different reanalysis products, Coupled Model Intercomparison Project phase 5 (CMIP5) simulations are characterized by a bias of the maximum and tilt in the North Atlantic, leading to more zonally oriented storm tracks (Zappa et al., 2013). While many cyclones can be identified in the extratropics, only a subset of strong cyclones lead to a high wind speed. See Sect. 4.1 for related storm indices.
2.4 Large-scale circulation characteristics and their impact on wind
Storms and their related wind gusts are local in nature. Nonetheless, the large-scale background circulation can still provide information in which areas strong winds are likely to occur. Here, we apply the concept of atmospheric weather regimes (Hannachi et al., 2017) to determine the characteristics of the large-scale circulation. Atmospheric weather regimes are recurrent, dynamically relevant circulation patterns and allow the description of low-frequency variability due to transitions between distinct regimes. Because of their preferred occurrence locations, they potentially provide prediction and downscaling possibilities for smaller-scale weather events and extremes (Cassou et al., 2005).
To demonstrate the relation of specific regimes to preferred jet stream patterns and storms, we show weather regimes based on sea level pressure fields from the latest European Centre for Medium-Range Weather Forecasts Reanalysis (ERA5) (Hersbach et al., 2020) over the North Atlantic–Eurasian region (30–90∘ N, 90∘ W–90∘ E) for winters (December through March, DJFM) from 1979–2020. The details of the applied regime analysis are described in Crasemann et al. (2017). We identify five regime states (Fig. 1a): (1) Scandinavian–Ural blocking (SCA-URAL BL), (2) the North Atlantic Oscillation in the positive phase (NAO+), (3) blocking over the North Atlantic (ATL BL), (4) North Atlantic trough (ATL TR) and (5) the NAO in its negative phase (NAO−).
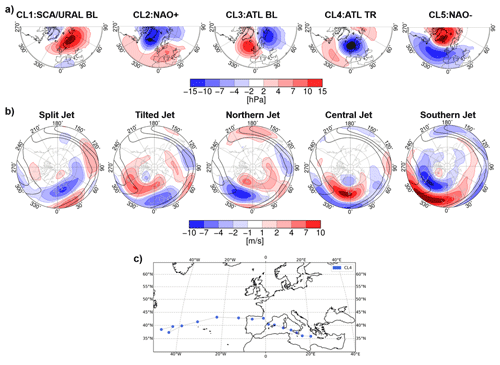
Figure 1(a) Weather regimes determined from ERA5 reanalysis data for December to March (DJFM). Shown are the regime patterns in terms of sea level pressure anomalies (shading); black contours indicate the climatology for DJFM. Shown are isolines at 1000, 1005, 1010 and 1015 hPa. (b) The jet stream patterns are associated with the individual weather regimes, obtained by composites of zonal wind anomalies at 250 hPa (shading). Black contours indicate the zonal wind climatology for DJFM. Shown are isolines at 20, 30 and 40 m s−1. (c) eXtreme Wind Storms XWS database (Roberts et al., 2014) track for Storm Klaus based on ERA5 data, identified with the method of Leckebusch et al. (2008). Storms are defined by the exceedance of the local 98th percentile of near-surface wind speed. Each dot represents the position of the wind field centre of Storm Klaus for 6 h time steps from 22 January 2009 at 06:00 local time (LT) to 26 January 2009 at 00:00 LT. The colour of the dots shows the weather regime of that date.
The characteristic patterns for the jet stream associated with these weather regimes have been obtained by compositing the zonal wind anomalies at 250 hPa over the days assigned to each regime. The five jet stream patterns (Fig. 1b) are very similar to those obtained by previous studies (Dorrington and Strommen, 2020; Woollings et al., 2010; Franzke et al., 2011).
The regime analysis assigns the atmospheric circulation of each day over the period 1979–2020 to one specific cluster and enables a characterization of the large-scale background for specific windstorm events. As one example, Fig. 1c shows the eastward movement of the extreme storm Klaus from 22 to 26 January 2009 along an unusual southerly path. The storm Klaus was characterized by strong and record-breaking wind speed over northern Iberia and southern France. During the formation, intensification, and eastward movement of Klaus, the Atlantic trough weather regime associated with the central jet stream configuration prevails (Fig. 1c). This central jet stream pattern sets the necessary large-scale background flow for the development and movement of this extreme storm (Liberato et al., 2011).
The concept of weather regimes enables the characterization of the large-scale atmospheric circulation, in particular the jet stream pattern, during extreme storm events. If changes in the occurrence of these extremes can be related to an anomalous frequency of occurrence of a specific weather regime, the use of these regime states offers potential predictability of large- as well as small-scale wind impacts.
2.5 Temporal characteristics of storms and seasonal variability
The occurrence of extreme wind speed and storms is subject to a strong seasonal pattern in Europe. According to Young et al. (1999), windstorms occur 30 % more frequently in winter than in summer (see also Fig. S1 in the Supplement). We compared the wind gusts from three reanalysis products (ERA5, Hersbach et al., 2020; COSMO-REA6, Bollmeyer et al., 2015; and COSMO-REA2, Wahl et al., 2017) to 145 German station observations (Kaspar et al., 2013). While a direct comparison is difficult, qualitative statements on seasonality can be made with all data sets. The number of occurrences of wind gusts is determined for certain wind speed intervals, which are shown against the warning levels (WLs) of the Deutscher Wetterdienst (DWD). The warning levels are defined by six different wind speed thresholds: 14, 18, 25, 29, 33 and 39 m s−1 (Primo, 2016), referring to four WLs (WL1–WL4), with WL2 and WL3 being divided into two intervals (DWD, 2021). Compared to observations, wind gust frequencies are underestimated in reanalyses. The higher the wind gusts, the higher the underestimation. Therefore, COSMO-REA2 shows a significantly better agreement with the reference, especially for WL3 in summer and WL4 in winter. The benefit of the higher resolution provided by regional reanalyses compared to their global counterparts is well documented for near-surface wind speed (Niermann et al., 2019). Results shown in Fig. S1 emphasize the importance of using high-resolution models to represent extreme wind gusts in reanalysis products.
Above 25 m s−1 there is a clear difference between summer and winter months, which becomes stronger the higher wind speed is considered. In summer, wind speed over 30 m s−1 does not appear in the coarser reanalysis products ERA5 (∼30 km) and COSMO-REA6 (∼6 km) at all, and for the high-resolution reanalysis COSMO-REA2 (2 km) and the point observations the occurrence of wind gusts of WL3 or WL4 in summer is smaller than in winter by a factor of 10 to 100.
The intra-annual variability is not only visible in meteorological data but also in loss data from insurance companies (Hoeppe, 2016; Franzke, 2017), which shows the strong impact of storms and especially winter storms on society and economic areas (Klawa and Ulbrich, 2003). The energy sector is strongly affected by the occurrence of windstorms, especially their seasonal variability. Due to the worldwide effort to convert the energy system to renewable sources, the industry will have to deal more with seasonal fluctuations in energy availability. The interest and the need for precise knowledge of the wind conditions in various regions is therefore growing, as energy production directly depends on it; for more details about wind-based energy production please see Sect. 4.6.
2.6 Winds induced by convective activity
Most of the wind damage in temperate latitudes is due to extratropical cyclones. However, damage can also occur to structures, crops and forests from winds produced by convective storms (Gatzen et al., 2020; Parodi et al., 2019); since our focus is more on extratropical storms, we keep this part rather brief. The following conditions need to be met for convection to occur (Wallace and Hobbs, 2006): (1) the atmosphere needs to be conditionally unstable, (2) there needs to be a reservoir of substantial moisture in the boundary layer, and (3) there needs to be sufficient lifting due to low level convergence to cross the threshold to start the instability.
Convective systems and storms can lead to severe wind speed connected to tornadoes, gust fronts and downbursts (Wallace and Hobbs, 2006). Tornadoes are rapidly rotating air systems which connect with the ground and can lead to devastatingly strong winds. Downbursts are downward directed winds due to the negative buoyancy of the downdraught air. Convective storms can also have gust fronts. The gust fronts form due to downdraughts in the convective storm forming a pool of cold, dense air which replaces the warmer, buoyant air of the environment.
These downdraughts can lead to severe wind gust speeds at the surface (Bunkers and Hjelmfelt, 2021) with speeds of up to 42 m s−1. So far, relatively little attention has been paid to wind damage to infrastructure, forests and agriculture from such events besides the studies by Jim and Liu (1997) and Peterson (2000). Forest damage from thunderstorms in areas which previously were rarely affected, such as eastern parts of Europe (Nosnikau et al., 2018; Sulik and Kejna, 2020), has experienced an increase in convectively available potential energy and near-surface moisture which can cause more thunderstorm activity (Taszarek et al., 2021). It is expected that anthropogenic global warming will lead to an increase of convective storms (Lepore et al., 2021; Taszarek et al., 2021; Diffenbaugh et al., 2013).
Another type of convective storm is derechos, which are a clustering of downbursts, organized by a line of thunderstorms (also called a squall line) that lead to widespread straight-line winds, and can cause damaging winds. They occur frequently in the Great Plains area of the USA (Ashley and Mote, 2005) but can occur around the world, including central and eastern Europe (Gatzen et al., 2020). Some examples of the devastating impact of derechos on forests are described in Goff et al. (2021) and Negrón-Juárez et al. (2010).
3.1 The physics of fine-scale interactions between surfaces and wind
The characteristics of the wind speed and gustiness in a given environment are dependent on surface characteristics, such as its roughness, all of which are highly influential on the levels of damage caused. The momentum of the mean horizontal wind is vertically transferred by turbulence; i.e. near the surface, large whirling air packages break up into smaller ones and their momentum dissipates into thermal energy or is absorbed by roughness elements, such as trees and buildings. The strength of the wind is altered by topography and the roughness of the surface (Stull, 2017; Kaimal and Finnigan, 1994; Finnigan et al., 2020). Thus, the damage level can vary dramatically at small scales (Gromke and Ruck, 2018; Forzieri et al., 2020).
Typically, the boundary layer above the Earth's surface is subdivided into three sublayers: (1) a roughness sublayer that is characterized by the flow around obstacles and varies locally and where mechanical turbulence dominates; (2) one or more inertial sublayers, where the influence of the individual obstacles and surfaces is blended together and the vertical energy fluxes are constant with height; and (3) a mixing layer above, where the Coriolis force gains influence and is often separated from the free atmosphere by a capping inversion and an entrainment zone (Stull, 1988; Kaimal and Finnigan, 1994). The effect of buoyancy and thermal stability is very important for the formation of strong winds, i.e. for cyclones and thunderstorms. During storm events, high wind speed increases friction within the lower boundary layer and also increases form drag by obstacles. The instability of the shear in the flow created by the drag of the surface leads to turbulence, which affects the vertical exchange of mass, momentum and scalars. Thermal gradients near the surface are reduced or disappear due to this mixing, which results in neutral stratification near the surface; i.e. thermal stability need not be considered in the equations of the vertical wind profile (Stull, 1988).
As turbulent movements play a major role in the momentum transfer to the surface, it is important to regard shear forces and gustiness as the damaging characteristics of the wind field (Gromke and Ruck, 2018). For example, in forest ecosystems trees are blown down at a mean wind speed considerably lower than those estimated by pulling experiments (Oliver and Mayhead, 1974; Milne, 1991). Boundary layer eddies create a local increase in wind speed and wind shear close to the surface (Romanic and Hangan, 2020) and leading to coherent eddies (Raupach et al., 1996). The loading due to these turbulent structures with higher energy and momentum can be accounted for in a gust factor (Hale et al., 2015; Chen et al., 2018; Holland et al., 2006; Usbeck et al., 2010). Since trees react to gusts like damped harmonic oscillators (Mayer, 1987; Gardiner, 1992), there has been considerable debate about whether the arrival frequencies of these coherent eddies could lead to resonant failure (Gardiner, 1995; Peltola, 1996); however, this does not happen (Schindler and Mohr, 2019; Schindler and Kolbe, 2020; Kamimura et al., 2022), probably due to the efficient damping of trees (Spatz and Theckes, 2013). Besides the drag force of a plant (Rudnicki et al., 2004; Queck et al., 2012; Vollsinger et al., 2005), the level of damage depends also on the acclimation of plants to the wind (Telewski, 1995; Nicoll et al., 2019), which is a function of the maximum wind speed (Bonnesoeur et al., 2016; Dèfossez et al., 2022). They are adapted to wind forces and build stronger roots and wood structures depending on the main wind direction and magnitude (Nicoll and Ray, 1996; Tomczak et al., 2020).
Furthermore, the development of turbulence above and within the canopy is different between naturally uneven aged woods and managed forests or plantations. Experiments showed that the inflection of the wind profile (i.e. maximum gradient of wind speed) is weaker in heterogeneous compared to homogeneous canopies and that it occurs deeper within the canopy; i.e. the displacement height is lower (Cionco, 1972; Belcher et al., 2012; Queck et al., 2016). Furthermore, homogeneous forests are more vulnerable than naturally uneven aged woods (Everham and Brokaw, 1996; Mitchell, 2013). Obviously, the adaptation to wind stress is not restricted to single trees but extends to the structure of natural mixed woods too. The characteristics of the tree (height, diameter, canopy size, wood properties) and the tree resistance to uprooting and breakage are all affected by the level of wind exposure (Gardiner et al., 2016). Recent experimental measurements of tree damage during a super typhoon (Kamimura et al., 2022) have also shown that collisions between the crowns of individual trees and the crowns of their neighbours are extremely important in reducing tree movement during strong winds and contributing to their overall stability. These adaptations of plants to living in a windy environment must be considered when modelling the risk of wind damage to tree stands.
Large eddy simulations (LESs) are used to better understand the complex current patterns and the acting wind forces near heterogeneous surfaces (Stoll et al., 2020; Takemi et al., 2020). These turbulence-resolving models include all the basic physical equations; however, they require considerable computer resources and are therefore unsuitable for operational use. Simplified mechanistic models (Holland et al., 2006; Gross, 2018; Duperat et al., 2021) parameterize the turbulence spectrum and operate on a larger spatial scale and thus need fewer computational resources. Statistical approaches (Jung and Schindler, 2015; Dupont, 2016) focus on predicting critical thresholds at which wind damage occurs and are therefore efficient for operational damage prediction. The indices discussed in Sect. 4 are based on empirical observations and have proven useful in a wide range of applications.
3.2 Mean wind and gust rates for different landscapes
The gustiness of the wind is critically important for assessing the likely impact of strong winds on forests, agriculture and structures (Usbeck et al., 2010; Gardiner et al., 2016). The level of gustiness is known to be influenced by surface roughness (Table 1), the height above the ground and wind speed (Ashcroft, 1994; Verkaik, 2000). Gust ratios are also affected by wind speed (see Born et al., 2012; their Fig. 2) and by the type of storm (Krayer and Marshall, 1992; Harper et al., 2008).
Table 1Wind rate (mean/gusts) for different landscapes; 3 s gust to 10 and 60 min mean wind at 10 m height, by terrain category. From Ashcroft (1994). Roughness classes: 1 is off-sea wind onto flat coastal areas; 2 is level grass plains (e.g. marsh); 3 is standard category (fairly level terrain– mostly open fields with a few houses and buildings); 4 is fairly level terrain with more hedges, trees and villages, farm buildings; 5 is many trees and hedges, or fairly level wooded country or more open suburban areas.
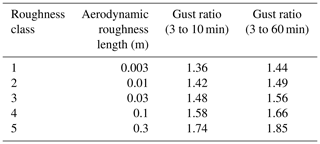
Wind- and storm-related indices and thresholds are a vital tool in assessing the likelihood and magnitude of damage. While there are many definitions for indices and thresholds, here we define an index as a number or a category, serving as an aggregated measure of a quality, which can be reached by means of observation, arithmetic calculation or different modelling techniques. A threshold is defined here as a value taken or calculated from a numerical or a categorical range, and when the threshold value is crossed, it indicates a significant increase in the probability for an event to take place or for a certain condition to be fulfilled. Indices can be used to predict damage caused directly by wind or a storm or when wind modulates the damage caused by another process such as fire or drought. Since indices and thresholds can be as effective as complex mechanistic models but more cost-effective, it is of no surprise that there is a plethora of indices. There are general indices that are not bound to a given sector or environment, but many of the indices and thresholds available require a careful selection process according to the target ecosystem. Below we provide an extensive review of available indices, focusing on five key terrestrial sectors.
4.1 General storm indices: scale and severity indices
Classical wind scales are defined by phenomena caused by the interactions between wind and the surface. A very prominent example is given by the Beaufort scale (Stull, 2017). It classifies the effect of wind on wave generation, tree movement and the damage of buildings. Similar scales exist for tornados, e.g. the Fujita scale and the Torro scale (Kirk, 2014), which relate the tornado intensity to damage description. As short gusts and shear forces are very important factors of storm risk, the Enhanced Fujita scale includes further information on derived maximal tangential 3 s gust speeds (Fujita, 1981). Recently an improved wind speed scale and damage description has been suggested for central Europe (Feuerstein et al., 2011). Finally, the Saffir–Simpson hurricane wind scale (Ellis et al., 2020) is based on the highest wind speed averaged over a 1 min interval 10 m above the surface. It can provide some indication of the potential damage a hurricane will cause upon landfall.
Several storm severity indices have been developed to quantify the severity of a windstorm regardless of the land cover. These indices are used to identify severe winter storms and analyse their impacts and to investigate storm trends in past and future climate conditions. They often include the cube of the wind speed, assuming a proportionality of the dissipation rate of the wind kinetic energy to damage. A selection of these indices is presented in Table S1 in the Supplement.
From an historical context, one of the earliest storm severity indices was developed by Lamb (1991) to grade and rank storms based on the greatest observed wind speed over land, the area affected by damaging winds and the overall duration of occurrence of damaging winds. Later, in a study by Klawa and Ulbrich (2003), the wind speed values were scaled with the local 98th percentile. Based on this approach, Leckebusch et al. (2008) identified and tracked windstorms in time and space and computed an event-based storm severity index that quantifies the potential impact of a storm. This index considers the relation of the maximum daily wind speed to a certain local percentile of maximum daily wind speed (e.g. the 95th or 98th) as well as the affected area. For example, in their study they found a trend for an increase in severity of storms during 1960–2000 and for 2070–2100 under anthropogenic climate change conditions. Pinto et al. (2012) extended this approach by taking into account the exposure and including local population levels in a loss index, resulting in the finding that the maximum storm losses for current climate conditions are likely to be exceeded in the future. Additionally, Haylock (2011) used a storm severity index to identify the severest storms for 72 h storm footprints. This index considers the latitude and the excess of the maximum wind speed over a 72 h period taken from six-hourly values over a threshold (e.g. the local 90th percentile of wind speed).
4.2 Forests
4.2.1 Topographic indices
Many topographic indices have been used for assessing the risk of wind damage to forests (see Table S2). These indices can be based on elevation, slope characteristics such as compass angle, aspect, and curvature, or are more complex such as TOPEX (Topographic exposure; Quine and White, 1998), which was developed as part of a risk assessment method (Windthrow Hazard Classification) to predict the height at which trees could be expected to be first damaged (Miller, 1986). TOPEX is the sum of the angle to the horizon in the eight principal points of the compass and can be calculated for different distances from the point of interest. Furthermore, such indices can be used to create even more complex predictive systems. For instance, when TOPEX is combined with elevation and aspect it produces a system called DAMS (Detailed Aspect Method of Scoring; Quine and White, 1993) for predicting wind speed variation in the landscape. This system is entirely based on topographic measures and compares favourably with modelling systems based on solutions of the fluid equations (Suárez et al., 1999).
The actual variation of wind speed with height above the ground is a function of the surface roughness and the topography. Predicting variations of wind speed across flat surfaces is relatively straightforward, especially for strong winds by using a measure of the aerodynamic roughness of the surface and a logarithmic wind profile (Garratt, 1980; Stull, 1988). Even in stable or unstable conditions the profile can be modified with the addition of the diabatic term ψm (Kaimal and Finnigan, 1994; Panofsky and Dutton, 1984). Often the roughness of the surface is simplified into different roughness classes (Troen and Petersen, 1989) to allow for easier estimation of the surface roughness. However, when even strong winds flow over topography the simple logarithmic profile breaks down and the shape of the wind profile strongly varies between the upwind slope, the crest of the hill and the downwind slope, where the flow may even separate (Belcher et al., 2012). Thus, one should not only calculate topographic indices for the target locations but calculate also for the neighbouring areas and assess the change in value between the target location and its surroundings (Ruel et al., 1997; Schindler et al., 2012; Murshed and Reed, 2016).
We reviewed the literature, focusing on studies using topographic indices to assess and predict damage caused by strong winds, as topographic indices are a common feature in modelling wind damage in forests (Table S3). The most commonly used variables were (Fig. 2) elevation, slope, aspect and TOPEX. We assessed the usefulness of the four most used topographic indices in modelling forest damage according to their inclusion in final models and according to the importance/influence metrics reported. We note that most studies employed a multivariate modelling approach; thus, a certain variable may appear less useful due to overlap in the variance explained with another variable, but not necessarily due to the variable's lack of explanatory power (Scott and Mitchell, 2005). Furthermore, there are other topographic indices that have not been tested so far for their contribution in forest damage prediction (see Florinsky, 2017).
Elevation was useful in about a third of the studies and was particularly useful when the study area was very large, encompassing an entire region, state or country (Díaz-Yáñez et al., 2019; Kramer et al., 2001; Torun and Altunel, 2020; Mayer et al., 2005) or when there was a strong gradient of elevation, preferably reaching above 900 m above sea level (Krejci et al., 2018; Pasztor et al., 2015; Torun and Altunel, 2020; Kramer et al., 2001; Mayer et al., 2005). The trend in the correlation between elevation and forest damage was found to be inconclusive, to be both positive (Díaz-Yáñez et al., 2019; Krejci et al., 2018; Pasztor et al., 2015) and negative (Mayer et al., 2005; Albrecht et al., 2013), or only present for a certain range of elevation (Albrecht et al., 2013; Torun and Altunel, 2020). While there is an expectation for an increase in forest damage with higher elevation due to an increase in wind speed (Machar et al., 2014), diversity of trends can stem from the involvement of other topographic indices that may contain similar information (e.g. slope or TOPEX), and also due to varying levels of acclimation of trees to the wind conditions present at different elevations (Gardiner, 2021).
The slope was shown to be useful in about half of all articles; however it is difficult to observe a clear relation to forest damage. In articles that identified a contribution of slope, the relation of damage with slope was found to be either positive (Díaz-Yáñez et al., 2019) or negative (Mayer et al., 2005; Morimoto et al., 2019; Schütz et al., 2006). But an important deciding factor can be the aspect of the slope (useful in about 40 % of all articles) as there is often an interaction between the two (Suvanto et al., 2018, 2016; Díaz-Yáñez et al., 2019; Hanewinkel et al., 2014). In this sense, the aspect likely indicates the forest's susceptibility to wind coming from a certain direction, as in most cases of usefulness of aspect, the slope was also useful. Finally, TOPEX was found by 77 % of articles as useful, and when a trend was reported, all studies reported higher damage or probabilities for forest damage being associated with more exposed locations (Albrecht et al., 2012, 2013; Jung et al., 2016; Morimoto et al., 2019; Mitchell et al., 2001; Taylor et al., 2019). One of the reasons for TOPEX's usefulness is that it does not strongly overlap with the information contained in other wind-based variables (Albrecht et al., 2019; Schindler et al., 2012). However, when TOPEX is calculated only for a certain cardinal direction (e.g. west) it contains information that is very similar to aspect.
4.2.2 Fine-scale wind and surface interactions
Interactions between the surface and the wind field are controlled by surface roughness, absolute wind velocity and atmospheric stability. The commonly used index related to surface interactions that is relevant for wind and storm damage is the critical wind speed (CWS). The CWS defines the threshold wind speed for overcoming the maximum resistance to stem breakage or uprooting of a tree (Gardiner et al., 2016; Peterson et al., 2019; Hale et al., 2015; Chen et al., 2018; Holland et al., 2006). CWS is a standard term in forest ecology. The typical averaging interval for CWS is a period of a few minutes, e.g. 3 min (Peltola and Kellomäki, 1993), 10 min (Dupont et al., 2015; Peltola et al., 1999) or 60 min (Hale et al., 2015). CWS is estimated either at a height of 10 m above the canopy or at the tree top at the stand edge.
One of the governing quantities to describe the interactions between wind forces and stem breakage or uprooting is the applied maximum bending moment (BMmax) (Quine et al., 2020), which is the sum of wind forces in the tree crown and the additional turning moment due to stem bending and deflection of the stem and crown of a tree (Peltola, 2006). BMmax calculation refers typically to the mean bending moment (BMmean) and a gust factor (see e.g. Gardiner et al., 1997). A tree uproots if its BMmax at the ground level exceeds the resistance of the root–soil plate, and a tree breaks if its BMmax at breast height (1.3 m) exceeds the critical value of the stem's modulus of rupture (Peltola et al., 1999; Quine et al., 2020). The gust factor is parameterized by wind measurements (field or wind tunnel) and depends on the spacing / height ratio of tree stands and the location relative to the forest or stand edge (Gardiner et al., 1997; Quine et al., 2020). The wind measurements are taken from the top of the canopy, and the bending moment is typically determined from the level of zero-plane displacement (e.g. 0.8 of the tree height; Gardiner et al., 1997). Nevertheless, measurements of the effects (Gardiner et al., 1997) as well as directly solved finite element models of the crown architecture (Ruy et al., 2022) have shown the influence of crown architecture on the maximum bending moment. Therefore, the gust factor used in the calculation of BMmax may need to be varied according to stand composition and tree type.
The probability of occurrence of CWS, as a measure of storm damage risk for specific forest stands, depends on the statistics of wind velocity, e.g. on hourly maximum synoptic winds (umax: Usbeck et al., 2010, Chen et al., 2018) or maximum geostrophic wind speed (Blennow and Olofsson, 2008). CWS is used to parameterize impact models for the estimation of storm risk in forests such as ORCHIDEE-CAN (Chen et al., 2018), SWAN+ADCIRC (Akbar et al., 2017), GALES and HWIND (Peltola et al., 1999; Gardiner et al., 2000, 2008).
The key parameter in the calculation of CWS is the diameter at breast height (DBH), which is a standard parameter in forest inventories. DBH is commonly defined as the stem diameter at 1.3 m above the ground (Peterson et al., 2019; Gardiner, 2021; Hale et al., 2015; Chen et al., 2018; Holland et al., 2006; Hanewinkel et al., 2014; Beck and Dotzek, 2010; Gardiner et al., 2008; Peltola, 2006). DBH is the most used structural parameter due to its easy and practicable measurement and due to its widespread application in forest management (Liu et al., 2018). DBH is also used to derive other structural parameters like tree height and leaf area index (LAI), which can also be derived from normalized difference vegetation index (NDVI) as a standard product of satellite remote sensing. These structural quantities are important both for statistical analysis and for the parameterization of storm risk models.
Other important parameters for calculating CWS are the mean drag coefficient (cd), which is part of the equation of the drag force (Vogel, 1989; Akbar et al., 2017; Dupont et al., 2015), turbulence intensity, gust duration (Hale et al., 2015; Chen et al., 2018), tree density (Peterson et al., 2019; Albrecht et al., 2015), tree height, crown projection area and crown volume (Peterson et al., 2019; Gardiner et al., 2010, 2021; Albrecht et al., 2015; Hale et al., 2015; Chen et al., 2018; Dupont et al., 2015; Peltola, 2006), and tree species (Hanewinkel et al., 2014). Additionally, the edge factor index describes the influence of a tree's position relative to a forest edge, the shape of the forest edge and the width of any upwind gap (Chen et al., 2018; Gardiner et al., 2010; Peltola, 2006).
The severity of storm damage depends on the ability of a tree to resist the applied bending moment from the wind and on the stability of the root soil complex (Nicoll et al., 2006). If soil water content is close to saturation the critical resistive moment of trees (BMcrit) can be reduced significantly during storm events, which could become increasingly important with the increasing frequency of heavy winter rain in temperate forests in the context of regional climate change (Défossez et al., 2021).
The uncertainty of CWS results from the consecutive solving of analytic equations including accumulated uncertainties of the different input quantities. Additional uncertainties result from the differences in the models used. Sensitivity tests using GALES (Locatelli et al., 2017) and HWIND with a variation of the input parameters of ±20 % lead to a more than 20 % change in CWS. For example, CWS is especially sensitive to changes in DBH. The measurement uncertainty of the DBH ranges between 2 % and 10 % depending on the absolute diameter (Qin et al., 2019). Applied in HWIND and GALES the variation of DBH of ±20 % leads to changes of CWS of +30 % and −46 % (Gardiner et al., 2000). The most comprehensive analysis of wind risk model uncertainty was made by Locatelli et al. (2017), who found that tree DBH, tree height and inter-tree spacing were the most critical factors.
4.3 Urban areas
4.3.1 The urban boundary layer
The small-scale interactions of the wind field with urban surfaces are significantly different from natural surfaces due to high three-dimensional (3D) variability of impermeable artificial obstacles (buildings). These differences lead to a higher mean surface roughness of the urban surface (Grimmond and Oke, 1999; Oke et al., 2017) combined with a general attenuation of the mean wind speed, the wind speed averaged over some time period (Chen et al., 2020), as compared with more natural surfaces. The level of increase in roughness depends on the morphology – density, size and composition – of the obstacles along the flow direction. The height of the roughness layer is 2–3 times the mean height of the buildings. Within this layer, mechanical turbulence generation dominates, and average wind profiles can only be assumed above the roughness layer, within the inertial sublayer. The averaged roughness of an urban surface is described by roughness length z0 within equations for vertical wind profiles. This parameter serves as a useful index for the prediction of turbulent impulse transfer and for damage prediction, which are derived based on building height, areal fraction and frontal area index (Grimmond and Oke, 1999). At finer scales, wind speed shows high spatio-temporal variability. Thus, when using indices based on averaged wind speed, it is also important to consider that due to the small-scale aerodynamic and thermal heterogeneities of urban infrastructure (buildings and trees), the local magnitude of the wind speed is temporarily larger than under rural conditions (Droste et al., 2018). The reasons for this anomaly are again the inflexibility and impermeability of technical structures and buildings. These features cause canalization of flows and stronger turbulence generation compared to natural surfaces. There is also a diurnal–nocturnal distinction in the formation of local thermal wind systems, with street canyon wind during the day and a nocturnal inflow to the urban heat island (Droste et al., 2018; Lindén and Holmer, 2011). Thus, indices in urban areas should account for both spatial and temporal heterogeneities.
4.3.2 Indices for estimating damage to individual buildings
Damage occurs either directly by wind pressure or indirectly by the impact at high speed of objects and debris moved by the wind (Tamura, 2009). At the level of individual buildings, air movement results in wind pressure on the building surface and an applied force. Damage to buildings caused by extreme wind loads includes resonance- and vibration-induced damage, damage to roof tiles or sheet roofing, roof lift-off, and the collapse of walls or entire houses.
The occurrence and type of damage depend on the level of exposure as well as the structural vulnerability of the individual buildings to severe local winds. The European wind loading code EN 1991-1-4 regulates how to adapt the structural design of buildings to the local wind climate. The code defines basic wind velocities for different geographical wind zones based on the 50-year return level of 10 min wind speed at a 10 m height. In Germany, for example, the basic wind velocities range from 22.5 m s−1 in wind zone 1 (inland areas in southern Germany) up to 30 m s−1 in wind zone 4 (coastal areas). The basic wind velocities are further adjusted based on the height above ground and the terrain roughness to account for short-term wind fluctuations. Terrain roughness is classified in five categories ranging from coastal areas to cities with a high building density. Additionally, where topography (e.g. hills, cliffs) increases wind velocities by more than 5 %, the effect is taken into account using a topographic index, as the ratio of the mean wind velocity at the height above the terrain to the mean wind velocity above flat terrain. Finally, the wind speed is used to compute the local peak velocity pressure, which is a fundamental index for the determination of all wind loads for a specific building (Schmidt, 2019). Nonetheless, assigning critical wind speed thresholds to building damage is rather difficult given the heterogeneity of buildings, topography and land cover.
4.3.3 Storm loss models: estimating damage on a district level
Often there is little to no information on the actual damage to individual buildings or small-scale urban structures. Instead, storm loss models come into play, and they relate wind speed to actual building damage data, usually by applying statistical modelling techniques. In some cases, these models rely on the use of wind indices like the exceedance of local wind speed over a critical threshold to calculate monetary loss. In other cases, the model itself calculates a damage index. The purpose of storm loss models is, among other things, to assess current risk to residential structures or to estimate expected losses in future climate conditions. It is often assumed that the maximum daily gust speed (24 h maximum) is the most influential factor compared to other wind parameters like daily mean wind speed or wind direction and is commonly used in indices as well as in loss models (Donat et al., 2011; Klawa and Ulbrich, 2003; Koks and Haer 2020; Leckebusch et al., 2008; Pardowitz et al., 2016; Welker et al., 2021).
Building damage data on a district level are usually provided by insurance companies and are analysed in the form of the loss ratio, which is the amount of insured loss per day and district, divided by the corresponding sum of insured value, or claim ratio, which is the number of affected insurance contracts per day and district, divided by the corresponding total number of insurance contracts (Prahl et al., 2015).
The functional relationships between wind and damage are usually referred to as damage functions. As the relationship between damage and wind depends strongly on local conditions like building or city structure, there is no universal function or model, and instead a variety of different damage function formulations are in use. A detailed overview can be found in Prahl et al. (2015). Power-law damage functions are common. Different exponents for these functions can be found in the literature ranging from 2 to 12 (Münchener Rückversicherungs-Gesellschaft, 1999; Heneka et al., 2006; Prahl et al., 2012). Some damage functions also assume an exponential form (Prahl et al., 2015).
Another type of model is probabilistic models which calculate the probability that a certain loss threshold is exceeded (Pardowitz et al., 2016; Prahl et al., 2012). Some examples of existing models are shown in Table 2. Most models still need to be fitted to local conditions and validated with existing damage data. Model selection depends on the available data.
Table 2A selection of damage functions including exponential damage relationships (Dorland et al., 1999), power law damage functions (Klawa and Ulbrich, 2003; Heneka et al., 2006) and probabilistic damage functions (Pardowitz et al., 2016). a and b denote coefficients, D a damage index, f(vcrit) a normal distribution of the critical wind speed, G a damage ratio, L a loss, LR a loss ratio, P(LR>th) a probability that a certain loss threshold will be exceeded, th a loss threshold, v a mean daily wind speed, v98 the 98th percentile of the local wind speed, vcrit a critical wind speed at which buildings are assumed to suffer damage (comparable to the CWS used for trees), vmax the maximum daily gust speed, and vtot the building total wind speed at which maximum damage is reached.
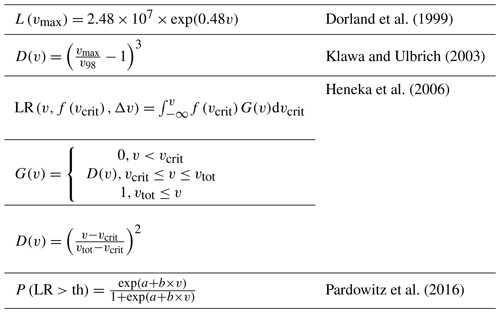
4.4 Transport
Transport systems are the backbones of modern societies. Disruptions within the transport systems can have serious cascading effects that can cause large costs. Weather in general, and windstorms in particular, can affect all aspects and functions of transport systems (Leviäkangas et al., 2011). However, relevant thresholds of wind speed and their impacts are different depending on the mode of transport. Vajda et al. (2014) identify three wind gust thresholds of increasing magnitude, which they relate to general impacts and consequences within different parts of the European transport system:
- i.
During wind gusts >17 m s−1, adverse impacts on the transport system may start to occur, especially if the resilience of the exposed part of the system is low, but disruptions are rather local. For example, some windthrow of trees can occur along railways and roads, leading to local problems with road and rail traffic. Furthermore, operation of smaller boats could be suspended due to reduced manoeuvrability.
- ii.
During wind gusts >25 m s−1, some adverse impacts can be expected, such as windthrow and electricity cuts occurring on a larger scale. In addition, delays and cancellations in air, rail, road traffic and disturbances of ferry traffic can be expected.
- iii.
During wind gusts >32 m s−1, adverse impacts are very likely to occur, and windthrow of trees can be expected on a large scale, leading to long-lasting power failures and delays, and cancellation of rail and road traffic. Furthermore, damage to traffic control devices and structures can occur, airports can be closed, and ferries stay in harbour due to reduced visibility and high waves.
The effect of wind on road safety is not extensively explored in the literature (Theofilatos and Yannis, 2014). In general, the number of road vehicle crashes caused by strong wind is small compared to the total number of crashes (Edwards, 1998). However, studies have identified specific types of crashes which typically occur under strong wind conditions: overturning, side slip and rotation crashes (Baker, 1986), with trucks, vans or buses being particularly affected (Becker et al., 2022; Baker and Reynolds, 1992). A critical rollover wind velocity of 20 m s−1 was found for high-sided lorries in crosswind situations (Snaebjornsson et al., 2007). Particularly dangerous situations with strong crosswinds can occur on bridges (Wang et al., 2014; Charuvisit et al., 2004). A vehicle overturning model is applied by the UK Met Office (Hemingway and Robbins, 2020). It estimates the risk of overturning based on wind gust thresholds ranging from 23 to 45 m s−1, depending on vehicle type, loading, driving speed and wind direction. In addition to direct effects of high wind speed on road vehicles, indirect effects like blocked roads due to falling trees or drifting snow can affect road transport (Leviäkangas et al., 2011).
The most frequent impact of high wind speed on railway transport is the blockage of tracks due to windthrow of trees or drifting snow, as well as loss of electricity due to damaged overhead lines (Leviäkangas et al., 2011), an example of a compound event. Only in rare cases can extreme gusts exceeding 40 m s−1 blow trains off the track (Sprenger et al., 2018). Mean winds above 17 m s−1 or wind gusts above 30 m s−1 have been identified as thresholds relevant for wind-induced damage to railway transport (Thornes and Davis, 2002). Shaking of overhead cables can cause damage to masts and pantographs on trains. Consequences of windthrow can be collisions of trains with fallen trees. Precursory measures to prevent collisions are reduced travelling speeds or cancelling/limiting train services, commonly leading to widespread delays.
The most common impacts on ports are delays due to the disruption of loading and unloading procedures, as well as direct damage to infrastructure. For example, maximum wind speed recommended for crane operations is around 18 m s−1, depending on the design of the crane (TT Club et al., 2011). This can have effects on the overall efficiency of ports (Garcia-Alonso et al., 2020). From 88 disruptive events affecting ports and their surrounding seas in the UK between 1950 and 2014, 36 % were attributed to wind storms and 12 % to storm surges, while the others were mainly related to human error and mechanical faults (Adam et al., 2016).
In the case of inland waterway transport, there is generally no large impact of wind on vessels, since they are sufficiently wide and stable (Leviäkangas et al., 2011). However, at specific locations with high local wind speed due to topography or at locations which are difficult to navigate, navigation of pushed convoys without bow thrusters may be suspended in case of high wind speed. In addition to location-specific issues, the vulnerability of vessels to strong wind is strongly dependent on the vessel's characteristics (Schweighofer, 2014). For specific types of inland container vessels, a mean wind speed of 18 m s−1 can lead to flooding of open cargo holds due to heeling and rolling (Hofman and Bačkalov, 2010) and increase the risk of sliding of empty containers on the upper tiers.
In the case of deep-sea shipping, vessels like large container ships are rarely lost at sea. However, high wind speed impose the danger of container losses (Allianz, 2019). The global average annual loss of containers is estimated to be up to 10 000 yr−1 (Frey and DeVogelaere, 2014). These numbers are low compared to a total number of more than 200 million containers transported per year, but each container lost at sea can lead to a significant safety and environmental hazard. In contrast to container ships, losses of dry bulk carriers are often related to heavy weather conditions (INTERCARGO, 2018). Forecasts of ocean surface conditions are important for route planning to avoid areas affected by windstorms (Kite-Powell, 2011).
Aeroplanes are affected by strong winds mainly during take-off and landing. Dangerous situations related to wind are mainly caused by abrupt changes in wind speed due to wind gusts, wind shear or microbursts (strong downward movements of air within and below thunderstorms). In the USA, for example, 48 % of weather-related aviation accidents are due to adverse wind conditions, and of those wind-related accidents 34 % are due to crosswinds and 29 % due to wind gusts (Jenamani and Kumar, 2013). Therefore, for safety reasons, separation distances between aeroplanes are increased under high-wind conditions. Furthermore, depending on the wind direction, runways may need to be closed. At London Heathrow, for example, tailwinds of more than 2.6 m s−1 and crosswinds above 13 m s−1 are avoided by changing flight direction or runways (Pejovic et al., 2009). This can lead to delays, diversions and cancellations of flights. At London Heathrow Airport, an increase in wind speed of 0.5 m s−1 above the mean increases the probability of delay by 8 % (Pejovic et al., 2009).
4.5 Agriculture
4.5.1 Wind damage in the agriculture
Agricultural production levels are crucial for the worldwide economy. Wind leads to substantial environmental, social and economic losses and has distinct impacts on agriculture: physical damage to crops and related infrastructure, soil erosion including nutrient and soil carbon removal, dust storms, higher evapotranspiration rates of plants, and negative impacts on flowering, pollinators and fruits (Torshizi et al., 2020).
Wind can damage crops through various mechanisms. Most vegetables already react to low wind speed of around 4 m s−1 with physiological adaptations that affect the quantity or quality of the harvest (Rouse and Hodges, 2004). Most kinds of crops can also be directly damaged by abrasion from windblown dust particles or rubbing leaves (Brandle et al., 2004). In orchards, wind can cause a considerable loss by breaking branches or damaging the fruit set (Gardiner et al., 2016). For cereals, lodging (i.e. flattening) is probably the most important impact of wind. For instance, wheat yield is usually reduced about 25 % when fields are lodged (Baker et al., 2014), but the loss can reach up to 50 %–68 % (Berry and Spink, 2012) and the yield of other cereals can decrease by 35 %–50 % under these conditions (Rajkumara, 2008). In most cases lodging is caused by strong wind accompanied by heavy rain, whereby the maximum wind speed is the critical parameter (Mohammadi et al., 2020; Niu et al., 2016). The vulnerability of plants to lodging depends on many factors; for example, excessive usage of nitrogen fertilizers increases lodging vulnerability of wheat (Berry et al., 2019). It is therefore difficult to determine general threshold values for a critical wind speed. However, typical lodging threshold wind speeds at 10 m above the ground for maize, oilseed rape, oats and wheat can be assumed to be 11.5, 14.8, 15.1 and 16.5 m s−1 respectively (Joseph et al., 2020; Baker et al., 2014).
In general, plants exposed to wind are shorter and have thicker leaves, and mature plants are less vulnerable to wind stress than younger plants (Brandle et al., 2004). Therefore, land users must carefully balance between the investment in wind adaptation measures and yields (Wiréhn et al., 2020). However, the careful selection of wind resistant varieties with short stems (Berry et al., 2014), climate resilient plants or the use of cultivar mixtures can significantly improve wind lodging stress resistance, as demonstrated in wheat (Kong et al., 2022). Field fruits react differently to wind exposure: vegetables in general have a very low tolerance to wind stress. Cucumber, pepper and cabbage for example can be damaged by even a low wind speed of around 5 m s−1; corn and cotton are a bit more resistant than most vegetables but also susceptible to wind damage when wind speed exceeds 6 m s−1 (Rouse and Hodges, 2004). Overall, critical thresholds for damage linked to wind speed vary substantially.
Whether or not wind-related agricultural damage will increase under continued warming is unclear. Peña-Angulo et al. (2020) found that none of the five metrics linked to wind speed show a significant trend in either direction. However, the results are subject to considerable uncertainty given that convective events, which are associated with downbursts and straight-line winds, are poorly simulated in the current generation of global circulation models.
4.5.2 Wind erosion, dust storms and agricultural drought
In regions with open and sandy arable land, wind can cause wind erosion and dust storms. Wind erosion refers to the loss of fertile topsoil, whereas dust storms are singular events where strong winds displace huge amounts of soil in a short time. Dust storms are particularly frequent in the so-called dust belt reaching from the north of Africa through the Middle East to central Asia (Gholizadeh et al., 2021). However, soil loss due to wind erosion is also an important issue in less erosion-prone areas such as Europe (Borrelli et al., 2017). While wind is the main forcing factor, there are other climatic factors such as precipitation, soil moisture and radiation, which affect the soil surface and thus influence soil erosion (Bärring et al., 2003).
The threshold values for the mean wind speed at which soil particles start to be dislodged vary greatly depending on the type and condition of the soil (Shahabinejad et al., 2019). According to Rouse and Hodges (2004) the minimum mean wind speed to create erosion is normally about 5–6 m s−1 at 30 cm above the ground. Shahabinejad et al. (2019) found CWS values of 5.7–8.9 m s−1 at 10 m height for soils in Iran. Plants can suffer from dust storms due to loss of plant tissue through abrasion resulting in reduced photosynthesis and burial of seedlings (Stefanski and Sivakumar, 2009). This can result in considerable economic losses for farmers. For example, Gholizadeh et al. (2021) demonstrate that a dust storm lasting 1 h can reduce the annual income of farmers by up to 1.2 %. Erosion reduces soil fertility for long periods due to removal of soil containing essential nutrients. In many cases, extreme drought conditions precede dust storms (Sivakumar, 2005; Sissakian et al., 2013), as dry soil disaggregates faster and thus dislodges more easily enhancing erosion. Wind erosion is thereby closely related to land use practices.
Physiological water stress can be enhanced by increased evapotranspiration, due to high wind speed. The longer such wind conditions last, the more severe the risk as exemplified by a recent drought event in India (Masroor et al., 2020). Thus, wind can exacerbate drought conditions and lead to crop failure. While wind speed is not expected to increase as a global average (McVicar et al., 2012), evapotranspiration likely will increase in many regions due to the increased evaporative demand caused by higher air temperatures (Tomas-Burguera et al., 2020) and a reduced number of days with rainfall. The fact that some plants react to hot and windy weather conditions by closing their stomata may balance some of the enhanced evapotranspiration deficit. However, this is at the expense of plant growth.
4.5.3 Protection measures against wind
Because of the direct wind damage in agriculture, it is necessary or even indispensable to take countermeasures to minimize the risks. Such measures can be a better choice of location according to topographic features or using windbreaks. Windbreaks usually consist of natural barriers such as tree rows. The most important aspect of a windbreak is its height (Brandle et al., 2004). Indeed, windbreak effects on adjacent crops result in a yield reduction due to water and light competition up to a distance of one to two windbreak heights, which is followed by a yield increase up to a distance of 8–12 heights (Weninger et al., 2021). To moderate effects of wind flow around the windbreak, it should be at least 10 times as broad as it is high (Brandle et al., 2004).
4.6 Wind-based energy production
Wind indices are of interest for estimating the wind potential and wind energy. Extreme wind events on different spatial and temporal scales (e.g. storms, gustiness or low-level jets) affect the energy production, the structural integrity and operational safety of wind turbines. Microscale variability in the wind field occurs temporally (e.g. gustiness) and spatially (e.g. vertical wind shear). These variations of the wind field depend on the time of day and thus on the stability of the atmospheric stratification. There is also a dependence on the characteristics of the wind turbine site (land use, terrain). Microscale variations of the wind field influence both the wind potential and the operational reliability of a wind turbine.
Wind indices are typically defined as the ratio of the current values of a variable to the long-term mean. The variable is either related to the wind speed or to the wind energy production. Extreme wind events are directly related to wind-speed-based indices. To identify the energy potential at a site, the power density wind index can be used (Katinas et al., 2018; Celik, 2003). It is based on parameters of wind speed frequency distribution. The power density index results in significantly higher variations than the real energy production of the wind turbine at the location and should be applied carefully. In practice, both the current values of the wind speed are needed (control of the turbine) and the evaluation of the annual energy yield compared to the long-term average using wind indices (planning of turbines, financing).
When addressing wind climate at a location, including the occurrence of strong wind events, which includes both productive and destructive events, much attention was given to the connection between the wind climate and the wind energy potential (Carta and Mentado, 2007). In comparison to the wind speed-based indices, the production-based indices use the energy yield of turbines as input data. The wind energy production index can be based on a wind speed index (Ritter et al., 2015) calculated from wind speed data by an additional application of a power curve (Hahn and Rohrig, 2003; Ding et al., 2005). Another possibility is the use of energy yield data of a wind turbine directly. The BDB index (BDB, 2021) describes the ratio of monthly reported energy yields from wind turbines in a region to the long-term mean yields of these wind turbines. High wind speed or wind shear due to storms or low-level jets need to be taken into account when calculating wind speed indices. However, the energy-production-based indices contain the effects of such events only when the wind turbine is working, i.e. until reaching the turbine cut-out wind speed. Due to their design, most systems switch off at a wind speed above 25 m s−1 (Christakos et al., 2016), but there are also slightly higher and lower shutdown wind speed values for different system types (Chauhan and Saini, 2014). An analysis showed that storms had a positive effect on the wind energy production for southwestern Europe and the Iberian Peninsula (Gonçalves et al., 2020, 2021). As such, the highest values of wind energy production result for stormy weather conditions (Petrović and Bottasso, 2014). Climate change impacts on wind energy have been investigated for a few years (Pryor and Barthelmie, 2010; Moemken et al., 2018). The studies are mostly in agreement on a minimal effect of climate change on the wind energy production (Jung and Schindler, 2020).
Topographic effects are another example of small-scale effects on the wind field, leading to a local wind speed-up, separation and reattachment. These processes can be studied by numerical models (Uchida and Ohya, 2003, 2008, 2011; Uchida and Li, 2018; Uchida, and Sugitani, 2020). Uchida and Kawashima (2019) defined two indices to evaluate the terrain-induced turbulence and the fatigue damage based on the measurement data and the design value. These studies indicated the need for further development of standards. A commonly used turbulence index is the effective turbulence for site-specific fatigue assessment of wind turbines (Slot et al., 2019). Additionally, the usage of the effective turbulence index significantly reduces the number of aero-elastic simulations needed for checking the loads on major components of the wind turbine.
4.7 Compound indices
Strong winds often co-occur with other phenomena, and their co-occurrence affects the damage levels observed. This is an integral part of the compound event concept in which multiple phenomena or hazards form a complex causal chain of events that can lead to a more extreme impact than each phenomenon by itself (Zscheischler et al., 2018). A compound event is often associated with one driver (e.g. an extreme cyclone) which may cause multiple hazards (e.g. strong wind and heavy precipitation), but it can have more complex characteristics (Zscheischler et al., 2020). For example, strong wind can also serve as a modulator for hazards like drought and wildfire. A full typology of compound events can be found in Zscheischler et al. (2020).
4.7.1 Precipitation
Strong wind speed often co-occurs with heavy precipitation (Martius et al., 2016), causing multivariate compound events. Additionally, it is argued that wind and precipitation enhance the impact by extratropical cyclones, since cyclones with extreme precipitation often have a longer lifetime than cyclones with only extreme wind speed (Messmer and Simmonds, 2021). Furthermore, the impact of such multivariate compound events is much higher than a hazard containing only wind or precipitation (Martius et al., 2016). In coastal areas, even when wind is not considered as a hazard itself, wind together with heavy precipitation can cause storm surges and coastal flooding (Wahl et al., 2015; Couasnon et al., 2020). Furthermore, precipitation is important when saturating the soil prior to the occurrence of a windstorm. Soil water content is an index that governs the stability of the root sector of trees during storm events (Everham and Brokaw, 1996; Défossez et al., 2021).
4.7.2 Air temperature
Wind and low air temperatures are both drivers, causing windchill as human health and agricultural hazards among other risks. Each driver, when acting by itself, would have caused less of an impact than the compound effect (Danielsson, 1996). Windchill is a threat mainly in cold climates, where enhanced wind speed increases the heat transfer from an object. Such heat loss can cause injuries and mortality both in animals and plants. Windchill can be calculated as the windchill temperature, also called windchill factor (Quayle and Steadman, 1998; Bluestein and Zecher, 1999), which is usually taken as the air temperature at which there would be an equivalent rate of heat loss. Also, low air temperatures can lead to the freezing of soil and enhances the stability of trees against windthrow during windstorms (Pasztor et al., 2015). In contrast, trees in frozen soil are more likely to undergo stem breakage than uprooting (Everham and Brokaw, 1996; Peltola, 2006).
4.7.3 Drought
The impact of wind on drought is comparatively small compared with other drivers like temperature and (lack of) precipitation, but it has an effect in terms of the evapotranspiration. Wind is thus included in some drought indices through evapotranspiration in the Penman or Penman–Monteith equation, such as in the Baumgartner index (Baumgartner et al., 1967). These indices are therefore short-term indices that operate on a scale of days and typically do not take into account the long-term impacts of drought on the risk of wind damage to forests. Drought can be considered as a pre-condition, which potentially amplifies the impact of winds. Csilléry et al. (2017) showed that long-term drought can increase the risk of wind damage on sites where drought can lead to a weakening of trees but can also decrease the risk of damage on normally extremely wet sites.
4.7.4 Fire
Indices used for assessing fire risk include often wind and topography to determine the rate of spread and damage caused by a wildfire. Wind and slope are viewed as the major factors influencing fire development (Byram 1959a, b; Sharples 2008). The most used indices for fire risk are based on the Canadian Forest Fire Weather Index (FWI) system (Van Wagner and Forest, 1987) that uses information on fuel loading and meteorological conditions (rainfall, temperature, humidity and wind speed) to predict the probability of a fire starting and then the probable spread of the fire. Humidity, wind speed and air temperature are used to calculate the day-to-day drying of the fuel load. The initial spread index is then used to adjust the FWI as an exponential function of wind speed (doubles the FWI for every increase of wind speed by 19 km h−1 or 5.3 m s−1). The spread of the fire will also be affected by the topography and, in particular, how the topography modifies the wind speed and direction.
Wind can alter the angle of the fire toward unburnt fuel, extending the preheating range and increasing the rate of spread. Slope has a similar effect by affecting the distance between the flames and the fuel. Thus, typically the greatest rate of spread is found when an upslope is combined with upward winds and vice versa (Sharples, 2008). Since topography influences wind traits, it can create a channelling effect enhancing fire intensity, but with the strength of the effect depending on the overlap between wind direction and landscape orientations (Barros et al., 2012; Mansuy et al., 2014). Kushla and Ripple (1997) found in a review that a higher relative elevation, proximity to ridges and increased exposure to wind all led to greater fire damage in forests. Additionally, aspects that are associated with greater exposure to dry winds increased fire damage in forests, and damage was lower in aspects with cold and moist winds. There is an index combining slope, aspect and wind speed (wind-topo), but it had a rather low importance for the final model chosen for statistical interpretation (Masoudvaziri et al., 2022).
4.8 Wind speed warning levels used at national meteorological services and sector-related critical thresholds
Advanced storm warnings are crucial for the protection of property and lives. Meteorological services operate a structured warning system for windstorms and recommend appropriate protective measures and rules of conduct depending on the warning level (e.g. Germany: DWD, 2021; Ireland: MetEireann, 2023; or Sweden: SMHI, 2023). The warnings will be published when the event reaches a certain probability level to occur. It can be well spatially located, especially when the warning criterion is met, such as wind speed or precipitation exceeding a certain threshold value. These threshold values are set individually by all meteorological services. In some cases, the weather services already indicate possible consequences due to the wind speed, by warning of damage to infrastructure, forests or energy systems at differing warning levels. There are even variants of weather forecasting systems that follow a more risk-based approach; i.e. the probabilities and consequences of extreme events are integrated into the forecasting system in order to achieve an improved warning management (Neal et al., 2014 or Kaltenberger et al., 2020). A Europe-wide overview of warnings and, in part, possible impacts are provided by Meteoalarm (https://www.meteoalarm.org, last access: 27 April 2023), developed by EUMETNET (European Meteorological Network), which provides relevant information on extreme weather events from 37 national meteorological services.
We collected many critical thresholds from the literature for the five sectors which are the focus of this article. The vulnerability of each sector to wind speed is illustrated in Table S1. Figure 3 provides a synthesis and comparison of thresholds from the five sectors. The agriculture sector seems to be the most sensitive to wind, as negative effects are already noticeable at mean wind speed well below the first official warning level of the DWD (WL1 =14 m s−1; Rouse and Hodges, 2004).
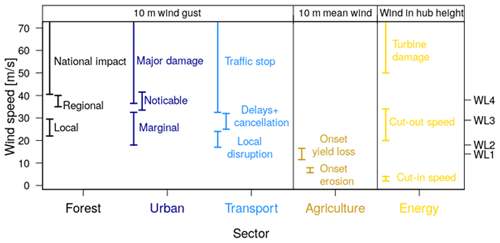
Figure 3Critical threshold ranges of wind speed (mean wind speed (averaging interval 1 h) and wind gusts) for five affected sectors. Warning levels (WL1-4 at speeds of 14, 18, 29 and 39 m s−1, respectively) of the DWD for wind gusts (Primo, 2016) are marked on the right axis. For each sector different ranges of critical mean wind speed from the literature are plotted, to show the mean wind speed (or gust), where impacts are expected. Thresholds in the first three sectors (forest, urban areas and transport) refer to wind gusts at 10 m height. For agriculture we present the mean wind speed at 10 m height, and the shown thresholds for the energy sector refer to the mean wind speed measured at the height of the wind turbines (wind in hub height). The upper bar (e.g. “National impact”) is left open as damage will occur also at higher wind speeds. The threshold ranges mean the following: (1) forest local – limited area of damage; forest regional – damage level is meaningful for the affected forest and short-term forest planning and timber price; forest national – damage can occur across several countries. (2) Urban marginal: light objects, tiles can be lifted or come loose. Urban noticeable: heavier objects are lifted, and first damage to individual building components is possible. Urban major: large vehicles overturn; roofs are severely damaged. (3) Transport local disruption: blocked roads through windthrow or sliding containers at ships. Transport delays: cancellation through electricity cuts and increasing number of wind-related accidents. Transport traffic stop: damage of overhead cables and longer power failures as well as airport and harbour closures. (4) Agriculture erosion: soil loss to wind erosion. Agriculture yield loss: damage to leaves and yield loss due to lodged fields. (5) Energy cut-in speed: start of energy production. Energy cut-out speed: automatic shutdown of wind turbines.
At WL2 (18–29 m s−1), initial restrictions must already be expected in all five sectors, but these are initially localized. In the forest, individual trees and areas may be affected (Gardiner et al., 2010, 2013, 2016). Buildings may show slight roof damage but no structural damage yet (Feuerstein et al., 2011). In road traffic there may be some accidents, and there may be delays in train and air traffic (Vajda et al., 2014). Concerning wind energy, no damage is expected yet, but depending on the type of turbine, precautionary shutdowns of turbines may occur. For WL3 (29–39 m s−1), the literature describes significant impacts in the forest, building and transportation sectors. Damage will be significant, and impacts are already affecting regional areas. The influence of storms on transportation can quickly impact society at regional to national levels.
Severe damage is described at the national level from WL4 onwards, including damage to wind turbines (Quaschning, 2016), massive building damage (Feuerstein et al., 2011), or even the shutdown of entire transport sectors (air and railway) (Vajda et al., 2014). While forest, urban areas and transport are affected by wind speed of the same order of magnitude (i.e. consequences for society are mostly locally at WL2, regionally at WL3 and nationally at WL4), the energy production from wind is impacted at a much higher wind speed (when only damage is considered), while agricultural productivity is impacted at a much lower wind speed.
In this review we covered a wide range of topics dealing with wind damage to terrestrial ecosystems with an emphasis on studies dealing with central Europe. To conclude, we address issues of importance in the near future and topics that require further research. The most intriguing question in this field is how wind-related damage levels may change in future decades, given the strong dominance of decadal variability (Feser et al., 2015). Therefore, attention was given to identifying drivers of future changes in windstorms and cyclone characteristics which are particularly important for the predictability of present-day and long-term trends in socio-economic damage (Koks and Haer, 2020; Hoeppe, 2016; Franzke, 2021). The key current drivers that contribute to future changes in storms are well known; many studies assume that the atmospheric moisture content will increase due to global warming (IPCC, 2021). Idealized studies suggest that this increase in moisture will lead to a stronger circulation, more intense storms (including stronger winds and more rainfall) and, thus, to an expansion of the windstorm footprint (Catto et al., 2019). Additionally, studies show that the lower-tropospheric meridional air temperature gradient will decrease due to Arctic amplification, whereas the upper-tropospheric meridional air temperature gradient will increase due to the warming of the tropical upper troposphere and the cooling of the polar lower stratosphere (Lee et al., 2019). However, it is still uncertain how these contrasting forcing mechanisms will contribute to the future changes in storms quantitatively (Catto et al., 2019; their Fig. 2). The recently extended ERA5 reanalysis product could enable further studies to deal with wind-related damage in the past, present and future, reducing uncertainties. Indeed, increasing the resolution of climate models may improve their capacity to quantify statistical storm properties. CMIP6 models already indicate a general improvement in future storm tracking (Priestley et al., 2020a; Harvey et al., 2020). As a result, more accurate projections of wind and storm damage based on future emission scenarios and climate change may be attainable in the future. According to a recent study, winter storm-related wind gusts could increase towards the second half of the 21st century in Germany (Jung and Schindler, 2021). This demonstrates the need for more studies in damage analysis.
Methodologically, the usage of the indices described here in damage analysis has many advantages, but their creation can be time-consuming, and their usage may lead to statistical pitfalls. For example, it is important to choose indices while trying to avoid an overlap in variability explained by different topographic indices (Mitchell et al., 2001). In this sense, we are lacking a clear methodology that can select the most suitable indices in advance, especially when many indices are easily available. There are three main approaches: (1) a hypothesis-based approach, where typically only few variables are used in the analyses because these variables can be well explained and justified due to past research, incorporation of expert knowledge in the development of indices using co-design and familiarity with the study site (Gebhardt et al., 2019; Merz et al., 2020); (2) a computational approach, where a feature-selection algorithm (e.g. genetic algorithm) is first used to trim down the number of independent variables before performing an analysis; and (3) an exploratory approach with little limitation on the number of independent variables used, where one can examine, for example, if a certain group of indices is more useful than another (e.g. gust-related indices vs. topographic indices) in achieving accurate models according to a given evaluation metric (e.g. coefficient of determination or area under the curve). The choice of method is dependent not only on the specific research goals, but also on the skill set and computational resources available. For instance, an exploratory analysis including many variables on a large area may demand access to high-performance computing. Furthermore, when modelling on a large spatial scale, it is important to choose analysis tools that test and quantify the homogeneity of the relation between indices and damage variables across the different subregions at the study site. Thus, taking into account that key parameters may change within the study area, such as the topography or the vegetation structure, altering the relations between an independent variable and storm damage. Finally, when analysing socio-economic impacts, the availability of data is often a limiting factor, and these limitations shape the selection and analysis approach.
We identify that the area most in need of new indices for wind-related damage analysis is compound events. Damage from extreme climatic events most commonly occurs through interactions between different hazards (Zscheischler et al., 2020). The main challenge is to handle the different timescales of each factor; for example, a storm may last from hours to days, but drought can last years. Therefore, we require indices that incorporate a multitude of factors that are very site specific, as both the topography and the land cover can strongly modify these interactions. Another important challenge is the inclusion of non-climate drivers related to exposure and vulnerability in the compound indices. Concerning the five sectors dealt with here, we present sector-specific outlooks.
5.1 Forest
In a forest setting, there are very few measurements of tree damage due to storms (Kamimura et al., 2022) and very few studies of the dynamic nature of damage at the timescale of a storm. Such studies are required to understand damage initiation and propagation during storms (Dupont et al., 2015). In addition, predicting airflow over complex terrain is still difficult when there are steep slopes and multiple changes in vegetation height (Finnigan et al., 2020). Similarly, there is a need for improvement of land surface information and, in particular, the acquirement of highly resolved 3D distributions of vegetation elements at the landscape scale to enable the creation of fine-scale maps for risk assessment. To this end, it is often difficult to assess damage or risk at the most relevant spatial scale. The recent developments in remote sensing techniques (terrestrial and airborne laser scanning) promise effective assessments of surfaces structures (Favorskaya and Jain, 2017) and may prove useful for many of these issues. However, it may take much time to achieve a sufficient level of data collection. For example, terrestrial laser scanning is accurate but confined to small areas. Furthermore, using airborne laser scanning and satellite data does not allow an effective assessment, since these techniques do not provide a sufficient resolution and, thus, need further development. Another consequence is that we still lack in monitoring and modelling the small-scale variability in the interactions of the wind field with the surface. The main research questions for the future are as follows: how does the structure of a forest canopy influence the turbulence within and above the canopy? And, as they grow, stems, roots and canopies acclimate to the wind forces, so what is the optimal cultivation and canopy structure to reduce damage (Dèfossez et al., 2022)?
5.2 Urban
In urban settings, storm and loss indices as well as damage functions do not usually consider differences in the exposure and vulnerability of different building types or types of urban areas. To further assess wind damage risks on a smaller spatial scale, investigations of individual building damage or damage to specific types of neighbourhoods are needed, together with modelling of urban areas. However, damage data at a fine spatial scale are difficult to obtain, and it is a priority to improve the documentation of urban damage to support the development of new indices. The availability of data, such as the spatial extent of wind damage to individual buildings or green spaces, is key in developing mitigation strategies. For example, wind channelling as a function of wind speed and direction needs to be reliably simulated during the development phase of new building projects.
5.3 Transport
Studies addressing wind effects on transport usually focus on direct effects in a particular part of the transport system. Results from such studies can strongly depend on the region, data and methodologies used for the study. Studies with a more unified approach addressing wind effects on transport on a broader scale could lead to more comparable results. Furthermore, little research is available that takes into account cascading effects that propagate through different parts of the transport system. In general, it remains unclear how climate change and resulting changes in the wind extremes will affect the transport system. Studies addressing this question should consider not only future changes in wind extremes, but also potential changes of the transport system as part of climate change mitigation measures. Such measures could make the transport system more vulnerable to extreme winds. For example, a shift from road to rail transport to reduce CO2 emissions could lead to a higher vulnerability to wind-related tree fall, because single storm events can lead to a collapse of rail transport over whole countries for periods of several days.
5.4 Agriculture
The future of the agriculture sector is closely linked to the global challenge of feeding a still growing population, which is expected to reach 9.7 billion people by 2050 (United Nations Department of Economic and Social Affairs, 2022). In response to this challenge, the awareness for sustainable and efficient agricultural practices has been gradually increasing. Wind damage in agriculture landscapes is thereby a growing concern due to the potential change in frequency and intensity of wind events as the climate continues to warm (Seneviratne et al., 2021). In order to optimize crop yields and reduce waste, the relationship between wind damage and crop yields needs to be investigated in more detail to quantify this impact. For example, understanding and better short-term prediction of wind events are key to improved crop management. Furthermore, there is a lack of simple indices incorporating soil properties and their tendency to lead to soil erosion and nutrient loss, or wind erosion, as such events can be a major challenge for farmers. There is much space to develop new practices to mitigate wind damage in agriculture by using vegetation and cover crops reduce wind damage. Since the positioning of vegetation (e.g. trees as windbreaks) alters the small-scale interactions of the wind field with the soil and crops, a more accurate positioning of vegetation would be supported by the creation or adaptation of existing compound indices or modelling platforms. Such manipulations of the surface cover can be highly flexible in the spatial scale of the wind-field modification, thus providing a good counter measure to different types of vulnerability in agricultural sector.
5.5 Renewable energy
It is important to follow the influence of climate change projections on wind energy production. With the increase in the reliance on renewable energy, it will be important to reduce uncertainties in wind potential and the risk for technical and safety issues in the operation of the wind turbines. Furthermore, while we know where turbines are located and their characteristics, it is important that the data on the turbines wind field and the energy generated are accessible for scientific projects and to the private sector. Currently much of the data are not made publicly available. For instance, we especially lack wind data at hub height for the evaluation of numerical models. Other key challenges that are similar to other sectors are the acquirement of high-spatial-resolution measurements and past and future modelling of the wind field over heterogeneous surfaces and complex terrains.
In conclusion, predicting and assessing the damage caused by wind and storms is a complex matter, but there are effective and simple methodologies to support assessment and decision making. In the light of future uncertainties, it is vital to continue developing tools to prepare for the next calamities that are bound to occur.
The calculation of the circulation regimes with k-means clustering has been done with R (R Core Team, 2020; https://www.R-project.org/) using the R function “kmeans”.
The data used for Fig. 1a and b comprise ERA5 daily data for sea level pressure (Hersbach et al., 2018, https://doi.org/10.24381/cds.bd0915c6) and were accessed at DKRZ.
The data used were accessed via the collaborative evaluation system XCES, based on Freva technology (Kadow et al., 2021, https://doi.org/10.5334/jors.253), hosted at DKRZ. The reanalysis data are publicly and freely available from the producers ERA5 at the Copernicus Climate Data store (CDS; https://doi.org/10.24381/cds.adbb2d47, Hersbach et al., 2023), COSMO-Rea6 at DWD Open Data Server (https://opendata.dwd.de/climate_environment/REA/COSMO_REA6/, German Meteorological Service, DWD, 2023a), and COSMO-REA2 at University of Bonn (https://reanalysis.meteo.uni-bonn.de/?Download_Data___COSMO-REA2, University of Bonn, 2023). Also, the station observations used are freely available under the DWD Open Data Server (https://opendata.dwd.de, German Meteorological Service, DWD, 2023b).
The supplement related to this article is available online at: https://doi.org/10.5194/nhess-23-2171-2023-supplement.
DG and CLEF designed the outline of the review paper. All authors contributed to writing of the manuscript.
At least one of the (co-)authors is a member of the editorial board of Natural Hazards and Earth System Sciences, and at least one of the (co-)authors is a guest member of the editorial board of Natural Hazards and Earth System Sciences for the special issue “Past and future European atmospheric extreme events under climate change”. The peer-review process was guided by an independent editor, and the authors also have no other competing interests to declare.
Publisher’s note: Copernicus Publications remains neutral with regard to jurisdictional claims in published maps and institutional affiliations.
This article is part of the special issue “Past and future European atmospheric extreme events under climate change”. It is not associated with a conference.
We thank the two anonymous reviewers for their comments which helped to improve this paper.
This publication is the outcome of a working group of the project ClimXtreme, funded by the German Bundesministerium für Bildung und Forschung (Alexia Karwat, Daniel Gliksman, Dörthe Handorf, Deborah Niermann, Hilke S. Lentink, Jens Grieger). Astrid Ziemann was funded by Project QuWind100 (German Federal Ministry for Economic Affairs and Energy, BMWi, based on a decision by the German Bundestag, funding code 0325940A). Christian L. E. Franzke was supported by the Institute for Basic Science (IBS), Republic of Korea, under IBS-R028-D1 and by the National Research Foundation of Korea (NRF-2022M3K3A1097082). Joaquim G. Pinto was supported by the AXA Research Fund. Nico Becker’s work was carried out within the framework of the Hans Ertel Centre for Weather Research. This research network of universities, research institutes, and the Deutscher Wetterdienst is funded by the Bundesministerium für Digitales und Verkehr (grant no. 4818DWDP3A).
This paper was edited by Christian Ohlwein and reviewed by two anonymous referees.
Adam, E. F., Brown, S., Nicholls, R. J., and Tsimplis, M.: A systematic assessment of maritime disruptions affecting UK ports, coastal areas and surrounding seas from 1950 to 2014, Nat. Hazards, 83, 691–713, https://doi.org/10.1007/s11069-016-2347-4, 2016.
Akbar, M. K., Kanjanda, S., and Musinguzi, A.: Effect of Bottom Friction, Wind Drag Coefficient, and Meteorological Forcing in Hindcast of Hurricane Rita Storm Surge Using SWAN + ADCIRC Model, J. Marine Sci. Eng., 5, 38, https://doi.org/10.3390/jmse5030038, 2017.
Albrecht, A., Hanewinkel, M., Bauhus, J., and Kohnle, U.: How does silviculture affect storm damage in forests of south-western Germany? Results from empirical modeling based on long-term observations, Eur. J. Forest Res., 131, 229–247, https://doi.org/10.1007/s10342-010-0432-x, 2012.
Albrecht, A., Kohnle, U., Hanewinkel, M., and Bauhus, J.: Storm damage of Douglas-fir unexpectedly high compared to Norway spruce, Ann. Forest Sci., 70, 195–207, https://doi.org/10.1007/s13595-012-0244-x, 2013.
Albrecht, A. T., Fortin, M., Kohnle, U., and Ningre, F.: Coupling a tree growth model with storm damage modeling – conceptual approach and results of scenario simulations, Environ. Modell. Softw., 69, 63–76, 2015.
Albrecht, A. T., Jung, C., and Schindler, D.: Improving empirical storm damage models by coupling with high-resolution gust speed data, Agr. Forest Meteorol., 268, 23–31, https://doi.org/10.1016/j.agrformet.2018.12.017, 2019.
Allianz: Safety and shipping review 2019 – An annual review of trends and developments in shipping losses and safety, Allianz Global Corporate & Specialty, Munich, Germany, https://www.agcs.allianz.com/content/dam/onemarketing/agcs/agcs/reports/AGCS-Safety-Shipping-Review-2019.pdf (last access: 14 December 2021), 2019.
Ashcroft, J.: The relationship between the gust ratio, terrain roughness, gust duration and the hourly mean wind speed, J. Wind Eng. Ind. Aerod., 53, 331–355, https://doi.org/10.1016/0167-6105(94)90090-6, 1994.
Ashley, W. S. and Mote, T. L.: Derecho hazards in the United States, B. Am. Meteorol. Soc., 86, 1577–1592, https://doi.org/10.1175/BAMS-86-11-1577, 2005.
Baker, C. J.: A simplified analysis of various types of wind-induced road vehicle accidents, J. Wind Eng. Ind. Aerodyn., 22, 69–85, https://doi.org/10.1016/0167-6105(86)90012-7, 1986.
Baker, C. J. and Reynolds, S.: Wind-induced accidents of road vehicles, Accident Anal. Prev., 24, 559–575, https://doi.org/10.1016/0001-4575(92)90009-8, 1992.
Baker, C. J., Sterling, M., and Berry, P.: A generalised model of crop lodging, J. Theor. Biol., 363, 1–12. https://doi.org/10.1016/j.jtbi.2014.07.032, 2014.
Bärring, L., Jönsson, P., Mattsson, J. O., and Åhman, R.: Wind erosion on arable land in Scania, Sweden and the relation to the wind climate – a review, Catena, 52, 173–190, 2003.
Barros, A. M. G., Pereira, J. M. C., and Lund, U. J.: Identifying geographical patterns of wildfire orientation: A watershed-based analysis, Forest Ecol. Manag., 264, 98–107, https://doi.org/10.1016/j.foreco.2011.09.027, 2012.
Baumgartner, A., Klemmer, L., Raschke, E., and Waldmann, G.: Waldbrände in Bayern 1950 bis 1959, Mitteilungen aus der Staatsforstverwaltung Bayerns, 36, 57-79, 1967.
BDB: WEA-Standortdaten, Betreiber-Datenbasis (BDB), http://www.btrdb.de/sto.html, last access: 21 December 2021.
Beck, V. and Dotzek, N.: Reconstruction of Near-Surface Tornado Wind Fields from Forest Damage, J. Appl. Meteorol. Clim., 49, 1517–1537, https://doi.org/10.1175/2010JAMC2254.1, 2010.
Becker, N., Rust, H. W., and Ulbrich, U.: Weather impacts on various types of road crashes: a quantitative analysis using generalized additive models, Eur. Transp. Res. Rev., 14, 37, https://doi.org/10.1186/s12544-022-00561-2, 2022.
Belcher, S. E., Harman, I. N., and Finnigan, J. J.: The Wind in the Willows: Flows in Forest Canopies in Complex Terrain, Annu. Rev. Fluid. Mech., 44, 479–504, https://doi.org/10.1146/annurev-fluid-120710-101036, 2012.
Berry, P., Kendall, S., Rutterford, Z., Orford, S., and Griffiths, S.: Historical analysis of the effects of breeding on the height of winter wheat (Triticum aestivum) and consequences for lodging, Euphytica, 203, 375–383, https://doi.org/10.1007/s10681-014-1286-y, 2014.
Berry, P., Blackburn, A., Sterling, M., Miao, Y., Hatley, D., Gullick, D., Joseph, G., Whyatt, D., Soper, D., Murray, J., and Baker, C.: A multi-disciplinary approach for the precision management of lodging risk, in: Precision agriculture '19 – Papers presented at the 12th European Conference on Precision Agriculture, Montpellier, France, 8–11 July 2019, edited by: Stafford, J. V., Wageningen Academic Publishers, Wageningen, 969–975, https://doi.org/10.3920/978-90-8686-888-9, 2019.
Berry, P. M. and Spink, J.: Predicting yield losses caused by lodging in wheat, Field Crop. Res., 137, 19–26, https://doi.org/10.1016/j.fcr.2012.07.019, 2012.
Berry, P. M., Baker, C. J., Hatley, D., Dong, R., Wang, X., Blackburn, G. A., Miao, Y., Sterling, M., and Whyatt, J. D.: Development and application of a model for calculating the risk of stem and root lodging in maize, Field Crop. Res., 262, 108037, https://doi.org/10.1016/j.fcr.2020.108037, 2021.
Bittelli, M., Ventura, F., Campbell, G., Snyder, R., Gallegati, F., and Pisa, P.: Coupling of heat, water vapor, and liquid water fluxes to compute evaporation in bare soils, J. Hydrol., 362, 191–205, https://doi.org/10.1016/j.jhydrol.2008.08.014, 2008.
Bjerknes, J.: Life cycle of cyclones and the polar front theory of atmospheric circulation. Geophys. Publik., 3, 1–18, 1922.
Blender, R., Fraedrich, K., and Lunkeit, F.: Identification of cyclone-track regimes in the North Atlantic, Q. J. Roy. Meteor. Soc., 123, 727–741, 1997.
Blender, R., Raible, C., and Lunkeit, F.: Non-exponential return time distributions for vorticity extremes explained by fractional Poisson processes, Q. J. Roy. Meteor. Soc., 141, 249–257, 2015.
Blennow, K. and Olofsson, E.: The probability of wind damage in forestry under a changed wind climate, Climatic Change, 87, 347–360, https://doi.org/10.1007/s10584-007-9290-z, 2008.
Bluestein, M. and Zecher, J.: A New Approach to an Accurate Wind Chill Factor, B. Am. Meteorol. Soc., 80, 1893–1900, https://doi.org/10.1175/1520-0477(1999)080<1893:ANATAA>2.0.CO;2, 1999.
Bollmeyer, C., Keller, J. D., Ohlwein, C., Wahl, S., Crewell, S., Friederichs, P., Hense, A., Keune, J., Kneifel, S., Pscheidt, I., Redl, S., and Steinke, S.: Towards a high-resolution regional reanalysis for the European CORDEX domain, Q. J. Roy. Meteor. Soc., 141, 1–15, https://doi.org/10.1002/qj.2486, 2015.
Bonnesoeur, V., Constant, T., Moulia, B., and Fournier, M.: Forest trees filter chronic wind-signals to acclimate to high winds, New Phytol., 210, 850–860, https://doi.org/10.1111/nph.13836, 2016.
Born, K., Ludwig, P., and Pinto, J. G.: Wind gust estimation for Mid-European winter storms: Towards a probabilistic view, Tellus A, 64, 17471, https://doi.org/10.3402/tellusa.v64i0.17471, 2012.
Borrelli, P., Lugato, E., Montanarella, L., and Panagos, P.: A New Assessment of Soil Loss Due to Wind Erosion in European Agricultural Soils Using a Quantitative Spatially Distributed Modelling Approach, Land Degrad. Dev., 28, 335–344, https://doi.org/10.1002/ldr.2588, 2017.
Brandle, J. R., Hodges, L., and Zhou, X. H.: Windbreaks in North American agricultural systems, in: New Vistas in Agroforestry: A Compendium for 1st World Congress of Agroforestry, Springer Netherlands, 65–78, https://doi.org/10.1007/978-94-017-2424-1_5, 2004.
Brasseur, O.: Development and application of a physical approach to estimating wind gusts, Mon. Weather Rev., 129, 5–25, 2001.
Bunkers, M. and Hjelmfelt, M.: Chapter Three – Thunderstorm downbursts: Windstorms and blowdowns, in: Plant Disturbance Ecology, 2nd edn., edited by: Johnson, E. and Miyanishi, K., Academic Press, San Diego, 65–115, https://doi.org/10.1016/B978-0-12-818813-2.00003-4, 2021.
Byram, G.: Combustion of forest fuels, in Forest fire: control and use, edited by: Davis, K. P., McGraw-Hill, New York, 61–89, 1959a.
Byram, G.: Forest fire behaviour, in: Forest Fire: Control and Use, edited by: Davis, K., McGraw-Hill, New York, 584 pp., 1959b.
Carta, J. and Mentado, D.: A continuous bivariate model for wind power density and wind turbine energy output estimations, Energ. Convers. Manage., 48, 420–432, https://doi.org/10.1016/J.ENCONMAN.2006.06.019, 2007.
Cassou, C., Terray, L., and Phillips, A.: Tropical Atlantic influence on European heat waves, J. Climate, 18, 2805–2811, https://doi.org/10.1175/JCLI3506.1, 2005.
Catto, J., Ackerley, D., Booth, J. Champion, A. J., Colle, B. A., Pfahl, S., Pinto, J. G., Quinting, J. F., and Seiler, C.: The Future of Midlatitude Cyclones, Current Climate Change Reports, 5, 407–420, https://doi.org/10.1007/s40641-019-00149-4, 2019.
Celik, A.: Assessing the suitability of wind speed probability distribution functions based on wind power density, Renew. Energ., 28, 1563–1574, https://doi.org/10.1016/S0960-1481(03)00018-1, 2003.
Charuvisit, S., Kimura, K., and Fujino, Y.: Effects of wind barrier on a vehicle passing in the wake of a bridge tower in cross wind and its response, J. Wind Eng. Ind. Aerodyn., 92, 609–639, https://doi.org/10.1016/j.jweia.2004.03.006, 2004.
Chauhan, A. and Saini, R.: Statistical analysis of wind speed data using Weibull distribution parameters, in: 2014 1st International Conference on Non-Conventional Energy (ICONCE 2014), Kalyani, India, 16–17 January 2014, IEEE, 160–163, https://doi.org/10.1109/ICONCE.2014.6808712, 2014.
Chang, E., Lee, S., and Swanson, K.: Storm track dynamics, J. Climate, 15, 2163–2183, 2002.
Chen, X., Jeong, S., Park, H., Kim, J., and Park, C.-R.: Urbanization has stronger impacts than regional climate change on wind stilling: a lesson from South Korea, Environ. Res. Lett., 15, 054016, https://doi.org/10.1088/1748-9326/ab7e51, 2020.
Chen, Y.-Y., Gardiner, B., Pasztor, F., Blennow, K., Ryder, J., Valade, A., Naudts, K., Otto, J., McGrath, M. J., Planque, C., and Luyssaert, S.: Simulating damage for wind storms in the land surface model ORCHIDEE-CAN (revision 4262), Geosci. Model Dev., 11, 771–791, https://doi.org/10.5194/gmd-11-771-2018, 2018.
Christakos, K., Cheliotis, I., Varlas, G., and Steeneveld, G.-J.: Offshore Wind Energy Analysis of Cyclone Xaver over North Europe, Energy Proced., 94, 37–44, https://doi.org/10.1016/j.egypro.2016.09.187, 2016.
Cionco, R.: A wind-profile index for canopy flow, Bound.-Lay. Meteorol., 3, 255–263, https://doi.org/10.1007/BF02033923, 1972.
Clark, P. and Gray, S.: Sting jets in extratropical cyclones: A review, Q. J. Roy. Meteor. Soc., 144, 943–969, 2018.
Crasemann, B., Handorf, D., Jaiser, R., Dethloff, K., Nakamura, T., Ukita, J., and Yamazaki, K.: Can preferred atmospheric circulation patterns over the North-Atlantic-Eurasian region be associated with Arctic sea ice loss?, Polar Sci., 14, 9–20, https://doi.org/10.1016/j.polar.2017.09.002, 2017.
Csilléry, K., Kunstler, G., Courbaud, B., Allard, D., Lassegues, P., Haslinger, K., Gardiner, B., Lassègues, P., Haslinger, K., and Gardiner, B.: Coupled effects of wind-storms and drought on tree mortality across 115 forest stands from the Western Alps and the Jura mountains, Glob. Change Biol., 23, 5092–5107, https://doi.org/10.1111/gcb.13773, 2017.
Couasnon, A., Eilander, D., Muis, S., Veldkamp, T. I. E., Haigh, I. D., Wahl, T., Winsemius, H. C., and Ward, P. J.: Measuring compound flood potential from river discharge and storm surge extremes at the global scale, Nat. Hazards Earth Syst. Sci., 20, 489–504, https://doi.org/10.5194/nhess-20-489-2020, 2020.
Dacre, H.: A review of extratropical cyclones: observations and conceptual models over the past 100 years, Weather, 75, 4–7, 2020.
Dacre, H. and Gray, S.: The spatial distribution and evolution characteristics of North Atlantic cyclones, Mon. Weather Rev., 137, 99–115, 2009.
Dacre, H. and Pinto, J. G.: Serial clustering of extratropical cyclones: a review of where, when and why it occurs, npj Climate and Atmospheric Science, 3, 48, https://doi.org/10.1038/s41612-020-00152-9, 2020.
Danielsson, U.: Windchill and the risk of tissue freezing, J. Appl. Physiol., 81, 2666–2673, https://doi.org/10.1152/jappl.1996.81.6.2666, 1996.
Dèfossez, P., Veylon, G., Yang, M., Bonnefond, J., Garrigou, D., Trichet, P., and Danjon, F.: Impact of soil water content on the overturning resistance of young Pinus Pinaster in sandy soil, Forest Ecol. Manag., 480, 118614, https://doi.org/10.1016/j.foreco.2020.118614, 2021.
Dèfossez, P., Rajaonalison, F., and Bosc, A.: How wind acclimation impacts Pinus pinaster growth in comparison to resource availability, Forestry: An International Journal of Forest Research, 95, 118–129, https://doi.org/10.1093/forestry/cpab028, 2022.
Díaz-Yáñez, O., Mola-Yudego, B., and González-Olabarria, J.: Modelling damage occurrence by snow and wind in forest ecosystems, Ecol. Model., 408, 108741, https://doi.org/10.1016/j.ecolmodel.2019.108741, 2019.
Diffenbaugh, N., Scherer, M., and Trapp, R.: Robust increases in severe thunderstorm environments in response to greenhouse forcing, P. Natl. Acad. Sci. USA, 110, 16361–16366, 2013.
Ding, M., Wu, Y.-C., and Zhang, L.-J.: Study on the algorithm to the probabilistic distribution parameters of wind speed in wind farms. Proc. Chinese Soc. Elec. Eng., 25, 107–110, 2005.
Donat, M. G., Pardowitz, T., Leckebusch, G. C., Ulbrich, U., and Burghoff, O.: High-resolution refinement of a storm loss model and estimation of return periods of loss-intensive storms over Germany, Nat. Hazards Earth Syst. Sci., 11, 2821–2833, https://doi.org/10.5194/nhess-11-2821-2011, 2011.
Dorland, C., Tol, R., and Palutikof, J.: Vulnerability of the Netherlands and Northwest Europe to storm damage under climate change, Climatic Change, 43, 513–535, https://doi.org/10.1023/A:1005492126814, 1999.
Dorrington, J. and Strommen, K.: Jet speed variability obscures Euro-Atlantic regime structure, Geophys. Res. Lett., 47, e2020GL087907, https://doi.org/10.1029/2020GL087907, 2020.
Droste, A., Steeneveld, G., and Holtslag, A.: Introducing the urban wind island effect, Environ. Res. Lett., 13, 094007, https://doi.org/10.1088/1748-9326/aad8ef, 2018.
Duperat, M., Gardiner, B., and Ruel, J.-C.: Testing an individual tree wind damage risk model in a naturally regenerated balsam fir stand: potential impact of thinning on the level of risk, Forestry: An International Journal of Forest Research, 94, 141–150, https://doi.org/10.1093/forestry/cpaa023, 2021.
Dupont, S.: A simple wind–tree interaction model predicting the probability of wind damage at stand level, Agr. Forest Meteorol., 224, 49–63, https://doi.org/10.1016/j.agrformet.2016.04.014, 2016.
Dupont, S., Ikonen, V.-P., Väisänen,, H., and Peltola, H.: Predicting tree damage in fragmented landscapes using a wind risk model coupled with an airflow model, Can. J. Forest Res., 45, 1065–1076, https://doi.org/10.1139/cjfr-2015-0066, 2015.
DWD: Warnkriterien, Deutscher Wetterdienst (DWD), https://www.dwd.de/DE/wetter/warnungen_aktuell/kriterien/warnkriterien.html, last access: 15 December 2021.
Economou, T., Stephenson, D., Pinto, J. G., Shaffrey, L., and Zappa, G.: Serial clustering of extratropical cyclones in a multi-model ensemble of historical and future simulations, Q. J. Roy. Meteor. Soc., 141, 3076–3087, 2015.
Edwards, J.: The relationship between road accident severity and recorded weather, J. Safety Res., 29, 249–262, https://doi.org/10.1016/S0022-4375(98)00051-6, 1998.
Ellis, J., Harris, M., Román-Rivera, M., Ferguson, J., Tereszkiewicz, P., and McGill, S.: Application of the Saffir-Simpson Hurricane Wind Scale to Assess Sand Dune Response to Tropical Storms, Journal of Marine Science and Engineering, 8, 670, https://doi.org/10.3390/jmse8090670, 2020.
Everham, E. and Brokaw, N.: Forest damage and recovery from catastrophic wind, Bot. Rev., 62, 113–185, https://doi.org/10.1007/BF02857920, 1996.
Favorskaya, M. N. and Jain, L. C.: Innovations in Remote Sensing of Forests, in: Handbook on Advances in Remote Sensing and Geographic Information Systems, Intelligent Systems Reference Library, vol. 122, Springer, Cham, https://doi.org/10.1007/978-3-319-52308-8_1, 2017.
Feldstein, S. and Franzke, C.: Atmospheric teleconnection patterns, in: Nonlinear and stochastic climate dynamics, Cambridge University Press, 54–104, https://doi.org/10.1017/9781316339251, ISBN: 9781316339251, 2017.
Feser, F., Barcikowska, M., Krueger, O., Schenk, F., Weisse, R., and Xia, L: Storminess over the North Atlantic and Northwestern Europe – a review, Q. J. Roy. Meteor. Soc., 141, 350–382, 2015.
Feuerstein, B., Groenemeijer, P., Dirksen, E., Hubrig, M., Holzer, A., and Dotzek, N.: Towards an improved wind speed scale and damage description adapted for Central Europe, Atmos. Res., 100, 547–564, https://doi.org/10.1016/j.atmosres.2010.12.026, 2011.
Fink, A. H., Brücher, T., Ermert, V., Krüger, A., and Pinto, J. G.: The European storm Kyrill in January 2007: synoptic evolution, meteorological impacts and some considerations with respect to climate change, Nat. Hazards Earth Syst. Sci., 9, 405–423, https://doi.org/10.5194/nhess-9-405-2009, 2009.
Finnigan, J., Ayotte, K., Harman, I., Katul, G., Oldroyd, H., Patton, E., Poggi, D., Ross, A., and Taylor, P.: Boundary-Layer Flow Over Complex Topography, Bound.-Lay. Meteorol., 177, 247–313, https://doi.org/10.1007/s10546-020-00564-3, 2020.
Flather, R.: Storm surges, in: Encyclopedia of Ocean Sciences, edited by: Steele, J., Thorpe, S., and Turekian, K., Academic, San Diego, California, 2882–2892, ISBN 978-0-12-813082-7, 2001.
Florinsky, I.: An illustrated introduction to general geomorphometry, Prog. Phys. Geog., 41, 723–752, 2017.
Forzieri, G., Pecchi, M., Girardello, M., Mauri, A., Klaus, M., Nikolov, C., Rüetschi, M., Gardiner, B., Tomaštík, J., Small, D., Nistor, C., Jonikavicius, D., Spinoni, J., Feyen, L., Giannetti, F., Comino, R., Wolynski, A., Pirotti, F., Maistrelli, F., Savulescu, I., Wurpillot-Lucas, S., Karlsson, S., Zieba-Kulawik, K., Strejczek-Jazwinska, P., Mokroš, M., Franz, S., Krejci, L., Haidu, I., Nilsson, M., Wezyk, P., Catani, F., Chen, Y.-Y., Luyssaert, S., Chirici, G., Cescatti, A., and Beck, P. S. A.: A spatially explicit database of wind disturbances in European forests over the period 2000–2018, Earth Syst. Sci. Data, 12, 257–276, https://doi.org/10.5194/essd-12-257-2020, 2020.
Franzke, C.: Persistent regimes and extreme events of the North Atlantic atmospheric circulation, Philos. T. Roy. Soc. A, 371, 20110471, https://doi.org/10.1098/rsta.2011.0471, 2013.
Franzke, C.: Impacts of a Changing Climate on Economic Damages and Insurance, Economics of Disasters and Climate Change, 1, 95–110, https://doi.org/10.1007/s41885-017-0004-3, 2017.
Franzke, C.: Towards the development of economic damage functions for weather and climate extremes, Ecol. Econ., 189, 107172, https://doi.org/10.1016/j.ecolecon.2021.107172, 2021.
Franzke, C., Woollings, T., and Martius, O.: Persistent circulation regimes and preferred regime transitions in the North Atlantic, J. Atmos. Sci., 68, 2809–2825, 2011.
Frey, O. and DeVogelaere, A.: The Containerized Shipping Industry and the Phenomenon of Containers Lost at Sea, Marine Sanctuaries Conservation Series, ONMS-14, 1–51, https://sanctuaries.noaa.gov/science/conservation/lostcontainers.html (last access: 14 December 2021), 2014.
Fujita, T.: Tornadoes and Downbursts in the Context of Generalized Planetary Scales, J. Atmos. Sci., 38, 1511–1534, https://doi.org/10.1175/1520-0469(1981)038<1511:TADITC>2.0.CO;2, 1981.
Ganbat, G., Seo, J., Han, J., and Baik, J.-J.: A theoretical study of the interactions of urban breeze circulation with mountain slope winds, Theor. Appl. Clim., 121, 545–555, https://doi.org/10.1007/s00704-014-1252-6, 2015.
Garcia-Alonso, L., Moura, T. G. Z., and Roibas, D.: The effect of weather conditions on port technical efficiency, Mar. Policy, 113, 103816, https://doi.org/10.1016/j.marpol.2020.103816, 2020.
Gardiner, B.: Mathematical modelling of the static and dynamic characteristics of plantation trees, in: Mathematical Modelling of Forest Ecosystems, edited by: Franke, J. and Roeder, A., Sauerländers Verlag, 40–61, ISBN 3793908003, 1992.
Gardiner, B.: The interactions of wind and tree movement in forest canopies, in: Wind and Trees, edited by: Coutts, M. and Grace, J., Cambridge University Press, https://doi.org/10.1017/CBO9780511600425, ISBN 9780511600425, 1995.
Gardiner, B.: Wind damage to forests and trees: a review with an emphasis on planted and managed forests, J. Forest Res., 26, 248–266, https://doi.org/10.1080/13416979.2021.1940665, 2021.
Gardiner, B. A., Stacey, G. R., Belcher, R. E., and Wood, C. J.: Field and wind tunnel assessments of the implications of respacing on tree stability, Forestry, 70, 233–252, https://doi.org/10.1093/forestry/70.3.233, 1997.
Gardiner, B., Peltola, H., and Kellomäki, S.: Comparison of two models for predicting the critical wind speed required to damage coniferous trees, Ecol. Model., 129, 1–23, https://doi.org/10.1016/S0304-3800(00)00220-9, 2000.
Gardiner, B., Byrne, K., Hale, S., Kamimura, K., Mitchell, S., Peltola, H., and Ruel, J.-C.: A review of mechanistic modelling of wind damage risk to forests, Forestry: An International Journal of Forest Research, 81, 447–463, https://doi.org/10.1093/forestry/cpn022, 2008.
Gardiner, B., Blennow, K., Carnus, J. M., Fleischer, P., Ingemarson, F., Landmann, G., Lindner, M., Marzano, M., Nicoll, B., Orazio, C., Peyron, J., Reviron, M., Schelhaas, M., Schuck, A., Spielmann, M., and Usbeck, T.: Destructive storms in European forests: past and forthcoming impacts, Final report to European Commission-DG Environment, European Forest Institute, https://library.wur.nl/WebQuery/wurpubs/fulltext/162053 (last access: May 2023), 2010.
Gardiner, B., Schuck, A., Schelhaas, M.-J., Orazio, C., Blennow, K., and Nicoll, B.: Living with Storm Damage to Forests: What Science Can Tell Us, edited by: Gardiner, B., Schuck, A., Schelhaas, M.-J., Orazio, C., Blennow, K., and Nicoll, B., European Forest Institute, Joensuu, ISBN: 978-952-5980-08-0, 2013.
Gardiner, B., Berry, P., and Moulia, B.: Review: Wind impacts on plant growth, mechanics and damage, Plant Sci., 245, 94–118, https://doi.org/10.1016/j.plantsci.2016.01.006, 2016.
Garratt, J.: Surface influence upon vertical profiles in the atmospheric near-surface layer, Q. J. Roy. Meteor. Soc., 106, 803–819, https://doi.org/10.1256/smsqj.45010, 1980.
Gatzen, C. P., Fink, A. H., Schultz, D. M., and Pinto, J. G.: An 18-year climatology of derechos in Germany, Nat. Hazards Earth Syst. Sci., 20, 1335–1351, https://doi.org/10.5194/nhess-20-1335-2020, 2020.
Gebhardt, O., Kuhlicke, C., Wolf, L., Vitolo, C., Duo, E., van Lanen, H., Rohrer, M., Sutanto, S., and Stoffel, M.: Results of the co-evaluation of the ANYWHERE tools, products and services at the pilot sites (Deliverable 1.4), UFZ, Leipzig, Germany, http://anywhere-h2020.eu/docs/ (last access: 30 May 2023), 2019.
German Meteorological Service (DWD): COSMO-REA6 data set, DWD [data set], https://opendata.dwd.de/climate_environment/REA/COSMO_REA6/, last access: 31 May 2023a.
German Meteorological Service (DWD): DWD station network, https://opendata.dwd.de/, last access: 31 May 2023b.
Gholizadeh, H., Zoghipour, M., Torshizi, M.; Nazari, M., and Moradkhani, N.: Gone with the wind: Impact of soil-dust storms on farm income, Ecol. Econ., 188, 107133, https://doi.org/10.1016/j.ecolecon.2021.107133, 2021.
Goff, T., Nelson, M., Liknes, G., Feeley, T., Pugh, S., and Morin, R.: Rapid assessment of tree damage resulting from a 2020 windstorm in Iowa, USA, Forests, 12, 1–13, https://doi.org/10.3390/f12050555, 2021.
Gonçalves, A., Liberato, M., and Nieto, R.: Wind Energy Assessment during High-Impact Winter Storms in the Iberian Peninsula, Environ. Sci. Proc., 4, 28, https://doi.org/10.3390/ecas2020-08132, 2020.
Gonçalves, A., Liberato, M., and Nieto, R.: Wind Energy Assessment during High-Impact Winter Storms in Southwestern Europe, Atmosphere, 12, 509, https://doi.org/10.3390/atmos12040509, 2021.
Grams, C. M., Binder, H., Pfahl, S., Piaget, N., and Wernli, H.: Atmospheric processes triggering the central European floods in June 2013, Nat. Hazards Earth Syst. Sci., 14, 1691–1702, https://doi.org/10.5194/nhess-14-1691-2014, 2014.
Grimmond, C. and Oke, T.: Aerodynamic Properties of Urban Areas Derived from Analysis of Surface Form, J. App. Meteorol. Clim., 38, 1262–1292, https://doi.org/10.1175/1520-0450(1999)038<1262:APOUAD>2.0.CO;2, 1999.
Gromke, C. and Ruck, B.: On Wind Forces in the Forest-Edge Region During Extreme-Gust Passages and Their Implications for Damage Patterns, Bound.-Lay. Meteorol., 168, 269–288, https://doi.org/10.1007/s10546-018-0348-4, 2018.
Gross, G.: A windthrow model for urban trees with application to storm “Xavier”, Meteorol. Z., 27, 299–308, https://doi.org/10.15488/4901, 2018.
Gyakum, J. and Danielson, R.: Analysis of meteorological precursors to ordinary and explosive cyclogenesis in the western North Pacific, Mon. Weather Rev., 128, 851–863, 2000.
Hahn, B. and Rohrig, K.: ISET-Wind-Index Assessment of the Annual Available Wind Energy, Proc. Europ. Wind Energy Conf. Exh. EWEC2003, Madrid, Spain, 16–19 June 2003, http://citeseerx.ist.psu.edu/viewdoc/download?doi=10.1.1.196.1675&rep=rep1&type=pdf (last access: 21 December 2021), 2003.
Hale, S., Gardiner, B., Peace, A., Nicoll, B., Taylor, P., and Pizzirani, S.: Comparison and validation of three versions of a forest wind risk model, Environ. Modell. Softw., 68, 27–41, https://doi.org/10.1016/j.envsoft.2015.01.016, 2015.
Hanewinkel, M., Kuhn, T., Bugmann, H., Lanz, A., and Brang, P.: Vulnerability of uneven-aged forests to storm damage, Forestry, 87, 525–534, https://doi.org/10.1093/forestry/cpu008, 2014.
Hannachi, A., Straus, D., Franzke, C., Corti, S., and Woollings, T.: Low-frequency nonlinearity and regime behavior in the Northern Hemisphere extratropical atmosphere, Rev. Geophys., 55, 199–234, https://doi.org/10.1175/2009JCLI3087.1, 2017.
Harper, B., Kepert, J., and Ginger, J.: Wind speed time averaging conversions for tropical cyclone conditions, in: AMS 28th Conference on Hurricanes and Tropical Meteorology, Orlando, FL, 28 April–2 May 2008, 1–2, 2008.
Harvey, B., Cook, P., Shaffrey, L., and Schiemann, R.: The response of the northern hemisphere storm tracks and jet streams to climate change in the CMIP3, CMIP5, and CMIP6 climate models. J. Geophys. Res.-Atmos., 125, e2020JD032701, https://doi.org/10.1029/2020JD032701, 2020.
Haylock, M. R.: European extra-tropical storm damage risk from a multi-model ensemble of dynamically-downscaled global climate models, Nat. Hazards Earth Syst. Sci., 11, 2847–2857, https://doi.org/10.5194/nhess-11-2847-2011, 2011.
Held, I.: 100 years of progress in understanding the general circulation of the atmosphere, Meteor. Mon., 59, 6.1–6.23, 2019.
Held, I. M.: Momentum transport by quasi-geostrophic eddies, J. Atmos. Sci., 32, 1494–1497, 1975.
Held, I. M. and Hou, A. Y.: Nonlinear axially symmetric circulations in a nearly inviscid atmosphere, J. Atmos. Sci., 37, 515–533, 1980.
Hemingway, R. and Robbins, J.: Developing a hazard-impact model to support impact-based forecasts and warnings: The Vehicle OverTurning (VOT) Model, Meteorol. Appl., 27, e1819, https://doi.org/10.1002/met.1819, 2020.
Heneka, P., Hofherr, T., Ruck, B., and Kottmeier, C.: Winter storm risk of residential structures – model development and application to the German state of Baden-Württemberg, Nat. Hazards Earth Syst. Sci., 6, 721–733, https://doi.org/10.5194/nhess-6-721-2006, 2006.
Hersbach, H., Bell, B., Berrisford, P., Biavati, G., Horányi, A., Muñoz Sabater, J., Nicolas, J., Peubey, C., Radu, R., Rozum, I., Schepers, D., Simmons, A., Soci, C., Dee, D., and Thépaut, J.-N.: ERA5 hourly data on pressure levels from 1940 to present, Copernicus Climate Change Service (C3S) Climate Data Store (CDS) [data set], https://doi.org/10.24381/cds.bd0915c6, 2018.
Hersbach, H., Bell, B., Berrisford, P., Hirahara, S., Horányi, A., Muñoz-Sabater, J., Nicolas, J., Peubey, C., Radu, R., Schepers, D., Simmons, A., Soci, C., Abdalla, S., Abellan, X., Balsamo, G., Bechtold, P., Biavati, G., Bidlot, J., Bonavita, M., De Chiara, G., Dahlgren, P., Dee, D., Diamantakis, M., Dragani, R., Flemming, J., Forbes, R., Fuentes, M., Geer, A., Haimberger, L., Healy, S., Hogan, R. J., Hólm, E., Janisková, M., Keeley, S., Laloyaux, P., Lopez, P., Lupu, C., Radnoti, G., de Rosnay, P., Rozum, I., Vamborg, F., Villaume, S., and Thépaut, J.-N.: The ERA5 global reanalysis, Q. J. Roy. Meteor. Soc., 146, 1999–2049, https://doi.org/10.1002/qj.3803, 2020.
Hersbach, H., Bell, B., Berrisford, P., Biavati, G., Horányi, A., Muñoz Sabater, J., Nicolas, J., Peubey, C., Radu, R., Rozum, I., Schepers, D., Simmons, A., Soci, C., Dee, D., and Thépaut, J.-N.: ERA5 hourly data on single levels from 1940 to present, Copernicus Climate Change Service (C3S) Climate Data Store (CDS) [data set], https://doi.org/10.24381/cds.adbb2d47, 2023.
Hewson, T. and Neu, U.: Cyclones, windstorms and the IMILAST project, Tellus A, 67, 27128, https://doi.org/10.3402/tellusa.v67.27128, 2015.
Hoeppe, P.: Trends in weather related disasters–Consequences for insurers and society, Weather and Climate Extremes, 11, 70–79, 2016.
Hofman, M. and Bačkalov, I.: Risk-based analysis of inland vessel stability, in: Proceedings of the 11th International Ship Stability Workshop, Wageningen, 21–23 June 2010, 67–72, http://www.shipstab.org/files/Proceedings/ISSW/ISSW_2010_Wageningen_The_Netherlands/ISSW_2010_s03-p2.pdf (last access: 14 December 2021), 2010.
Holland, A., Riordan, A., and Franklin, E.: A Simple Model for Simulating Tornado Damage in Forests, J. App. Meteorol. Clim., 45, 1597–1611, https://doi.org/10.1175/JAM2413.1, 2006.
Holton, J. and Hakim, G.: An Introduction to Dynamic Meteorology, Wiley, ISBN: 9780123848666, 2012.
Hoskins, B. and Valdes, P.: On the existence of storm tracks, J. Atmos. Sci., 47, 1854–1864, 1990.
INTERCARGO: Bulk Carrier Casualty Report – Years 2008 to 2017 and the trends, International Organization of Dry Cargo Shipowners, London, United Kingdom, https://www.intercargo.org/wp-content/uploads/2018/05/bulk-carrier-casualty-report-2017.pdf (last access: 14 December 2021), 2018.
IPCC: Summary for Policymakers, in: Climate Change 2021: The Physical Science Basis. Contribution of Working Group I to the Sixth Assessment Report of the Intergovernmental Panel on Climate Change, edited by: Masson-Delmotte, V., Zhai, P., Pirani, A., Connors, S. L., Péan, C., Berger, S., Caud, N., Chen, Y., Goldfarb, L., Gomis, M. I., Huang, M., Leitzell, K., Lonnoy, E., Matthews, J. B. R., Maycock, T. K., Waterfield, T., Yelekçi, O., Yu, R., and Zhou, B., Cambridge University Press, Cambridge, United Kingdom and New York, NY, USA, https://www.ipcc.ch/report/ar6/wg1/#SPM (last access: 1 May 2023), 2021.
Jenamani, R. and Kumar, A.: Bad weather and aircraft accidents–global vis-à-vis Indian scenario, Curr. Sci. India, 104, 316–325, https://www.jstor.org/stable/24089632 (last access: 14 December 2021), 2013.
Jim, C. and Liu, H.: Storm damage on urban trees in Guangzhou, China, Landscape Urban Plan., 38, 45–59, https://doi.org/10.1016/S0169-2046(97)00018-2, 1997.
Joseph, G., Mohammadi, M., Sterling, M., Baker, C., Gillmeier, S., Soper, D., Jesson, M., Blackburn, G. A., Whyatt, J. D., Gullick, D., Murray, J., Berry, P., Hartley, D., and Finnan, J.: Determination of crop dynamic and aerodynamic parameters for lodging prediction, J. Wind Eng. Ind. Aerod., 202, 104169, https://doi.org/10.1016/j.jweia.2020.104169, 2020.
Jung, C. and Schindler, D.: Statistical Modeling of Near-surface Wind Speed: A Case Study from Baden-Wuerttemberg (Southwest Germany), Austin J. Earth Sci., 2, 1006, ISSN: 2380-0771, 2015.
Jung, C. and Schindler, D.: Introducing a new approach for wind energy potential assessment under climate change at the wind turbine scale, Energ. Convers. Manage., 225, 113425, https://doi.org/10.1016/j.enconman.2020.113425, 2020.
Jung, C. and Schindler, D.: Does the winter storm-related wind gust intensity in Germany increase under warming climate? – A high-resolution assessment, Weather and Climate Extremes, 33, 100360. https://doi.org/10.1016/j.wace.2021.100360, 2021.
Jung, C., Schindler, D., Albrecht, A., and Buchholz, A.: The role of highly-resolved gust speed in simulations of storm damage in forests at the landscape scale: A case study from southwest Germany, Atmosphere, 7, 7, https://doi.org/10.3390/atmos7010007, 2016.
Kadow, C., Illing, S., Lucio-Eceiza, E. E., Bergemann, M., Ramadoss, M., Sommer, P. S., Kunst, O., Schartner, T., Pankatz, K., Grieger, J., Schuster, M., Richling, A., Thiemann, H., Kirchner, I., Rust, H. W., Ludwig, T., Cubasch, U., and Ulbrich, U.: Introduction to Freva – A Free Evaluation System Framework for Earth System Modeling, Journal of Open Research Software, 9, p. 13, https://doi.org/10.5334/jors.253, 2021.
Kaimal, J. and Finnigan, J.: Atmospheric boundary layer flows: Their structure and measurement, Oxford University Press, ISBN: 9780197560167, 1994.
Kaltenberger, R., Schaffhauser, A., and Staudinger, M.: “What the weather will do” – results of a survey on impact-oriented and impact-based warnings in European NMHSs, Adv. Sci. Res., 17, 29–38, https://doi.org/10.5194/asr-17-29-2020, 2020.
Kamimura, K., Nanko, K., Matsumoto, A., Ueno, S., and Gardiner, B.: Tree dynamic response and survival in a category-5 tropical cyclone: The case of super typhoon Trami, Science Advances, 8, 1–11, 2022.
Kaspar, F., Müller-Westermeier, G., Penda, E., Mächel, H., Zimmermann, K., Kaiser-Weiss, A., and Deutschländer, T.: Monitoring of climate change in Germany – data, products and services of Germany's National Climate Data Centre, Adv. Sci. Res., 10, 99–106, https://doi.org/10.5194/asr-10-99-2013, 2013.
Katinas, V., Gecevicius, G., and Marciukaitis, M.: An investigation of wind power density distribution at location with low and high wind speed using statistical model, Appl. Energ., 218, 442–451, https://doi.org/10.1016/j.apenergy.2018.02.163, 2018.
Kelman, I.: Defining Risk, FloodRiskNet Newsletter, Issue 2, 3 pp., http://www.ilankelman.org/abstracts/kelman2003frn.pdf (last access: 1 May 2023), 2003.
Kirk, P.: An updated tornado climatology for the UK: 1981–2010, Weather, 69, 171–175, https://doi.org/10.1002/wea.2247, 2014.
Kite-Powell, H.: The value of ocean surface wind information for maritime commerce, Mar. Technol. Soc. J., 45, 75–84, https://doi.org/10.4031/MTSJ.45.1.13, 2011.
Klawa, M. and Ulbrich, U.: A model for the estimation of storm losses and the identification of severe winter storms in Germany, Nat. Hazards Earth Syst. Sci., 3, 725–732, https://doi.org/10.5194/nhess-3-725-2003, 2003.
Koks, E. and Haer, T.: A high-resolution wind damage model for Europe, Sci. Rep.-UK, 10, 6866, https://doi.org/10.1038/s41598-020-63580-w, 2020.
Kong, X., Peng, P., Li, L., Zhang, K., Hu, Z., Wang, X., and Zhao, G.: Wind lodging-associated yield loss is reduced by wheat genetic diversity, Eur. J. Agron., 133, 126441, https://doi.org/10.1016/j.eja.2021.126441, 2022.
Kramer, M., Hansen, A., Taper, M., and Kissinger, E.: Abiotic controls on long-term windthrow disturbance and temperate rain forest dynamics in southeast Alaska, Ecology, 82, 2749–2768, 2001.
Krayer, W. and Marshall, R.: Gust factors applied to hurricane winds, B. Am. Meteorol. Soc., 73, 613–617, https://doi.org/10.1175/1520-0477(1992)073<0613:gfathw>2.0.co;2, 1992.
Krejci, L., Kolejka, J., Vozenilek, V., and Machar, I.: Application of GIS to empirical windthrow risk model in mountain forested landscapes, Forests, 9, 96, https://doi.org/10.3390/f9020096, 2018.
Kushla, J. and Ripple, W.: The role of terrain in a fire mosaic of a temperate coniferous forest, Forest Ecol. Manag., 95, 97–107, https://doi.org/10.1016/S0378-1127(97)82929-5, 1997.
Lamb, H.: Historic storms of the North Sea, British Isles, and Northwest Europe, Cambridge University Press, ISBN 10: 0521619319, 1991.
Leckebusch, G., Renggli, D., and Ulbrich, U.: Development and application of an objective storm severity measure for the Northeast Atlantic region, Meteorol. Z., 17, 575–587, https://doi.org/10.1127/0941-2948/2008/0323, 2008.
Lee, S., Furtado, J., and Charlton-Perez, A.: Wintertime North American weather regimes and the Arctic stratospheric polar vortex, Geophys. Res. Lett., 46, 14892–14900, https://doi.org/10.1029/2019GL085592, 2019.
Lepore, C., Abernathey, R., Henderson, N., Allen, J. T., and Tippett, M. K.: Future global convective environments in CMIP6 models, Earth's Future, 9, e2021EF002277, https://doi.org/10.1029/2021EF002277, 2021.
Leviäkangas, P., Tuominen, A., Molarius, R., Kojo, H., Schabel, J., Toivonen, S., Keränen, J., Ludvigsen, J., Vajda, A., Tuomenvirta, H., Juga, I., Nurmi, P., Rauhala, J., Rehm, F., Gerz, T., Muehlhausen, T., Schweighofer, J., Michaelides, S., Papadakis, M., Dotzek, N., and Groenemeijer, P.: Extreme weather impacts on transport systems, VTT Working Papers 168, VTT Technical Research Centre of Finland, https://www.vttresearch.com/sites/default/files/pdf/workingpapers/2011/W168.pdf, (last access: 14 December 2021), 2011.
Liberato, M., Pinto, J. G., Trigo, I., and Trigo, R.: Klaus – an exceptional winter storm over Northern Iberia and Southern France, Weather, 66, 330–334, https://doi.org/10.1002/wea.755, 2011.
Lindén, J. and Holmer, B.: Thermally induced wind patterns in the Sahelian city of Ouagadougou, Burkina Faso, Theor. Appl. Climatol., 105, 229–241, https://doi.org/10.1007/s00704-010-0383-7, 2011.
Liu, R., Liu, S., Yang, X., Lu, H., Pan, X., Xu, Z., Ma, Y., and Xu, T.: Wind Dynamics Over a Highly Heterogeneous Oasis Area: An Experimental and Numerical Study, J. Geophys, Res.-Atmos., 123, 8418–8440, https://doi.org/10.1029/2018JD028397, 2018.
Locatelli, T., Tarantola, S., Gardiner, B., and Patenaude, G.: Variance-based sensitivity analysis of a wind risk model - Model behaviour and lessons for forest modelling, Environ. Modell. Softw., 87, 84–109, https://doi.org/10.1016/j.envsoft.2016.10.010, 2017.
Ma, Q., Lembo, V., and Franzke, C.: The Lorenz energy cycle: trends and the impact of modes of climate variability, Tellus A, 73, 1–15, 2021.
Machar, I., Pechanec, V., Brus, J., Kilianova, H., and Kirchner, K.: Forest management at the upper treeline in Jeseniky Mountains (Czech Republic), in: International Multidisciplinary Scientific GeoConference – SGEM 2014, Albena, Bulgaria, 17–26 June 2014, Albena, Bulgaria, STEF92 Technology Ltd., Sofia, Bulgaria, 361–366, ISBN 978-619-7105-17-9, 2014.
Mailier, P., Stephenson, D., Ferro, C., and Hodges, K.: Serial clustering of extratropical cyclones, Mon. Weather Rev., 134, 2224–2240, 2006.
Mansuy, N., Boulanger, Y., Terrier, A., Gauthier, S., Robitaille, A., and Bergeron, Y.: Spatial attributes of fire regime in eastern Canada: influences of regional landscape physiography and climate, Landscape Ecol., 29, 1157–1170, https://doi.org/10.1007/s10980-014-0049-4, 2014.
Martius, O., Pfahl, S., and Chevalier, C.: A global quantification of compound precipitation and wind extremes, Geophys. Res. Lett., 43, 7709–7717, https://doi.org/10.1002/2016GL070017, 2016.
Masoudvaziri, N., Ganguly, P., Mukherjee, S., and Sun, K.: Impact of geophysical and anthropogenic factors on wildfire size: a spatiotemporal data-driven risk assessment approach using statistical learning, Stoch. Env. Res. Risk A., 36, 1103–1129, https://doi.org/10.1007/s00477-021-02087-w, 2022.
Masroor, M., Rehman, S., Avtar, R., Sahana, M., Ahmed, R., and Sajjad, H.: Exploring climate variability and its impact on drought occurrence: evidence from Godavari Middle sub-basin, India, Weather and Climate Extremes, 30, 100277, https://doi.org/10.1016/j.wace.2020.100277, 2020.
Mayer, H.: Wind-induced tree sways, Trees, 1, 195–206, https://doi.org/10.1007/BF01816816, 1987.
Mayer, P., Brang, P., Dobbertin, M., Hallenbarter, D., Renaud, J., Walthert, L., and Zimmermann, S.: Forest storm damage is more frequent on acidic soils, Ann. Forest Sci., 62, 303–311, 2005.
McGinley, J.: A Diagnosis of Alpine Lee Cyclogenesis, Mon. Weather Rev., 110, 1271–1287, 1982.
McVicar, T., Roderick, M., Donohue, R., Li, L., van Niel, T., Thomas, A., Grieser, J., Jhajharia, D., Himri, Y., Mahowald, N. M., Mescherskaya, A. V., Kruger, A. C., Rehman, S., and Dinpashoh, Y.: Global review and synthesis of trends in observed terrestrial near-surface wind speed: Implications for evaporation, J. Hydrol., 416–417, 182–205, https://doi.org/10.1016/j.jhydrol.2011.10.024, 2012.
Merz, B., Kuhlicke, C., Kunz, M., Pittore, M., Babeyko, A., Bresch, D. N., Domeisen, D. I., Feser, F., Koszalka, I., Kreibich, H., and Pantillon, F.: Impact forecasting to support emergency management of natural hazards, Rev. Geophys., 58, e2020RG000704, https://doi.org/10.1029/2020RG000704, 2020.
Messmer, M. and Simmonds, I.: Global analysis of cyclone-induced compound precipitation and wind extreme events, Weather and Climate Extremes, 32, 100324, https://doi.org/10.1016/j.wace.2021.100324, 2021.
MetEireann warning levels: https://www.met.ie/met-eireann-warning-system-explained, last access: 10 January 2023.
Miller, K. F.: Windthrow hazard in conifer plantations, Irish Forestry, 43, 66–78, 1986.
Milne, R.: Dynamics of swaying of Picea sitchensis, Tree Physiol., 9, 383–399, https://doi.org/10.1093/treephys/9.3.383, 1991.
Mitchell, S.: Wind as a natural disturbance agent in forests: a synthesis, Forestry, 86, 147–157, https://doi.org/10.1093/forestry/cps058, 2013.
Mitchell, S., Hailemariam, T., and Kulis, Y.: Empirical modeling of cutblock edge windthrow risk on Vancouver Island, Canada, using stand level information, Forest Ecol. Manag., 154, 117–130, 2001.
Mitchell-Wallace, K., Jones, M., Hillier, J., and Foote, M.: Natural catastrophe risk management and modelling: A practitioner's guide, John Wiley & Sons, https://doi.org/10.1002/9781118906057, ISBN: 9781118906040, ISBN: 9781118906057, 2017.
Moemken, J., Reyers, M., Feldmann, H., and Pinto, J. G.: Future Changes of Wind Speed and Wind Energy Potentials in EURO-CORDEX Ensemble Simulations, J. Geophys. Res., 123, 6373–6389, https://doi.org/10.1029/2018JD028473, 2018.
Mohammadi, M., Finnan, J., Sterling, M., and Baker, C.: A calibrated oat lodging model compared with agronomic measurements, Field Crop. Res., 255, 107784, https://doi.org/10.1016/j.fcr.2020.107784, 2020.
Morimoto, J., Nakagawa, K., Takano, K., Aiba, M., Oguro, M., Furukawa, Y., Mishima, Y., Ogawa, K., Ito, R., Takemi, T., Nakamura, F., and Peterson, C.: Comparison of vulnerability to catastrophic wind between Abies plantation forests and natural mixed forests in northern Japan, Forestry, 92, 436–443, https://doi.org/10.1093/forestry/cpy045, 2019.
Münchener Rückversicherungs-Gesellschaft: Munich Re: Winterstürme in Europa (II) – Schadenanalyse 1999 – Schadenspotentiale, Münchener Rück, 1999.
Murshed, S. and Reed, E.: Mapping of the vulnerability of forest resources due to extreme winter storms in the state of Baden-Württemberg in Germany, AutoCarto, 128–140, 2016.
Negrón-Juárez, R., Chambers, J., Guimaraes, G., Zeng, H., Raupp, C., Marra, D. M., Ribeiro, G., Saatchi, S., Nelson, B., and Higuchi, N.: Widespread Amazon forest tree mortality from a single cross-basin squall line event, Geophys. Res. Lett., 37, L16701, https://doi.org/10.1029/2010GL043733, 2010.
Neal, R. A., Boyle, P., Grahame, N., Mylne, K., and Sharpe, M.: Ensemble based first guess support towards a risk-based severe weather warning service: ensemble severe weather forecasts, Meteorol. Appl., 21, 563–577, https://doi.org/10.1002/met.1377, 2014.
Neu, U., Akperov, M., Bellenbaum, N., Benestad, R., Blender, R., Caballero, R., Cocozza, A., Dacre, H., Feng, Y., Fraedrich, K., Grieger, J., Gulev, S., Hanley, J., Hewson, T., Inatsu, M., Keay, K., Kew, S., Kindem, I., Leckebusch, G., Liberato, M., Lionello, P., Mokhov, I., Pinto, J. G., Raible, C., Reale, M., Rudeva, I., Schuster, M., Simmonds, I., Sinclair, M., Sprenger, M., Tilinina, N., Trigo, I., Ulbrich, S., Ulbrich, U., Wang, X., and Wernli, H.: IMILAST: a community effort to intercompare extratropical cyclone detection and tracking algorithms, B. Am. Meteorol. Soc., 94, 529–547, 2013.
Nicoll, B. and Ray, D.: Adaptive growth of tree root systems in response to wind action and site conditions, Tree Physiol., 16, 891–898, https://doi.org/10.1093/treephys/16.11-12.891, 1996.
Nicoll, B., Gardiner, B., Rayner, B., and Peace, A.: Anchorage of coniferous trees in relation to species, soil type, and rooting depth, Can. J. Forest Res., 36, 1871–1883, https://doi.org/10.1139/x06-072, 2006.
Nicoll, B., Connolly, T., and Gardiner, B.: Changes in Spruce Growth and Biomass Allocation Following Thinning and Guying Treatments, Forests, 10, 253, https://doi.org/10.3390/f10030253, 2019.
Niermann, D., Borsche, M., Kaiser-Weiss, A., and Kaspar, F.: Evaluating renewable-energy-relevant parameters of COSMO-REA6 by comparison with satellite data, station observations and other reanalyses, Meteorol. Z., 28, 347–360, https://doi.org/10.1127/metz/2019/0945, 2019.
Niu, L., Feng, S., Ding, W., and Li, G.: Influence of Speed and Rainfall on Large-Scale Wheat Lodging from 2007 to 2014 in China, PloS ONE, 11, e0157677, https://doi.org/10.1371/journal.pone.0157677, 2016.
Nosnikau, V., Gardiner, B., Donis, J., and Fedarovich, L.: The Overview of Existing Silvicultural Approaches Under the Influence of Adverse Weather Phenomena and Recommendations for Increasing the Forest Resilience to High Wind Events in Belarus, World Bank: GFDRR, Minsk, https://documents.worldbank.org/en/publication/documents- reports/documentdetail/874711556620591536/the-overview-of-existing-silvicultural-approaches-under-the-influence-of-adverse-weather-phenomena-and-recommendations-for-increasing-the-forest-resilience-to-high-wind-events-in-belarus (lats access: 1 May 2023), 2018.
Oke, D., Mills, D., Christen, D., and Voogt, J.: Urban Climates, Cambridge University Press, Cambridge, 582 pp., https://doi.org/10.1017/9781139016476, ISBN: 9781139016476, 2017.
Oliver, H. and Mayhead, G.: Wind Measurements in a Pine Forest During a Destructive Gale, Forestry: An International Journal of Forest Research, 47, 185–194, https://doi.org/10.1093/forestry/47.2.185, 1974.
Panofsky, H. and Dutton, J.: Atmospheric Turbulence, Wiley, New York, ISBN 10: 0471057142, 1984.
Pardowitz, T., Osinski, R., Kruschke, T., and Ulbrich, U.: An analysis of uncertainties and skill in forecasts of winter storm losses, Nat. Hazards Earth Syst. Sci., 16, 2391–2402, https://doi.org/10.5194/nhess-16-2391-2016, 2016.
Parker, D.: Secondary frontal waves in the North Atlantic region: a dynamical perspective of current ideas, Q. J. Roy. Meteor. Soc., 124, 829–856, 1998.
Parodi, A., Lagasio, M., Maugeri, M., Turato, B., and Gallus, W.: Observational and modelling study of a major downburst event in Liguria: The 14 October 2016 case, Atmosphere, 10, 1–17, https://doi.org/10.3390/ATMOS10120788, 2019.
Pasztor, F., Matulla, C., Zuvela-Aloise, M., Rammer, W., and Lexer, M.: Developing predictive models of wind damage in Austrian forests, Ann. Forest Sci., 72, 289–301, https://doi.org/10.1007/s13595-014-0386-0, 2015.
Pejovic, T., Williams, V. A., Noland, R. B., and Toumi, R.: Factors Affecting the Frequency and Severity of Airport Weather Delays and the Implications of Climate Change for Future Delays, Transp. Res. Record, 2139, 97–106, https://doi.org/10.3141/2139-12, 2009.
Peltola, H.: Swaying of trees in response to wind and thinning in a stand of Scots pine, Bound.-Lay. Meteorol., 77, 285–304, https://doi.org/10.1007/BF00123529, 1996.
Peltola, H.: Mechanical stability of trees under static loads, Am. J. Bot., 93, 1501–1511, https://doi.org/10.3732/ajb.93.10.1501, 2006.
Peltola, H. and Kellomaki, S.: A mechanistic model for calculating windthrow and stem breakage of Scots pines at stand edge, Silva Fenn., 27, 99–111, 1993.
Peltola, H., Kellomäki, S., Väisänen, H., and Ikonen, V.-P.: A mechanistic model for assessing the risk of wind and snow damage to single trees and stands of Scots pine, Norway spruce, and birch, Can. J. Forest Res., 29, 647–661, https://doi.org/10.1139/x99-029, 1999.
Peña-Angulo, D., Reig-Gracia, F., Domínguez-Castro, F., Revuelto, J., Aguilar, E., van der Schrier, G., and Vicente-Serrano, S. M.: ECTACI: European Climatology and Trend Atlas of Climate Indices (1979–2017), J. Geophys. Res.-Atmos., 125, e2020JD032798, https://doi.org/10.1029/2020JD032798, 2020.
Peterson, C.: Catastrophic wind damage to North American forests and the potential impact of climate change, Sci. Total Environ., 262, 287–311, https://doi.org/10.1016/S0048-9697(00)00529-5, 2000.
Peterson, C., Ribeiro, G., Negrón-Juárez, R., Marra, D., Chambers, J., Higuchi, N., Lima, A., and Cannon, J.: Critical wind speed suggest wind could be an important disturbance agent in Amazonian forests, Forestry: An International Journal of Forest Research, 92, 444–459, 2019.
Petrović, V. and Bottasso, C.: Wind turbine optimal control during storms, J. Phys. Conf. Ser., 524, 012052, https://doi.org/10.1088/1742-6596/524/1/012052, 2014.
Pinto, J. G., Spangehl, T., Ulbrich, U., and Speth, P.: Sensitivities of a cyclone detection and tracking algorithm: individual tracks and climatology, Meteorol. Z., 14, 823–838, https://doi.org/10.1127/0941-2948/2005/0068, 2005.
Pinto, J. G., Karremann, M., Born, K., Della-Marta, P., and Klawa, M.: Loss potentials associated with European windstorms under future climate conditions, Clim. Res., 54, 1–20, https://doi.org/10.3354/cr01111, 2012.
Pinto, J. G., Gómara, I., Masato, G., Dacre, H., Woollings, T., and Caballero, R.: Large-scale dynamics associated with clustering of extratropical cyclones affecting Western Europe, J. Geophys. Res., 119, 13704–13719, https://doi.org/10.1002/2014JD022305, 2014.
Prahl, B. F., Rybski, D., Kropp, J., Burghoff, O., and Held, H.: Applying stochastic small-scale damage functions to German winter storms: Damage functions for German storm loss, Geophys. Res. Lett., 39, L06806, https://doi.org/10.1029/2012GL050961, 2012.
Prahl, B. F., Rybski, D., Burghoff, O., and Kropp, J. P.: Comparison of storm damage functions and their performance, Nat. Hazards Earth Syst. Sci., 15, 769–788, https://doi.org/10.5194/nhess-15-769-2015, 2015.
Priestley, M., Ackerley, D., Catto, J., Hodges, K., McDonald, R., and Lee, R.: An Overview of the Extratropical Storm Tracks in CMIP6 Historical Simulations, J. Climate, 33, 6315–6343, 2020a.
Priestley, M., Dacre, H., Shaffrey, L., Schemm, S., and Pinto, J. G.: The role of secondary cyclones and cyclone families for the North Atlantic storm track and clustering over western Europe, Q. J. Roy. Meteor. Soc., 146, 1184–1205, https://doi.org/10.1002/qj.3733, 2020b.
Primo, C.: Wind gust warning verification, Adv. Sci. Res., 13, 113–120, https://doi.org/10.5194/asr-13-113-2016, 2016.
Pryor, S. and Barthelmie, R.: Climate change impacts on wind energy: A review, Renewable and Sustainable Energy Reviews, 14, 430–437, https://doi.org/10.1016/j.rser.2009.07.028, 2010.
Quaschning, V.: Understanding renewable energy systems, Routledge, https://doi.org/10.4324/9781315769431, 2016.
Quayle, R. and Steadman, R.: The Steadman Wind Chill: An Improvement over Present Scales, Weather Forecast., 13, 1187–1193, https://doi.org/10.1175/1520-0434(1998)013<1187:TSWCAI>2.0.CO;2, 1998.
Queck, R., Bienert, A., Maas, H.-G., Harmansa, S., Goldberg, V., and Bernhofer, C.: Wind fields in heterogeneous conifer canopies: parameterisation of momentum absorption using high-resolution 3D vegetation scans, Eur. J. Forest Res., 131, 165–176, https://doi.org/10.1007/s10342-011-0550-0, 2012.
Queck, R., Bernhofer, C., Bienert, A., and Schlegel, F.: The TurbEFA Field Experiment – Measuring the Influence of a Forest Clearing on the Turbulent Wind Field, Bound.-Lay. Meteorol., 160, 397–423, https://doi.org/10.1007/s10546-016-0151-z, 2016.
Qin, L., Liu, Q., Zhang, M., and Saeed, S.: Effect of measurement errors on the estimation of tree biomass, Can. J. Forest Res., 49, 1371–1378, https://doi.org/10.1139/cjfr-2019-0034, 2019.
Quine, C. and White, I.: Revised windiness scores for the windthrow hazard classification: the revised scoring method, Research Information Note 230, Research Division of the Forestry Authority, https://cdn.forestresearch.gov.uk/2022/02/rin230.pdf (31 May 2023), 1993.
Quine, C. and White, I.: The potential of distance-limited topex in the prediction of site windiness, Forestry: An International Journal of Forest Research, 71, 325–332, 1998.
Quine, C., Gardiner, B., and Moore, J.: Chapter Four - Wind disturbance in forests: The process of wind created gaps, tree overturning, and stem breakage, in: Plant Disturbance Ecology, Elsevier, New York, 117–184, https://doi.org/10.1016/b978-0-12-818813-2.00004-6, 2020.
Radinovic, D.: Mediterranean cyclones and their influence on the weather and climate, PSMP Rep Series 24, WMO, Geneva, Switzerland, 131 pp., 1987.
Rajkumara, S.: Lodging in cereals – a review, Agricultural Reviews, 29, 55–60, 2008.
Raupach, M., Finnigan, J., and Brunet, Y.: Coherent eddies and turbulence in vegetation canopies: the mixing-layer analogy, Bound.-Lay. Meteorol., 78, 351–382, 1996.
R Core Team: R: A language and environment for statistical computing, R Foundation for Statistical Computing, Vienna, Austria, https://www.R-project.org/ (last access: 1 May 2023), 2020.
Ritter, M., Shen, Z., López Cabrera, B., Odening, M., and Deckert, L.: Designing an index for assessing wind energy potential, Renew. Energ., 83, 416–424, https://doi.org/10.1016/j.renene.2015.04.038, 2015.
Roberts, J. F., Champion, A. J., Dawkins, L. C., Hodges, K. I., Shaffrey, L. C., Stephenson, D. B., Stringer, M. A., Thornton, H. E., and Youngman, B. D.: The XWS open access catalogue of extreme European windstorms from 1979 to 2012, Nat. Hazards Earth Syst. Sci., 14, 2487–2501, https://doi.org/10.5194/nhess-14-2487-2014, 2014.
Romanic, D. and Hangan, H.: Experimental investigation of the interaction between near-surface atmospheric boundary layer winds and downburst outflows, J. Wind Eng. Ind. Aerod., 205, 104323, https://doi.org/10.1016/j.jweia.2020.104323, 2020.
Rouse, R. and Hodges, L.: Windbreaks, Agronomy and Horticulture Department, Agronomy and Horticulture – Faculty Publications, ISBN: 0935817875, 2004.
Rudnicki, M., Mitchell, S., and Novak, M.: Wind tunnel measurements of crown streamlining and drag relationships for three conifer species, Can. J. Forest Res., 34, 666–676, https://doi.org/10.1139/x03-233, 2004.
Ruel, J., Pin, D., Spacek, L., Cooper, K., and Benoit, R.: The estimation of wind exposure for windthrow hazard rating: comparison between Strongblow, MC2, Topex and a wind tunnel study, Forestry: An International Journal of Forest Research, 70, 253–266, https://doi.org/10.1093/forestry/70.3.253, 1997.
Ruy, M., Gonçalves, R., and Vicente, W.: Effect of dimensional variables on the behavior of trees for biomechanical studies, Appl. Sci., 12, 3815, https://doi.org/10.3390/app12083815, 2022.
Schindler, D. and Kolbe, S.: Assessment of the Response of a Scots Pine Tree to Effective Wind Loading, Forests, 11, 145, https://doi.org/10.3390/f11020145, 2020.
Schindler, D. and Mohr, M.: No resonant response of Scots pine trees to wind excitation, Agr. Forest Meteorol., 265, 227–244, https://doi.org/10.1016/j.agrformet.2018.11.021, 2019.
Schindler, D., Grebhan, K., Albrecht, A., Schönborn, J., and Kohnle, U.: GIS-based estimation of the winter storm damage probability in forests: A case study from Baden-Wuerttemberg (Southwest Germany), Int. J. Biometeorol., 56, 57–69, https://doi.org/10.1007/s00484-010-0397-y, 2012.
Schmidt, P.: Lastannahmen – Einwirkungen auf Tragwerke: Grundlagen und Anwendung nach EC 1, Springer Vieweg, ISBN: 978-3-8348-9855-5, 2019.
Schultz, D., Bosart, L., Colle, B., Davies, H., Dearden, C., Keyser, D., Martius, O., Roebber, P., Steenburgh, W., Volkert, H., and Winters, A.: Extratropical cyclones: a century of research on meteorology's centerpiece. Meteor. Mon., 59, 16–1, 2019.
Schütz, J., Götz, M., Schmid, W., and Mandallaz, D.: Vulnerability of spruce (Picea abies) and beech (Fagus sylvatica) forest stands to storms and consequences for silviculture, Eur. J. Forest Res., 125, 291–302, https://doi.org/10.1007/s10342-006-0111-0, 2006.
Schweighofer, J.: The impact of extreme weather and climate change on inland waterway transport, Nat. Hazards, 72, 23–40, https://doi.org/10.1007/s11069-012-0541-6, 2014.
Scott, R. and Mitchell, S.: Empirical modelling of windthrow risk in partially harvested stands using tree, neighbourhood, and stand attributes, Forest Ecol. Manag., 218, 193–209, https://doi.org/10.1016/j.foreco.2005.07.012, 2005.
Seneviratne, S. I., Zhang, X., Adnan, M., Badi, W., Dereczynski, C., Di Luca, A., Ghosh, S., Iskandar, I., Kossin, J., Lewis, S., Otto, F., Pinto, I., Satoh, M., Vicente-Serrano, S. M., Wehner, M., and Zhou, B.: Weather and Climate Extreme Events in a Changing Climate, in: Climate Change 2021: The Physical Science Basis. Contribution of Working Group I to the Sixth Assessment Report of the Intergovernmental Panel on Climate Change, edited by: Masson-Delmotte, V., Zhai, P., Pirani, A., Connors, S. L., Péan, C., Berger, S., Caud, N., Chen, Y., Goldfarb, L., Gomis, M. I., Huang, M., Leitzell, K., Lonnoy, E., Matthews, J. B. R., Maycock, T. K., Waterfield, T., Yelekçi, O., Yu, R., and Zhou, B., Cambridge University Press, Cambridge, United Kingdom and New York, NY, USA, 1513–1766, https://www.ipcc.ch/report/ar6/wg1/chapter/chapter-11/ (last access: 1 May 2023), 2021.
Shahabinejad, N., Mahmoodabadi, M., Jalalian, A., and Chavoshi, E.: In situ field measurement of wind erosion and threshold velocity in relation to soil properties in arid and semiarid environments, Environ. Earth Sci., 78, https://doi.org/10.1007/s12665-019-8508-5, 2019.
Shapiro, M. and Keyser, D.: Fronts, jet-streams and the tropopause, in: Extratropical cyclones, American Meteorological Society, Boston, MA, 167–191, https://doi.org/10.1007/978-1-944970-33-8_10, 1990.
Sharples, J.: Review of formal methodologies for wind-slope correction of wildfire rate of spread, Int. J. Wildland Fire, 17, 179–193, 2008.
Sissakian, V., Al-Ansari, N., and Knutsson, S.: Sand and dust storm events in Iraq, Natural Science, 5, 1084–1094, 2013.
Sivakumar, M.: Impacts of sand storms/dust storms on agriculture, in: Natural Disasters and Extreme Events in Agriculture, Springer, Berlin, Heidelberg, 159–177, https://doi.org/10.1007/3-540-28307-2_10, 2005.
Slot, R. M. M., Sørensen, J. D., Svenningsen, L., Moser, W., and Thøgersen, M. L.: Effective turbulence and its implications in wind turbine fatigue assessment, Wind Energy, 22, 1699–1715, https://doi.org/10.1002/we.2397, 2019.
SMHI: SMHI Warnings and advisories, https://www.smhi.se/en/weather/sweden-weather/warnings, last access: 10 January 2023.
Snæbjörnsson, J., Baker, C., and Sigbjörnsson, R.: Probabilistic assessment of road vehicle safety in windy environments, J. Wind Eng. Ind. Aerodyn., 95, 1445–1462, https://doi.org/10.1016/j.jweia.2007.02.020, 2007.
Spatz, H.-C. and Theckes, B.: Oscillation damping in trees, Plant Sci., 207, 66–71, https://doi.org/10.1016/j.plantsci.2013.02.015, 2013.
Sprenger, M., Schmidli, J., and Egloff, L.: The Laseyer wind storm-case studies and a climatology, Meteorol. Z., 27, 15–32, https://doi.org/10.3929/ethz-b-000239023, 2018.
Stefanski, R. and Sivakumar, M.: Impacts of sand and dust storms on agriculture and potential agricultural applications of a SDSWS, IOP C. Ser. Earth Env., 7, p. 12016, https://doi.org/10.1088/1755-1307/7/1/012016, 2009.
Stoll, R., Gibbs, J. A., Salesky, S. T., Anderson, W., and Calaf, M.: Large-Eddy Simulation of the Atmospheric Boundary Layer, Bound.-Lay. Meteorol., 177, 541–581, https://doi.org/10.1007/s10546-020-00556-3, 2020.
Stull, R.: An introduction to boundary layer meteorology, Springer Dordrecht, https://doi.org/10.1007/978-94-009-3027-8, 1988.
Stull, R.: Practical Meteorology: An Algebra-based Survey of Atmospheric Science, Black and White edn., Sundog Publishing, LLC, Vancouver, 942 pp., ISBN 978-0-88865-283-6, 2017.
Suárez, J., Gardiner, B., and Quine, C.: A comparison of three methods for predicting wind speed in complex forested terrain, Meteorol. Appl., 6, 329–342, 1999.
Sulik, S. and Kejna, M.: The origin and course of severe thunderstorm outbreaks in Poland on 10 and 11 August, 2017, Bulletin of Geography, Physical Geography Series, 18, 25–39, https://doi.org/10.2478/bgeo-2020-0003, 2020.
Suvanto, S., Henttonen, H., Nöjd, P., and Mäkinen, H.: Forest susceptibility to storm damage is affected by similar factors regardless of storm type: Comparison of thunder storms and autumn extra-tropical cyclones in Finland, Forest Ecol. Manag., 381, 17–28, https://doi.org/10.1016/j.foreco.2016.09.005, 2016.
Suvanto, S., Henttonen, H., Nöjd, P., and Mäkinen, H.: High-resolution topographical information improves tree-level storm damage models, Can. J. Forest Res., 48, 721–728, https://doi.org/10.1139/cjfr-2017-0315, 2018.
Takemi, T., Yoshida, T., Horiguchi, M., and Vanderbauwhede, W.: Large-Eddy-simulation analysis of airflows and strong wind hazards in urban areas, Urban Climate, 32, 100625, https://doi.org/10.1016/j.uclim.2020.100625, 2020.
Tamura, Y.: Wind-induced damage to buildings and disaster risk reduction, in: The Seventh Asia-Pacific Conference on Wind Engineering, Taipei, Taiwan, 8–12 November 2009, 23 pp., 2009.
Taszarek, M., Allen, J. T., Brooks, H. E., Pilguj, N., and Czernecki, B.: Differing trends in United States and European severe thunderstorm environments in a warming climate, B. Am. Meteorol. Soc., 102, E296–E322, https://doi.org/10.1175/BAMS-D-20-0004.1, 2021.
Taylor, A., Dracup, E., MacLean, D., Boulanger, Y., and Endicott, S.: Forest structure more important than topography in determining windthrow during Hurricane Juan in Canada's Acadian Forest, Forest Ecol. Manag., 434, 255–263, https://doi.org/10.1016/j.foreco.2018.12.026, 2019.
Telewski, F.: 14 - Wind-induced physiological and developmental responses in trees, in: Wind and Trees, edited by: Grace, J. and Coutts, M., Cambridge University Press, 237–263, https://doi.org/10.1017/CBO9780511600425.015, 1995.
Theofilatos, A. and Yannis, G.: A review of the effect of traffic and weather characteristics on road safety, Accident Anal. Prev., 72, 244–256, https://doi.org/10.1016/j.aap.2014.06.017, 2014.
Thornes, J. and Davis, B.: Mitigating the impact of weather and climate on railway operations in the UK, in: ASME/IEEE Joint Railroad Conference, Washington, DC, USA, 23–25 April 2002, IEEE, 29–38, https://doi.org/10.1109/RRCON.2002.1000089, 2002.
Tomas-Burguera, M., Vicente-Serrano, S. M., Peña-Angulo, D., Domínguez-Castro, F., Noguera, I., and El Kenawy, A.: Global Characterization of the Varying Responses of the Standardized Precipitation Evapotranspiration Index to Atmospheric Evaporative Demand, J. Geophys. Res.-Atmos, 125, e2020JD033017, https://doi.org/10.1029/2020JD033017, 2020.
Tomczak, A., Jelonek, T., Grzywiński, W., Mania, P., Tomczak, K., and Pazdrowski, W.: The Effects of Wind Exposure on Scots Pine Trees: Within-Stem Variability of Wood Density and Mechanical Properties, Forests, 11, 1095, https://doi.org/10.3390/f11101095, 2020.
Torshizi, M., Miri, A., Shahriari, A., Dong, Z., and Davidson-Arnott, R.: The effectiveness of a multi-row Tamarix windbreak in reducing aeolian erosion and sediment flux, Niatak area, Iran, J. Environ. Manage., 265, 110486, https://doi.org/10.1016/j.jenvman.2020.110486, 2020.
Torun, P. and Altunel, A.: Effects of environmental factors and forest management on landscape-scale forest storm damage in Turkey, Ann. Forest Sci., 77, 1–13, https://doi.org/10.1007/s13595-020-00945-1, 2020.
Trigo, I., Davies, T., and Bigg, G.: Objective climatology of cyclones in the Mediterranean region, J. Climate, 12, 1685–1696, 1999.
Troen, I. and Petersen, E.: European Wind Atlas, 1st ed., Risø National Laboratory, Roskilde, ISBN: 87-550-1482-8, 1989.
TT Club, ICHCA International, and Port Equipment Manufacturers Association: Recommended minimum safety specifications for quay container cranes, TT Club (in collaboration with ICHCA International and PEMA), https://www.ttclub.com/-/media/files/tt-club/port-equipment-information-papers/ recommended-minimum-safety-features-for-quay-container-cranes.pdf (last access: 14 December 2021), 2011.
Uchida, T. and Kawashima, Y.: New Assessment Scales for Evaluating the Degree of Risk of Wind Turbine Blade Damage Caused by Terrain-Induced Turbulence, Energies, 12, 2624, https://doi.org/10.3390/en12132624, 2019.
Uchida, T. and Li, G.: Comparison of RANS and LES in the Prediction of Airflow Field over Steep Complex Terrain, Open Journal of Fluid Dynamics, 8, 286, https://doi.org/10.4236/ojfd.2018.83018, 2018.
Uchida, T. and Ohya, Y.: Large-eddy simulation of turbulent airflow over complex terrain, J. Wind Eng. Ind. Aerod., 91, 219–229, https://doi.org/10.1016/S0167-6105(02)00347-1, 2003.
Uchida, T. and Ohya, Y.: Micro-siting technique for wind turbine generators by using large-eddy simulation, J. Wind Eng. Ind. Aerodyn., 96, 2121–2138, https://doi.org/10.1016/j.jweia.2008.02.047, 2008.
Uchida, T. and Ohya, Y.: Latest Developments in Numerical Wind Synopsis Prediction Using the RIAM-COMPACT® CFD Model – Design Wind Speed Evaluation and Wind Risk (Terrain-Induced Turbulence) Diagnostics in Japan, Energies, 4, 458–474, https://doi.org/10.3390/en4030458, 2011.
Uchida, T. and Sugitani, K.: Numerical and Experimental Study of Topographic Speed-Up Effects in Complex Terrain, Energies, 13, 3896, https://doi.org/10.3390/en13153896, 2020.
Ulbrich, U., Leckebusch, G., and Pinto, J. G.: Extra-tropical cyclones in the present and future climate: a review, Theor. Appl. Climatol., 96, 117–131, https://doi.org/10.1007/s00704-008-0083-8, 2009.
United Nations Department of Economic and Social Affairs: World Population Prospects 2022: Summary of Results, United Nations Department of Economic and Social Affairs, UN DESA/POP/2022/TR/NO. 3, Population Division, New York, https://population.un.org/wpp/Publications/ (last access: 1 May 2023), 2022.
University of Bonn: COSMO-REA2 data set, University of Bonn [data set], https://reanalysis.meteo.uni-bonn.de/?Download_Data___COSMO-REA2, last access 31 May 2023.
Usbeck, T., Wohlgemuth, T., Pfister, C., Volz, R., Beniston, M., and Dobbertin, M.: Wind speed measurements and forest damage in Canton Zurich (Central Europe) from 1891 to winter 2007: Wind Speed Measurements and Forest Damage in Canton Zurich, Int. J. Climatol., 30, 347–358, https://doi.org/10.1002/joc.1895, 2010.
Vajda, A., Tuomenvirta, H., Juga, I., Nurmi, P., Jokinen, P., and Rauhala, J.: Severe weather affecting European transport systems: the identification, classification and frequencies of events, Nat. Hazard., 72, 169–188, https://doi.org/10.1007/s11069-013-0895-4, 2014.
van Oldenborgh, G. J., Krikken, F., Lewis, S., Leach, N. J., Lehner, F., Saunders, K. R., van Weele, M., Haustein, K., Li, S., Wallom, D., Sparrow, S., Arrighi, J., Singh, R. K., van Aalst, M. K., Philip, S. Y., Vautard, R., and Otto, F. E. L.: Attribution of the Australian bushfire risk to anthropogenic climate change, Nat. Hazards Earth Syst. Sci., 21, 941–960, https://doi.org/10.5194/nhess-21-941-2021, 2021.
Van Wagner, C. and Forest, P.: Development and structure of the Canadian forest fire weather index system, Canadian Forestry Service, Forestry Tech. Rep., 35 pp., https://cfs.nrcan.gc.ca/publications?id=19927 (last access: 1 May 2023), 1987.
Verkaik, J. W.: Evaluation of Two Gustiness Models for Exposure Correction Calculations, J. Appl. Meteorol., 39, 1613–1626, https://doi.org/10.1175/1520-0450(2000)039<1613:EOTGMF>2.0.CO;2, 2000.
Vitolo, R., Stephenson, D., Cook, I., and Mitchell-Wallace, K.: Serial clustering of intense European storms, Meteorol. Z, 18, 411–424, 2009.
Vogel, S.: Drag and Reconfiguration of Broad Leaves in High Winds, J. Exp. Bot., 40, 941–948, 1989.
Vollsinger, S., Mitchell, S. J., Byrne, K. E., Novak, M. D., and Rudnicki, M.: Wind tunnel measurements of crown streamlining and drag relationships for several hardwood species, Can. J. Forest Res., 35, 1238–1249, https://doi.org/10.1139/x05-051, 2005.
Wahl, S., Bollmeyer, C., Crewell, S., Figura, C., Friederichs, P., Hense, A., Keller, J. D., and Ohlwein, C.: A novel convective-scale regional reanalysis COSMO-REA2: Improving the representation of precipitation, Meteorol. Z., 26, 345–361, https://doi.org/10.1127/metz/2017/0824, 2017.
Wahl, T., Jain, S., Bender, J., Meyers, S., and Luther, M.: Increasing risk of compound flooding from storm surge and rainfall for major US cities, Nat. Clim. Change, 5, 1093–1097, https://doi.org/10.1038/nclimate2736, 2015.
Wang, B., Xu, Y., Zhu, L., and Li, Y.: Crosswind effect studies on road vehicle passing by bridge tower using computational fluid dynamics, Eng. Appl. Comp. Fluid, 8, 330–344, https://doi.org/10.1080/19942060.2014.11015519, 2014.
Wallace, J. and Hobbs, P.: Atmospheric science: an introductory survey, Vol. 92, Elsevier, ISBN: 9780127329512, 2006.
Welker, C., Röösli, T., and Bresch, D. N.: Comparing an insurer's perspective on building damages with modelled damages from pan-European winter windstorm event sets: a case study from Zurich, Switzerland, Nat. Hazards Earth Syst. Sci., 21, 279–299, https://doi.org/10.5194/nhess-21-279-2021, 2021.
Weninger, T., Scheper, S., Lackóová, L., Kitzler, B., Gartner, K., King, N. W., Cornelis, W., Strauss, P., and Michel, K.: Ecosystem services of tree windbreaks in rural landscapes–a systematic review, Environ. Res. Lett., 16, 103002, https://doi.org/10.1088/1748-9326/ac1d0d, 2021.
Wiréhn, L., Käyhkö, J., Neset, T. S., and Juhola, S.: Analysing trade-offs in adaptation decision-making – agricultural management under climate change in Finland and Sweden, Reg. Environ. Change, 20, 1–14, 2020.
Woollings, T., Hannachi, A., and Hoskins, B.: Variability of the North Atlantic eddy-driven jet-stream, Q. J. Roy. Meteor. Soc., 136, 856–868, https://doi.org/10.1002/qj.625, 2010.
Young, I.: Seasonal variability of the global ocean wind and wave climate, Int. J. Climatol., 19, 931–950, https://doi.org/10.1002/(SICI)1097-0088(199907)19:9<931::AID-JOC412>3.0.CO;2-O, 1999.
Zappa, G., Shaffrey, L., Hodges, K., Sansom, P., and Stephenson, D.: A multimodel assessment of future projections of North Atlantic and European extratropical cyclones in the CMIP5 climate models, J. Climate, 26, 5846–5862, 2013.
Zscheischler, J., Westra, S., van den Hurk, B., Seneviratne, S., Ward, P., Pitman, A., AghaKouchak, A., Bresch, D., Leonard, M., Wahl, T., and Zhang, X.: Future climate risk from compound events, Nat. Clim. Change, 8, 469–477, https://doi.org/10.1038/s41558-018-0156-3, 2018.
Zscheischler, J., Martius, O., Westra, S., Bevacqua, E., Raymond, C., Horton, R. M., van den Hurk, B., AghaKouchak, A., Jézéquel, A., Mahecha, M. D., Maraun, D., Ramos, A., Ridder, N., Thiery, W., and Vignotto, E.: A typology of compound weather and climate events, Nature Reviews Earth & Environment, 1, 333–347, https://doi.org/10.1038/s43017-020-0060-z, 2020.
- Abstract
- General introduction
- Wind and storm formation – mechanisms and concepts
- Wind–surface interaction
- Wind- and storm-related indices and critical thresholds
- Outlook and open research questions
- Code availability
- Data availability
- Author contributions
- Competing interests
- Disclaimer
- Special issue statement
- Acknowledgements
- Financial support
- Review statement
- References
- Supplement
- Abstract
- General introduction
- Wind and storm formation – mechanisms and concepts
- Wind–surface interaction
- Wind- and storm-related indices and critical thresholds
- Outlook and open research questions
- Code availability
- Data availability
- Author contributions
- Competing interests
- Disclaimer
- Special issue statement
- Acknowledgements
- Financial support
- Review statement
- References
- Supplement