the Creative Commons Attribution 4.0 License.
the Creative Commons Attribution 4.0 License.
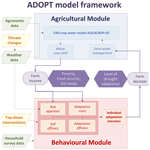
Education, financial aid, and awareness can reduce smallholder farmers' vulnerability to drought under climate change
Anne F. van Loon
Ted I. E. Veldkamp
Jeroen C. J. H. Aerts
Analyses of future agricultural drought impacts require a multidisciplinary approach in which both human and environmental dynamics are studied. In this study, we used the socio-hydrologic, agent-based drought risk adaptation model ADOPT. This model simulates the decisions of smallholder farmers regarding on-farm drought adaptation measures and the resulting dynamics in household vulnerability and drought impact over time. We applied ADOPT to assess the effect of four top-down disaster risk reduction interventions on smallholder farmers' drought risk in the Kenyan drylands: the robustness of additional extension services, lowered credit rates, ex ante rather than ex post cash transfers, and improved early warnings were evaluated under different climate change scenarios.
Model results suggest that extension services increase the adoption of newer low-cost drought adaptation measures while credit schemes are useful for measures with a high investment cost, and ex ante cash transfers allow the least wealthy households to adopt low-cost, well-known measures. Early warning systems are shown to be more effective in climate scenarios with less frequent droughts. Combining all four interventions displays a mutually reinforcing effect with a sharp increase in the adoption of on-farm drought adaptation measures, resulting in reduced food insecurity, decreased poverty levels, and drastically lower need for emergency aid, even under hotter and drier climate conditions. These nonlinear synergies indicate that a holistic perspective is needed to support smallholder resilience in the Kenyan drylands.
- Article
(3903 KB) - Full-text XML
- BibTeX
- EndNote
Droughts, defined as below-normal meteorological or hydrological conditions, are a pressing threat to the food production in the drylands of sub-Saharan Africa (Brown et al., 2011; Cervigni and Morris, 2016; UNDP et al., 2009). Over the last few decades, increasing temperatures and erratic or inadequate rainfall have already intensified drought disasters (Khisa and Gladys, 2017). Climate change, population growth, and socio-economic development will lead to additional pressures on water resources (Erenstein et al., 2011; Kitonyo et al., 2013). In Kenya, three-quarters of the population depend on smallholder rain-fed agricultural production and nearly half of the population is annually exposed to recurring drought disasters causing income insecurity, malnutrition and health issues (D'alessandro et al., 2015; Khisa, 2018; Mutunga et al., 2017; Rudari et al., 2019; UNDP, 2012). Reducing drought risk is imperative to enhance the resilience of the agriculture sector, to protect the livelihoods of the rural population, and to avoid food insecurity and famine in Kenya's drylands (Khisa and Gladys, 2017; Shikuku et al., 2017).
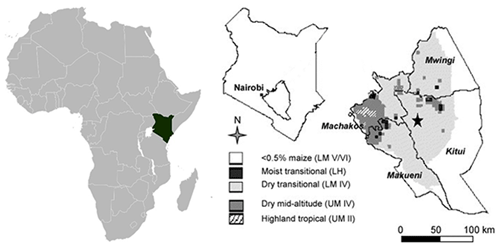
Figure 1Study area. Dry transitional maize agro-ecological zone (right) located in south-eastern Kenya (centre) in the Horn of Africa (left). The area where the survey data (Wens et al., 2021) are collected is indicated with a star on the right map. Map adjusted from Barron and Okwach (2005).
Drought risk models are important tools to inform policymakers about the potential effectiveness of adaptation policies and enable the design of customized drought adaptation strategies under different future climate scenarios (Carrao et al., 2016; De Stefano et al., 2015). Traditionally, such models express disaster risk as the product of hazard, exposure and vulnerability and are based on historical risk data. Recent disaster risk models have dealt with climate change adaptation in a two-stage framework: first describing a few scenarios regarding adaptation choices of representative households and then estimating the impacts of adaptation on (future) welfare while assuming climate change scenarios (Di Falco, 2014). However, most existing research does not account for more complex dynamics in adaptation and vulnerability (Conway et al., 2019), for the heterogeneity in human adaptive behaviour (Aerts et al., 2018), or for the feedback between risk dynamics and adaptive-behaviour dynamics (Di Baldassarre et al., 2017), though these are the aspects that determine, in large part, the actual risk (Eiser et al., 2012).
It appears that farmers often act boundedly rationally towards drought adaptation rather than economically rationally: their economic rationality is bounded in terms of cognitive capability, information available, perceptions, heuristics, and biases (Schrieks et al., 2021; Wens et al., 2021). To account for such individual adaptive behaviour in drought risk assessments, an agent-based modelling technique can be applied (Blair and Buytaert, 2016; Filatova et al., 2013; Kelly et al., 2013; Matthews et al., 2007; Smajgl et al., 2011; Smajgl and Barreteau, 2017). Agent-based models allow explicit simulation of the bottom-up individual human adaptation decisions and capture the macro-scale consequences that emerge from the interactions between individual agents and their environments. Combining risk models with an agent-based approach is thus a promising way to analyse drought risk, as well as the evolution of it through time, in a more realistic way (Wens et al., 2019).
Here we present how an agent-based drought risk adaptation model, ADOPT (design described in Wens et al., 2020), can increase our understanding of the effect of drought policies on community-scale drought risk for smallholder farmers in Kenya's drylands. The design of ADOPT as an agent-based drought risk adaptation model is described in Wens et al. (2020). Moreover, Wens et al. (2021) detail the empirical data on past adaptive behaviour (used to calibrate the model), as well as empirical data on adaptation intentions that can be used for comparison with the model outputs.
In this study, we apply the ADOPT model to test the variation in household drought risk under different drought management policies: (i) a reactive government only providing emergency aid; (ii) a proactive government, which provides sufficient drought early warnings and ex ante cash transfer in the face of droughts; and (iii) a prospective government that, in addition to early warnings and ex ante transfers, subsidizes adaptation credit schemes and provides regular drought adaptation extension services to farmers. In addition, ADOPT is used to evaluate the robustness of these policies under different climate change scenarios. We acknowledge that ADOPT should be subject to additional validation steps in order to more accurately and precisely predict future drought risk. Yet, in this study we elaborate the potential of this proof-of-concept model by showcasing the trends in drought risk under risk reduction interventions and climate change for a case study in semi-arid Kenya.
The ADOPT model has been applied to the context of smallholder maize production in the dryland communities in the areas Kitui, Makueni, and Machakos in south-eastern Kenya (Fig. 1). This semi-arid to sub-humid region is drought-prone, being hit by drought disasters in 1983–1984, 1991–1992, 1995–1996, 1998–2000, 2004–2005, 2008–2011, and 2014–2018 (data from EM-DAT and DesInventar). The majority of the population in this dry transitional farming zone is directly or indirectly employed through agriculture. However, technology adoption and the production level remain rather low, making the region very vulnerable to droughts and climate change (Khisa and Oteng, 2014; Mutunga et al., 2017).
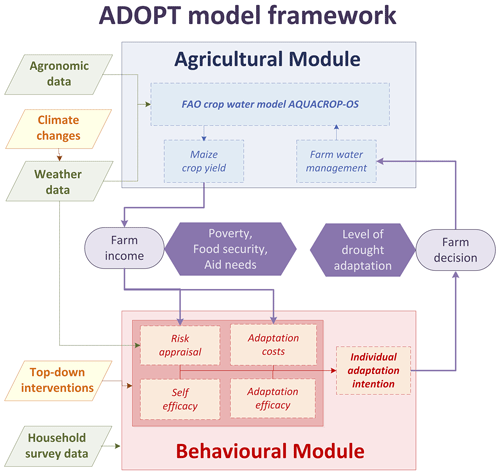
Figure 2ADOPT model overview, adjusted from Wens et al. (2020). Description of the model (overview, design concepts, and details) in Appendix A.
In Kenya, 75 % of the country's maize is produced by smallholder farms. Maize is grown in the two rainy seasons, with the aim to meet household food needs (subsistence farming) (Erenstein et al., 2011; Ifejika Speranza et al., 2008). While during the long rainy season (March–April–May) multiple crops are planted, the short rainy season (October–November–December) is considered the main growing season for maize in the region (Rao et al., 2011).
Reported smallholder maize yields often do not exceed 0.7 t ha−1. However, with optimal soil water management, maize yields can easily be around 1.3 t ha−1 in the semi-arid medium-potential maize-growing zone in south-eastern Kenya (Omoyo et al., 2015). Few farmers use pesticides or improved seeds or other adaptation strategies (Tongruksawattana and Wainaina, 2019). In Kitui, Makueni and Machakos, the most preferred seed variety is the high-yielding but less drought resistant Kikamba/Kinyaya variety (120 growing days) with a potential yield of only 1.1 t ha−1 (Ifejika Speranza, 2010; Recha et al., 2015). Trend analysis (1994–2008) shows that yields are declining due to the increasing pace of recurring droughts (Nyandiko et al., 2014).
Over 97 % of the smallholder farmers in this area grow maize, mainly for their own consumption or local markets (Brooks et al., 2009; Kariuki, 2016; Nyariki and Wiggins, 1997). It is the main staple food, providing more than a third of caloric intake, and is also the primary ingredient used in animal feeds in Kenya (Adamtey et al., 2016; FAO, 2008). Only about 20 % of the farmers are able to sell their excess crops, while 66 % have to buy maize to complement their own production (Muyanga, 2004).
ADOPT (Fig. 2; Wens et al., 2020; ODD + D (overview, design concept, details + decision) protocol in Appendix A) is an agent-based model that links a crop production module to a behavioural module evaluating the two-way feedback between drought impacts and drought adaptation decisions. ADOPT was parameterized with information from expert interviews, and a farm household survey with 260 households including a semi-structured questionnaire executed in the Kitui region, Kenya (Wens et al., 2021). Moreover, a discrete choice experiment (a quantitative method to elicit preferences from participants without directly asking them to state their preferred options) was executed to obtain information on changes in adaptation intentions under future top-down disaster risk reduction (DRR) interventions (Wens et al., 2021). This empirical dataset feeds the decision rules in ADOPT describing farm households' adaptive behaviour in the face of changing environmental conditions (drought events), social networks (actions of neighbouring farmers), and top-down interventions (drought management policies). In ADOPT, crop production is modelled using AquaCrop-OS (Foster et al., 2017), simulating crop growth on a daily basis and producing crop yield values at harvest time twice per year. Calibrated for the Kenyan dryland conditions (Ngetich et al., 2012; Wamari et al., 2007), AquaCrop-OS considers the current water management of the farm (i.e. the applied drought adaptation measures), and yields vary with weather conditions. The adaptive behaviour of the farm households (agents) is modelled based on protection motivation theory (PMT; Rogers, 1983). This theory was identified as promising in an earlier study (Wens et al., 2020) and includes multiple relevant factors that drive the observed behaviour of farm households (Wens et al., 2021). In this application of ADOPT, the model was run over 30 historical years as the baseline followed by 30 years of future scenarios (combinations of policy and climate changes; the start of these changes is indicated as “year 0”). Through a sensitivity analysis, both the average effect of individual adaptation decisions and its endogenous model variability are analysed (similarly to in Wens et al., 2020). We used 12 different initializations per scenario to include variations in model initialization, the stochasticity that determines the individual adaptation decisions, and the relative weights of factors influencing behaviour (see Sect. 3.1).
3.1 Individual adaptive behaviour in ADOPT
Various soil water management practices, further called drought adaptation measures, can be adopted by smallholder farmers in ADOPT. There are shallow wells to provide irrigation water, the option to connect these to drip irrigation infrastructure, and fanya juu terraces as on-farm water harvesting techniques. Moreover, a soil protection measure reducing the evaporative stress, mulching, is included. These measures are beneficial in most – if not all – of the years and have a particularly good effect on maize yields in drought years. Nonetheless, current adoption rates of these measures are quite varied and often remain rather low (Gicheru, 1994; Kiboi et al., 2017; Kulecho and Weatherhead, 2006; Mo et al., 2016; Ngigi, 2008; Ngigi et al., 2000; Rutten, 2005; Ararso et al., 2016).
ADOPT applies protection motivation theory, a psychological theory often used to model farmer's boundedly rational adaptation behaviour (Schrieks et al., 2021). It describes how individuals adapt to shocks such as droughts and are motivated to react in a self-protective way towards a perceived threat (Grothmann and Patt, 2005; Maddux and Rogers, 1983). Four main factors determining farmers' adaptation intention under risk are modelled: (1) risk perception is modelled through the number of experienced droughts and number of adopted measures, household vulnerability, and experienced impact severity. Moreover, trust in early warnings is added, which can influence the risk appraisal if a warning is sent out. Coping appraisal is modelled through a farmer's (2) self-efficacy (household size/labour power, belief in God, vulnerability), (3) adaptation efficacy (perceived efficiency, cost and benefits, seasons in water scarcity, choices of neighbours, number of measures), and (4) adaptation costs (farm income, off-farm income, adaptation spending, access to credit). These four PMT factors receive a value between 0 and 1 and define a farmer's intention to adopt measures. Which smallholder farmers adopt which measures in which years is then stochastically determined based on this adaptation intention. More information regarding the decision-making can be found in Appendix A.
3.2 Drought risk indicators in ADOPT
In ADOPT, annual maize yield influences the income and thus assets of the (largely) subsistence farm households. This influence is indirect because the farm households are assumed to be both producers and consumers, securing their own food needs. The influence is also a direct one because these farm households sell their excess maize on the market at a price sensitive to demand and availability. Farm households that cannot satisfy their food needs by their own production turn to this same market. They buy the needed maize – if they can afford it and if there is still maize available on the market. If they do not have the financial capacity or if there is a market shortage, they are deemed to be food insecure. Their food shortage (the kilograms of maize short to meet household food demand) is multiplied by the market price to estimate their food aid needs. Adding the farm income of the household to their income from potential other sources of income, it is estimated whether they fall below the poverty line of USD 1.9 per day. Climate and weather variability cause maize yields to fluctuate over time, as do the prevalence of poverty, the share of households in food insecurity, and the total food aid needs. These factors can be seen as proxies for drought risk and were evaluated over time.
3.3 Climate change scenarios
Multiple climate change scenarios – all accounting for increased atmospheric carbon dioxide levels – were tested: a rising temperature of 10 %, a drying trend of 15 %, a wetting trend of 15 %, and various combinations of these (Table 1). The warming and drying trends were based on a continuation of the trends observed in the last 30 years of daily NCEP temperature (Kalnay et al., 1996) and CHIRPS precipitation (Funk et al., 2015) data (authors' calculations; similar trends found in Gebrechorkos et al., 2020). The wetting trend was inspired by the projections from most climate change models, which predict an increase in precipitation in the long rain season – a phenomenon known as the “East African climate paradox” (Gebrechorkos et al., 2019; Lyon and Vigaud, 2017; Niang et al., 2015). The no-change scenario was a repetition of the baseline period, without changing precipitation or temperature and hence only including elevated carbon dioxide levels. Reference evaporation was calculated for each scenario using the Penman–Monteith model and was thus influenced by temperature changes (Allen, 2005; Droogers and Allen, 2002).
These trends were added to time series of 30 years of observed data. While such an approach does not account for increased variability, it allows us to account for the temporal coherence in the data and the interrelationships among different weather variables (weather generators – another option to downscale projected climate – still have some progress to make in order to accurately account for extreme events; Mehan et al., 2017; Ailliot et al., 2015). This resulted in 30 years of synthetic “future” data, for each of the six – wet, hot–wet, hot, dry, hot–dry, and no change – scenarios. While they do not have a known probability of occurring, they enable testing the effect of the on-farm adaptations and top-down drought disaster risk reduction strategies on drought risk under changing average hydro-meteorological conditions.
Droughts, here defined as at least 3 months with standardized precipitation index (SPEI) values below −1, have a different rate of occurrence under these different future climate scenarios (Fig. 3). The SPEI is calculated through standardizing a fitted generalized extreme value distribution over the historical monthly time series and superimposing this onto the climate scenario time series. Under the no-change scenario, 25 % of the 30 simulated years fall below this threshold. Under the wet scenario, fewer droughts occur (15 % of the years), but under the dry scenario, the number of drought years more than doubles (54 % of the years). Temperature is dominant over precipitation in determining drought conditions, as under the hot–wet scenario, 41 % of years are recorded as drought years, and under hot–dry conditions, 78 % of the years can be considered drought years.
3.4 Drought risk reduction intervention scenarios
Kenya Vision 2030 for the arid and semi-arid lands (ASAL) promotes drought management through extension services and aims to increase access to financial services such as affordable credit schemes (Government of the Republic of Kenya, 2013). Besides, building on the Ending Drought Emergencies plan, the National Drought Management Authority prioritizes the customization, improvement, and dissemination of drought early warning systems. It aims to establish trigger levels for ex ante cash transfer so as to upscale drought risk financing (Government of the Republic of Kenya, 2013; National Drought Management Authority, 2016; Republic of Kenya, 2015). Improved extension services tailored to the changing needs of farm households (Muyanga and Jayne, 2006), a better early warning system with longer lead times (Van Eeuwijk, 2021), ex ante cash transfers to the most vulnerable when a drought is expected (Guimarães Nobre et al., 2019), and access to credit markets (Berger et al., 2017) are all assumed to increase farmers' intentions to adopt new measures.
As shown in Wens et al. (2021), extension services are most effective when offered to younger, less rich, and less educated people or to those who have already adopted the most common measures. Similarly, early warning systems change the intention to adapt mostly for less educated, less rich farmers or those who are not part of farmer knowledge exchange groups. The ex ante cash transfer drives the adoption of more expensive measures the most for those who already spend a lot of money on adaptation. Access to credit is preferred by less rich farmers who have a larger land size, are members of a farm group, have been to extension training, have easy access to information, and/or are highly educated (Wens et al., 2021).
In this application of ADOPT, the effects of these four interventions – extension services, early warning systems, ex ante cash transfer, and credit schemes – were tested individually. Additionally, three scenarios, combining different types of interventions, were evaluated, all initiated in year 0 in the model run.
In the reactive policy intervention “supporting drought recovery”, no (new, proactive) interventions are implemented. Only emergency aid (standard in the ADOPT model to avoid household members dying) is given to farmers who lost their livelihoods after drought disasters; this food aid is distributed to farmers who are on the verge of poverty to avoid famine.
In the proactive policy intervention plan “preparing for drought disasters”, improved early warnings are sent out each season if a drought is expected. This is assumed to raise all farmers' risk appraisal by 20 %. Ex ante cash transfers are given to all smallholder farmers (those without income off-farm and without commercialization) to strengthen resilience in the face of a drought. This is done when severe and extreme droughts (SPEI < −1 and ) are expected that could lead to crop yield lower than 500 and 300 kg ha−1, respectively. A money equivalent to the food insecurity following these yields is paid out to farmers with few external-income sources. Moreover, like in the reactive government scenario, emergency aid is given to farmers who need it.
In the prospective policy intervention plan (UNDRR, 2021) “mitigating (future) drought disasters”, credit rates are lowered so that it is affordable to people to take a loan for adaptation measures, at an interest rate of 2 % and a payback period of 5 years. Besides, emergency services are provided in the form of frequent training given in communities with poor practices to improve their capacity related to drought adaptation practices for agriculture. Moreover, like in the proactive government scenario, an improved early warning system is set up and an ex ante cash transfer is given. Lastly, emergency aid is given to farmers who need it.
4.1 Maize yield under different adaptation measures and future climate scenarios
The annual average maize yields under the different climate scenarios, for the four on-farm drought adaptation measures implemented in ADOPT – mulch, fanya juu bunds, shallow wells, and drip irrigation, were calculated using AquaCrop-OS (Fig. 4). Under wetter future climate conditions, maize yields are expected to increase under all management scenarios, with mulch having a particularly positive effect on the soil moisture conditions throughout the full growing season. Hotter climate conditions reduce yields slightly: the assumptions in this model about the frequency and amount of manual irrigation or drip irrigation water are not sufficient to diminish this effect, even under wetter conditions. Paired with drier conditions, this hotter future has dramatically negative effects on yields, showing on average 28 % lower yields compared to the no-climate-change scenario over all management scenarios.
4.2 The adoption of adaptation measures over time
In ADOPT, all evaluated top-down interventions increased the adoption rate of the evaluated adaptation measures compared to the reactive “no-intervention” scenario (Fig. 5): reduced credit rates, improved early warning systems, tailored extension services, and ex ante cash transfers, as well as the proactive and prospective scenarios, lead to increases in adoption as compared to the reactive scenario (colours in Fig. 5).
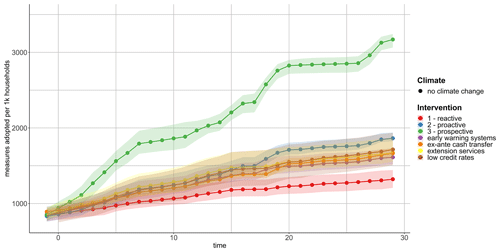
Figure 5Total number of measures adopted per 1000 initialized households under no climate change, averaged over all runs. The shaded area indicates the variation – uncertainty introduced by different model initializations and by the different relative importance of the PMT factors for the decisions of households (sensitivity analysis). Year 0 marks the initiation of policy drought risk reduction interventions (indicated with different line colours).
Looking in detail at the effect of possible policy interventions (Fig. 5, Table B2), affordable credit schemes had the highest effect on the adoption rate of drought adaptation measures. Furthermore, ex ante cash transfers (which cannot be seen as large sums of investment money but as a mere means to keep families food secure) were more effective in increasing adoption of the more affordable measures. Indeed, richer families had mostly already adopted these measures before policy interventions were in place. Extended extension service training increased the adoption of less popular measures and decreased the adoption of the popular but not as cost-effective fanya juu terraces. Early warning systems had more effect in the wetter climate conditions. The dry–hot scenario has so many drought episodes that risk perception is automatically high, while the alert level lowers when droughts become scarcer in the less dry scenarios.
Overall, although the processes through which the interventions support households to adapt differ significantly, the differences in the eventual adoption rate under the different interventions were small (they overlap in the uncertainty intervals). Also, the effect of climate change on the adoption rate (Fig. B1, Table B2) was rather small when evaluating the reactive (no-intervention) scenario. However, with interventions, the climate change scenarios differed more.
When examining the effect of the three intervention scenarios (Fig. B2, Table B2), it is clear that implementing multiple policies at once resulted in a stronger increase in adoption: a proactive and prospective intervention plan increased the adoption of different adaptation measures 40 % and 140 % more, respectively, than under the “reactive, no-climate-change” scenario where no intervention takes place. Both a proactive and a prospective approach increased the adoption of cheaper adaptation measures to close to 100 % of the farm households. For the more expensive measures, the proactive scenario was shown to be less effective, while the prospective scenario reached quite high adoption rates in the more extreme climate scenarios.
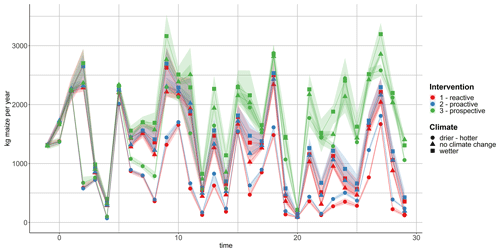
Figure 6Household maize harvest (kg per year, sum of two growing seasons) over 30 “scenario years” under different climate change and policy intervention scenarios. The shaded area indicates the variation – uncertainty introduced by different model initializations and by the different relative importance of the PMT factors for the decisions of households (sensitivity analysis).
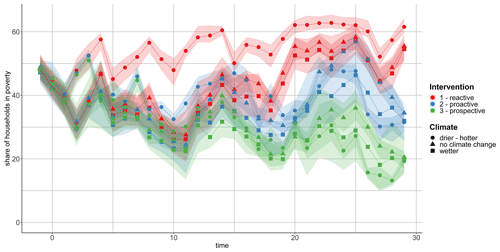
Figure 7Share of households in poverty (earning under the USD 2 per day income line, under different climate and policy intervention scenarios). The shaded area indicates the variation – uncertainty introduced by different model initializations and by the different relative importance of the PMT factors for the decisions of households (sensitivity analysis).
The adoption of adaptation measures by households influenced their maize yield and thus affected the average and median maize harvest under the different future climates and drought risk reduction interventions – with an increasing effect over the years (increasing difference in harvest between reactive and other scenarios, Fig. 6). This becomes clear comparing the first 30 baseline years with the following 30 scenario years: when no policy interventions were in place, average maize yields increased almost 30 % under a wet–hot future and decreased by over 25 % under a dry–hot climate. Under a prospective government supporting the adoption of adaptation measures, average maize yields increased by up to 100 % under a wet–hot future and increased by over 60 % under dry–hot future conditions. Clearly, an increased uptake of measures under this intervention scenario would potentially offset a potentially harmful drying climate trend.
4.3 Drought risk dynamics under policy and climate change
Assuming off-farm income fluctuates randomly but does not steadily increase or decrease, the changing harvests over time directly affected the poverty rate and the share of households in food insecurity (Fig. 7). Both trends in yield caused by droughts or by the adoption of new adaptation measures could drive farm households in or out of poverty. Running ADOPT with a reactive and no-climate-change scenario, a slight increase of 5 percentage points (pp) in poverty levels was visible. Poverty levels increased by up to 15 pp compared to the baseline situation, when a drier and/or hotter climate scenario was run. A proactive intervention plan reduced poverty by 11 pp under no climate change. In the dry–hot climate scenario this combination of improved early warning systems and ex ante cash transfers lead to reductions of 20–30 pp compared to the baseline years. However, the prospective government scenario showed the most prominent results, projecting reductions of 45 pp under no climate change and around 60 pp under drier and hotter climate conditions. It is important to remark that the difference between the intervention scenarios and the reactive scenario is only clearly visible after more than 10 years under most future climate scenarios.
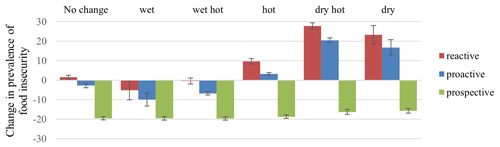
Figure 8Absolute change (average and standard deviation introduced by sensitivity analysis – variation caused by different model initializations and by the different relative importance of the PMT factors for the decisions of households) in the average share of households with food shortages of the last 20 years of the scenario run, compared to the first 20 years of the baseline run before year 0, under different climate and policy intervention scenarios. ADOPT model output.
Food insecurity is partly caused by a lack of income or assets but also by the farm market mechanism. Droughts, climate change, and adaptation levels influence the availability of maize on this market. Farm households which do not produce enough to be self-sufficient buy maize on the market if they have the money and if there is maize locally available. Households are assumed to face food shortages if they have to rely on food aid to fulfil their caloric needs. On average in the no-climate-change and “no-policy-intervention” scenarios, food security rates were predicted to remain stable compared to the baseline period (Fig. 8). However, policy interventions and climate change can alter this balance.
Improving extension services or providing ex ante cash transfers individually showed on average 7.5 % more reduction in food insecurity than the reactive government scenario. Improved early warning systems showed on average – over all climate scenarios – an increased reduction of 4.5 %. It should be kept in mind that ADOPT does not consider (illicit) coping activities in the face of droughts, which can – if a drought warning is sent out – allow households to avoid buying food at high market prices or to engage in other income-generating activities such as food stocking or charcoal burning (Eriksen et al., 2005). However, both of these interventions might reduce the food security threat. Credit schemes at 2 %, individually, lead to a more than 8 % reduction in food insecurity levels as compared to the reactive scenario; but even then, on average net food insecurity rates increase due to climate change. A proactive intervention resulted in a food insecurity rate which is 6 pp lower than under the reactive scenario but still showed increases in the prevalence of food insecurity under hotter and drier conditions. A prospective intervention, combining all four interventions, was able to consistently reduce the food insecurity levels over time, even under the dry–hot climate scenario. This scenario was able to counteract the increase in food insecurity, achieving a reduction in households with food shortages over time with on average 28 % compared to the reactive scenario, all climate scenarios considered.
Expressing drought impacts in the average annual food aid required (in USD) can help to evaluate the effect of different climate change scenarios or different policy intervention scenarios on the drought risk of the community. These estimations are translated to USD, assuming a maize price for shortage markets, as price volatility is considered. Table 2 shows the change in aid needs compared to the no-climate-change, no-top-down-intervention baseline period (based on the 1980–2000 situation). When assuming no climate change, it seemed that the community was stable, only slightly increasing the share in vulnerable households. More measures were adopted as information was disseminated throughout the farmer networks, but those who stay behind face lower selling prices as markets become more stable and have a harder time accumulating assets. Under wetter conditions, drought emergency aid needs did reduce. However, drier, hotter climates had a detrimental effect on food needs, with more vulnerable people crossing the food shortage threshold.
Table 2Change in aid needs (%) in 2030–2050 compared to 1980–2000 (average and standard deviation introduced by sensitivity analysis – variation caused by different model initializations and by the different relative importance of the PMT factors for the decisions of households) under different climate and policy intervention scenarios. ADOPT model output.
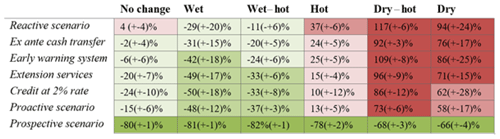
Under the no-climate-change scenario, each of the four policy interventions caused a reduction in aid needs, with credit schemes having the largest effect. Under wetter conditions, they also increased the reduction in aid needs compared to the reactive scenario. However, no individual measure was able to offset the effect of hotter and drier climate conditions. Even under a proactive intervention, there would still be an increase in aid needs under such climate conditions. Only under the prospective intervention scenario was a decrease in aid needs visible under all possible climate change scenarios.
5.1 The effect of early warning, extension services, ex ante transfers, and low interest rates
Under a reactive strategy (no intervention) and assuming no climate change, a slow but steady adoption of mulch, fanya juu, shallow well, and irrigation practices is estimated. This is a result of an ever-increasing information diffusion through the farmer networks and existing extension services, as also found in Hartwich et al. (2008), van Duinen et al. (2016), Villanueva et al. (2016), and Wossen et al. (2013). Yet, multiple smallholder households still suffer from the effects of droughts, indicated by the elevated food insecurity rates and poverty rates. While some can break the cycle of drought and subsequent income losses, others are trapped by financial or other barriers and end up in poverty and facing recurring food insecurity. This is also found by, for example, Enfors and Gordon (2008), Mango et al. (2009), Mosberg and Eriksen (2015), and Sherwood (2013). In the reactive scenario, it is clear that adaptation intention is limited by factors such as a low risk perception, high (initial) adaptation costs, a limited knowledge of the adaptation efficacy, or a low self-efficacy. Some of these barriers are alleviated through the different government interventions.
As compared to this reactive scenario, an increased rate of adoption is observed for all policy interventions. This translates into a comparatively lower drought risk (expressed by the indicators of the community poverty rate, food security, and aid needs). While initially extension services have the largest effect on the adoption of on-farm drought adaptation measures, over time access to credit results in the highest adoption rates and is also estimated to decrease emergency aid the most. The former, alleviating the knowledge (self-efficacy) barrier, increases adoption under no climate change with 27 % as compared to no intervention. It is indeed widely recognized as an innovation diffusion tool in different contexts (e.g. Aker, 2011; Hartwich et al., 2008; Wossen et al., 2013). The latter, alleviating the financial (adaptation costs) barrier, increases adoption under no climate change with 30 % as compared to no intervention. It is also found to be an effective policy to reduce poverty in Ghana by Wossen and Berger (2015). Ex ante cash transfers also tackle the financial barrier but less effectively (the cash sum is small and fixed – only significant for less wealthy households), increasing adoption under no climate change with 25 % as compared to no intervention. This matches empirical evidence on the positive effects of ex ante cash transfers (Asfaw et al., 2017; Barrientos, 2016; Pople et al., 2021). However, ADOPT model estimations might be an underestimation as the model does not account for many preparedness strategies of households such as stocking up on food while the price is still low, fallowing land to reduce farm expenses, or searching for other sources of income (Khisa and Oteng, 2014). Seasonal early warning systems, which raise awareness of upcoming droughts, increase the adoption of measures with 22 % as compared to no intervention. Early warnings have a stronger effect on the adoption of mulching or fanya juu terraces (cheaper measures, lower financial barrier) than of drip irrigation. Clearly, the positive effect of the interventions on household resilience varies, which is confirmed by the empirical findings of Wens et al. (2021).
The proactive government scenario, preparing for drought disasters by improving early warning systems and supporting ex ante cash transfers, has a larger effect on drought risk. However, this effect is not as much as the sum of the effect of the two interventions. In contrast, the prospective government scenario, mitigating drought disasters by combining all four interventions, alleviates multiple barriers to adoption at once. This creates a significant nonlinear increase in adoption, matching the significant positive correlation between the preferences for extension services, credit, and early warning in Wens et al. (2021). Consequently, this scenario results in a clear growth in resilience of the farm households, shown by more stable income, lower poverty rates, and less food insecurity. However, depending on the climate scenario applied, the effect of increased adoption due to prospective interventions on household maize production and thus on food security and poverty is only visible after a few years under drier conditions and after more than 10 years under wetter conditions.
5.2 The robustness of drought risk reduction interventions under climate change
Climate change influences the effectivity of the measures as well as farm households' experiences with droughts. Under all climate change scenarios, a lower adoption of adaptation measures compared to the no-climate-change assumption is observed. This could be explained by the fact that the perceived need to adapt is lower under wet conditions and the financial strength to adapt is lower under dry or hot conditions. This highlights two different barriers to adoption: risk appraisal lowers when the occurrence of drought impacts is less frequent, while coping appraisal lowers due to experiencing more drought impacts. This link between drought experiences, poverty and adaptation was also found in other studies (e.g. Gebrehiwot and van der Veen, 2015; Holden, 2015; Makoti and Waswa, 2015; Mude et al., 2007; Oluoko-Odingo, 2011; Van Winsen et al., 2016).
While their effect on the adoption rates seems rather small, the diverse climate change scenarios have a distinctly different effect on the evolution of drought risk in rural communities. Due to the adaptation choices of the farm households, average maize harvests are estimated to slightly increase under the no-climate-change scenario. A major increase is estimated under wet and wet–hot conditions, where both increased adoption and better maize-producing weather conditions play a role. Under hot, dry, and dry–hot conditions, the average household harvests are estimated to decrease (also found in Wamari et al., 2007). Increases in median and mean assets (household wealth) are estimated to slightly increase under the no-climate-change scenario. In this case, adaptation efforts are able to reduce the drought disaster risk. Drier climates might lead to decreases in median and mean assets if farm households are not supported through top-down interventions. Hotter climates are estimated to result in decreased median assets but increased average assets of the households. In this case, adaptation rates are not high enough to avoid increasing drought risk for the median households.
The proactive government scenario is estimated to level poverty and food security under hotter or drier climate change scenarios. The prospective government scenario is the only scenario estimated to reduce emergency aid under all possible future climates. However, it should be noted that it takes 1 to 2 decades to make a significant difference between the reactive stance and prospective intervention plan. In other words, with climate change effects already visible through an increased frequency of drought disasters and more to be expected within the following 10–20 years, prospective intervention should be started now in order to be benefit from the increased resilience in time under any of the evaluated futures.
5.3 ADOPT as a dynamic drought risk adaptation model
In the past decade, the use of agent-based models (ABMs) in ex post and ex ante evaluations of agricultural policies and agricultural climate mitigation has been progressively increasing (Kremmydas et al., 2018; Huber et al., 2018). A pioneer in agricultural ABMs is Berger (2001), who couples economic and hydrologic components into a spatial multi-agent system. This has been followed more recently by, for example, Van Oel and Van Der Veen (2011), Mehryar et al. (2019), and Zagaria et al. (2021). The socio-hydrological, agent-based ADOPT model follows this trend in that it fully couples a biophysical model – AquaCrop-OS – and a social decision model – simulating adaptation decisions using behavioural theories – through both impact and adaptation interactions.
The initial ADOPT model setup was created through interviews with stakeholders (Wens et al., 2020), and the adaptive behaviour is based on both existing economic–psychological theory and empirical household data (Wens et al., 2021). The assumption of heterogeneous, boundedly rational behaviour has been addressed by only a few risk studies so far (e.g. van Duinen et al., 2015a, b, 2016; Hailegiorgis et al., 2018; Keshavarz and Karami, 2016; Pouladi et al., 2019). These studies have implemented empirically supported and complex behavioural theories in ABMs similarly to ADOPT (Schrieks et al., 2021; Jager, 2021; Taberna et al., 2020; Waldman et al., 2020).
ADOPT differs from these models, however, through its specific aim to evaluate households and community drought disaster risk beyond the number of measures adopted, crop yield, or water use. Rarely (except, for example, Dobbie et al., 2018) do innovation diffusion ABMs use socio-economic metrics to evaluate drought impacts over time – although such risk proxies are of great social relevance. As such, ADOPT evaluates the heterogeneous changes in drought risk for farm households, influenced by potential top-down drought disaster risk reduction (DRR) interventions. It does so through simulating their influence on individual bottom-up drought adaptation decisions by these farm households and their effect on socio-economic proxies for drought risk (poverty rate, food security and aid needs). To our knowledge, this is rather novel in the field of DRR and drought risk assessments.
5.4 Uncertainties in ADOPT and limitations of investigated measures and interventions
While yield data have been validated over the historical period (Wens et al., 2020), the model output cannot be used as a predicting tool. This would require more extensive validations for which, currently, data are not available. Such data would include longitudinal information on household vulnerability and adaptation choices from areas where certain policies are being implemented or detailed data on aid needs for the case study area. The past average poverty and food insecurity rates matched observations (Wens et al., 2020). However, absolute levels of emergency aid needs are sensitive to the averages and fluctuations of household assets, which have proven harder to verify. Besides, poverty and food insecurity also depend on external food or labour markets and other influences which might change in the future. Moreover, the simulated climate scenarios are not entirely realistic (because variability changes are ignored and because the synthetic future data are created based on statistics rather than physical climate and weather system changes). Moreover, the East African climate paradox (Funk et al., 2019) creates its own set of challenges in predicting future weather conditions in the study area.
Unavoidably, multiple possible smallholder adaptation measures are omitted in this study: many more agricultural water management measures, agronomic actions, and other options under the umbrella of climate-smart agriculture exist. Besides, only four different policy interventions are evaluated while various others exist. Costs of these top-down interventions are unknown, making cost–benefit estimates regarding drought risk reduction strategies not possible for this study. Studying additional measures or interventions is possible using the ADOPT model but requires (the collection of) more data for parameterization and calibration.
Another future improvement to the model could be to directly sample the empirical household survey data (Wens et al., 2020) to create a synthetic agent set. Now, the creation of agents (households) with different characteristics is drawn from distribution functions based on frequencies in the empirical data. Such a one-to-one data-driven approach is similar to microsimulation and gaining popularity in the design of ABMs (Hassan et al., 2010). Lastly, the model application assumes no shifts in the processes underlying weather and human decision-making: both the synthetic future weather situation and the decision-making processes are based on past observations. To avoid the effect of systemic changes and the black swan effect, only 30 years of the future is modelled.
Because the model setup could not be fully validated and scenarios do not provide a complete overview of all possibilities, this study does not claim to provide a prediction of the future for south-eastern Kenya. However, ADOPT is meant to – rather than forecast drought impact – increase understanding of the differentiated effect of adaptation policies: the relative differences in the risk indicators are informative for the comparison of these top-down interventions under different changes in temperature and precipitation. This study showcases the application of ADOPT as a decision support tool. It evaluates the robustness of a few dedicatedly chosen policy interventions on farm household drought risk under climate scenarios that are deemed to be relevant for the specific area. Future research can use ADOPT to study the differentiated effect of these interventions on different types of households in order to tailor strategies and target the right beneficiaries of government interventions.
Top-down interventions, providing drought and adaptation information as well as supporting the capacity to act on the basis of this information, are needed to increase the resilience of smallholder farmers to current and future drought risk. However, to what extent these interventions will steer farmers' intentions to adopt drought adaptation measures and hence how effective they are in reducing the farm household drought risk often remain unknown. In this study, the agent-based drought risk adaptation model ADOPT is applied to evaluate the effect of potential future scenarios regarding climate change and policy interventions on agricultural drought risk in south-eastern Kenya. The smallholder farmers in this region face barriers to adopt drought adaptation measures such as mulching, fanya juu terraces, shallow wells, and drip irrigation to stabilize production and income.
ADOPT simulates their adaptive behaviour, influenced by drought occurrences under changing climate conditions. Adaptive behaviour is also influenced by top-down drought risk reduction interventions such as the introduction of ex ante cash transfers, affordable credit schemes, improved early warning systems, and tailored extension services. We demonstrate that the investigated interventions all increase the uptake of adaptation measures as compared to the reactive scenario under no climate change (business as usual). Extension services (+27 % uptake) multiply adaptation knowledge and thus increase self-efficacy among the smallholders, which increases the adoption of less popular drought adaptation measures. Accessible credit schemes (+30 % uptake), alleviating a financial barrier, are effective, especially for more expensive drought adaptation measures. Early warning systems (+22 % uptake), creating risk awareness, are more effective in climate scenarios with less frequent drought. Ex ante cash transfers (+25 % uptake) allow the least endowed households to climb out of the poverty trap by adopting low-cost drought adaptation measures and thus reducing future shocks. The effect of climate change on the adoption of adaptation measures is limited.
Moreover, this study proves that alleviating only one barrier to adoption has a limited result on the drought risk of the farm households. Under the proactive scenario (+40 % uptake), combining early warning with ex ante cash transfers, smallholder farmers are better supported to adopt drought adaptation measures and to create, on average, more wealth. However the effect of climate change on farm households' risk differs significantly under this proactive scenario. While for wetter conditions, this scenario is able to increase food security and reduce poverty, this is not sufficient to diminish the need for external food aid under every evaluated climate scenario. Only by combining all four interventions (+139 % uptake) is a strong increase in the adoption of measures estimated. Simultaneously increasing risk perception, reducing investment costs, and elevating self-efficacy creates nonlinear synergies. Under such a prospective government approach, ADOPT implies significantly reduced food insecurity, decreased poverty levels, and drastically lower drought emergency aid needs after 10 to 20 years, under all investigated climate change scenarios.
This study suggests that, in order to reach the current targets of the Sendai Framework for Disaster Risk Reduction, which aims at building a culture of resilience, and to achieve the sustainable development goals of “zero hunger”, “sustainable water management”, and “climate resilience”, a holistic approach is needed. While we present a proof of concept rather than predictive model, the results improve the understanding of future agricultural drought disaster risk under socio-economic, policy and climate trends. We provide evidence that agent-based models such as ADOPT can serve as decision support tools to tailor drought risk reduction interventions under uncertain future climate conditions: more research into the heterogeneous effect of the investigated top-down interventions on households' adaptation decisions and drought risk can provide information for the effective and efficient tailoring of the policy interventions. However, from this study, it is clear that multiple interventions – both (risk and adaptation) information provision and the creation of action perspectives – should be combined now to build a sustainable future for smallholder farmers in Kenya's drylands.
A1 Overview
A1.1 Purpose
What is the purpose of the model?
The purpose of ADOPT is to improve agricultural drought disaster risk assessments by including the complex adaptive behaviour of smallholder farmers. The ADOPT model simulates the welfare (poverty level, food security, and aid needs) of smallholder farm households over time as a function of climate effects on agricultural production, mitigated by implemented adaptation measures, and simulates the adoption of such measures as a function of economic, social, and psychological household characteristics. Understanding the two-way feedback between households' adaptation decisions and maize yield losses over time can help optimize drought impact estimations under climate and policy changes. ADOPT can be used to evaluate the adoption rate of adaptation measures under different climate and policy scenarios and hence contrast their effect on the drought disaster risk – approximated by food security and welfare – of smallholder farmers.
For whom is the model designed?
The ADOPT model can allow scientists to increase their understanding of the socio-hydrological reality of drought disaster risk and drought adaptation in a smallholder farming context. It can also help decision makers to design drought policies that target specific farm households and evaluate the effect of these policies on the households' drought vulnerability.
A1.2 Entities, state variables, and scales
What kinds of entities are in the model?
The agents in ADOPT are individual farm households that have farms of varying sizes and potentially off-farm income sources. Two other entities exist: the cropland (multiple fields) that yields maize production and is owned by the farm households and the market (one) where maize is sold and bought.
By what attributes are these entities characterized?
Farm households (see unified modelling language (UML) diagram, Fig. A1) have a farm – characterized by its farm size and the adaptation measures implemented on it. They also have a family size; a household head (male/female) with a certain age and education level; financial assets (wealth, expressed in USD); off-farm employment; and farm, food, and other expenses. Household heads have a memory regarding past drought impacts; have a perception about their own capacity; and, in varying degrees, have information about potential adaptation measures.
Cropland (farms) (see UML, Fig. A1), belonging to households, produce maize under changing weather conditions, influenced by potential adaptation measures affecting water management conditions. The market (see UML, Fig. A1) is influenced by local production and consumption, which results in a variable maize price depending on the balance between supply and demand. In the presented case study, we consider relatively isolated areas, less subjected to globalized market systems: maize price is variable following the total amount of locally produced maize to replicate the observed price volatility (with minimum and maximum prices derived from FEWS NET) during years of reduced production.
What are the exogenous factors/drivers of the model?
Two exogenous factors influence the farm household systems: daily weather (influenced by gradual climate change) and drought disaster risk reduction policies (top-down policy interventions supporting smallholder farmers). The first factor might alter the frequency and severity of droughts – which may lead to failed crop yields, while the latter affects the knowledge, access to credit, and risk perceptions of household members who are recipient of the policies.
How is space included in the model?
ADOPT runs on the scale of farm fields (size adjusted to the case study area). On this field scale, agricultural water management decisions (adaptation) interact with rainfall variability (drought hazard). However, spatially explicit fields are used only in the initialization phase, so neighbouring farms can be identified but do not play any further role: space is only represented in a spatially implicit way – all farms (cropland) receive the same amount of rain and sun, have the same soil type with a similar slope, and differ only in their farm size and management applied.
What are the temporal resolution and extent of the model?
One time step of ADOPT represents 1 year. The crop model part runs on a daily basis, producing the maize crop yield in every cropping season, but decisions by the farm households to eventually adopt new adaptation measures are only made once a year. Each year, the poverty status, food security situation, and potential food aid needs of all farm households are evaluated. The model runs 30 years of the historical baseline (+10 initialization years) and 30 scenario years.
A1.3 Process overview and scheduling
What entity does what and in what order?
Every year, farm income of the households is updated with the maize harvest sold at the current market price (see centre of the flowchart in Fig. A2). This harvest depends on the farm size of the household; the maize yields (defined by AquaCrop-OS), which may be affected by a drought potentially mitigated by implemented drought adaptation measures; and the food needs of the household (subsistence is prioritized over selling; household members can die or be born (stochastically determined, based on birth and mortality rates in the study area)). This farm income, together with a potential (fixed) off-farm income and with farm-size-dependent farm expenses, family-size-dependent household expenses, and potentially extra food expenses (if the household's own production was not sufficient to fulfil household food needs), alters the assets of the farm household. The farm household's memory of drought impacts (risk perception) is updated, and household members interact (in a random order) with their network of neighbours, exchanging information on adaptation measures.
Once a year, the household head decides whether they want to adopt a new drought adaptation measure. They make this decision based on their memory of past drought impacts, their perception of the adaptation costs, the knowledge of adaptation measures through their networks and training, and their perception of their own capacity. The adoption of a new measure changes the farm management of those farmers, directly changes their wealth (implementation costs) and the farm expenses for the following years (maintenance costs), and influences crop yield and crop vulnerability to drought – thus potential farm income – during the following years.
A2 Design concepts
A2.1 Theoretical and empirical background
Which general concepts, theories, or hypotheses underlie the model's design at the system level or at the level(s) of the sub-model(s)?
The multidisciplinary modelling approach of ADOPT is rooted in socio-hydrology (Sivapalan et al., 2012), where the human system both influences and adapts to the changing physical environment (in this case agricultural drought), and applies an agent-based approach to deal with heterogeneity in adaptive behaviour of smallholder households.
The setup/design of the model (the drought disaster risk system) is a result of participatory concept mapping with researchers and students of South Eastern Kenya University (SEKU); technical advisors of the Kitui County Ministry of Agriculture, Water and Livestock; experts from the Sasol Foundation; and five pilot households that have example farms for agricultural extension services. This information informed the decision context of ADOPT.
On what assumptions is/are the agents' decision model(s) based?
In the first design of ADOPT, three adaptive-behaviour scenarios were analysed, with increasing complexity. A “business-as-usual” scenario with no changing drought adaptation measures was tested, characterizing the “fixed adaptation” approach. The conventional expected utility theory (Von Neumann and Morgenstern, 1944) represents the widely used economist assessment of choice under risk and uncertainty. Simulating boundedly rational rather than economically rational adaptation decisions, protection motivation theory (Rogers, 1983) is used as a way to include psychological factors in the heterogeneous adaptive behaviour of smallholders.
Indeed, it is often stated that households' adaptive behaviour is boundedly rational and embedded in the economic, technological, social, and climatic context of the farmer (Adger, 2006). Knowing the risk is not enough to adapt; farmers should also believe the adaptation measure will be effective, be convinced that they have the ability to implement the measure, and be able to reasonably pay the costs (van Duinen et al., 2015b). Financial or knowledge constraints may limit economically rational decisions. Also age, gender, and education – intrinsic factors – can play a role (Burton, 2014). The perceived ability to do something (coping appraisal) influences the decision-making process (Eiser et al., 2012). This coping appraisal can be subject to intrinsic factors such as education level, sources of income, farm size, family size, gender, confidence and beliefs, risk aversion, and age (Shikuku et al., 2017; Okumu, 2013; Le Dang et al., 2014; Zhang et al., 2019).
In order to understand the observed adaptive behaviour of smallholder households, it is critical to incorporate such socio-economic factors into the decision-making framework of drought adaptation models (van Duinen et al., 2015a, b, 2016; Keshavarz and Karami, 2016; Rezaei et al., 2017; Singh and Chudasama, 2017; O'Brien et al., 2007; Lalani et al., 2016; Gbetibouo, 2009; Bryan et al., 2009, 2013; Deressa et al., 2009; Mandleni and Anim, 2011; Wheeler et al., 2013; Gebrehiwot and van der Veen, 2015). After we had promising results running ADOPT with the boundedly rational scenario, it was assumed that farmers show a bounded rationality in the further application of ADOPT.
Why is a certain decision model or certain decision models chosen?
Analysis of the past and intended behaviour of farm households in the region provided support for the choice of theory but also showed the need to include networks influencing risk perception and the capacity of the households. Besides helping to parameterize the model, it also helped to calibrate the influence of the different factors affecting the decision-making process of the farm household. Showing the effect of different assumptions about decision-making in the first exploration of ADOPT (Wens et al., 2020) and with empiric evidence on the adaptive behaviour (Wens et al., 2021), the decision rules in ADOPT are assumed be a good enough representation of the decision-making process regarding drought adaptation.
If the model/a sub-model (e.g. the decision model) is based on empirical data, where do the data come from?
ADOPT is designed/initialized with data from existing longitudinal household surveys (TAPRA, 2000, 2004, 2007, 2010) and from a fuzzy cognitive map of key informants and is parameterized/partially calibrated with data from a semi-structured household questionnaire among 260 smallholder farmers. Survey reports can be found here:
-
https://research.vu.nl/en/publications/survey-report-kitui-kenya-expert-evaluation-of-model- setup-and-pr (last access: 10 July 2021) and
-
https://research.vu.nl/en/publications/survey-report-kitui-kenya-results-of-a-questionaire- regardings-us (last access: 10 July 2021).
At which level of aggregation are the data available?
Data from the surveys are available on the individual household level.
A2.2 Individual decision-making
What are the subjects and objects of decision-making? On which level of aggregation is decision-making modelled?
In ADOPT, individual farm households make individual adaptation decisions about their farm water management (in the case study in Kenya – mulching, fanya juu terraces, drip irrigation, or shallow wells) to reduce their production vulnerability to droughts. There are no multiple levels of decision-making included.
What is the basic rationality behind agents' decision-making in the model? Do agents pursue an explicit objective or have other success criteria?
Farmers generally try to reduce their drought disaster risk (achieve food security, evade poverty, and avoid needing emergency aid) and thus try to maximize crop yields (diminish yield reduction under water-limited conditions) given the capacity they have to adopt adaptation measures.
How do agents make their decisions?
Protection motivation theory (Maddux and Rogers, 1983) (see Sect. A2.1) is used to explain the decision-making process of the households. PMT consists of two underlying cognitive mediating processes that cause individuals to adopt protective behaviours when faced with a hazard (Floyd et al., 2000): it suggests that the intention to protect (in this study, the farmers' intentions to adopt a new adaptation measure) is motivated by a person's risk appraisal and the perceived options to cope with risks. The former depends on, for example, farmers' risk perceptions, their own experiences with drought disasters and memory thereof, and experiences of risk events in their social networks. The latter is related to different factors such as perceived self-efficacy (i.e. assets and sources of income, education level, and family size), adaptation efficacy (land size, adaptation measure characteristics), and adaptation costs (expenses in relation to their income) (Gebrehiwot and van der Veen, 2015; Keshavarz and Karami, 2016; van Duinen et al., 2015a, b, 2016). Households do not have any other objective or success criteria. A detailed description of how PMT is modelled – including the sensitivity analysis regarding the relative weights of the PMT factors – can be found in Wens et al. (2019): in ADOPT, farm households develop an intention to adapt (protect) for each potential adaptation measure (m), which changes every year (t). If a household has the financial capacity to pay for a considered measure (Stefanović et al., 2015), the intention to adapt is translated into the likelihood the household will adopt this measure in the following years. (This can be influenced by having access to credit.) The actual adoption is stochastically derived from this likelihood to adopt a measure.
Although Stefanović et al. (2015), van Duinen et al. (2015a), and Keshavarz and Karami (2016) have found positive relationships between the factors of PMT and observed protective behaviour, a level of uncertainty exists related to the relative importance of risk appraisal and coping appraisal in the specific context of smallholder households' adaptation decisions in semi-arid Kenya. Therefore, the α and β parameters were introduced as weights for the two cognitive processes. To address the associated uncertainty, they were widely varied (α, β ϵ [0.334, 0.666]) in a sensitivity analysis.
Risk appraisal is formed by combining the perceived risk probability and perceived risk severity, shaped by rational and emotional factors (Deressa et al., 2009, 2011; van Duinen et al., 2015b). Whereas risk perception is based in part on past experiences, several studies have suggested that households place greater emphasis on recent harmful events (Gbetibouo, 2009; Rao et al., 2011; Eiser et al., 2012). To include this cognitive bias, risk appraisal is seen as a sort of subjective, personal drought disaster memory, defined as follows (Viglione et al., 2014):
with .
The drought occurrence in year t is a binary value with a value of 1 if the SPEI-3 value falls below −1. The disaster damage of a household is related to its harvest loss during the drought year, which is defined as the difference between its current and average harvest over the last 10 years.
Coping appraisal represents a household's subjective “ability to act to the costs of a drought adaptation measures, given the adaptation measures' efficiency in reducing risk” (Stefanović et al., 2015; van Duinen et al., 2015a). It is a combination of the household's self-efficacy, adaptation efficacy of the measure, and the measure's adaptation costs:
Although Stefanović et al. (2015), van Duinen et al. (2015b), and Keshavarz and Karami (2016) quantified the relationships between the factors driving the subjective coping appraisal of individuals, a level of uncertainty remains related to the relative importance of these drivers in the context of smallholder households' adaptation decisions in semi-arid Kenya. Therefore, weights (γ, δ, εϵ [0.25, 0.50]) were introduced and varied in a sensitivity analysis using different ADOPT model runs.
The adaptation costs of the possible measures are expressed in terms of a percentage of the households' assets. The adaptation efficacy is calculated as the percentage of yield gain per measure compared to the current yield. This can be influenced by access to extension services (which gives an objective yield gain based on future climate rather than an estimate based on current practices of neighbours).
Self-efficacy is assumed to be influenced by education level (capacity), household size (labour force), age, and gender, all being social factors found to influence risk aversion and adaptation decisions (Okumu, 2013; Charles et al., 2014; Tongruksawattana, 2014; Muriu-Ng'ang'a et al., 2017).
Do the agents adapt their behaviour to changing endogenous and exogenous state variables? And, if yes, how?
Exogenous factors influencing adaptation decisions in ADOPT include the climate and the policy context in which households exist. Crop losses induced by drought (a feature of the climate context) steer a household's perception of the drought disaster risks faced (risk appraisal). For example, experiences of historical droughts or receiving early warnings about upcoming drought affects individuals' evaluations of drought disaster risk, leading to a personal drought disaster risk judgement (e.g. Keshavarz et al., 2014; Singh and Chudasama, 2017). Besides, access to extension services (a feature of the climate context) can have a profound effect on whether or not individuals take proactive action (Kitinya et al., 2012; Shikuku et al., 2017). Endogenous factors, as explained above, include age, household size, education level, maize yield variability, and assets (and the potential access to the credit market).
Do spatial aspects play a role in the decision process?
Farmer networks (connections with neighbours) exist, and information is passed through these social networks.
Do temporal aspects play a role in the decision process?
Yes. Risk memory is based on the crop yield variability of the accumulated past years and gives farm households an expectation about the upcoming crop yield.
Do social norms or cultural values play a role in the decision-making process?
No (only implicitly included; see Sect. A2.9).
To what extent and how is uncertainty included in the agents' decision rules?
It is not included in the model.
A2.3 Learning
Is individual learning included in the decision process? How do individuals change their decision rules over time as a consequence of their experience?
Decision rules follow PMT and are thus fixed, but some rules differ among types of households. Households that do not regularly receive extension services are limited to only implementing measures that their neighbours have installed as they are not aware of the existence of others. Besides, farmers who receive training will form their perception of the adaptation efficacy in a more objective way (as they have knowledge of average yield results under the adaptation measures, while other farmers estimate this based on the yield of their peers with such measures).
Is collective learning implemented in the model?
No.
A2.4 Individual sensing
What endogenous and exogenous state variables are individuals assumed to sense and consider in their decisions? Is the sensing process erroneous?
Households are aware of their assets, past yields, income sources and stability, and household food needs (Fig. A1). Following the socio-hydrologic setup of the model, households with boundedly rational behaviour are embedded in and interact with their social and natural environment. Changes in rainfall patterns during the growing season will change households' risk perceptions through fluctuations in crop yield; drought memory will influence the adaptive behaviour of these households. Besides, there is a diffusion of technology due to interactions and knowledge exchanges among farm households as discussed above.
What state variables of other individuals can an individual perceive?
Households know not only their own but also their neighbours' current yields and management practices. They make assumptions about the adaptation efficacy based on this.
What is the spatial scale of sensing?
Individual sensing happens not only on a household level but also through the individual social network that the farmers have, containing 3 to 30 other farmers.
Are the mechanisms by which agents obtain information modelled explicitly, or are individuals simply assumed to know these variables?
Households can obtain information about early warnings and through extension training. Households also have a simulated information transfer moment with the farmers in their neighbourhood to exchange information on risk and yields.
Are the costs for cognition and the costs for gathering information explicitly included in the model?
No.
A2.5 Individual prediction
Which data use the agent to predict future conditions?
By extrapolating from historical yield experiences, farmers have expectations about their maize yield every year. If an early warning system is in place, farmers know about upcoming droughts that can influence their crop yield.
What internal models are agents assumed to use to estimate future conditions or consequences of their decisions?
Households receiving extension services have knowledge about the average (future) yield gain of adopting a new adaptation measure, which will influence their coping appraisal.
Might agents be erroneous in the prediction process, and how is it implemented?
Households without this access to training will predict the yield gain based on the extra yield of their neighbours who have already adopted the considered adaptation measure.
A2.6 Interaction
Are interactions among agents and entities assumed to be direct or indirect?
In ADOPT, households interact with their neighbours, shaping risk awareness and response attitude (Okumu, 2013; Koome, 2017; van Duinen et al., 2016). Such networks can enhance social learning and knowledge spillover, which influences people's adaptation intentions and choices of specific measures (Below et al., 2010; Tongruksawattana, 2014). Smallholder households learn from the other households in their social network about the implementation and benefits of drought adaptation measures (Below et al., 2010; Shikuku et al., 2017). In ADOPT, exchanges with neighbours shape risk perception – the individual perception moves in the direction of the social network average – and also shape perceived adaptation effectivity. Moreover, households with no access to extension services can only adopt measures already implemented by neighbours.
On what do the interactions depend?
Households are either more self-oriented, discussing matters with 10 neighbours, or group-oriented, sharing knowledge within a group/collective of 30 neighbouring households.
Spatial distance (neighbourhood) at initialization is the key driver of networks; it is assumed that householders would not walk more than 5 km to reach people in their network.
If the interactions involve communication, how are such communications represented?
Communication is not explicitly modelled.
If a coordination network exists, how does it affect the agent behaviour? Is the structure of the network imposed or emergent?
No coordination network exists.
A2.7 Collectives
Do the individuals form or belong to aggregations that affect and are affected by the individuals? How are collectives represented?
No. No fixed collectives exist as the social networks the agents have are individual in nature.
A2.8 Heterogeneity
Are the agents heterogeneous? If yes, which state variables and/or processes differ between the agents?
Household agents are heterogeneous in terms of state variables (i.e. farm size, household size, assets) and differ in their access to the credit market, extension services, and early warning systems, changing their adaptive behaviour (Asfaw et al., 2017; Shikuku et al., 2017; Okumu, 2013).
Are the agents heterogeneous in their decision-making? If yes, which decision models or decision objects differ between the agents?
Okumu (2013) and Shikuku et al. (2017), among others, found that state variables such as age, beliefs, gender, education of the household head, and the household size have significant effects on risk attitude. These factors are included in the model application of protection motivation theory through the self-efficacy factor.
A2.9 Stochasticity
What processes (including initialization) are modelled by assuming they are random or partly random?
The likelihood to adopt a measure of a household is directly derived from the intention to adapt the measure with the highest intention for that household. This is stochastically transferred into an actual decision about whether or not to adopt the measure. For every time step of the simulation, a random number between 0–1 is drawn for each household; if this is lower than their adaptation intention (also between 0–1) and the household is able to pay for the measure, then the household adopts it. This probabilistic way of looking at adaptation intention and the stochastic step to derive the actual decisions allow us to account for non-included factors introducing uncertainty into adaptive behaviour such as conservatism, social/cultural norms, physical health, or ambitiousness of the households. Moreover, a stochastic perturbation (multiplied with a random number with average 1 and SD 0.1) is also added to the maize yield per farm as calculated through AquaCrop-OS. This additional heterogeneity-inducing step is made to include effects of pests and diseases on the income and food security of farming households.
A2.10 Observation
What data are collected from the ABM for testing, understanding, and analysing it, and how and when are they collected?
The adoption of adaptation measures and their effect on the total crop production (and food stock on the market) and individual household wealth are tracked over the simulated years.
What key results, outputs, or characteristics of the model emerge from the individuals?
Drought disaster risk (the annual average of impacts over the run period) – expressed in terms of the average annual poverty rate, level of food security, and total emergency aid needs – emerges from the model. It is defined based on the socio-economic conditions of individual farm households.
A3 Details
A3.1 Implementation
How has the model been implemented?
The model is coded in R, which is able to link the two sub-models in NetLogo (the adaptive-behaviour sub-model) and MATLAB (AquaCrop-OS).
Is the model accessible and, if so, where?
No. The model is not (yet) accessible.
A3.2 Initialization
What is the initial state of the model world, i.e. at time t=0 of a simulation run?
At the initial stage, households and their characteristics are randomly created based on the mean and standard deviation (Table A1) derived from the household dataset, obtained from a survey on agricultural drought disaster risk with smallholders in the case study area (Wens, 2019). Off-farm income is linearly related to the household size and education level and negatively related to the farm size. Food and non-food expenditures are linearly related to the household size. Farm expenditures are linearly related to the farm size.
Is initialization always the same, or is it allowed to vary among simulations?
In ADOPT, multiple climate change scenarios and policy scenarios were initialized – this changed the exogenous variables in the model. Moreover, each initialization creates another synthetic agent set based on the average household characteristics. Besides, a sensitivity analysis is performed to evaluate assumptions about the relative weights of the PMT factors (Sect. A2.2). Each combination of climate and policy scenario is run 12 times (3 possible α values; 4 possible combinations of γ, δ, ε) to account for the endogenous variability and uncertainty.
Are initial values chosen arbitrarily or based on data?
The initialization values are based on observed household data. Survey data include a short questionnaire among employees of the Kenyan national disaster coordination units (n=10); semi-structured expert interviews (n=8) with NGOs, governmental water authorities, and pioneer farmers in the Kitui district in Kenya; and an in-depth questionnaire among 260 smallholder farmers in central Kitui. Extra information is derived from household surveys of 2000, 2004, 2007, and 2010, conducted by the Tegemeo Agricultural Policy Research and Analysis (TARAA) project of the Tegemeo Institute. Besides, the model initialization draws heavily from reports of CIAT (CIAT and World Bank, 2015), the FAO (Ansah et al., 2014), IFPRI (Erenstein et al., 2011) and the government of Kenya (Kitui County Integrated report 2013–2017, 2017), CCAFS (CCAFS, 2015), and research (e.g. Muhammad et al., 2010).
A3.3 Input data
Does the model use input from external sources such as data files or other models to represent processes that change over time?
The daily weather conditions from 1980–2010 (from CHIRPS and CFSR) are used as input time series; for the future climate scenarios, the same data but with temperature are used.
Besides, survey data on household behaviour and drought risk context are used. Raw reporting can be found in Wens (2018, 2019).
Where do data come from? How are they collected? What is the level of available data? How are they structured?
Data (also discussed in Wens et al., 2021) are collected in the field using a multi-method data survey approach (key informant interviews, fuzzy cognitive map, household questionnaire, and choice experiment). These data are used to design the model, to validate the use of PMT, to initialize the agent set, and to calibrate model outputs.
What are the variables, entities, and classes available in the data? What do they represent?
A full set of behavioural factors were evaluated through the household questionnaire, and these were linked to actual behaviour and to behavioural intentions, as well as to the results of the choice experiment investigating future behaviour (Wens et al., 2021). Besides, socio-economic and farm characteristics were questioned.
How are data selected to form the agent entities? How is the agent population generated and synthesized?
As discussed above, the data are used to create a representative set of agents. Household variable means and standard deviations were used to create distribution functions, and a synthetic agent set was created based on random draws from these functions. Moreover, correlation between different variables was maintained.
What are the relationships and patterns that exist in the data?
As discussed above, a relationship between the household income and household head education level or farm size exists. Next to correlations between socio-economic or agricultural characteristics, correlations between psychological factors and actual or prospective adaptation decisions were investigated and used to design the behavioural module of ADOPT.
A3.4 Sub-models
What, in detail, are the sub-models that represent the processes listed in “Process overview and scheduling”?
The FAO crop water model AquaCrop-OS (coded in MATLAB by Tim Foster; Foster et al., 2017) calculates seasonal crop production, based on hydro-climatologic conditions provided by the climate data and based on the agricultural management of the households. The agent-based model in which farming households decide on their drought adaptation measures is coded in NetLogo, a language specialized in ABMs. This contains the decision-making module, which is a model application of protection motivation theory as explained in Sect. A2.1. More detailed explanation about how this is done can be found in Wens et al. (2020).
How were sub-models designed or chosen, and how were they parameterized and then tested?
AquaCrop-OS was applied, parameterized, and calibrated following Ngetich et al. (2012) and Omyo et al. (2015), who both analysed and approved the functioning of this model to simulate maize yield under different climates in Kenya.
The decision sub-model is described above in the sub-sections about decision-making and theoretical foundations (Sect. A2.2). A more detailed description can be found in Wens et al. (2020).
What are the model parameters and their dimensions and reference values?
For AquaCrop-OS, Tables A3 and A4 give an overview of the parameters that are used. For the decision-making module, Table A2 gives an overview of the factors used.
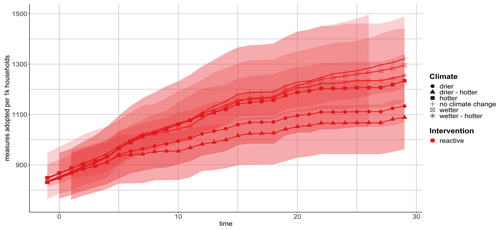
Figure B1Total number of measures adopted per 1000 initialized households under the reactive scenario, averaged over all runs. The shaded area indicates the uncertainty introduced by different model initializations and by the different relative importance of the PMT factors for the decisions of households. Year 0 marks the initiation of the policy drought risk reduction intervention.
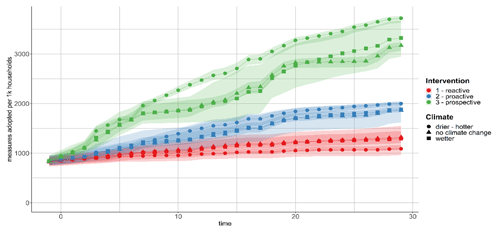
Figure B2Total number of measures adopted per 1000 initialized households under the three intervention scenarios and three climate change scenarios, averaged over all runs. The shaded area indicates the uncertainty introduced by different model initializations and by the different relative importance of the PMT factors for the decisions of households. Year 0 marks the initiation of policy drought risk reduction interventions (indicated with different line colours).
Table B1Adoption ratio (as a share of the population) at run year 30 under different climate and intervention scenarios. Note that the model showed an adoption rate of 25 % for mulch, 70 % for fanya juu terraces, 9 % for wells and 4 % for irrigation at run year 0 (start of climate change and policy scenarios).
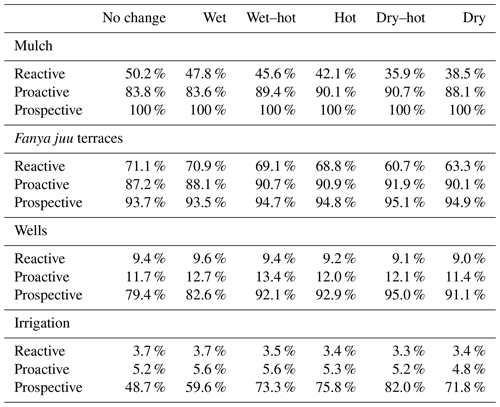
The input data used for this model application can be found in the following field reports:
MLKW took the lead in model development, scenario development, and writing the manuscript. TIEV assisted in model development; AFvL assisted with manuscript writing; and both TIEV and AFvL contributed to the scenario development. JCJHA was at the basis of the creative process of the model setup, development, and model application and contributed to the manuscript writing.
The contact author has declared that neither they nor their co-authors have any competing interests.
Publisher's note: Copernicus Publications remains neutral with regard to jurisdictional claims in published maps and institutional affiliations.
This article is part of the special issue “Drought vulnerability, risk, and impact assessments: bridging the science-policy gap”. It is not associated with a conference.
The authors would like to thank Moses N. Mwangi, Mary Mburu, Mutinda Munguti, and the entire Sasol staff for their help in creating the model contexts, discussing the initial model setup, and assisting with the data collection to calibrate the model.
This research has been supported by the Nederlandse Organisatie voor Wetenschappelijk Onderzoek (grant no. 453-583 13-006) and European Research Council (grants nos. 884442 and 948601).
This paper was edited by Lucia De Stefano and reviewed by Christian Troost and one anonymous referee.
Adamtey, N., Musyoka, M. W., Zundel, C., Cobo, J. G., Karanja, E., Fiaboe, K. K. M., Muriuki, A., Mucheru-Muna, M., Vanlauwe, B., Berset, E., Messmer, M. M., Gattinger, A., Bhullar, G. S., Cadisch, G., Fliessbach, A., Mäder, P., Niggli, U., and Foster, D.: Productivity, profitability and partial nutrient balance in maize-based conventional and organic farming systems in Kenya, Agric. Ecosyst. Environ., 235, 61–79, https://doi.org/10.1016/j.agee.2016.10.001, 2016.
Adger, W. N.: Vulnerability, Global Environ. Change, 16, 268–281, https://doi.org/10.1016/j.gloenvcha.2006.02.006, 2006.
Aerts, J. C. J. H. J. H., Botzen, W. J., Clarke, K. C., Cutter, S. L., Hall, J. W., Merz, B., Michel-Kerjan, E., Mysiak, J., Surminski, S., and Kunreuther, H.: Integrating human behaviour dynamics into flood disaster risk assessment, Nat. Clim. Change, 8, 193–199, https://doi.org/10.1038/s41558-018-0085-1, 2018.
Ailliot, P., Allard, D., Monbet, V., Naveau, P., Ailliot, P., Allard, D., Monbet, V., and Naveau, P.: Stochastic weather generators: an overview of weather type models Titre: Générateurs stochastiques de condition météorologiques: une revue des modèles à type de temps, J. la Société Française Stat., 156, 101–113, 2015.
Aker, J. C.: Dial “A” for agriculture: a review of information and communication technologies for agricultural extension in developing countries, Agric. Econ., 42, 631–647, https://doi.org/10.1111/j.1574-0862.2011.00545.x, 2011.
Allen, R.: Penman–Monteith Equation, in: Encyclopedia of Soils in the Environment, Elsevier, 180–188, https://doi.org/10.1016/B0-12-348530-4/00399-4, 2005.
Ansah, I. G. K., Oduro, H., and Osae, A. L.: A Comparative Analysis of Profit Efficiency in Maize and Cowpea Production in the Ejura Sekyedumase District of the Ashanti Region, Ghana, Res. Appl. Econ., 6, 106, https://doi.org/10.5296/rae.v6i4.6320, 2014.
Ararso, E., Geremu, T., Ayele, G., Mamo, D., Diriba, A., and Zone, W. H.: Effects of Level Fanya Juu and Fanya Chin Structures on Grain Yield of Maize in Moisture Stress Areas of Daro Labu District, J. Biol. Agricult. Healthcare, 6, 94–98, 2016.
Asfaw, S., Carraro, A., Davis, B., Handa, S., and Seidenfeld, D.: Cash Transfer Programmes for Managing Climate Risk: Evidence from a Randomized Experiment in Zambia, FAO, Rome, 35 pp., 2017.
Barrientos, A.: From Evidence to Action: The Story of Cash Transfers and Impact Evaluation in Sub-Saharan Africa, Edited by Benjamin Davis, Sudhanshu Handa, J. Dev. Stud., 52, 1831–1832, https://doi.org/10.1080/00220388.2016.1222679, 2016.
Barron, J. and Okwach, G.: Run-off water harvesting for dry spell mitigation in maize (Zea mays L.): results from on-farm research in semi-arid Kenya, J. Agricult. Water Manage., 74, 1–21, https://doi.org/10.1016/j.agwat.2004.11.002, 2005.
Below, T., Artner, A., Siebert, R., and Seiber, S.: Micro-level Practices to Adapt to Climate Change for African Small-scale Farmers: a review of selected literature, Discuss. Pap., IFPRI, 0953, 28, 2010.
Berger, T.: Agent-based spatial models applied to agriculture: a simulation tool for technology diffusion, resource use changes and policy analysis, Agric. Econ., 25, 245–260, https://doi.org/10.1016/S0169-5150(01)00082-2, 2001.
Berger, T., Troost, C., Wossen, T., Latynskiy, E., Tesfaye, K., and Gbegbelegbe, S.: Can smallholder farmers adapt to climate variability, and how effective are policy interventions? Agent-based simulation results for Ethiopia, Agric. Econ., 48, 693–706, https://doi.org/10.1111/agec.12367, 2017.
Blair, P. and Buytaert, W.: Socio-hydrological modelling: A review asking “why, what and how?”, Hydrol. Earth Syst. Sci., 20, 443–478, https://doi.org/10.5194/hess-20-443-2016, 2016.
Brooks, S., Thompson, J., Odame, H., Kibaara, B., Nderitu, S., Karin, F., and Millstone, E.: Environmental change and maize innovation in Kenya: Exploring pathways in and out of maize, STEPS Work. Pap., 1–75, 2009.
Brown, C., Meeks, R., Hunu, K., and Yu, W.: Hydroclimate risk to economic growth in sub-Saharan Africa, Climatic Change, 106, 621–647, https://doi.org/10.1007/s10584-010-9956-9, 2011.
Bryan, E., Deressa, T. T., Gbetibouo, G. A., and Ringler, C.: Adaptation to climate change in Ethiopia and South Africa: options and constraints, Environ. Sci. Policy, 12, 413–426, https://doi.org/10.1016/j.envsci.2008.11.002, 2009.
Bryan, E., Ringler, C., Okoba, B., Roncoli, C., Silvestri, S., and Herrero, M.: Adapting agriculture to climate change in Kenya: Household strategies and determinants, J. Environ. Manage., 114, 26–35, https://doi.org/10.1016/j.jenvman.2012.10.036, 2013.
Burton, R. J. F.: The influence of farmer demographic characteristics on environmental behaviour: A review, J. Environ. Manage., 135, 19–26, https://doi.org/10.1016/j.jenvman.2013.12.005, 2014.
Carrao, H., Naumann, G., and Barbosa, P.: Mapping global patterns of drought risk: An empirical framework based on sub-national estimates of hazard, exposure and vulnerability, Global Environ. Change, 39, 108–124, https://doi.org/10.1016/j.gloenvcha.2016.04.012, 2016.
CCAFS: Baseline Indicators for Makueni/Wote in Kenya, East Africa, CGIAR Research Program on Climate Change, Agriculture and Food Security (CCAFS), Copenhagen, Denmark, 2015.
Cervigni, R. and Morris, M. (Eds.): Confronting Drought in Africa's Drylands: Opportunities for Enhancing Resilience, Africa Development Forum series, World Bank, Washington, D.C., https://doi.org/10.1596/978-1-4648-0817-3, 2016.
Charles, N., Rashid, H., and James, C.: Analysis of determinants of farm-level adaptation measures to climate change in Southern Africa, J. Dev. Agric. Econ., 6, 232–241, https://doi.org/10.5897/JDAE12.0441, 2014.
CIAT and World Bank: Climate-Smart Agriculture in Kenya, CSA Country Profiles for Africa, Asia, and Latin America and the Caribbean Series, The World Bank Group, Washington, D.C., https://cgspace.cgiar.org/handle/10568/69545Washington (last access: May 2021), 2015.
Conway, D., Nicholls, R. J., Brown, S., Tebboth, M. G. L., Adger, W. N., Ahmad, B., Biemans, H., Crick, F., Lutz, A. F., De Campos, R. S., Said, M., Singh, C., Zaroug, M. A. H., Ludi, E., New, M., and Wester, P.: The need for bottom-up assessments of climate risks and adaptation in climate-sensitive regions, Nat. Clim. Change, 9, 503–511, https://doi.org/10.1038/s41558-019-0502-0, 2019.
D'alessandro, S. P., Caballero, J., Lichte, J., and Simpkin, S.: Kenya Agricultural Risk Assessment, Washington, D.C., https://openknowledge.worldbank.org/handle/10986/23350 (last access: May 2021), 2015.
Deressa, T. T., Hassan, R. M., Ringler, C., Alemu, T., and Yesuf, M.: Determinants of farmers' choice of adaptation methods to climate change in the Nile Basin of Ethiopia, Global Environ. Change, 19, 248–255, https://doi.org/10.1016/j.gloenvcha.2009.01.002, 2009.
Deressa, T. T., Hassan, R. M., and Ringler, C.: Perception of and adaptation to climate change by farmers in the Nile basin of Ethiopia, J. Agric. Sci., 149, 23–31, https://doi.org/10.1017/S0021859610000687, 2011.
De Stefano, L., Tánago, I. G., Ballesteros, M., Urquijo, J., Blauhut, V., James, H., and Stahl, K.: Methodological Approach Considering Different Factors Influencing Vulnerability – Pan-European Scale, Technical Report, 128 pp., https://www.academia.edu/14388306/METHODOLOGICAL_APPROACH_CONSIDERING_DIFFERENT_FACTORS_INFLUENCING_VULNERABILITY_PAN_EUROPEAN_SCALE (last access: May 2021), 2015.
Di Baldassarre, G., Martinez, F., Kalantari, Z., and Viglione, A.: Drought and flood in the Anthropocene: Feedback mechanisms in reservoir operation, Earth Syst. Dynam., 8, 225–233, https://doi.org/10.5194/esd-8-225-2017, 2017.
Di Falco, S.: Adaptation to climate change in Sub-Saharan agriculture: Assessing the evidence and rethinking the drivers, Eur. Rev. Agricult. Econ., 41, 405–430, https://doi.org/10.1093/erae/jbu014, 2014.
Dobbie, S., Schreckenberg, K., Dyke, J. G., Schaafsma, M., and Balbi, S.: Agent-Based Modelling to Assess Community Food Security and Sustainable Livelihoods, J. Artif. Soc. Soc. Simul., 21, 9, https://doi.org/10.18564/jasss.3639, 2018.
Droogers, P. and Allen, R. G.: Estimating reference evapotranspiration under inaccurate data conditions, Irrig. Drain. Syst., 16, 33–45, https://doi.org/10.1023/A:1015508322413, 2002.
Eiser, J. R., Bostrom, A., Burton, I., Johnston, D. M., McClure, J., Paton, D., van der Pligt, J., and White, M. P.: Risk interpretation and action: A conceptual framework for responses to natural hazards, Int. J. Disast. Risk Reduct., 1, 5–16, https://doi.org/10.1016/j.ijdrr.2012.05.002, 2012.
Enfors, E. I. and Gordon, L. J.: Dealing with drought: The challenge of using water system technologies to break dryland poverty traps, Global Environ. Change, 18, 607–616, https://doi.org/10.1016/j.gloenvcha.2008.07.006, 2008.
Erenstein, O., Kassie, G. T., and Mwangi, W.: Comparative analysis of maize based livelihoods in drought prone regions of eastern Africa: Adaptation lessons for climate change, Increasing Agric. Product. Enhancing Food Secur. Africa New Challenges Oppor., 1–13, https://ccafs.cgiar.org/resources/publications/comparative-analysis-maize-based-livelihoods-drought-prone -regions (last access: May 2021), 2011.
Eriksen, S. H., Brown, K., and Kelly, M.: The dynamics of vulnerability: locating coping strategies in Kenya and Tanzania, Geogr. J., 171, 287–305, 2005.
FAO: Farmer field schools on land and water management, 24–29, https://www.fao.org/documents/card/en/c/56bcd1cd-7912-5c89-baa1-ddf1fafd5f6c/ (last access: May 2021), 2008.
Filatova, T., Verburg, P. H., Parker, D. C., and Stannard, C. A.: Spatial agent-based models for socio-ecological systems: Challenges and prospects, Environ. Model. Softw., 45, 1–7, https://doi.org/10.1016/j.envsoft.2013.03.017, 2013.
Floyd, D. L., Prentice-Dunn, S., and Rogers, R. W.: A meta-analysis of research on protection motivation theory, J. Appl. Soc. Psychol., 30, 407–429, https://doi.org/10.1111/j.1559-1816.2000.tb02323.x, 2000.
Foster, T., Brozović, N., Butler, A. P., Neale, C. M. U., Raes, D., Steduto, P., Fereres, E., and Hsiao, T. C.: AquaCrop-OS: An open source version of FAO's crop water productivity model, Agr. Water Manage., 181, 18–22, https://doi.org/10.1016/j.agwat.2016.11.015, 2017.
Funk, C., Peterson, P., Landsfeld, M., Pedreros, D., Verdin, J., Shukla, S., Husak, G., Rowland, J., Harrison, L., Hoell, A., and Michaelsen, J.: The climate hazards infrared precipitation with stations – a new environmental record for monitoring extremes, Sci. Data, 2, 150066, https://doi.org/10.1038/sdata.2015.66, 2015.
Funk, C. C., Nicholson, S. E., and Fink, A.: 3A.6A Moving Beyond the East African Climate Paradox, AMS 99th annual meeting, https://ams.confex.com/ams/2019Annual/webprogram/Paper352397.html (last access: May 2021), 2019.
Gbetibouo, G. A.: Understanding Farmers' Perceptions and Adaptations to Climate Change and Variability: The Case of the Limpopo Basin, South Africa, https://www.ifpri.org/publication/understanding-farmers-perceptions-and-adaptations-climate- (last access: May 2021), 2009.
Gebrechorkos, S. H., Hülsmann, S., and Bernhofer, C.: Analysis of climate variability and droughts in East Africa using high-resolution climate data products, Global Planet. Change, 186, 103130, https://doi.org/10.1016/j.gloplacha.2020.103130, 2020.
Gebrehiwot, T. and van der Veen, A.: Farmers Prone to Drought Risk: Why Some Farmers Undertake Farm-Level Risk-Reduction Measures While Others Not?, Environ. Manage., 55, 588–602, https://doi.org/10.1007/s00267-014-0415-7, 2015.
Gicheru, P. T.: Effects of residue mulch and tillage on soil moisture conservation, Soil Technol., 7, 209–220, https://doi.org/10.1016/0933-3630(94)90022-1, 1994.
Government of the Republic of Kenya: Sector plan for drought risk management and ending drought risk, Drought, Risk Management, and Policy, CRC Press, Nairoibi, https://doi.org/10.1201/b14918, 2013.
Grothmann, T. and Patt, A.: Adaptive capacity and human cognition : The process of individual adaptation to climate change, Global Environ. Change, 15, 199–213, https://doi.org/10.1016/j.gloenvcha.2005.01.002, 2005.
Guimarães Nobre, G., Davenport, F., Bischiniotis, K., Veldkamp, T., Jongman, B., Funk, C. C., Husak, G., Ward, P. J., and Aerts, J. C. J. H.: Financing agricultural drought risk through ex-ante cash transfers, Sci. Total Environ., 653, 523–535, https://doi.org/10.1016/j.scitotenv.2018.10.406, 2019.
Hailegiorgis, A., Crooks, A., and Cioffi-Revilla, C.: An agent-based model of rural households' adaptation to climate change, J. Artif. Soc. Soc. Simul., 21, 4, https://doi.org/10.18564/jasss.3812, 2018.
Hartwich, F., Halgin, D., and Monge, M.: How Change Agents and Social Capital Influence the Adoption of Innovations among Small Farmers. Evidence from Social Networks in Rural Bolivia, IFPRI Discussion Paper, 76 ST-How Change Agents and Social Capital Infl pp., ET – 00761, https://doi.org/10.5367/000000008784648889, 2008.
Hassan, S., Antunes, L., and Gilbert, N.: Simulating Interacting Agents and Social Phenomena, edited by: Takadama, K., Cioffi-Revilla, C., and Deffuant, G., Springer, Tokyo, Japan, https://doi.org/10.1007/978-4-431-99781-8, 2010.
Holden, S. T.: Risk Preferences, Shocks and Technology Adoption: Farmers' Responses to Drought Risk, https://www.econstor.eu/handle/10419/242738 (last access: May 2021), 2015.
Huber, R., Bakker, M., Balmann, A., Berger, T., Bithell, M., Brown, C., Grêt-Regamey, A., Xiong, H., Le, Q. B., Mack, G., Meyfroidt, P., Millington, J., Müller, B., Polhill, J. G., Sun, Z., Seidl, R., Troost, C., and Finger, R.: Representation of decision-making in European agricultural agent-based models, Agric. Syst., 167, 143–160, https://doi.org/10.1016/j.agsy.2018.09.007, 2018.
Ifejika Speranza, C.: Drought Coping and Adaptation Strategies: Understanding Adaptations to Climate Change in Agro-pastoral Livestock Production in Makueni District, Kenya, Eur. J. Dev. Res., 22, 623–642, https://doi.org/10.1057/ejdr.2010.39, 2010.
Ifejika Speranza, C., Kiteme, B., and Wiesmann, U.: Droughts and famines: The underlying factors and the causal links among agro-pastoral households in semi-arid Makueni district, Kenya, Global Environ. Change, 18, 220–233, https://doi.org/10.1016/j.gloenvcha.2007.05.001, 2008.
Jager, W.: Using agent-based modelling to explore behavioural dynamics affecting our climate, Curr. Opin. Psychol., 42, 133–139, https://doi.org/10.1016/j.copsyc.2021.06.024, 2021.
Kalnay, E., Kanamitsu, M., Kistler, R., Collins, W., Deaven, D., Gandin, L., Iredell, M., Saha, S., White, G., Woollen, J., Zhu, Y., Chelliah, M., Ebisuzaki, W., Higgins, J., Janowiak, K., Ropelewski, C., Wang, J., Leetmaa, A., Reynolds, R., Jenne, R., and Joseph, D.: 40-Year Reanalysis Project, B. Am. Meteorol. Soc., 77, 437–470, 1996.
Kariuki, G. M.: Effect of Climate Variability on Output and Yields of Selected Crops in Kenya, Kenyatta University, https://ir-library.ku.ac.ke/bitstream/handle/123456789/17622/Effect of climate variability on output.....pdf?isAllowed=y&sequence=1 (last access: May 2021), 2016.
Kelly, R. A., Jakeman, A. J., Barreteau, O., Borsuk, M. E., ElSawah, S., Hamilton, S. H., Henriksen, H. J., Kuikka, S., Maier, H. R., Rizzoli, A. E., van Delden, H., and Voinov, A. A.: Selecting among five common modelling approaches for integrated environmental assessment and management, Environ. Model. Softw., 47, 159–181, https://doi.org/10.1016/j.envsoft.2013.05.005, 2013.
Keshavarz, M. and Karami, E.: Farmers' pro-environmental behavior under drought: Application of protection motivation theory, J. Arid Environ., 127, 128–136, https://doi.org/10.1016/j.jaridenv.2015.11.010, 2016.
Keshavarz, M., Karami, E., and Zibaei, M.: Adaptation of Iranian farmers to climate variability and change, Reg. Environ. Change, 14, 1163–1174, https://doi.org/10.1007/s10113-013-0558-8, 2014.
Khisa, G. V.: People's perception on climate change and its effects on livelihood in Kitui County, Int. J. Dev. Sustain., 7, 70–81, 2018.
Khisa, G. V. and Gladys, K. V.: Rainfall and temperature variability and its effect on food security in Kitui county, Kenya, Int. J. Dev. Sustain., 6, 924–939, 2017.
Khisa, G. V. and Oteng, S. B.: Coping Strategies against Climate Change in Agricultural Production in Kitui District, Kenya, J. Agric. Nat. Resour. Sci., 1, 71–86, 2014.
Kiboi, M. N., Ngetich, K. F., Diels, J., Mucheru-Muna, M., Mugwe, J., and Mugendi, D. N.: Minimum tillage, tied ridging and mulching for better maize yield and yield stability in the Central Highlands of Kenya, Soil Tillage Res., 170, 157–166, https://doi.org/10.1016/j.still.2017.04.001, 2017.
Kitinya, K. T., Onwonga, R. N., Onyango, C., Mbuvi, J. P., and Kironchi, G.: Climate Change and Variability: Farmers' Perception, Experience and Adaptation Strategies in C in Makueni County, Kenya, Asian J. Agriculut. Rural Dev., 2, 411–421, 2012.
Kitonyo, O. M., Chemining'wa, G. N., and Muthomi, J. W.: Productivity of farmer-preferred maize varieties intercropped with beans in semi-arid Kenya, Int. J. Agron. Agricult. Res., 3, 6–16, 2013.
Kitui County Integrated report 2013–2017: Republic Of Kenya County Government Of Kitui First County Integrated Development Plan 2013–2017, Planning for Sustainable Socio-Economic Growth and Development Towards a Globally Competitive and Prosperous Nation, 424 pp., https://repository.kippra.or.ke/handle/123456789/585?show=full (last access: May 2021), 2017.
Koome, D. N.: Factors Influencing the Adoption of “Zai” Pit Farming Technology To Enhance Food Security: the Case of Makueni County, Kenya By, University of Nairobi, Nairobi, http://erepository.uonbi.ac.ke/handle/11295/103267 (last access: May 2021), 2017.
Kremmydas, D., Athanasiadis, I. N., and Rozakis, S.: A review of Agent Based Modeling for agricultural policy evaluation, Agric. Syst., 164, 95–106, https://doi.org/10.1016/j.agsy.2018.03.010, 2018.
Kulecho, I. K. and Weatherhead, E. K.: Adoption and experience of low-cost drip irrigation in Kenya, Irrig. Drain., 55, 435–444, https://doi.org/10.1002/ird.261, 2006.
Laatabi, A., Marilleau, N., Nguyen-Huu, T., Hbid, H., Babram, M. A., and Abdellah, M.: ODD+2D: An ODD Based Protocol for Mapping Data to Empirical ABMs, J. Artific. Soc. Social Simul., 21, 9, https://doi.org/10.18564/jasss.3646, 2018.
Lalani, B., Dorward, P., Holloway, G., and Wauters, E.: Smallholder farmers' motivations for using Conservation Agriculture and the roles of yield, labour and soil fertility in decision making, Agric. Syst., 146, 80–90, https://doi.org/10.1016/j.agsy.2016.04.002, 2016.
Le Dang, H., Li, E., Nuberg, I., and Bruwer, J.: Farmers' assessments of private adaptive measures to climate change and influential factors: A study in the Mekong Delta, Vietnam, Nat. Hazards, 71, 385–401, https://doi.org/10.1007/s11069-013-0931-4, 2014.
Lyon, B. and Vigaud, N.: Unraveling East Africa's Climate Paradox, AGU, 265–281, https://doi.org/10.1002/9781119068020.ch16, 2017.
Maddux, J. E. and Rogers, R. W.: Protection motivation and self-efficacy: A revised theory of fear appeals and attitude change, J. Exp. Soc. Psychol., 19, 469–479, https://doi.org/10.1016/0022-1031(83)90023-9, 1983.
Makoti, A. and Waswa, F.: Rural Community Coping Strategies with Drought-Driven Food Insecurity in Kwale County, Kenya, J. Food Secur., 3, 87–93, https://doi.org/10.12691/jfs-3-3-4, 2015.
Mandleni, B. and Anim, F. D. K.: Climate Change Awareness and Decision on Adaptation Measures by Livestock Farmers in South Africa, J. Agric. Sci., 3, 1–26, https://doi.org/10.5539/jas.v3n3p258, 2011.
Mango, N., Kristjanson, P., Krishna, A., Radeny, M., Omolo, A., and Arunga, M.: Why is it some households fall into poverty at the same time others are escaping poverty? Evidence from Kenya, Nairobi, https://www.ilri.org/publications/why-it-some-households-fall-poverty-same-time-others-are- (last access: May 2021), 2009.
Matthews, R. B., Gilbert, N. G., Roach, A., Polhill, J. G., and Gotts, N. M.: Agent-based land-use models: A review of applications, Landscape Ecol., 22, 1447–1459, https://doi.org/10.1007/s10980-007-9135-1, 2007.
Mehan, S., Guo, T., Gitau, M., and Flanagan, D. C.: Comparative Study of Different Stochastic Weather Generators for Long-Term Climate Data Simulation, MDPI Climate, 5, 26, https://doi.org/10.3390/cli5020026, 2017.
Mehryar, S., Sliuzas, R., Schwarz, N., Sharifi, A., and van Maarseveen, M.: From individual Fuzzy Cognitive Maps to Agent Based Models: Modeling multi-factorial and multi-stakeholder decision-making for water scarcity, J. Environ. Manage., 250, 109482, https://doi.org/10.1016/j.jenvman.2019.109482, 2019.
Mo, F., Wang, J.-Y., Xiong, Y.-C., Nguluu, S. N., and Li, F.-M.: Ridge-furrow mulching system in semiarid Kenya: A promising solution to improve soil water availability and maize productivity, Eur. J. Agron., 80, 124–136, https://doi.org/10.1016/j.eja.2016.07.005, 2016.
Mosberg, M. and Eriksen, S. H.: Responding to climate variability and change in dryland Kenya: The role of illicit coping strategies in the politics of adaptation, Global Environ. Change, 35, 545–557, https://doi.org/10.1016/j.gloenvcha.2015.09.006, 2015.
Mude, A., Ouma, R., Steeg, J. van de, Kariuki, J., Opiyo, D., and Tipilda, A.: Kenya Adaptation to Climate Change in the Arid Lands: Anticipating, Adapting to and Coping with Climate Risks in Kenya – Operational Recommendations for KACCAL, ILRI Research Report 18, ILRI – International Livestock Research Institute, Nairobi, 135 pp., https://cgspace.cgiar.org/handle/10568/2186 (last access: May 2021), 2007.
Muhammad, L., Mwabu, D., Mulwa, R., Mwangi, W., Langyintuo, A., and La Rovere, R.: Characterization of Maize Producing Households in Machakos and Makueni Districts in Kenya, 32 pp., https://repository.cimmyt.org/handle/10883/1092 (last access: May 2021), 2010.
Müller, B., Bohn, F., Dreßler, G., Groeneveld, J., Klassert, C., Martin, R., Schlüter, M., Schulze, J., Weise, H., and Schwarz, N.: Describing human decisions in agent-based models – ODD+D, an extension of the ODD protocol, Environ. Model. Softw., 48, 37–48, https://doi.org/10.1016/j.envsoft.2013.06.003, 2013.
Muriu-Ng'ang'a, F. W., Mucheru-Muna, M., Waswa, F., and Mairura, F. S.: Socio-economic factors influencing utilisation of rain water harvesting and saving technologies in Tharaka South, Eastern Kenya, Agr. Water Manage., 194, 150–159, https://doi.org/10.1016/j.agwat.2017.09.005, 2017.
Mutunga, E., Ndungu, C., and Muendo, P.: Smallholder Farmers Perceptions and Adaptations to Climate Change and Variability in Kitui County, Kenya, J. Earth Sci. Clim. Change, 8, 389, https://doi.org/10.4172/2157-7617.1000389, 2017.
Muyanga, M.: Insights to rural household food insecurity in Kenya, Uganda, J. Agric. Sci., 9, 791–796, 2004.
Muyanga, M. and Jayne, T. S.: Agricultural Extension in Kenya: Practice and Policy Lessons, Policy, Nairobi, https://ageconsearch.umn.edu/record/55168/?ln=en (last access: May 2021), 2006.
National Drought Management Authority: Hazard Atlas, 41 pp., https://www.rcmrd.org/hazard-atlas-development (last access: May 2021), 2016.
Ngetich, K. F., Raes, D., Shisanya, C. A., Mugwe, J., Mucheru-Muna, M., Mugendi, D. N., and Diels, J.: Calibration and validation of AquaCrop model for maize in sub-humid and semi-arid regions of central highlands of Kenya, in: Third RUFORUM Bienn. Meet., 1525–1548, https://repository.ruforum.org/sites/default/files/Ngetich, K.F. et al..pdf (last access: May 2021), 2012.
Ngigi, S. N.: Technical evaluation and development of low-head drip irrigation systems in Kenya, Irrig. Drain., 57, 450–462, https://doi.org/10.1002/ird.360, 2008.
Ngigi, S. N., Thome, J. N., Waweru, D. W., and Blank, H. G.: Low-cost Irrigation for Poverty Reduction: an Evaluation of Low-head Drip Irrigation technologies in Kenya, Int. Water Manag. Inst., 23–29, 2000.
Niang, I., Ruppel, O. C., Abdrabo, M. A., Essel, A., Lennard, C., Padgham, J., and Urquhart, P.: Africa, in: Contribution of Working Group II to the Fifth Assessment Report of the Intergovernmental Panel on Climate Change Chapter 22, edited by: Barros, V. R., Field, C. B., Dokken, D. J., Mastrandrea, M. D., Mach, K. J., Bilir, T. E., Chatterjee, M., Ebi, K. L., Estrada, Y. O., Genova, R. C., Girma, B., Kissel, E. S., Levy, A. N., MacCracken, S., Mastrandrea, P. R., and White, L. L., Cambridge University Press, Cambridge, UK and New York, NY, USA, 1199–1266, https://doi.org/10.1017/CBO9781107415386.002, 2015.
Nyandiko, N. O., Wakhungu, J., and Oteng'i, S. B.: Analysis of maize yield responses to climate in the arid and semi arid lands of lower eastern Kenya, http://ir-library.mmust.ac.ke:8080/xmlui/handle/190/57 (last access: May 2021), 2014.
Nyariki, D. M. and Wiggins, S.: Household food insecurity in sub‐Saharan Africa: lessons from Kenya, Br. Food J., 99, 249–262, https://doi.org/10.1108/00070709710179363, 1997.
O'Brien, K., Eriksen, S., Nygaard, L. P., and Schjolden, A.: Why different interpretations of vulnerability matter in climate change discourses, Clim. Policy, 7, 73–88, https://doi.org/10.1080/14693062.2007.9685639, 2007.
Okumu, O. F.: Small-scale farmers' perception and adaptation measures to cliamte change in Kitui county, Kenya, University of Nairobi, Nairobi, http://erepository.uonbi.ac.ke/handle/11295/59852 (last access: May 2021), 2013.
Oluoko-Odingo, A. A.: Vulnerability and adaptation to food insecurity and poverty in Kenya, Ann. Assoc. Am. Geogr., 101, 1–20, https://doi.org/10.1080/00045608.2010.532739, 2011.
Omoyo, N. N., Wakhungu, J., and Oteng'i, S.: Effects of climate variability on maize yield in the arid and semi arid lands of lower eastern Kenya, Agric. Food Secur., 4, 8, https://doi.org/10.1186/s40066-015-0028-2, 2015.
Pople, A., Hill, R., Dercon, S., and Brunckhorst, B.: Anticipatory Cash Transfers In Climate Disaster Response About the Centre for Disaster Protection, London, https://doi.org/10.1257/rct.6576-1.1, 2021.
Pouladi, P., Afshar, A., Afshar, M. H., Molajou, A., and Farahmand, H.: Agent-based socio-hydrological modeling for restoration of Urmia Lake: Application of theory of planned behavior, J. Hydrol., 576, 736–748, https://doi.org/10.1016/j.jhydrol.2019.06.080, 2019.
Rao, K. P. C., Ndegwa, W. G., Kizito, K., and Oyoo, A.: Climate variability and change: Farmer perceptions and understanding of intra-seasonal variability in rainfall and associated risk in semi-arid Kenya, Cambridge University Press, https://doi.org/10.1017/S0014479710000918, 2011.
Recha, J., Kinyangi, J., and Omondi, H.: Climate Related Risks and Opportunities for Agricultural Adaptation in Semi-Arid Eastern Kenya, CGSpace A Repository of Agricultural Research Outputs, Wageningen, 1–40, https://doi.org/10.13140/RG.2.1.2172.8728, 2015.
Republic of Kenya: Ending Drought Emergencies Common Programme Framework, Nairobi, https://www.ndma.go.ke/index.php/resource-center/category/43-ending-drought-emergencies (last access: May 2021), 2015.
Rezaei, A., Salmani, M., Razaghi, F., and Keshavarz, M.: An empirical analysis of effective factors on farmers adaptation behavior in water scarcity conditions in rural communities, Int. Soil Water Conserv. Res., 5, 265–272, https://doi.org/10.1016/j.iswcr.2017.08.002, 2017.
Rogers, E. M.: Diffusion of Innovations (1983), University of Illinois at Urbana-Champaign's Academy for Entrepreneurial Leadership Historical Research Reference in Entrepreneurship, https://papers.ssrn.com/sol3/papers.cfm?abstract_id=1496176 (last access: May 2021), 1983.
Rudari, R., Abbashar, A., Conijn, S., De Angeli, S., de Moel, H., Denis-Loupot, A., Ferraris, L., Ghizzoni, T., Gomes, I., Mosquera Calle, D., Mouakkid Soltesova, K., Massabò, M., Njoroge Kabubi, J., Rossi, L., Rossi, L., Schiano Lomoriello, R., Trasforini, E., and Wens, M.: Disaster Risk profile for Kenya, https://www.undrr.org/publication/disaster-risk-profile-kenya#:~:text=This Kenya country risk profile,their likelihood, and associated impacts (last access: May 2021), 2019.
Rutten, M. M. M. E. M.: Shallow wells: a sustainable and inexpensive alternative to boreholes in Kenya, ASC working paper, Leiden, 30 pp., http://hdl.handle.net/1887/9460 (last access: May 2021), 2005.
Schrieks, T., Botzen, W. J. W., Wens, M., Haer, T., and Aerts, J. C. J. H.: Integrating Behavioral Theories in Agent-Based Models for Agricultural Drought Risk Assessments, Front. Water, 3, https://doi.org/10.3389/frwa.2021.686329, 2021.
Sherwood, A.: Community adaptation to climate change: exploring drought and poverty traps in Gituamba location, Kenya, J. Nat. Resour. Policy Res., 5, 147–161, https://doi.org/10.1080/19390459.2013.811857, 2013.
Shikuku, K. M., Winowiecki, L., Twyman, J., Eitzinger, A., Perez, J. G., Mwongera, C., and Läderach, P.: Smallholder farmers' attitudes and determinants of adaptation to climate risks in East Africa, Clim. Risk Manage., 16, 234–245, https://doi.org/10.1016/j.crm.2017.03.001, 2017.
Singh, P. K. and Chudasama, H.: Pathways for drought resilient livelihoods based on people's perception, Climatic Change, 140, 179–193, https://doi.org/10.1007/s10584-016-1817-8, 2017.
Sivapalan, M., Savenije, H. H. G. G., and Blöschl, G.: Socio-hydrology: A new science of people and water, Hydrol. Process., 26, 1270–1276, https://doi.org/10.1002/hyp.8426, 2012.
Smajgl, A. and Barreteau, O.: Framing options for characterising and parameterising human agents in empirical ABM, Environ. Model. Softw., 93, 29–41, https://doi.org/10.1016/j.envsoft.2017.02.011, 2017.
Smajgl, A., Brown, D. G., Valbuena, D., and Huigen, M. G. A.: Empirical characterisation of agent behaviours in socio-ecological systems, Environ. Model. Softw., 26, 837–844, https://doi.org/10.1016/j.envsoft.2011.02.011, 2011.
Stefanović, J., Yang, H., and Zhou, Y.: Smallholder Farming Systems in Kenya: Climate Change Perception, Adaptation and Determinants, https://www.syngentafoundation.org/file/1541/download?token=fV7f7_ob (last access: May 2021), 2015.
Taberna, A., Filatova, T., Roy, D., and Noll, B.: Tracing resilience, social dynamics and behavioral change: a review of agent-based flood risk models, Socio-Environ. Syst. Model., 2, 17938, https://doi.org/10.18174/sesmo.2020a17938, 2020.
TAPRA – Tegemeo Institute: Tegemeo Agricultural Policy Research Analysis: Project – Household survey 2004, 1–14, https://www.tegemeo.org/images/_tegemeo_institute/downloads/data/2004-survey-documentation.pdf (last access: January 2021), 2004.
TAPRA – Tegemeo Institute: Tegemeo Agricultural Policy Research Analysis: Project – Household survey 2007, 1–14, https://www.tegemeo.org/images/_tegemeo_institute/downloads/data/2007_survey_doc_final.pdf (last access: January 2021), 2007.
TAPRA – Tegemeo Institute: Tegemeo Agricultural Policy Research Analysis: Project – Household survey 2010, 1–14, https://www.tegemeo.org/images/_tegemeo_institute/downloads/data/2010_surveydocumentation.pdf (last access: January 2021), 2010.
Tongruksawattana, S.: Climate shocks and choice of adaptation strategy for Kenyan maize-legume farmers: Insights from poverty, food security and gender perspectives, Socioeconomics Program Working Paper, Mexico, DF, 1–34, https://ccafs.cgiar.org/resources/publications/climate-shocks-and-choice-adaptation-strategy-kenyan-maize- (last access: May 2021), 2014.
Tongruksawattana, S. and Wainaina, P.: Climate shock adaptation for Kenyan maize-legume farmers: choice, complementarities and substitutions between strategies, Clim. Dev., 11, 710–722, https://doi.org/10.1080/17565529.2018.1562862, 2019.
UNDP: Climate Risks, Vulnerability and Governance in Kenya: A review, 83 pp., https://www.iisd.org/publications/report/climate-risks-vulnerability-and-governance-kenya-review (last acces: May 2021), 2012.
UNDP, UNCCD, and UNEP: Climate Change in the African Drylands : options and opportunities for adaptation and mitigation, Nairobi, https://portals.iucn.org/library/node/29098 (last access: May 2021), 2009.
UNDRR: GAR – Special Report on Drought 2021, UNDRR, Geneva, https://www.undrr.org/publication/gar-special-report-drought-2021#:~:text=The GAR Special Report on,and cosystems health and wellbeing, last access: May 2021.
van Duinen, R., Filatova, T., Geurts, P., and van der Veen, A.: Coping with drought risk: empirical analysis of farmers' drought adaptation in the south-west Netherlands, Reg. Environ. Change, 15, 1081–1093, https://doi.org/10.1007/s10113-014-0692-y, 2015a.
van Duinen, R., Filatova, T., Geurts, P., and van der Veen, A.: Empirical Analysis of Farmers' Drought Risk Perception: Objective Factors, Personal Circumstances, and Social Influence, Risk Anal., 35, 741–755, https://doi.org/10.1111/risa.12299, 2015b.
van Duinen, R., Filatova, T., Jager, W., and van der Veen, A.: Going beyond perfect rationality: drought risk, economic choices and the influence of social networks, Ann. Reg. Sci., 57, 335–369, https://doi.org/10.1007/s00168-015-0699-4, 2016.
Van Eeuwijk, M.: How accurate is the Famine Early Warning Systems Network? A Kenyan and Ugandan case study An accuracy assessment of the Famine Early Warning System Network, Vrije Universiteit Amsterdam, Amsterdam, https://www.gfdrr.org/sites/default/files/FinalReport_F4S_ChallengeFund.pdf, last access: May 2021.
Van Oel, P. R. and Van Der Veen, A.: Using agent-based modeling to depict basin closure in the Naivasha basin, Kenya: A framework of analysis, Procedia Environ. Sci., 7, 32–37, https://doi.org/10.1016/j.proenv.2011.07.007, 2011.
Van Winsen, F., de Mey, Y., Lauwers, L., Van Passel, S., Vancauteren, M., and Wauters, E.: Determinants of risk behaviour: effects of perceived risks and risk attitude on farmer's adoption of risk management strategies, J. Risk Res., 19, 56–78, https://doi.org/10.1080/13669877.2014.940597, 2016.
Viglione, A., Di Baldassarre, G., Brandimarte, L., Kuil, L., Carr, G., Salinas, J. L., Scolobig, A., and Blöschl, G.: Insights from socio-hydrology modelling on dealing with flood risk – Roles of collective memory, risk-taking attitude and trust, J. Hydrol., 518, 71–82, https://doi.org/10.1016/j.jhydrol.2014.01.018, 2014.
Villanueva, A. B., Jha, Y., Ogwal-omara, R., Welch, E., Wedajoo, S., and Halewood, M.: Influence of social networks on the adoption of climate smart technologies in East Africa Findings from two surveys and participatory exercises with farmers and local experts, CCAFS Info Note, https://cgspace.cgiar.org/handle/10568/71146 (last access: May 2021), 2016.
Von Neumann, J. and Morgenstern, O.: Theory of games and economic behavior, Princeton University Press, https://psycnet.apa.org/record/1945-00500-000 (last access: May 2021), 1944.
Waldman, K. B., Todd, P. M., Omar, S., Blekking, J. P., Giroux, S. A., Attari, S. Z., Baylis, K., and Evans, T. P.: Agricultural decision making and climate uncertainty in developing countries, Environ. Res. Lett., 15, 113004, https://doi.org/10.1088/1748-9326/abb909, 2020.
Wamari, J., Isaya, S., Kheng, L., Miriti, J., and Obutiati, E.: Use of Aquacrop Model to Predict Maize Yields under varying Rainfall and Temperature in a Semi-Arid Environment in Kenya, J. Meteorol. Relat. Sci., 6, 26–35, https://doi.org/10.20987/jmrs.08.2012.603, 2007.
Wens, M.: Survey report Kitui, Kenya: Expert evaluation of model setup and preparations of future fieldwork, Vrije Universiteit Amsterdam [data set], https://research.vu.nl/en/publications/survey-report-kitui-kenya-expert-evaluation-of-model-setup- (last access: February 2021), 2018.
Wens, M.: Survey report Kitui, Kenya: Results of a questionaire regardings usbsistence farmers' drought risk and adaptation behaviour, Vrije Universiteit Amsterdam [data set], https://research.vu.nl/en/publications/survey-report-kitui-kenya-results-of-a-questionaire-regardings-us (last access: February 2021), 2019.
Wens, M., Johnson, M. J., Zagaria, C., and Veldkamp, T. I. E.: Integrating human behavior dynamics into drought risk assessment – A sociohydrologic, agent-based approach, WIREs Water, 6, e1345, https://doi.org/10.1002/wat2.1345, 2019.
Wens, M., Veldkamp, T. I. E., Mwangi, M., Johnson, J. M., Lasage, R., Haer, T., and Aerts, J. C. J. H.: Simulating Small-Scale Agricultural Adaptation Decisions in Response to Drought Risk: An Empirical Agent-Based Model for Semi-Arid Kenya, Front. Water, 2, 1–21, https://doi.org/10.3389/frwa.2020.00015, 2020.
Wens, M. L. K., Mwangi, M. N., van Loon, A. F., and Aerts, J. C. J. H.: Complexities of drought adaptive behaviour: Linking theory to data on smallholder farmer adaptation decisions, Int. J. Disast. Risk Reduct., 63, 102435, https://doi.org/10.1016/j.ijdrr.2021.102435, 2021.
Wheeler, S., Zuo, A., and Bjornlund, H.: Farmers' climate change beliefs and adaptation strategies for a water scarce future in Australia, Global Environ. Change, 23, 537–547, https://doi.org/10.1016/j.gloenvcha.2012.11.008, 2013.
Wossen, T. and Berger, T.: Climate variability, food security and poverty: Agent-based assessment of policy options for farm households in Northern Ghana, Environ. Sci. Policy, 47, 95–107, https://doi.org/10.1016/j.envsci.2014.11.009, 2015.
Wossen, T., Berger, T., Mequaninte, T., and Alamirew, B.: Social network effects on the adoption of sustainable natural resource management practices in Ethiopia, Int. J. Sustain. Dev. World Ecol., 20, 477–483, https://doi.org/10.1080/13504509.2013.856048, 2013.
Zagaria, C., Schulp, C. J. E., Zavalloni, M., Viaggi, D., and Verburg, P. H.: Modelling transformational adaptation to climate change among crop farming systems in Romagna, Italy, Agric. Syst., 188, 103024, https://doi.org/10.1016/j.agsy.2020.103024, 2021.
Zhang, B., Fu, Z., Wang, J., and Zhang, L.: Farmers' adoption of water-saving irrigation technology alleviates water scarcity in metropolis suburbs: A case study of Beijing, China, Agr. Water Manage., 212, 349–357, https://doi.org/10.1016/j.agwat.2018.09.021, 2019.
- Abstract
- Introduction
- Case study description
- Model and scenario description
- Results
- Discussion
- Conclusion
- Appendix A: Description of the ADOPT model following the ODD + D protocol (Laatabi et al., 2018; Müller et al., 2013)
- Appendix B: Adoption rates of adaptation measures
- Data availability
- Author contributions
- Competing interests
- Disclaimer
- Special issue statement
- Acknowledgements
- Financial support
- Review statement
- References
- Abstract
- Introduction
- Case study description
- Model and scenario description
- Results
- Discussion
- Conclusion
- Appendix A: Description of the ADOPT model following the ODD + D protocol (Laatabi et al., 2018; Müller et al., 2013)
- Appendix B: Adoption rates of adaptation measures
- Data availability
- Author contributions
- Competing interests
- Disclaimer
- Special issue statement
- Acknowledgements
- Financial support
- Review statement
- References