the Creative Commons Attribution 4.0 License.
the Creative Commons Attribution 4.0 License.
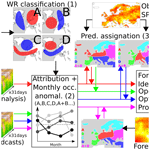
On the use of weather regimes to forecast meteorological drought over Europe
Christophe Lavaysse
Jürgen Vogt
Andrea Toreti
Marco L. Carrera
Florian Pappenberger
An early warning system for drought events can provide valuable information for decision makers dealing with water resources management and international aid. However, predicting such extreme events is still a big challenge. In this study, we compare two approaches for drought predictions based on forecasted precipitation derived from the Ensemble extended forecast model (ENS) of the ECMWF, and on forecasted monthly occurrence anomalies of weather regimes (MOAWRs), also derived from the ECMWF model.
Results show that the MOAWRs approach outperforms the one based on forecasted precipitation in winter in the north-eastern parts of the European continent, where more than 65 % of droughts are detected 1 month in advance. The approach based on forecasted precipitation achieves better performance in predicting drought events in central and eastern Europe in both spring and summer, when the local atmospheric forcing could be the key driver of the precipitation. Sensitivity tests also reveal the challenges in predicting small-scale droughts and drought onsets at longer lead times.
Finally, the results show that the ENS model of the ECMWF successfully represents most of the observed linkages between large-scale atmospheric patterns, depicted by the weather regimes and drought events over Europe.
- Article
(7160 KB) - Full-text XML
-
Supplement
(4016 KB) - BibTeX
- EndNote
Developing a robust early warning system for drought events is a key challenge for modellers and forecasters. The timescale of these events (generally from 1 to several months) requires accurate numerical weather forecasts with long lead times. Due to the uncertainties of the models, the chaotic nature of the atmospheric circulation and the errors in the initial conditions, the reliability of precipitation forecasts is close to climatology beyond 2-week lead time (Haiden et al., 2017; Vigaud et al., 2017). In a recent study (Lavaysse et al., 2015), it has been shown that about 40 % of the meteorological droughts, defined by an anomaly of the standardized precipitation index (SPI), can be detected 1 month in advance by using the forecasted precipitation provided by the ECMWF Ensemble extended forecast model (ENS). These forecasts might be improved by using post-processing techniques or predictors that are better simulated by atmospheric models (Ferranti et al., 2018; Lavers et al., 2016a, b).
The concept of weather regimes (WRs) was first introduced in the early 1950s on the assumption that the atmosphere evolves between a finite number of large-scale circulation states. It is based on recurrent, persistent and/or quasi stationary states of the atmosphere, generally diagnosed with anomalies of geopotential heights at 500 hPa (Michelangeli et al., 1995; Stephenson et al., 2004). First and principally studied in wintertime, when they are more stronger, four main states have been defined, namely the positive North Atlantic Oscillation phases (NAO+), the negative NAO (NAO−), the blocking regime and the Atlantic Ridge regime. They are well known to play an important role in creating large-scale conditions that either favour or inhibit precipitation in Europe (Plaut and Simonnet, 2001; Yiou and Nogaj, 2004), especially in extreme events (Boé, 2013; Cattiaux et al., 2010; Guérémy et al., 2012; Toreti et al., 2010; Yiou and Cattiaux, 2013). These impacts can be observed in both winter and summer (Pfahl, 2014). In Europe, well-identifiable spatial patterns of surface temperature and precipitation are associated with each regime. For instance, the NAO+ in winter is linked to above-normal temperature and precipitation over northern Europe and below-normal precipitation over southern and central Europe (Hurrell et al., 2013; Wanner et al., 2001). The opposite results of surface temperature and precipitation anomalies are generally observed during NAO− phases. The WRs can also drive extreme events. The NAO+ regime favours heavy precipitation in northern Europe and periods of drought in the Mediterranean area. The blocking regime determines the occurrence of dry periods in large parts of southern Scandinavia and central Europe (Yiou and Nogaj, 2004) and influences the heatwave occurrences in Russia and northern Europe (Schaller et al., 2018). The use of WRs is also interesting, since their occurrence and variability are connected to SST anomalies (Häkkinen et al., 2011; Peings and Magnusdottir, 2014; Zampieri et al., 2017) and thus somehow implicitly takes into account the Atlantic Ocean influence. The practical interest in classifying large-scale geopotential anomalies into a few pre-defined patterns relies on the fact that local weather conditions depend on large-scale atmospheric flows. If WRs can be better represented and forecasted by general circulation models (GCMs), they would provide additional information for local weather anomalies via statistical downscaling techniques, which derives linkages between large-scale geopotential anomalies (i.e. the WRs) and local weather phenomena (i.e. precipitation anomalies) for medium-range lead times.
Since geopotential and temperature fields are generally better forecasted than precipitation in numerical weather prediction systems (Vitart, 2014), and since long-term droughts are mainly driven by large-scale forcing (Kingston et al., 2015), the benefit of using WR occurrences as predictors of meteorological drought is analyzed with regard to the precipitation forecasts. The paper is organized into six sections. The data sets and the methods are presented in Sect. 2, and the different forecast methods are in Sect. 3. The comparison of predictability scores obtained by using precipitation and MOAWRs is provided in Sect. 4. The sources of uncertainties are then discussed in Sect. 5 and the main conclusions are drawn in Sect. 6.
2.1 Data sets
The observed daily cumulated precipitation data are retrieved from the European Climate Assessment & Dataset (ECA & D) and the ENS gridded data set (E-OBS) version 12, which provides daily station-based precipitation and temperature data on regular grids (Haylock et al., 2008). While the full E-OBS resolution is 0.25∘, here data have been upscaled by averaging to 1∘ due to the specific focus on large-scale drought with significant socio-economic impacts. E-OBS data are available from 1950 to the present.
Atmospheric predictors are identified by using the geopotential height at 500 hPa. The daily geopotential is derived from the ERA-Interim reanalysis (ERAI, Dee et al., 2011) with a spatial resolution of 1.125∘ covering the period from 1979 to the present.
The forecast products (precipitation and 500 hPa geopotential height) are derived from the ENS of the ECMWF (Molteni et al., 1996). The ENS is the latest version of the ECMWF Ensemble extended forecast model and is composed of one unperturbed member and 50 perturbed members, distinguished by different initial conditions and representations of model uncertainties. In addition to these forecasts, the ECMWF produces hindcasts that are initialized on the same date of the ENS for the last 20 years with five members only. In 2012, it was extended once a week to a 32-day lead time and the horizontal resolution varies from Tl639 (32 km) from t+0 to t+10 days to Tl319 (64 km) from t+11 to t+32 days. All of these data sets have been re-gridded onto a regular grid of 1∘ resolution based on an averaging upscaling method. The rationale of using coarser resolution is (i) to detect and so focus on larger-scale precipitation deficits and (ii) to take into account spatial bias in the model that could detect the right precipitation signal but with a slight spatial phasing error.
In order to build the baseline (following a normalization technique) and to have a time series long enough to calculate the scores, 21 years of hindcasts (November 1992 to November 2013) of the forecasts (November 2012 to November 2014) are used. To be coherent, the data sets of observed precipitation and the WR calculations (from ERAI) are restricted to the same period.
2.2 Weather regimes
In order to build the forecasts based on predictors (illustrated in Fig. S1 in the Supplement), the WR classification (i.e. definition of the WR patterns) is done exclusively using ERAI, by a K-means method nested within a genetic algorithm to avoid dependence on the initial conditions and the trap of the local minima (Toreti et al., 2010). The four meteorological seasons are treated independently: winter (December to February), spring (March to May), summer (June to August) and autumn (September to November) but to avoid inconsistency when moving from one season to another, each season is extended by adding the last month of the previous season. This method of classification has been extensively used (Michelangeli et al., 1995; Robertson and Ghil, 1999; Santos et al., 2005), but because in this study the WR classification needs to fit specific requests (20-year moving period of the hindcast, the four seasons), it is important to regenerate this classification. Nevertheless, the patterns of the geopotential anomalies (shown in Fig. S2) are strongly similar to those obtained in aforementioned studies. The choice of using only the ERAI classification and not ENS is justified by (i) looking at previous studies that have shown the relatively similar behaviour of ERAI and ENS forecasts (Ferranti et al., 2015) and (ii) considering that this choice avoids inconsistency (or the impossibility to derive a coherent classification) due to the continuous evolution of the ENS model. Four WRs are identified in winter and spring, while three WRs are detected in summer and autumn (see Fig. S2). The number of WRs is estimated by following Toreti et al. (2010) and depends both on the period (here from 1992 to 2013) and the region (North Atlantic) studied. This is why the number of summer WRs is different, e.g. with respect to Cassou et al. (2005).
Then, an assignation procedure is run to identify the closest WR to a given daily geopotential anomaly of ERAI and a given ENS member. To this aim, the method proposed by Ferranti et al. (2015) is applied here. Namely, a pattern-matching algorithm based on the minimum distance from the previously identified centroids is used to assign each day and individual forecast member to the closest weather regime. The climatology of the forecasted WRs is then calculated by summing the daily classification of each WR for all of the members and all the days inside a 30-day window. The same climatology is derived by using ERAI. The monthly occurrence anomaly of WRs (MOAWRs) is then calculated with respect to the climatological occurrences based on the hindcast period (1992–2013) and obtained by using ERAI and ENS independently.
To potentially increase the signal emerging from the linkage between MOAWRs and precipitation anomalies, different combinations (additions and subtractions of WR occurrences) of two WRs are tested. This could be useful when two WRs have the same or opposite impacts on precipitation. For example, in the case of two regimes, WRa and WRb, which are respectively associated with dry and wet conditions over a certain region, the occurrence difference between the two WRs could be more linked to the drought events over that region (this example will be discussed later in the document). In total, a set of 6 to 12 combinations (see the list in Table 1) is tested when three or four WRs (depending the season) are detected respectively.
2.3 Drought metrics
As suggested by the World Meteorological Organization (Svoboda et al., 2012), the standardized precipitation index (SPI) is one of the most relevant indicators, providing a clear and robust characterization of precipitation deficiencies and it is a good proxy for assessing meteorological droughts. The SPI calculation is relatively simple and it is performed independently at each grid point of the domain. This method is robust and has the advantage of being flexible in time, for the accumulation period studied, and in space, for the resolutions used. It also provides an unbiased product, which is important for comparing observational data sets and model simulations. In the SPI calculation, a gamma distribution is first fitted to monthly cumulated precipitation data. Then this distribution is transformed into a standard normal distribution (McKee et al., 1993, 1995). The choice of the statistical distribution has been verified in Lavaysse et al. (2015) and it was shown that this assumption is valid over a large proportion of Europe. Nevertheless, over the driest regions and in summer, some grid points (mainly in Spain and southern Italy) the significant tests are not verified. Both the observed and modelled daily precipitation values are accumulated over a period of 30 days (i.e. we use the SPI-1, where 1 refers to the accumulation period of 1 month). The choice of analyzing relatively short meteorological droughts is based on two main constraints: (i) a technical one connected to the limitation of the extended ENS that provides forecasts up to 33 days in the version analyzed here, and (ii) the chaotic nature of the atmosphere that limits the predictability of precipitation and geopotential forecasts after several weeks (Vigaud et al., 2018). This relative short-term drought information is also relevant for users and decision makers, since it provides valuable information about the onset, continuation or end of longer droughts (Stagge et al., 2015; Svoboda et al., 2012).
Table 1Definition of WRs and WR combinations. WR combinations are defined as either additions or subtractions of monthly WR frequencies. Asterisks indicate regimes that exist only in winter and spring when four WRs are detected.
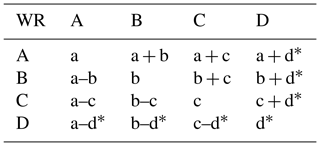
Based on this approach, both observed and forecasted SPI-1 values are calculated for the period 1992–2013. Here, a meteorological drought is defined as having SPI-1 values less than −1. According to the normal distribution of the SPI, this threshold corresponds to about 17.5 % of the driest events. Based on Lavaysse et al. (2015), the most reliable method for producing a dichotomous forecast of drought from probabilistic forecasts of precipitation, and more specifically from the extended ENS of the ECMWF, is to predict a drought as soon as more than 40 % of the ENS members are associated with a drought forecast (i.e. SPI-1 < −1).
2.4 Validation tools
To assess the forecasts of drought events, traditional scores for dichotomous products are applied. These scores make use of the contingency table (Table 2), which shows the types of agreement of observed and forecasted variables.
The percentage of observed events that had been correctly forecasted are provided by the probability of detection score (POD), whereas the percentage of events that had been forecasted but did not occur are indicated by the false alarm rate (FAR). Finally, to take into account the hits, misses and false alarms and to neglect the correct negative forecasts that will boost the scores for rare events, the Gilbert Skill Score (GSS, Jolliffe and Stephenson, 2003) is used. For rare events, such as droughts, it is more relevant to use this score than the Pierce's skill score, for instance. The GSS indicates how well the forecasted droughts correspond to the observed ones. This skill score is compared to the score obtained by the climatology. It is calculated as follows:
where hits. Based on these equations, a perfect forecast achieves a score equal to 1, while a score equal to 0 is assigned to the climatology (i.e. no forecast skill). All these scores are calculated independently for each season.
To forecast droughts using the MOAWRs approach, three steps are needed (see also Fig. S1): (1) the WR classification, to determine the main patterns of 500 hPa geopotential anomalies; (2) the daily WR attribution, to determine which is the closest WR classified previously for a forecasted (or reanalyzed) geopotential anomaly for each day and member, and the calculation of the MOAWRs; (3) the predictor assignation, to determine which WR, or combination of WRs, is the best predictor of droughts for each grid point. These different steps are now detailed.
Table 3Definition of the five sets of forecasts compared in that study. The differences are based on whether a predictor is used or not, the use of predictors derived from reanalyze or forecasted and for the assignation procedure, the use of observed or forecasted SPI.
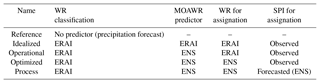
3.1 WR classification and MOAWRs calculation
The WR classification (step 1 in Fig. S1) is detailed previously. The patterns of the geopotential height anomalies for the four seasons are displayed in Fig. S2. The best-known WRs occur in winter, namely the NAO− (Fig. S2a), the NAO+ (Fig. S2e), the Atlantic Ridge (Fig. S2i) and the blocking regime (Fig. S2m). Once the WR classification is done, the closest WR to the daily geopotential of each ENS member is attributed to both ERAI and ENS. From daily attribution, the climatology and the anomalies of occurrence of each WR can be done (illustrated by the step 2 in Fig. S1) using ERAI or ENS depending on the configuration of the forecast experiment (see list in Table 3). These two steps allow daily attribution of WRs and then the MOAWRs for both the ERAI and ENS data sets.
3.2 Assignation of predictors
The objective of this step is to identify the best SPI-1-predictor within the three or four WRs identified for each season and their 6 to 12 possible combinations (step 3 in Fig. S1). This is done by using the temporal correlation between the MOAWRs (deriving from ERAI or ENS depending the forecast experiment) and the SPI-1 for each grid point and each MOAWR. This allows us to highlight the large-scale impacts of the WRs on precipitation (see Fig. S3 and comments). An automatic attribution is then applied based on the maximum of the absolute values of the correlations. The sign of the correlation is recorded to keep track of the type of linkage. An example of linkage over Scandinavia is provided in the Supplement (see Supplement and Fig. S4).
3.3 Forecast configurations
The potential benefits and limitations of using these predictors are assessed thanks to five different drought-forecasting approaches. These approaches are differentiated by the methodologies employed for the three steps listed previously and are illustrated in Fig. S1 and summarized in Table 3.
The first method of drought forecasting, called a “reference”, is based on forecasted precipitation (Lavaysse et al., 2015). The skill scores of this forecasting approach are used here as a benchmark.
The second method of forecasting, called “idealized” (red arrows in Fig. S1), uses exclusively the MOAWRs derived from ERAI and does not take into account the uncertainties related to the forecasts of WRs. In this method, the assignation of predictors is based on the best correlation between MOAWRs from observed ERAI and SPI-1. It is interesting to note that, following this approach, the large majority of SPI-1 in Europe is associated with a combination of WRs (Fig. 1). The highest absolute values of these correlations show significant spatial differences (Fig. 2). Throughout the year, there are generally higher linkages in northern Europe than in southern Europe. There is also a strong seasonal difference. In winter, the mean correlation is about 0.55, whereas it is about 0.28 in summer. The origin of precipitation, which is more synoptically driven in winter and more local in summer, can explain these results.
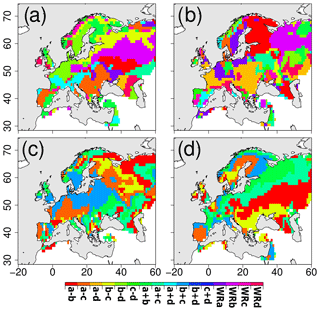
Figure 1Automatic attribution of the best predictors in winter (a), spring (b), summer (c) and autumn (d) based on the occurrence anomalies of WRs of ERAI and the observed precipitation (used for the operational and idealized forecasts). The names of the predictors are indicated on the colour scale.
The third forecasting method, called “operational”, computes MOAWRs derived from the ENS forecasts, but the WR classification (definition of the different regimes) and the WR assignation (best WR over each grid point) are still derived from ERAI and observed SPI-1 (see Table 3 and green arrows in Fig. S1). The advantage of this method is a real assessment of the model ability to forecast both the WRs and the relationship between the SPI and the WRs. As this assignation is constant (i.e. derived from ERAI and observations), it is also easier to set up operationally and there is no problem when the version of the operational ENS model changes. The disadvantage is the non-optimization of the forecast; i.e. there is no correction for bias in the forecasted WRs.
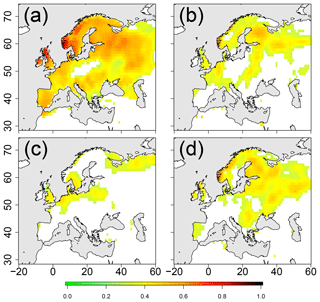
Figure 2Absolute values of temporal correlation between SPI-1 and MOAWR derived from ERAI (used for the operational and idealized forecasts) attributed from the 16 combinations in winter (a), spring (b), summer (c) and autumn (d). Only values with a confidence level larger than 90 % are plotted.
The fourth forecasting method, called “optimized” (see Table 3 and blue arrows in Fig. S1), is relatively close to the previous one but uses a different assignation procedure. It is defined as the best correlation between the observed SPI-1 and the forecasted MOAWRs by the ENS as predictor (instead of those derived from ERAI). This method derives the best relationships between forecasted MOAWRs and observed precipitation and by definition will obtain the best scores, even if the WRs are not correctly forecasted. This methodology tends then to optimize the forecasts by correcting some bias in the forecasted MOAWRs. The correlation values for observed SPI with MOAWRs derived from ENS for winter are provided in the Supplement (Fig. S5) and depict patterns very similar to those obtained with ERAI (Fig. S3), illustrating the good representation of the linkages in ENS.
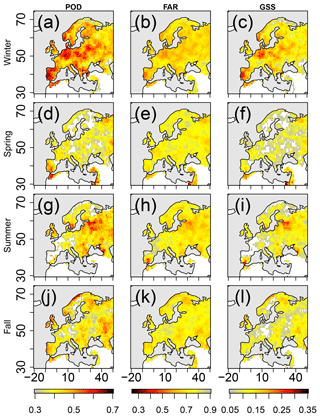
Figure 3POD (a, d, g, j), FAR (b, e, h, k; with reverse colours) and GSS (c, f, i, l) scores of droughts prediction calculated using the reference forecast. The scores are calculated for (from top to bottom) winter (DJF, a–c), spring (MAM, d–f), summer (JJA, g–i) and autumn (SON, j–l).
Finally the fifth forecasting method, called “process” (purple arrows in Fig. S1 and Table 3), can be used to investigate the skill of the model in representing observed processes. Except for the WR classification procedures, which are still derived from ERAI, this method uses ENS forecasts. The forecasted MOAWRs are then linked to the forecasted SPI-1 (instead of observed SPI for the other configurations) in the assignation procedure. This configuration allows an analysis of the modelled linkage between MOAWRs and precipitation, that will be compared to the observed ones provided by the idealized configuration.
4.1 Skill scores
The skill scores of the forecasted precipitation, called “reference”, are used as a benchmark. It is derived from Lavaysse et al. (2015), where a drought is forecasted when at least 40 % of members forecast SPI < −1. The best achieved performance (for winter in central Europe) shows how slightly more than 40 % of the observed drought events are correctly predicted with a 30-day lead time (Fig. 3a, d, g, j) with about 60 % of false alarms (Fig. 3b, e, h, k). For both POD and FAR, the spatial variability is small (standard deviation lower than 0.2), especially during spring and autumn. In winter, high scores can be noticed in Germany, Poland, Spain and Norway, whereas in summer, drought seems to be more predictable in eastern Europe. When the POD and the FAR are combined in the integrated GSS (Fig. 3c, f, i, l), higher seasonal and spatial differences appear. Overall, the score reaches up to 0.3 in winter, especially in northern Germany [50∘ N, 10∘ E], while the worst value is reached in spring and summer, especially in western Europe (France, Belgium). Due to the impacts of the local forcing on precipitation, the drought forecasts based on large-scale predictors are better in continental than in coastal regions (more details in Lavaysse et al., 2015).
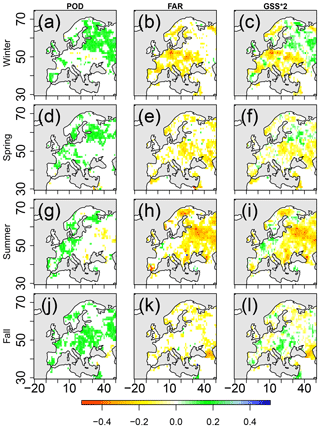
Figure 4Anomalies of POD (a, d, g, j), FAR (b, e, h, k) and GSS*2 (c, f, i, l) scores of drought prediction using the operational forecast with regard to the reference forecast. The scores are calculated for (from top to bottom) winter (a–c), spring (d–f), summer (g–i) and autumn (j–l). Improvement scores using the predictors are indicated in green (inverse scale for FAR). Only differences with confidence intervals larger than 90 % are plotted. GSS is multiplied by 2 to use the same scale as the other metrics.
The forecasts using predictors, using the operational forecast, can now be assessed with respect to the reference. In order to detect the same number of drought events when using the predictors and the precipitation, the threshold of the MOAWRs is chosen to be equal to 0.176 (0.824 for negative correlations). The POD, FAR and GSS anomalies with regard to the reference forecast (i.e. Fig. 3) for the four seasons are shown in Fig. 4. This is done using 20 years with a leave-one-out technique, which is a cross-validation method for small sample sizes enabling us to validate results by simply partitioning the series into a training and a test part. The operational forecast is more spatially variable. As for winter in the northern part of Europe, this forecast is significantly better in terms of both POD and GSS, whereas in central Europe the reference forecast is more reliable. Despite the fact that the patterns are less homogeneous for the other seasons, some positive impacts of this operational forecast appear, for example, in northern Russia in spring, western Europe in summer, central Europe during autumn. The same results have been plotted in the Supplement (Fig. S6) for the optimized forecasts and do not show significant differences with Fig. 4.
These results are consistent with the intensity of the linkage measured during the assignation procedure between the SPI and the WRs (Fig. 2) and highlight the regions where the large-scale atmospheric patterns associated with the WRs could better explain strong precipitation deficits when compared to local drivers (e.g. orography, soil moisture and coastline).
4.2 Intensity and initial conditions
To better understand the potential performance of the approach, sensitivity tests are conducted. In the previous section, the SPI-1 intensity threshold, which defines a drought, was fixed to −1. The previously used skill scores are derived here for SPI lower than −1.5 and −2 (∼7 % and ∼2.5 % of the most extreme cases). A second sensitivity test is done on the initial conditions, influencing all the results but also bringing useful information on drought onset and persistence. Most of the studies on drought focus on 3-month (or longer) cumulated precipitation that could have more severe impacts on, for example, agricultural and water resources. Due to the unpredictable nature of the weather and the limitation of the lead time of the ENS model, the assessment of drought forecasting is limited to a 1-month lead time in our study. Nevertheless, the information of the two previous months (observed SPI-2 with a threshold defined as −1) is taken into account to measure the impacts of these initial conditions and the ability to forecast drought persistence and onset.
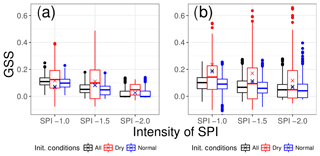
Figure 5Box plot of the GSS scores in winter using the reference forecast (a) and the operational forecast (b). The scores are calculated over the entire domain and the boxes display the spatial variability. The scores depend on the SPI intensities (−1, −1.5 and −2, x axis) and the initial conditions defined by the previous observed SPI-2 conditions (see text for more details). Crosses indicate the scores but are calculated by merging all the grid cells.
In Fig. 5 the GSS scores in winter for the whole domain shown in the previous figures are synthesized by using box plots. The results shown in Figs. 3a and 4a are represented by the black box plots for SPI < −1 in Fig. 5a and b. Overall, the predictability decreases with the drought intensity. In winter, dry initial conditions generate a favourable environment to better forecast droughts. In other words, the persistence of drought is better predicted than the onset. Finally, the last main result concerns the improvement of the operational forecasts. For the SPI lower than −1, all GSS values shown in Fig. 5 are quite close. But, as also highlighted by Fig. 4, there is a larger spatial variability with the MOAWRs approach. For more intense droughts, there is a global and significant improvement by using the operational forecast. Indeed for drought intensities with SPI lower than −2, the median of the GSS scores goes up from close to 0 (using the precipitation-based method) to 0.05 (using the MOAWRs).
The same sensitivity tests are conducted for the other seasons (Figs. S7–S9), and the decrease in predictability with increasing drought intensity is found for all of them. Nevertheless, the conclusions on the role of the initial conditions depend on the season. For instance in summer, drought onsets are slightly better predicted than drought persistence. The reason could be the higher temporal variability of the monthly precipitation deficits in summer than in winter due to the larger impact of local forcings. Finally, in all the seasons, the use of atmospheric predictors (i.e. operational forecast) leads to a better performance when looking at the most extreme events (SPI < −2).
To better discuss and understand the results and their uncertainties, additional tests are reported here. The main objective is to quantify the contribution of the uncertainties in WR predictions and the linkage between the SPI and the WRs.
5.1 Validation of the WR forecasts
The first question to address is about the quality of the forecasts of MOAWRs. The purpose here is not to provide a complete evaluation of the WRs forecasts that have been already studied (Ferranti et al., 2015; Matsueda and Palmer, 2015), but to focus on errors that could impact the drought forecasts.
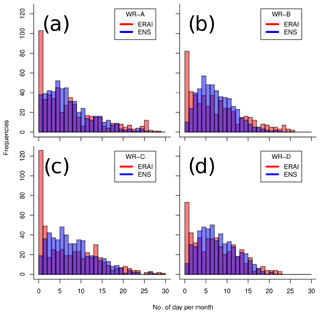
Figure 6Example of the frequency distribution of WR occurrences (in days per 30-day windows) in winter for WR-A (a), WR-B (b), WR-C (c) and WR-D (d) using ERAI and ENS (red and blue bars, purple when the two overlap).
To validate the forecast of the WRs, first the comparison of the frequency of occurrence of each daily WR is performed (Fig. 6). To do so, the climatology of the total occurrence of each WR among all the members and the entire lead time (5 members × 30-day LT) is calculated to extract the monthly anomalies. The forecasted anomalies are divided by the number of ENS members to create comparable results with the data provided by ERAI. The WR-distributions as given by the forecasts are characterized by a higher degree of similarity than the ones given by ERAI, with a peak of occurrence at around 5–8 days in winter (blue bars, Fig. 6). The same holds for the other seasons (not shown). The lower spread of the forecasted WR occurrences, associated with reduced tails (i.e. reduced occurrences for durations exceeding 20 days), could be explained by the underestimation of the long-term persistence of regimes. A further comparison of the MOAWRs from ERAI and ENS (scatter plots in Fig. 7) suggests that (i) the distribution of forecasted drought occurrences could be explained by the overestimation of low occurrences using ENS than the reanalysis (i.e. larger number of forecasted events compared to those derived from ERAI with durations shorter than 5 days), and (ii) the underestimation of longer duration events (i.e. lower events with durations longer than 15 days using ENS than ERAI, red dotted lines in Fig. 7). Despite this behaviour, the correlations appear significant with a maximum of 0.65 for the WRa (significance with 90 % of confidence at 0.58). These significant scores are obtained in winter, while for the other seasons the correlations are lower (see Table 4). In summer, they are not significant for two-thirds of the WRs.
5.2 Strength of MOAWR-precipitation linkage
According to the previous subsection, the WR forecast could be improved. Thus, it is important to assess the limitation of the method using predictors and so assess the strength of the MOAWR and precipitation linkage. To this aim, the idealized forecasts of MOAWRs, i.e. geopotential anomalies provided by ERAI without uncertainties, are compared to the forecast of precipitation discussed and shown in Fig. 3.
The POD scores are strongly improved between seasons and regions (Fig. 8a, d, g and j). These results are strongly connected to the correlation values obtained and shown in Fig. 2 with the same north–south and seasonal variabilities being observed. However, almost all of the northern part of Europe shows a better POD with the idealized than reference forecasts. Up to 70 % of observed drought events are correctly detected during winter. This percentage falls to about 17.5 % in summer (i.e. the climatological value) in the southern part of the domain. The results in terms of FAR are more variable depending on both the season and the region. On average, there is a small decrease in the FAR. However, the GSS shows a clear and significant improvement in the drought forecast when using the WR predictors. Compared to the scores using operational forecasts in Fig. 4, the bigger difference is more in terms of magnitude than spatial distribution. For instance, in winter a large improvement is observed in northern Europe (up to 0.2 for idealized against 0.1 for operational forecast over Scandinavia), whereas a low score is obtained in central Europe. Based on this sensitive analysis, the linkage between the SPI-1 < −1 and the MOAWRs is strong enough to provide significant improvements of the prediction scores in most of the regions. Nevertheless, this analysis also highlights the limitations of the methods used in this study when and where the influence of the WR on drought is lower (e.g. Germany and Poland in winter, eastern Europe in summer and southern Europe in autumn).
5.3 Modelled linkage
Some additional tests are also conducted on the predictor assignation procedures (definition of the best predictor for SPI-1 < −1 at each grid point) to see the impacts of using either ERAI or ENS (the latter could potentially correct bias of the ENS). This is done using the optimized forecast. Due to the errors associated with the WR forecasts, the procedures using WRs from ERAI or ENS provide different results (Fig. 9a compared to Fig. 1a). The assignation patterns done by using ERAI (Fig. 1a) for the operational and idealized forecasts have less homogeneous large-scale structures (i.e. more spatial variability) than the optimized forecast (Fig. 9a), showing more complex linkages using ERAI than ENS. Nevertheless over continental regions, there are similarities (impact of WRs b, b–a, a, c–d) illustrating the relatively good representation of the impacts of specific WR on precipitation by ENS. The correlations between the forecasted WRs and the observed precipitation are then plotted (Fig. 9b). The correlation values, which can be compared to the correlation shown in Fig. 2a, are low as a result of the relatively low predictability of the WRs previously discussed. The values are also sensitive to the strength of the linkage between WRs and precipitation (i.e. highest scores in southern Norway and the northern part of the UK, the lowest scores in central Europe.).
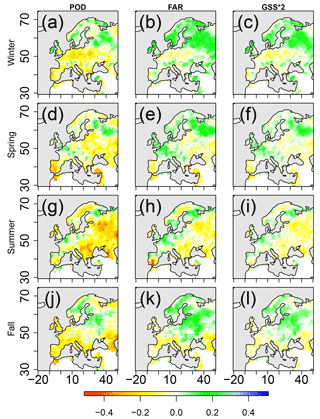
Figure 8Anomalies of POD (a, d, g, j), FAR (b, e, h, k) and GSS*2 (c, f, i, l) of the drought prediction based on the idealized forecasts with regard to the reference forecast, in winter (a–c), spring (d–f), summer (g–i) and autumn (j–l). Improvement scores using the predictors are indicated in green (inverse scale for FAR). Only differences with confidence intervals larger than 90 % are plotted. GSS is multiplied by 2 to use the same scale as the other metrics.
The last analysis is focused on the modelled linkage between the SPI and the WRs, both provided by the ENS (i.e. using the process forecast, Fig. 9c and d). The great similarities in the maps of assigned WRs between process and idealized forecasts are remarkable (correlation values greater than 0.65, Figs. 1a and 2a compared to Fig. 9c and d). This is especially true over the UK, Ireland, Scandinavia, Spain and north-western Russia. Despite some differences observed in southern France and Italy, where the process overestimates the large-scale forcing on precipitation (i.e. with stronger correlation with WRs than observed), the patterns obtained are very similar when comparing the correlation values between SPI-1 (observed or forecasted) and MOAWRs (from ERAI or ENS) in Figs. S3, S5 and S10. This highlights the overall good representation by ENS of the processes linking large-scale circulation and local precipitation deficits. So the ENS model succeeds in capturing the impacts of the WR occurrences on the precipitation anomalies as shown with observations and ERAI over a large part of Europe. These results could suggest limitations in using such predictors, as the lack of skill score could result from a failure in forecasting the large-scale atmospheric circulation rather than from a misrepresentation of the physical processes from the large-scale forcing to local weather.
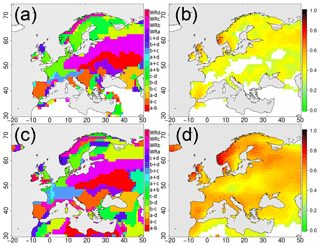
Figure 9Assigned winter WR (a) and associated absolute correlation values (b) for the optimized forecast (i.e. predictors defined using MOAWRs from ENS and observed SPI-1). Panels (c) and (d) are the same as (a) and (b) for the process forecast (i.e. predictors defined using MOAWRs from ENS and forecasted SPI-1).
In this study, a drought-forecasting method based on large-scale atmospheric predictors is proposed in order to improve the early warning of atmospheric drought events. The method is based on the monthly occurrence anomalies of weather regimes (MOAWRs) within a 30-day lead time. The methodology used to select the predictors is based on a three-step procedure. First, WRs (described by daily 500 hPa geopotential anomalies) are identified by using a genetic K-means algorithm for each season separately and for both ERAI and extended ENS forecasts. The climatological occurrences are calculated for each WR. The identified three/four WRs (depending on the season) are combined (added or subtracted) with each other to enhance the potential signal of their impacts. Second, the MOAWRs is used as a predictor of meteorological droughts at each grid point. The predictor assignation procedure is based on the correlation between the MOAWRs and the SPI-1. To select the best predictor, the MOAWR associated with the strongest absolute value of correlation is selected. The last step involves the forecasting of the SPI-1 lower than −1. Two approaches are derived and compared. The first one is based on the index developed by Lavaysse et al. (2015) for drought events and derived from the forecasted precipitation provided by the ENS and called “reference”. This represents a benchmark for the early warning of drought forecasting. At most, around 40 % of drought events are detected 1 month in advance with 65 % of false alarms. The second forecasting approach, called “operational”, is based on MOAWRs. In the north-eastern parts of the European continent, an improvement of the Gilbert Skill Score (GSS) is observed using the operational forecast with regard to the reference forecast. Nevertheless, this is balanced by other regions where the forecast skills is clearly lower (central Europe in winter, eastern Europe in summer) than the reference. The origin of this spatial and temporal variability in the skill scores is linked to the dynamic of the atmosphere associated with the precipitation. In winter, precipitation is much more closely related to large-scale atmospheric forcing, mainly captured by the MOAWRs. On the contrary, in summer, precipitation is more affected by local forcings that could influence, for instance, the trajectory and the occurrence of convective systems. In this study, this behaviour is captured by the better correlation between MOAWRs and precipitation in winter than in summer. The spatially variable skill scores are mainly controlled by the intensity of the linkage between the MOAWRs and SPI-1. Due to the distance between the geopotential anomalies to some target regions, or because of some local effects that could be predominant to the large-scale forcing, the impacts of these MOAWRs on precipitation could be low, as observed in winter over central Europe. According to these scores, the most reliable forecast could result from choosing the best method for each grid point independently. The influence of the initial conditions and the intensity of the drought highlight (i) the losses of predictability with increasing drought intensity and (ii) the better scores in predicting persistency rather than the onset of drought, especially in winter. Also, the benefits of using the WRs to predict droughts appear to be more important when the most intense droughts (i.e. SPI < −2) are forecasted.
This study shows the importance of improving the prediction of the WR occurrences. The methodology applied here could be compared to more complex methodologies using clustering of the members to define the most probable scenario or by taking into account the transition between WRs. Future work should also take into account the uncertainties in WR prediction, as also suggested by Magnusson (2017); Weisheimer et al. (2017). Recent studies (Matsueda and Palmer, 2014, 2015; Vigaud et al., 2018) have shown that WR prediction is still a big challenge for lead times greater than 15 days. Some improvements could be also done by using a multi-model ensemble such as the one recently developed in the framework of the Sub-seasonal to Seasonal (S2S) Project (Vitart et al., 2016). Finally, the physical drivers should be analyzed in detail to better understand why the predictors are more useful when predicting the most extreme events.
Most of the weather services provide new forecasts up to several months. For users, it appears essential to scientifically and statistically evaluate the added values of these forecasts for specific extreme events such as meteorological droughts. This is the main objective of this study. Nevertheless, evaluating the practical usefulness of this operational forecast is difficult without taking into account the costs for each case of the contingency table (hits, misses and false alarms) that strongly vary depending on their applications (civil protection, water management services, farmers' decision supporting systems, etc.). The statement provided in this study is based on statistical scores independent of these costs. According to the GSS, there is a significant improvement of using forecasts in relation to the climatology. Moreover, the forecasts using predictors generate, in some regions and some seasons, significant improvements of these forecasts by using the same score. To evaluate these improvements for specific users, the costs should be taken into account and this is a major perspective of this study.
The correspondence author could provide part of these datasets, but can not provide the forecasts that are provided by ECMWF and are protected by a specific licence.
The supplement related to this article is available online at: https://doi.org/10.5194/nhess-18-3297-2018-supplement.
The authors declare that they have no conflict of interest.
CL analysed the precipitation forecasts and developed the new prediction system. AT provided the WR detection and forecasts. CL, AT and JV interpreted the results. MC and FP provided comments and suggestions to improve the model and the article.
This study has been carried out with the support of ECMWF, which provided the
forecasted precipitation and 500 hPa geopotential heights. The authors would
like to thank the two anonymous reviewers for their fruitful comments.
Edited by: Joaquim G. Pinto
Reviewed by: two anonymous referees
Boé, J.: Modulation of soil moisture–precipitation interactions over France by large scale circulation, Clim. Dynam., 40, 875–892, 2013. a
Cassou, C., Terray, L., and Phillips, A. S.: Tropical Atlantic influence on European heat waves, J. Climate, 18, 2805–2811, 2005. a
Cattiaux, J., Vautard, R., Cassou, C., Yiou, P., Masson-Delmotte, V., and Codron, F.: Winter 2010 in Europe: a cold extreme in a warming climate, Geophys. Res. Lett., 37, L20704, https://doi.org/10.1029/2010GL044613, 2010. a
Dee, D., Uppala, S., Simmons, A., Berrisford, P., Poli, P., Kobayashi, S., Andrae, U., Balmaseda, M., Balsamo, G., Bauer, P., Bechtold, P., Beljaars, A. C., van de Berg, L., Bidlot, J., Bormann, N., Delsol, C., Dragani, R., Fuentes, M., Geer, A. J., Haimberger, L., Healy, S. B., Hersbach, H., Hólm, E. V., Isaksen, L., Kållberg, P., Köhler, M. , Matricardi, M., McNally, A. P., Monge-Sanz, B. M., Morcrette, J., Park, B., Peubey, C., de Rosnay, P., Tavolato, C., Thépaut, J., and Vitart, F.: The ERA-Interim reanalysis: Configuration and performance of the data assimilation system, Q. J. Roy. Meteorol. Soc., 137, 553–597, 2011. a
Ferranti, L., Corti, S., and Janousek, M.: Flow-dependent verification of the ECMWF ensemble over the Euro-Atlantic sector, Q. J. Roy. Meteorol. Soc., 141, 916–924, 2015. a, b, c
Ferranti, L., Magnusson, L., Vitart, F., and Richardson, D. S.: How far in advance can we predict changes in large-scale flow leading to severe cold conditions over Europe?, Q. J. Roy. Meteorol. Soc., 144, 1788–1802, https://doi.org/10.1002/qj.3341, 2018. a
Guérémy, J.-F., Laanaia, N., and Céron, J.-P.: Seasonal forecast of French Mediterranean heavy precipitating events linked to weather regimes, Nat. Hazards Earth Syst. Sci., 12, 2389–2398, https://doi.org/10.5194/nhess-12-2389-2012, 2012. a
Haiden, T., Janousek, M., Bidlot, J., Ferranti, L., Prates, F., Vitart, F., Bauer, P., and Richardson, D.: Evaluation of ECMWF forecasts, including 2016–2017 upgrades, ECMWF – Technical Memorandum, ECMWF, Reading, UK, 2017. a
Häkkinen, S., Rhines, P. B., and Worthen, D. L.: Atmospheric blocking and Atlantic multidecadal ocean variability, Science, 334, 655–659, 2011. a
Haylock, M., Hofstra, N., Klein Tank, A., Klok, E., Jones, P., and New, M.: A European daily high-resolution gridded data set of surface temperature and precipitation for 1950–2006, J. Geophys. Res.-Atmos., 113, D20119, https://doi.org/10.1029/2008JD010201, 2008. a
Hurrell, J. W., Kushnir, Y., Ottersen, G., and Visbeck, M.: An Overview of the North Atlantic Oscillation, in: The North Atlantic Oscillation: Climatic Significance and Environmental Impact, edited by: Hurrell, J. W., Kushnir, Y., Ottersen, G., and Visbeck, M., available at: https://agupubs.onlinelibrary.wiley.com/doi/10.1029/134GM01 (last access: December 2018), 2013. a
Jolliffe, I. and Stephenson, D.: Forecast Verification: A Practitioner's Guide in Atmospheric Science, John Wiley and Sons Ltd, Chichester, UK, 2003. a
Kingston, D. G., Stagge, J. H., Tallaksen, L. M., and Hannah, D. M.: European-scale drought: understanding connections between atmospheric circulation and meteorological drought indices, J. Climate, 28, 505–516, 2015. a
Lavaysse, C., Vogt, J., and Pappenberger, F.: Early warning of drought in Europe using the monthly ensemble system from ECMWF, Hydrol. Earth Syst. Sci., 19, 3273–3286, https://doi.org/10.5194/hess-19-3273-2015, 2015. a, b, c, d, e, f, g
Lavers, D. A., Pappenberger, F., Richardson, D. S., and Zsoter, E.: ECMWF Extreme Forecast Index for water vapor transport: A forecast tool for atmospheric rivers and extreme precipitation, Geophys. Res. Lett., 43, 11852–11858, https://doi.org/10.1002/2016GL071320, 2016a. a
Lavers, D. A., Waliser, D. E., Ralph, F. M., and Dettinger, M. D.: Predictability of horizontal water vapor transport relative to precipitation: Enhancing situational awareness for forecasting western US extreme precipitation and flooding, Geophys. Res. Lett., 43, 2275–2282, 2016b. a
Magnusson, L.: Diagnostic methods for understanding the origin of forecast errors, Q. J. Roy. Meteorol. Soc., 143, 2129–2142, 2017. a
Matsueda, M. and Palmer, T.: Predictability of wintertime Euro-Atlantic weather regimes in medium-range forecasts, in: EGU General Assembly Conference Abstracts, 16, 12945, 2014. a
Matsueda, M. and Palmer, T.: Predictability of summertime Euro-Atlantic weather regimes in medium-range forecasts, in: EGU General Assembly Conference Abstracts, 17, 11809, 2015. a, b
McKee, T. B., Doesken, N. J., and Kleist, J.: The relationship of drought frequency and duration to time scales, in: Proceedings of the 8th Conference on Applied Climatology, vol. 17, American Meteorological Society Boston, MA, USA, 179–183, 1993. a
McKee, T. B., Doesken, N. J., and Kleist, J.: Drought monitoring with multiple time scales, in: Proceedings of the 9th Conference on Applied Climatology, American Meteorological Society Dallas, Boston, MA, 233–236, 1995. a
Michelangeli, P.-A., Vautard, R., and Legras, B.: Weather regimes: Recurrence and quasi stationarity, J. Atmos. Sci., 52, 1237–1256, 1995. a, b
Molteni, F., Buizza, R., Palmer, T. N., and Petroliagis, T.: The ECMWF ensemble prediction system: Methodology and validation, Q. J. Roy. Meteorol. Soc., 122, 73–119, 1996. a
Peings, Y. and Magnusdottir, G.: Forcing of the wintertime atmospheric circulation by the multidecadal fluctuations of the North Atlantic ocean, Environ. Res. Lett., 9, 034018, https://doi.org/10.1088/1748-9326/9/3/034018, 2014. a
Pfahl, S.: Characterising the relationship between weather extremes in Europe and synoptic circulation features, Nat. Hazards Earth Syst. Sci., 14, 1461–1475, https://doi.org/10.5194/nhess-14-1461-2014, 2014. a
Plaut, G. and Simonnet, E.: Large-scale circulation classification, weather regimes, and local climate over France, the Alps and western Europe, Clim. Res., 17, 303–324, 2001. a
Robertson, A. W. and Ghil, M.: Large-scale weather regimes and local climate over the western United States, J. Climate, 12, 1796–1813, 1999. a
Santos, J., Corte-Real, J., and Leite, S.: Weather regimes and their connection to the winter rainfall in Portugal, Int. J. Climatol., 25, 33–50, 2005. a
Schaller, N., Sillmann, J., Anstey, J., Fischer, E., Grams, C., and Russo, S.: Influence of blocking on Northern European and Western Russian heatwaves in large climate model ensembles, Environ. Res. Lett., 13, 054015, https://doi.org/10.1088/1748-9326/aaba55, 2018. a
Stagge, J. H., Kohn, I., Tallaksen, L. M., and Stahl, K.: Modeling drought impact occurrence based on meteorological drought indices in Europe, J. Hydrol., 530, 37–50, 2015. a
Stephenson, D., Hannachi, A., and O'Neill, A.: On the existence of multiple climate regimes, Q. J. Roy. Meteorol. Soc., 130, 583–605, 2004. a
Svoboda, M., Hayes, M., and Hood, D.: Standardized Precipitation Index User Guide, World Meteorological Organization, Report WMO – No. 1090, WMO, Geneva, 2012. a, b
Toreti, A., Xoplaki, E., Maraun, D., Kuglitsch, F. G., Wanner, H., and Luterbacher, J.: Characterisation of extreme winter precipitation in Mediterranean coastal sites and associated anomalous atmospheric circulation patterns, Nat. Hazards Earth Syst. Sci., 10, 1037–1050, https://doi.org/10.5194/nhess-10-1037-2010, 2010. a, b, c
Vigaud, N., Robertson, A. W., and Tippett, M. K.: Multimodel ensembling of subseasonal precipitation forecasts over North America, Mon. Weather Rev., 145, 3913–3928, 2017. a
Vigaud, N., Robertson, A. W., and Tippett, M. K.: Predictability of recurrent weather regimes over North America during winter from submonthly reforecasts, Mon. Weather Rev., 146, 2559–2577, 2018. a, b
Vitart, F.: Evolution of ECMWF sub-seasonal forecast skill scores, Q. J. Roy. Meteorol. Soc., 140, 1889–1899, 2014. a
Vitart, F., Ardilouze, C., Bonet, A., Brookshaw, A., Chen, M., Codorean, C., Déqué, M., Ferranti, L., Fucile, E., Fuentes, M., Hendon, H., Hodgson, J., Kang, H., Kumar, A., Lin, H., Liu, G., Liu, X., Malguzzi, P., Mallas, I., Manoussakis, M., Mastrangelo, D., MacLachlan, C., McLean, P., Minami, A., Mladek, R., Nakazawa, T., Najm, S., Nie, Y., Rixen, M., Robertson, A. W., Ruti, P., Sun, C., Takaya, Y., Tolstykh, M., Venuti, F., Waliser, D., Woolnough, S., Wu, T., Won, D., Xiao, H., Zaripov, R., and Zhang, L.: The sub-seasonal to seasonal prediction (S2S) project database, B. Am. Meteorol. Soc., 98, 163–173, https://doi.org/10.1175/BAMS-D-16-0017.1, 2016. a
Wanner, H., Brönnimann, S., Casty, C., Gyalistras, D., Luterbacher, J., Schmutz, C., Stephenson, D. B., and Xoplaki, E.: North Atlantic Oscillation – concepts and studies, Surv. Geophys., 22, 321–381, 2001. a
Weisheimer, A., Schaller, N., O'Reilly, C., MacLeod, D. A., and Palmer, T.: Atmospheric seasonal forecasts of the twentieth century: multi-decadal variability in predictive skill of the winter North Atlantic Oscillation (NAO) and their potential value for extreme event attribution, Q. J. Roy. Meteorol. Soc., 143, 917–926, 2017. a
Yiou, P. and Cattiaux, J.: Contribution of atmospheric circulation to wet north European summer precipitation of 2012, B. Am. Meteorol. Soc., 94, S39–S41, 2013. a
Yiou, P. and Nogaj, M.: Extreme climatic events and weather regimes over the North Atlantic: When and where?, Geophys. Res. Lett., 31, L07202, https://doi.org/10.1029/2003GL019119, 2004. a, b
Zampieri, M., Toreti, A., Schindler, A., Scoccimarro, E., and Gualdi, S.: Atlantic multi-decadal oscillation influence on weather regimes over Europe and the Mediterranean in spring and summer, Global Planet. Change, 151, 92–100, 2017. a