the Creative Commons Attribution 4.0 License.
the Creative Commons Attribution 4.0 License.
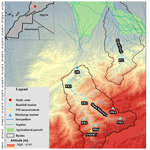
Challenges in flood modeling over data-scarce regions: how to exploit globally available soil moisture products to estimate antecedent soil wetness conditions in Morocco
El Mahdi El Khalki
Christian Massari
Luca Brocca
Vincent Simonneaux
Simon Gascoin
Mohamed El Mehdi Saidi
The Mediterranean region is characterized by intense rainfall events giving rise to devastating floods. In Maghreb countries such as Morocco, there is a strong need for forecasting systems to reduce the impacts of floods. The development of such a system in the case of ungauged catchments is complicated, but remote-sensing products could overcome the lack of in situ measurements. The soil moisture content can strongly modulate the magnitude of flood events and consequently is a crucial parameter to take into account for flood modeling. In this study, different soil moisture products (European Space Agency Climate Change Initiative, ESA-CCI; Soil Moisture and Ocean Salinity, SMOS; Soil Moisture and Ocean Salinity by the Institut National de la Recherche Agronomique and Centre d'Etudes Spatiales de la Biosphère, SMOS-IC; Advanced Scatterometer, ASCAT; and ERA5 reanalysis) are compared to in situ measurements and one continuous soil-moisture-accounting (SMA) model for basins located in the High Atlas Mountains, upstream of the city of Marrakech. The results show that the SMOS-IC satellite product and the ERA5 reanalysis are best correlated with observed soil moisture and with the SMA model outputs. The different soil moisture datasets were also compared to estimate the initial soil moisture condition for an event-based hydrological model based on the Soil Conservation Service curve number (SCS-CN). The ASCAT, SMOS-IC, and ERA5 products performed equally well in validation to simulate floods, outperforming daily in situ soil moisture measurements that may not be representative of the whole catchment soil moisture conditions. The results also indicated that the daily time step may not fully represent the saturation state before a flood event due to the rapid decay of soil moisture after rainfall in these semiarid environments. Indeed, at the hourly time step, ERA5 and in situ measurements were found to better represent the initial soil moisture conditions of the SCS-CN model by comparison with the daily time step. The results of this work could be used to implement efficient flood modeling and forecasting systems in semiarid regions where soil moisture measurements are lacking.
- Article
(9935 KB) - Full-text XML
-
Supplement
(169 KB) - BibTeX
- EndNote
The Mediterranean region is characterized by intense rainfall events generating floods with a very short response time (Gaume et al., 2004; Merheb et al., 2016; Tramblay et al., 2011). The socioeconomic consequences of these floods are very important in terms of fatalities or damages to the infrastructures in particular for southern countries (Vinet et al., 2016). This highlights the need for forecasting systems to reduce the impacts of floods. Unfortunately, the development of such systems is very complicated in the case of ungauged catchments (Creutin and Borga, 2003) such as in North Africa and requires remote-sensing products to overcome the lack of in situ measurements. Furthermore, while several studies have been focused on northern Mediterranean catchments for flood modeling, only a few studies are available for southern basins, yet those are probably the most vulnerable to floods.
The Moroccan catchments are exposed to intense flash floods, such as the event of 17 August 1995 in the Ourika river, where the max discharge reached a peak discharge of 1030 m3 s−1 in 45 min, causing extensive damages and more than 200 casualties (Saidi et al., 2003). Few studies have been carried out in Morocco to minimize the impact of floods by improving the forecasting systems, either by event-based modeling of floods (El Alaoui El Fels et al., 2017; Boumenni et al., 2017; El Khalki et al., 2018) or by hydrogeomorphological approaches (Bennani et al., 2019) to identify the areas at risk of flooding. The severity of floods in these semiarid regions is controlled by several factors including precipitation intensity, soil permeability, steep slopes, and soil moisture content at the beginning of an event (El Khalki et al., 2018; Tramblay et al., 2012). In Mediterranean regions, the soil moisture content varies between events and is known to strongly modulate the magnitude of floods (Brocca et al., 2017; Tuttle and Salvucci, 2014) and particularly to be useful for flood modeling and forecasting systems (Brocca et al., 2011; El Khalki et al., 2018; Koster et al., 2009; Marchandise and Viel, 2010; Tramblay et al., 2012). However, studies in North African basins are lacking to document the rainfall runoff relationship with soil moisture during floods (Merheb et al., 2016).
In most Mediterranean regions and particularly in North Africa, only a few measurements of soil moisture are available. To represent spatial variability, several measurements at different locations are needed due to the potentially large spatial variability of soil moisture for a wide range of scales (Massari et al., 2014; Schulte et al., 2005; Western and Blöschl, 1999). However, even the in situ data may not represent the spatial variability over a very wide area in the case of large basins. In contrast, satellite soil moisture products provide coverage of the earth's surface by microwave sensors. There are two types of microwave sensors – active and passive: (1) the Advanced Scatterometer (ASCAT) soil moisture product is on board MetOp with good radiometric accuracy and stability. This product provides a spatial resolution of 25 km with a temporal resolution of 1 d since January 2007 (Wagner et al., 2013). (2) The Soil Moisture and Ocean Salinity (SMOS) mission product begins in January 2010 with a spatial resolution of 50 km (Kerr et al., 2012). The improvement of the robustness of satellite soil moisture products can be achieved by merging passive and active microwave sensors as initiated and distributed by ESA-CCI (European Space Agency Climate Change Initiative; Liu et al., 2011), providing data from 1978 to 2018. However, remote-sensing products might suffer from several problems in complex topography or very dense vegetation and snow cover (Brocca et al., 2017). For this reason and before any use of the data, it is necessary to validate them (Al-Yaari et al., 2014; Van doninck et al., 2012; Ochsner et al., 2013) either by in situ measurements, if they exist, or by using soil-moisture-accounting (SMA) models (Javelle et al., 2010; Tramblay et al., 2012) to simulate soil moisture in the ungauged basins.
In this context, with an increasing number of satellite products becoming available to estimate soil moisture, clear guidelines and recommendations about the most suitable products to estimate the initial soil moisture content prior to floods are lacking for the semiarid basins of North Africa. There is a knowledge gap in the evaluation of soil moisture products in North Africa (Jiang and Wang, 2019) that the present study aims to fill. The purpose of this study is to compare different satellite soil moisture products with in situ soil moisture measurements and the recently developed ERA5 reanalysis to estimate the initial soil moisture before flood events. The goal is to identify the best products to be used for flood modeling that could improve forecasting systems. This comparison is performed for two basins representative of medium-sized catchments of North Africa that are the most sensitive to flash flood events. The validation of the different soil moisture products is made with a SMA model to test the capabilities of the different soil moisture products for the sake of estimating the initial conditions for an event-based hydrological model for floods. The paper is organized as follows: in Sect. 2, an overview of the study area and all used data (hydrometeorological and soil moisture products) is given. Section 3 explains the methods adopted in this paper. Section 4 presents the results. The conclusion and perspectives are given in the last section.
2.1 Rheraya and Issyl catchments
The Rheraya research catchment (Jarlan et al., 2015) is located in the Moroccan High Atlas Mountains (Fig. 1), with an altitude ranging from 1027 to 4167 m and an area of 225 km2. The climate in the basin is semiarid and strongly influenced by altitude, with a mean annual precipitation of 732 mm, including 30 % as snow in altitudes above 2000 m (Boudhar et al., 2009). The geology is characterized by volcanic formations that are considered impermeable in the highest elevation areas, while the lowest elevation areas are made of granites with clays and marls. In the highest elevation areas, very steep slopes are found with an average of 19 % (Chaponnière et al., 2008). The vegetation cover is only located in the lowest areas, with a concentration of cultivated areas found along the river channel. These natural conditions favor runoff generation. There is very low human disturbance for runoff, with only some local water uptake in the lower part of the river.
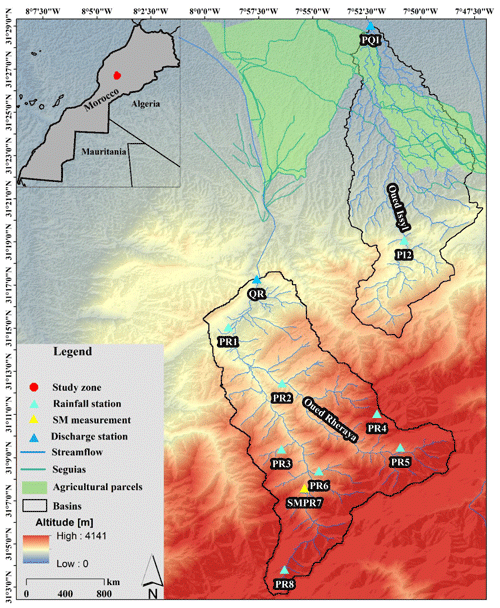
Figure 1Location of Rheraya and Issyl basins, the seguia network, the agricultural parcels, and the hydrometeorological network – PR: rainfall station in Rheraya; SMPR: soil moisture measurement + rainfall station in Rheraya; PQI: rainfall and discharge station in Issyl; QR: discharge station in Rheraya.
The Issyl basin (Fig. 1) is located in the foothills of the Moroccan High Atlas Mountains, with an altitude ranging from 632 to 2300 m, an area of 160 km2, and a mean annual precipitation of 666 mm. It is an ephemeral river with discharge occurring only after rainfall events. The climate is semiarid to arid, and the downstream part of the basin reaches the city of Marrakech. The geological formations in this downstream are alluvial conglomerates that are relatively permeable. The upstream of the basin consists of clays and calcareous marl. The basin area includes agricultural activities that are irrigated in the downstream part of the basin. The irrigation comes from seguias, earthen-made channels that traditionally draw their water supply from the river itself by building small diverting dams on the side of the river (Pérennès, 1994). The seguias channels are usually filled up during floods, and water is distributed to the neighboring agricultural parcels. A map of the seguias in the Issyl basin can be seen in Fig. 1, covering the northern part of the basin. The system is unmonitored, and in the context of high evaporation rates, the portion of runoff diverted from the stream is not quantified. Due to the temporary nature of seguias, they can be partially destroyed during large floods, and consequently their hydraulic properties and the amount of water collected can be modified over time. In the Ourika catchment, located upstream of the Issyl, Bouimouass et al. (2020) estimated that irrigation by streamflow diversion due to seguias could represent up to 65 % of the total surface runoff.
2.2 Hydrometeorological data
In the Rheraya basin, we used eight rainfall stations (Table 1), five of them from the data network of the Joint International Laboratory Télédétection et Ressources en Eau en Méditerranée semi-Aride “LMI TREMA” (Jarlan et al., 2015; Khabba et al., 2013) and the remaining ones from the Tensift Hydraulic Basin Agency. The data cover the period 2008 to 2016. For the Issyl basin, only two rainfall gauges are available from the Tensift Hydraulic Basin Agency, covering the years from 2010 to 2015. In this type of basin, the spatial variability of rainfall is very significant (Chaponnière et al., 2008). The hydrometric data were provided by a radar sensor installed in each basin's outlet. The data cover only the year 2014 for Rheraya since the sensor was installed at the end of 2013 and the years 2010 to 2015 for Issyl. The discharge data are provided with a time step of 10 min converted into an hourly time step as for rainfall.
Table 1, in front of the ligne containing Tahnaout, add ”Precipitation and discharge” (similarly to the ligne right below)
The precipitation data are missing for some events, especially for high-altitude gauges during snowfall events. The percentage of missing values ranges from 2.4 % at PR5 to 10.85 % at PR7. The highest percentage of missing data is 19.7 % at PR1 where the gauge underwent technical problems. Overall, the total percentage of missing values (7.8 %) is low; hence no gap filling method is used. The discharge data are missing in some events that are not selected. For this reason we considered only the events with complete discharge data. Some of the flood events considered in this study (Table 2) occurred in the winter season, when rainfall can be in the form of snow above 2000 m elevation. According to El Khalki et al. (2018), the snow does not contribute to runoff during the winter season in the Rheraya basin because it does not melt during the coldest months (Hajhouji et al., 2018), when only 17 % of the basin area is occupied by snow. The runoff coefficient is calculated by relating the amount of direct runoff to the amount of precipitation for each selected event. It is larger when the basin has low infiltration and lower for permeable basins. In our case, the runoff coefficient ranges from 13.1 % to 34.1 % for Rheraya and from 1.2 % to 7.2 % for Issyl. This indicates the important role of initial conditions in both basins, with a much higher infiltration capacity in the Issyl basin in addition to potential water loss due to irrigation. We used five temperature stations located in the Rheraya basin and one temperature station located in the Issyl basin with an hourly time step to calculate the average temperature over each basin, ranging from 2008 to 2016. These data enabled us to calculate potential evapotranspiration (PET) with the Oudin formula (Oudin et al., 2005), requiring temperature only. This formula was previously applied in Morocco (Marchane et al., 2017; Tramblay et al., 2013) and in Tunisia (Dakhlaoui et al., 2020).
2.3 Soil moisture data
We used seven different types of soil moisture data over the Rheraya basin and six types in the Issyl basin due to the absence of measurements in this basin. Covering the same period of rainfall data mentioned in Sect. 2.2, we used
- 1.
in situ measurement with three ThetaProbes at 5 and 30 cm depth in the Rheraya basin, located at the SMPR7 station (Fig. 1)
- 2.
simulated soil moisture from a soil-moisture-accounting (SMA) model
- 3.
ASCAT satellite soil moisture
- 4.
SMOS satellite soil moisture
- 5.
SMOS-IC satellite soil moisture
- 6.
ESA-CCI satellite soil moisture
- 7.
ERA5 reanalysis soil moisture
2.3.1 In situ measurements
Soil moisture measurements are available at one location with three ThetaProbes at two different depths (5 and 30 cm). In this study we used ThetaProbes with 5 cm depth, which is comparable with the depths of satellite products (Massari et al., 2014). The site is located in Rheraya basin, with an altitude of 2030 m and a slope of 30 % (Fig. 1). The data cover the time period from 2013 to 2016, with a 30 min time step converted to a daily time step.
2.3.2 Soil-moisture-accounting model
The SMA model is a continuous soil-moisture-accounting model that can be used in the absence of soil moisture data to represent the degree of saturation for flood modeling (Anctil et al., 2004; Tramblay et al., 2012). In this study, a simplified version of the SMA model is used, adopting the same approach used by Tramblay et al. (2012) and Javelle et al. (2010). The SMA model calculates the level of the soil reservoir (S∕A), ranging between 0 and 1, by calibrating its single parameter, A, which represents the maximum reservoir capacity of the soil. An interpolated daily rainfall dataset created by the inverse distance method and evapotranspiration data computed from daily temperature with the Oudin equation (Oudin et al., 2005) are used as inputs to the SMA model.
2.3.3 Soil moisture products
In this study we used three different types of satellite products and a reanalysis product (Table 3):
- 1.
The Advanced Scatterometer (ASCAT) is a soil moisture product onboard a MetOp-A, MetOp-B, and MetOp-C satellite. It is a C-band (5.255 GHz) scatterometer onboard the MetOp satellite series. It has a spatial sampling of 12.5 km and one to two observations per day (Wagner et al., 2013). The soil moisture product was provided within the EUMETSAT project (http://hsaf.meteoam.it/, last access: 23 September 2020), denoted as H115.
- 2.
The Soil Moisture and Ocean Salinity (SMOS) mission is a radiometer operating at L band (1.4 GHz), providing soil moisture data with ∼50 km spatial sampling and one observation per 2 or 3 d (Kerr et al., 2001). The Centre Aval de Traitement des Données (CATDS; https://www.catds.fr/, last access: 23 September 2020) SMOS provided the version RE04 (level 3) for this study. This version is gridded on the 25 km EASEv2 grid.
- 3.
The Soil Moisture and Ocean Salinity INRA–CESBIO (SMOS-IC) is an algorithm designed by the Insitut National de la Recherche Agronomique (INRA) and Centre d'Etudes Spatiales de la Biosphère (CESBIO) for a global retrieval of soil moisture and L-band vegetation optical depth. Two parameters of inversion of the L-band microwave emission of the biosphere model are used in the SMOS-IC (Wigneron et al., 2007) with a consideration of the pixel as homogeneous. This version is 105 and has a spatial sampling of 25 km with an EASEv2 grid (Fernandez-Moran et al., 2017).
- 4.
The ESA-CCI soil moisture product (https://www.esa-soilmoisture-cci.org/, last access: 23 September 2020) regroups active and passive microwave sensors to measure soil moisture, giving three type of products: active, passive, and combined (active + passive). In this paper, the ESA-CCI V4.5 combined product is used (Dorigo et al., 2017; Gruber et al., 2017, 2019). The product has been validated to be useful by 600 ground-based measurement points around the globe (Dorigo et al., 2015), and it was compared with ERA-Interim products (Albergel et al., 2013). In the field of hydrological modeling, several global studies have used the ESA-CCI product to initiate the hydrological model (Dorigo et al., 2012, 2015; Massari et al., 2014) at the scale of Morocco (El Khalki et al., 2018). We extracted for each basin the pixel that corresponds to it.
- 5.
ERA5 (Copernicus Climate Change Service, C3S, 2017) developed by the European Centre for Medium-Range Weather Forecasts (ECMWF), is the latest version of atmospheric reanalysis available for the public since February 2019. The ERA5 replaced ERA-Interim with improvement at different scales, particularly a higher spatial and temporal resolution and a better global balance of precipitation and evaporation. The spatial resolution is 31 km instead of 79 km, hourly resolution is used instead of 6 h, and the covered period will be extended to 1950 in future. The ERA5 product was applied in some recent studies in the hydroclimatic field (Albergel et al., 2018; Hwang et al., 2019; Mahto and Mishra, 2019; Olauson, 2018). We selected the volumetric soil water of the first soil layer. This new product is tested in our study for the first time in Morocco. An alternative dataset, ERA5-Land using an improved land surface scheme with a spatial resolution of 10 km, was also tested, providing the same results as ERA5 since there is a strong correlation between soil moisture simulated by the two products.
It should be noted that the soil moisture products have a different percentage of missing data over each basin (Table 4). The ESA-CCI product shows a significant percentage of missing values over the Rheraya basin compared to ASCAT that is included in the ESA-CCI product. This is due to the filter used in the ESA-CCI product to ensure the data quality. The difference in the percentage of missing values between Rheraya and Issyl is related to the complex topography and also to the frozen zones in the Rheraya basin: a more detailed description about the applied filters can be found in Dorigo et al. (2017). However, the percentage of missing values for the SMOS product is quite similar between the two basins, which is related to the low temporal resolution (one observation per 2 or 3 d).
3.1 Evaluation of different soil moisture datasets
In situ data preparation consists of averaging the 5 cm depth probes in order to get a single value to work with and take into account the plot-scale variability of the measurements. These data are considered as a reference for soil moisture data in the Rheraya basin so that all the other soil moisture products are compared to it. The different soil moisture products are compared to the observed soil moisture over the entire period and also on a seasonal basis.
The SMA model is used to represent the soil moisture aggregated at the catchment scale. The rationale behind the use of such a model here is that continuous rainfall and temperature series are often available in monitored catchments, unlike soil moisture, and a calibrated SMA model can sometimes palliate the lack of soil moisture measurements (Tramblay et al., 2012). For the SMA model, the A parameter, representing the soil water holding capacity, is calibrated to obtain the best correlation between observed and simulated soil moisture (S∕A). The calibration with observed data can only be performed in the Rheraya basin where soil moisture is measured. In addition to this calibration, other values of A, ranging from 1 to 1000 mm, are tested in the SMA model to maximize the correlations with the different soil moisture products. The choice of this approach is to check whether there are any possible uncertainties that can be related to the in situ soil moisture measurements, located on a steep slope plot that may not fully represent the average soil moisture conditions over the whole basin. In the case of the Issyl basin, since there is no observed soil moisture data, the model is run for a range of different values of the A parameter. The best value of the A parameter is selected as the one yielding the best correlations with the different satellite products.
The values from ASCAT and SMA are given in percent (values range between 0 and 1), while SMOS, SMOS-IC, ERA5, ESA-CCI, and observations are in cubic meters per cubic meter. To allow a comparison for all soil moisture datasets a rescaling procedure is needed. Before applying the rescaling procedure, according to Albergel et al. (2010), a 95 % confidence interval is chosen to define the higher and lower values to exclude any abnormal outliers using Eqs. (1) and (2). The resulting data are then rescaled to their own maximum and minimum values considering the whole period using Eq. (3). The issue in the validation of satellite soil moisture products and reanalysis product with in situ measurements is the spatial resolution (Jackson et al., 2010). Several studies mentioned that, in the case of the temporal stability introduced by Vachaud et al. (1985), one in situ measurement point can represent the soil moisture condition of a larger area (Brocca et al., 2009b, 2010; Loew and Mauser, 2008; Loew and Schlenz, 2011; Martínez-Fernández and Ceballos, 2005; Miralles et al., 2010; Wagner et al., 2008). According to (Massari et al., 2015), the coarse satellite observations can be beneficial for small basins if the in situ observation falls in the satellite product pixel. This means that the in situ measurements can represent a good benchmark (Liu et al., 2011). In this study we considered the in situ measurement as a benchmark to validate different soil moisture products.
where UpSM and LowSM are the limits of the confidence interval (the upper and the lower 95 %):
The correlation coefficient of Pearson (Eq. 4) and the root mean square deviation (RMSD; Eq. 5) are used to compare in situ measurements and humidity modeled by the SMA model and the different soil moisture products.
where SMInsitu is the in situ measurements of soil moisture or the SMA model, which are considered as references; SMsat is the soil moisture from satellite data or reanalysis; and N is the number of values.
3.2 Event-based hydrological model for floods
In this study, we used the Soil Conservation Service curve number (SCS-CN) model for each basin, implemented in the Hydrologic Engineering Center – Hydrologic Modeling System “HEC-HMS” software (US Army Corps of Engineers, 2015). This model is known by its widespread popularity and the simplicity of the application method (Miliani et al., 2011). SCS-CN is often used in the semiarid context (Brocca et al., 2009a; El Khalki et al., 2018; Tramblay et al., 2010; Zema et al., 2017). Our methodology is based on the use of the SCS-CN model as a production function to compute net rainfall by automatically and manually calibrating the curve number (CN) parameter in order to obtain a realistic hydrograph shape. The value of CN is nondimensional, ranging from 0 (dry) to 100 (wet). The potential maximum retention, S, is related to CN as follows:
The transformation of precipitation excess into runoff is provided by the Clark unit hydrograph model (transfer function). The calibration procedure is based on calibrating the Clark unit hydrograph model parameters: storage coefficient (SC) and time of concentration (TC). The two functions (production and transfer) are calibrated separately to avoid the parameter dependence, and the calibration is based on the Nash–Sutcliffe (NS) criterion.
The validation procedure is based on two steps: first, testing the relationship between soil moisture data (in situ, SMA, ERA5, ASCAT, SMOS, SMOS-IC, and ESA-CCI) at two different timescales (daily and hourly) and the S parameter of the event-based model of all the flood events. The hourly time step concerns only the in situ data and ERA5 by choosing the soil moisture state 1 h before the starting time of rainfall for each event. Only the ERA5 product can be used in the Issyl basin at the hourly time step due to the absence of observed data. Then, the soil moisture products that are well correlated with the S parameter are used to validate the model by calculating the S parameter from the linear equation obtained between soil moisture and S using the leave-one-out resampling procedure; each event is successively removed, and a new relationship between the remaining event is recomputed. The relationship is good when the correlation is near . The negative correlation is related to the fact that the storage capacity (S) is larger when the soil is dry (soil moisture is near 0). The estimated S parameter for a given event is then used in the SCS-CN model in validation. For the Clark unit hydrograph model, the average of the SC and the TC parameters are used in validation in the leave-one-out resampling method; the parameters are recalibrated with the remaining events, and the mean of calibrated values are used in validation.
For the evaluation of the flows simulated by the flood event model, we compared the simulated discharge with those observed using the efficiency coefficient of Nash–Sutcliffe (Nash and Sutcliffe, 1970; Eq. 7) as well as through the bias on peak flow and on volume (Eq. 8).
where Qsim is the simulated discharge, Qobs is the observed discharge, and n is the number of events. The NS ranges between −∞ and 1; the 1 value of NS indicates that the simulated discharge perfectly matches the observed hydrograph.
4.1 Relationship between satellite soil moisture data and in situ measurements
The comparison between measured soil moisture at 5 cm depth and the different products of soil moisture shows that the SMOS-IC and ERA5 provide the best correlations, with r=0.77 and r=0.67, respectively, but it should be noted that all the correlations with the different products are also significant. Figure 2 shows that SMOS-IC and ERA5 reproduce dry periods well, whereas ERA5 reproduces wet periods well. This result is in accordance with the results of Massari et al. (2014), who found that ERA-Land is well correlated with in situ data. The ASCAT product shows a correlation of r=0.45, which is less than the correlation given in Albergel et al. (2010), who found r values ranging from 0.59 to 0.64; the lower correlation may be caused by the orography and the coarse resolution. In fact, this result shows that the use of a combined product as ESA-CCI gives an obvious advantage over one single satellite soil moisture product in terms of r values (Ma et al., 2019; Zeng et al., 2015).
4.2 Relationship between the SMA model outputs and soil moisture products
The best correlation between observed soil moisture and the soil moisture level (S∕A) modeled by the SMA model is obtained for A=8 mm with r=0.86. But it shows higher RMSD than observations (RMSD=0.23), which is due to the overestimation of the wet periods (Fig. 3). This can be related to the averaging of rainfall data in the SMA model over the basin, which could be higher than rainfall in the soil moisture measurement site. It should be noted that the value of the A parameter is very small by comparing to previous studies (Javelle et al., 2010; Tramblay et al., 2012), indicating a much lower soil storage capacity.
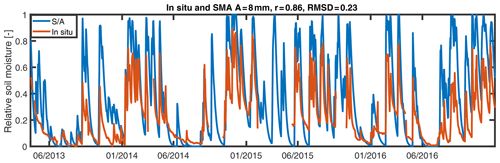
Figure 3Relationship between S∕A and observed soil moisture data between 8 April 2013 and 31 December 2016 for different values of A (Rheraya basin).
We correlated the SMA model output (for A=8 mm) with the satellite products of soil moisture, and the best correlations are found for SMOS-IC and ERA-5, with r=0.74 and r=0.63, respectively (Fig. 4). Other values of A that maximize the correlations with the different soil moisture products have also been tested. Optimal values of A range from 1 mm with ASCAT (with r=0.4) and 8 mm for SMOS (r=0.56), SMOS-IC (r=0.74), and ESA-CCI (r=0.59) up to 16 mm for ERA5 (r=0.68). Comparing Figs. 2 and 4 we notice that the soil moisture products better reproduce in situ measurements than modeled soil moisture with the SMA model, except for ESA-CCI and SMOS. This improvement is directly related to the SMA model performance, which overestimates soil moisture, and should be compared to Fig. 2, where ESA-CCI and SMOS products also overestimate in situ measurements.
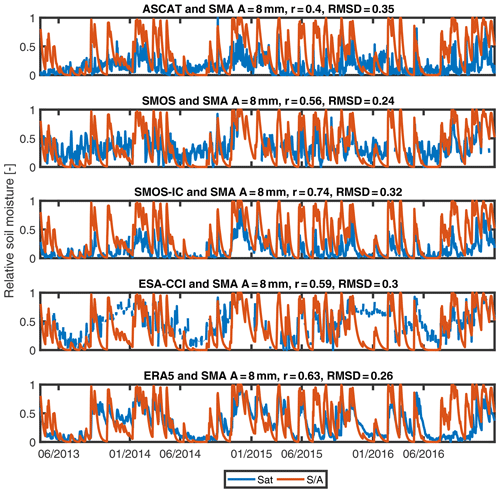
Figure 4Relationship between the different products of soil moisture and SMA model outputs between 8 April 2013 and 31 December 2016 over the Rheraya basin.
For the Issyl basin, as mentioned above, no observed soil moisture data are available to calibrate the A parameter of the SMA model. Therefore, different values of A are tested to correlate the SMA outputs with the different soil moisture datasets. Over all datasets, the value of A best correlated to the majority of soil moisture products is 30 mm. The best correlation is given by A=30 mm with r=0.78, 0.82, and 0.79 for ASCAT, SMOS-IC, and ESA-CCI, respectively. As for SMOS and ERA5, the best correlation is given for A=40 mm with r=0.7 and A=60 mm with r=0.8, respectively. In order to choose a single value of A that represents the basin, we have considered A=30 mm, the optimal value yielding the best correlations with the different soil moisture products. Figure 5 shows that the best correlation between satellite products and S∕A is obtained with SMOS-IC (r=0.82) and ESA-CCI (r=0.79). As observed over the Rheraya basin, the SMOS-IC and ERA5 products showed a good reproduction for dry periods, with a better reproduction of wet periods with ERA5; these results are similar to those of Ma et al. (2019), who found that SMOS-IC performs well in arid zones, with a median r value of 0.6. Overall, the higher value for the A parameter found for this basin is coherent with the fact that this basin is located in a plain area with a much higher soil moisture storage capacity than in the mountainous Rheraya basin.
4.3 Comparison of soil moisture datasets by season
Seasonal evaluation of satellite soil moisture and reanalysis data shows for the Rheraya basin that during the summer season there are low correlations (average r=0.34) for all the products, which is possibly due to very low precipitation amounts mostly as localized convective precipitation (Albergel et al., 2010). In contrast, better performance are obtained with the SMA model (r=0.59) that considers catchment-scale precipitations. Better correlations are obtained in autumn, with an average of r=0.61 and 0.58 for the in situ data and SMA, respectively (Table 5). In the winter we found a poor correlation using SMOS and ESA-CCI that can be related to the significant percentage of missing values. For the Issyl watershed, the satellite products show good correlations with the SMA model outputs (on average r=0.76) except for the SMOS product, especially in winter. The highest mean correlations (i.e., averaged for all the different products) are found during autumn in the Rheraya basin, with r=0.61 with in situ data and r=0.58 with SMA soil moisture. It should be noted that correlations with SMA model outputs in summer are similar, with r=0.59. For the Issyl basin, the correlations are also higher in the autumn, with a mean r=0.87 for the SMA model. The ERA5 product shows good correlations for most seasons. Complementary to this comparison of the different soil moisture products, an extended collocation analysis has also been performed, comforting the results obtained (see the Supplement).
4.4 Calibration of the event-based hydrological model
Calibration results (Table 6) of the individual flood events of Table 2 show that the difference between the values of the potential maximum soil moisture retention (S) of each basin is very significant, with larger values for the Issyl basin, where the soil depth is prominent. We noticed that the temporal variability of soil moisture can be significant between two successive events like the events of 2 and 5 April 2012 for the Issyl basin. The SCS-CN model reproduces the floods of the Rheraya basin well, with an average NS of 0.67 and bias on runoff peak (BIASQ) of 4 % (Table 6). The SCS-CN model in calibration is able to reproduce the shape of the different flood events even for the most complex ones (21 April and 22 November 2014). Similarly, for the Issyl basin the SCS-CN model gives good results, with an average NS of 0.66 and an average bias on runoff peak of 6.93 %. The simulated hydrographs are in good agreement with the observations. The lower NS coefficients obtained for the 23 January 2014 event in the Rheraya basin and for the 3 April 2011 and 28 September 2012 events in the Issyl basin are caused by a slight shift in the hydrograph, probably due to a time lag in instantaneous precipitation measurements. For the Clark unit hydrograph model, the averages of calibrated TC and SC parameters are considered for validation (SC=1.42 and 2.54 h and TC=2.85 and 3.64 h for Rheraya and Issyl, respectively).
Table 6Calibration results of the SCS-CN model: S is the potential maximum soil moisture retention; BIASQ is the difference between the observed and calibrated peak discharge of the event; BIASV is the difference between the observed and calibrated volume of the event.
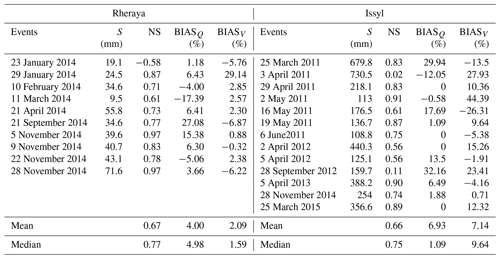
The S parameters of the hydrological models for the two basins are then compared to the soil moisture products. For the Rheraya basin, there are significant correlations of the S parameter with in situ soil moisture data, ERA5, and SMOS-IC (Table 7). The correlations using observed soil moisture, ESA-CCI, and SMOS data can be computed with only eight and six events, respectively, due to the presence of missing values. The time step of the soil moisture data in the Rheraya basin seems to play a key role in the representation of soil moisture conditions. Indeed, the daily time step shows a weakness in effectively representing the antecedent soil moisture conditions in the SCS model, which indicates the rapid change in soil moisture content in such a semiarid mountainous basin. For the Issyl basin, ESA-CCI is the only satellite product that is significantly correlated to the S parameter at the daily time step. The ERA5 product is also significantly correlated with the S parameter but at the hourly time step. The daily output of the SMA model is also able to estimate the initial condition of the model for the Issyl basin, with a correlation of −0.69 with S. Interestingly, the SMA model does not provide a good performance in the Rheraya basin. It can be due to the fact that in such a mountainous basin, there is a strong spatial variability of rainfall, and it is difficult to obtain reliable precipitation estimates for continuous simulations (Chaponnière et al., 2008).
4.5 Validation of the event-based hydrological model
The validation of the event-based hydrological model is performed on the events of Rheraya and Issyl using only the soil moisture datasets that show relatively good correlations with the initial condition (S) of the model from Table 8. These products include SMOS-IC, ERA5, and observed soil moisture for the Rheraya basin and ESA-CCI, ERA5, SMOS, and SMA for Issyl. The validation of the event-based model is performed with S calculated from the linear equation obtained from the correlation analysis between the different soil moisture products and the calibrated parameter S. The validation results show that for the Rheraya basin the events are well validated using both daily (Fig. 6) and hourly (Fig. 7) time steps of soil moisture products. The best validation result at the daily time step is obtained with SMOS-IC, with an average NS of 0.58 for all events (median NS=0.63). This result should be compared with the results found in the previous sections, where SMOS-IC showed the best correlations with observed soil moisture. ASCAT and ERA5 show similar results in terms of average NS (∼0.45). In contrast, the daily observed soil moisture shows a lower performance with an average NS of 0.25 (median NS=0.49). The hourly time step enhanced the performance of the model, with an average NS using the ERA5 product of 0.64 (median NS=0.73), and also a better performance with the hourly in situ data, with mean NS=0.54 (median NS=0.61). These results show that the hourly time step better represents the saturation content before the flood events in this basin. For the Issyl basin, the validation results are quite different (Fig. 8). For only five events (3 April 2011, 2 and 19 May 2011, 5 April 2012, and 25 March 2015) the event-based model can be validated using the ERA5 hourly data with an average NS coefficient of 0.46. For the events of 16 May and 6 June 2011, an significant spatial variability of precipitation is observed, with no precipitation at the PQI station. In addition to these events, the flood of 28 September 2012 showed an overestimation of the validated value of S compared to the calibrated value. This overestimation is related to the ERA5 estimation that considers the soil to be more saturated than it is. For all other events and with different soil moisture products, the NS coefficients are negative and the hydrographs not adequately reproduced. These validation results should be put in perspective with the fact that the Issyl basin has a land use characterized by agricultural activities with possible large water uptake in the diver channel during floods for irrigation. Some simple methods to compensate for the water losses due to irrigation, such as the application of a varying percentage of runoff added to the observed discharge to compensate for the part of water lost for irrigation, have been tested but with no improvement of the results. This is probably because the quantity taken for irrigation is not constant from one event to another, depending on the farmer needs, as shown by field surveys, and this amount may also depend on discharge thresholds.
Table 8Performance of the SCS-CN model in terms of NS coefficients for the Rheraya and Issyl events, using the daily or hourly time steps for the different soil moisture products.
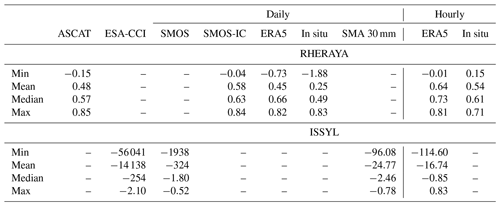
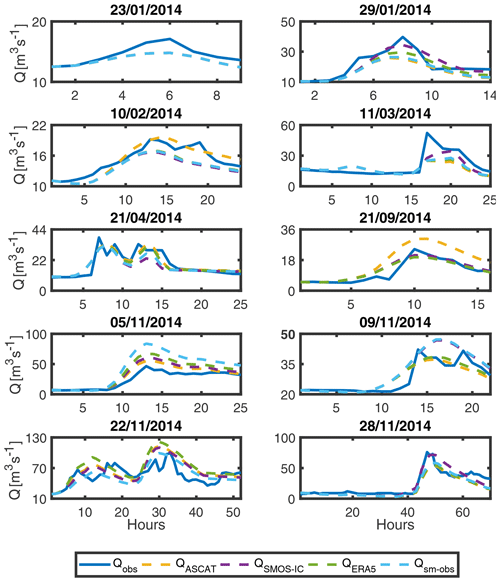
Figure 6Validation results of flood events simulated for the Rheraya using different soil moisture products with a daily time step: the observed hydrographs (Qobs) are compared to the simulated hydrographs using ASCAT (Qascat), SMOS-IC (QSMOS−IC), ERA5 (QERA5), or observed soil moisture (Qsm−obs) to estimate the antecedent soil moisture conditions. The selected flood events are described in Table 2.
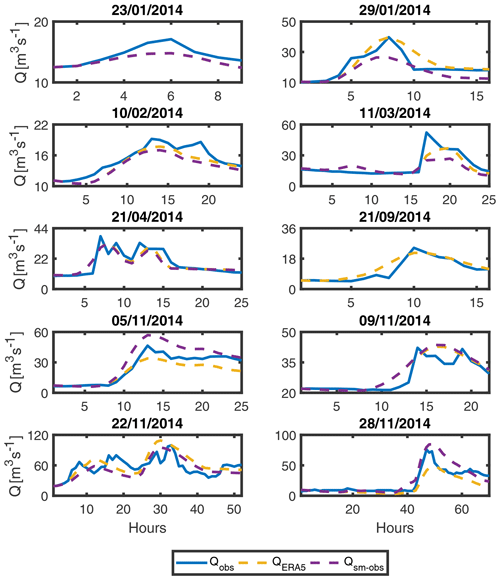
Figure 7Validation results of flood events simulated for the Rheraya using different soil moisture products with an hourly time step: the observed hydrographs (Qobs) are compared to the simulated hydrographs using ERA5 (QERA5) or observed soil moisture (Qsm−obs) to estimate the antecedent soil moisture conditions. The selected flood events are described in Table 2.
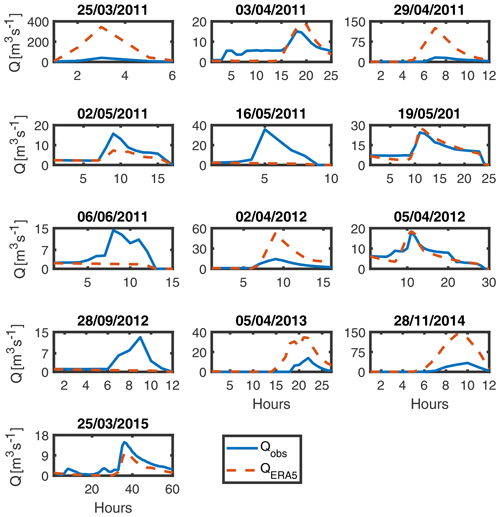
Figure 8Validation results of flood events simulated for the Issyl basin using ERA5 soil moisture at the hourly time step: the observed hydrographs (Qobs) are compared to the simulated hydrographs using ERA5 (QERA5) to estimate the antecedent soil moisture conditions. The selected flood events are described in Table 2.
This study performed an evaluation of different soil moisture products (ASCAT, ESA-CCI, SMOS, SMOS-IC, and ERA5) using in situ measurements and a soil-moisture-accounting (SMA) model over two basins located in the Moroccan High Atlas in order to estimate the initial soil moisture conditions before flood events. The results indicated that the SMOS-IC product is well correlated with both the in situ soil moisture measurements and simulated soil moisture from the SMA model over the two basins. Beside satellite products, the new ERA5 reanalysis also reproduced the in situ measurements over the mountainous basin well, which indicates the robustness of this product to estimate soil moisture in these semiarid environments. The seasonal analysis showed for both basins that the highest correlations are found in autumn, which encourages the use of these remote-sensing products for flood forecasting because the majority of events occur in autumn and early winter in these regions (El Khalki et al., 2018). One of the main findings of the present study is that different products, in particular SMOS-IC, ASCAT, and ERA5, are efficient to estimate the initial soil moisture conditions in an event-based hydrological model, which could improve the forecasting capability in data-scarce environments.
This study also showed that the hourly temporal resolution for soil moisture provides a better estimate of antecedent wetness conditions before flood events. Indeed, the use of hourly soil moisture measurements or ERA5 provided better performance than daily soil moisture to estimate the initial condition of the hydrological model. These results indicate that the temporal variability of soil moisture in these semiarid basins under high evapotranspiration rates can be very significant, causing a quick decay of soil moisture after a rainfall event. For this type of basin, the use of soil moisture products with an hourly temporal resolution could be required to estimate with accuracy the soil moisture content prior to flood events. This constitutes a research challenge to monitor soil moisture at the subdaily timescale without ground measurements since most remote-sensing products at present are not available at the hourly time step. As shown by this study, atmospheric reanalysis coupled with a land surface model, such as ERA5, could provide a valuable alternative, in particular since the resolution of these products is constantly improving along with a more realistic representation of water balance.
For the catchment that is the most influenced by agricultural activities, the Issyl basin located near Marrakech, the water uptake for irrigation made the validation of the hydrological model difficult. The model overestimates runoff for some flood events since the water uptake during floods from the river channel by small artisanal structures is not monitored and thus cannot be represented in the hydrological model. This example shows the difficulty in the implementation of a flood-forecasting system in such basins without good knowledge of the human influences on river discharge. This situation is not a particular case but deemed common in semiarid areas where rivers with a high risk of flooding are also a substantial water resource for agriculture. Therefore, as shown by our results, a hydrological model that does not account for water use and irrigation may not be efficient at reproducing flood events in an operational context. The resolution of this issue would require the development of an irrigation monitoring system that would need intensive field surveys and mapping but also the agreement of the local farmers that benefit from this system.
This study is a first step towards the development of operational flood-forecasting systems in semiarid North Africa basins highly impacted by floods. Indeed, the evaluation of the most suitable satellite or reanalysis products to estimate soil moisture for the monitoring of the basin saturation conditions before floods is a necessary first step prior to implementing flood warning systems based on rainfall and soil moisture thresholds or coupled hydrometeorological modeling (Javelle et al., 2016; Norbiato et al., 2008). Three important aspects that should be addressed in further research aiming at developing a flood-forecasting system are (1) the application of assimilation methods to correct the initial soil moisture condition of the basin and to increase the latency of soil moisture by using the observed discharge before the flood event (Coustau et al., 2013), (2) the joint assimilation of soil moisture and snow cover in order to better predict floods in the mountainous basins (Baba et al., 2018; Koster et al., 2010), and (3) the selection of soil moisture data based on the latency of soil moisture products. For instance, the ERA5 reanalysis is available within 5 d latency, while ASCAT or SMOS satellite products could be available with 3 h latency. With the issue of the latency to obtain some products, it should also be noted that the mismatch of spatial resolution between large-scale remote-sensing products and very local small-scale applications could be an additional issue. Prior to these developments, this type of evaluation should be generalized in Morocco and other sites in North Africa where soil moisture measurements are available for the development of reliable flood-forecasting systems using the outputs of meteorological models.
The data processed in the present work can be requested to the corresponding author.
The supplement related to this article is available online at: https://doi.org/10.5194/nhess-20-2591-2020-supplement.
EMEK performed the analysis and helped write the paper; YT designed the analysis and helped write the paper; CM and LB designed the analysis and contributed to the paper; CM performed the TC analysis; and MEMS, VS, and SG contributed to the paper.
The authors declare that they have no conflict of interest.
This article is part of the special issue “Hydrological cycle in the Mediterranean (ACP/AMT/GMD/HESS/NHESS/OS inter-journal SI)”. It is not associated with a conference.
This research has been conducted in the TREMA International Joint Laboratory (https://www.lmi-trema.ma/, last access: 23 September 2020), funded by the Cadi Ayyad University of Marrakech and the French IRD. This work is a contribution to the Hydrological cycle in the Mediterranean eXperiment (HyMeX) program through INSU-MISTRALS support. Thanks are due to the Tensift Hydrological Basin Agency (ABHT) and to the LMI TREMA for providing the data. The authors would like to thank Khalid Chaouch for his English revision and the associated editor Eric Martin and two anonymous reviewers that helped to improve the manuscript.
This research has been supported by the ERASMUS+ Mobility program and the Centre National de la Recherche Scientifique et Technique (CNRST).
This paper was edited by Eric Martin and reviewed by two anonymous referees.
Albergel, C., Calvet, J.-C., de Rosnay, P., Balsamo, G., Wagner, W., Hasenauer, S., Naeimi, V., Martin, E., Bazile, E., Bouyssel, F., and Mahfouf, J.-F.: Cross-evaluation of modelled and remotely sensed surface soil moisture with in situ data in southwestern France, Hydrol. Earth Syst. Sci., 14, 2177–2191, https://doi.org/10.5194/hess-14-2177-2010, 2010.
Albergel, C., Dorigo, W., Balsamo, G., Muñoz-Sabater, J., de Rosnay, P., Isaksen, L., Brocca, L., de Jeu, R., and Wagner, W.: Monitoring multi-decadal satellite earth observation of soil moisture products through land surface reanalyses, Remote Sens. Environ., 138, 77–89, https://doi.org/10.1016/J.RSE.2013.07.009, 2013.
Albergel, C., Dutra, E., Munier, S., Calvet, J.-C., Munoz-Sabater, J., de Rosnay, P., and Balsamo, G.: ERA-5 and ERA-Interim driven ISBA land surface model simulations: which one performs better?, Hydrol. Earth Syst. Sci., 22, 3515–3532, https://doi.org/10.5194/hess-22-3515-2018, 2018.
Al-Yaari, A., Wigneron, J.-P., Ducharne, A., Kerr, Y. H., Wagner, W., De Lannoy, G., Reichle, R., Al Bitar, A., Dorigo, W., Richaume, P., and Mialon, A.: Global-scale comparison of passive (SMOS) and active (ASCAT) satellite based microwave soil moisture retrievals with soil moisture simulations (MERRA-Land), Remote Sens. Environ., 152, 614–626, https://doi.org/10.1016/J.RSE.2014.07.013, 2014.
Anctil, F., Michel, C., Perrin, C., and Andréassian, V.: A soil moisture index as an auxiliary ANN input for stream flow forecasting, J. Hydrol., 286, 155–167, https://doi.org/10.1016/J.JHYDROL.2003.09.006, 2004.
Baba, M. W., Gascoin, S., and Hanich, L.: Assimilation of Sentinel-2 data into a snowpack model in the High Atlas of Morocco, Remote Sens., 10, 1–23, https://doi.org/10.3390/rs10121982, 2018.
Bennani, O., Druon, E., Leone, F., Tramblay, Y. and Saidi, M. E. M.: A spatial and integrated flood risk diagnosis, Disaster Prev. Manag., 28, 548–564, https://doi.org/10.1108/DPM-12-2018-0379, 2019.
Boudhar, A., Hanich, L., Boulet, G., Duchemin, B., Berjamy, B., and Chehbouni, A.: Evaluation of the Snowmelt Runoff Model in the Moroccan High Atlas Mountains using two snow-cover estimates, Hydrolog. Sci. J., 54, 1094–1113, https://doi.org/10.1623/hysj.54.6.1094, 2009.
Bouimouass, H., Fakir, Y., Tweed, S., and Leblanc, M.: Groundwater recharge sources in semiarid irrigated mountain fronts, Hydrol. Process., 34, 1598–1615, https://doi.org/10.1002/hyp.13685, 2020
Boumenni, H., Bachnou, A., and Alaa, N. E.: The rainfall-runoff model GR4J optimization of parameter by genetic algorithms and Gauss-Newton method: application for the watershed Ourika (High Atlas, Morocco), Arab. J. Geosci., 10, 343, https://doi.org/10.1007/s12517-017-3086-x, 2017.
Brocca, L., Melone, F., Moramarco, T., and Morbidelli, R.: Antecedent wetness conditions based on ERS scatterometer data, J. Hydrol., 364, 73–87, https://doi.org/10.1016/j.jhydrol.2008.10.007, 2009a.
Brocca, L., Melone, F., Moramarco, T., and Morbidelli, R.: Soil moisture temporal stability over experimental areas in Central Italy, Geoderma, 148, 364–374, https://doi.org/10.1016/j.geoderma.2008.11.004, 2009b.
Brocca, L., Melone, F., Moramarco, T., and Morbidelli, R.: Spatial-temporal variability of soil moisture and its estimation across scales, Water Resour. Res., 46, W02516, https://doi.org/10.1029/2009WR008016, 2010.
Brocca, L., Hasenauer, S., Lacava, T., Melone, F., Moramarco, T., Wagner, W., Dorigo, W., Matgen, P., Martínez-Fernández, J., Llorens, P., Latron, J., Martin, C., and Bittelli, M.: Soil moisture estimation through ASCAT and AMSR-E sensors: An intercomparison and validation study across Europe, Remote Sens. Environ., 115, 3390–3408, https://doi.org/10.1016/j.rse.2011.08.003, 2011.
Brocca, L., Crow, W. T., Ciabatta, L., Massari, C., De Rosnay, P., Enenkel, M., Hahn, S., Amarnath, G., Camici, S., Tarpanelli, A., and Wagner, W.: A Review of the Applications of ASCAT Soil Moisture Products, IEEE J. Sel. Top. Appl., 10, 2285–2306, https://doi.org/10.1109/JSTARS.2017.2651140, 2017.
Chaponnière, A., Boulet, G., Chehbouni, A., and Aresmouk, M.: Understanding hydrological processes with scarce data in a mountain environment, Hydrol. Process., 22, 1908–1921, https://doi.org/10.1002/hyp.6775, 2008.
Coustau, M., Ricci, S., Borrell-Estupina, V., Bouvier, C., and Thual, O.: Benefits and limitations of data assimilation for discharge forecasting using an event-based rainfall–runoff model, Nat. Hazards Earth Syst. Sci., 13, 583–596, https://doi.org/10.5194/nhess-13-583-2013, 2013.
Creutin, J.-D. and Borga, M.: Radar hydrology modifies the monitoring of flash-flood hazard, Hydrol. Process., 17, 1453–1456, https://doi.org/10.1002/hyp.5122, 2003.
Dakhlaoui, H., Seibert, J., and Hakala, K.: Sensitivity of discharge projections to potential evapotranspiration estimation in Northern Tunisia, Reg. Environ. Change, 20, 1–12, https://doi.org/10.1007/s10113-020-01615-8, 2020.
Dorigo, W., de Jeu, R., Chung, D., Parinussa, R., Liu, Y., Wagner, W., and Fernández-Prieto, D.: Evaluating global trends (1988–2010) in harmonized multi-satellite surface soil moisture, Geophys. Res. Lett., 39, L18405, https://doi.org/10.1029/2012GL052988, 2012.
Dorigo, W., Wagner, W., Albergel, C., Albrecht, F., Balsamo, G., Brocca, L., Chung, D., Ertl, M., Forkel, M., Gruber, A., Haas, E., Hamer, P. D., Hirschi, M., Ikonen, J., de Jeu, R., Kidd, R., Lahoz, W., Liu, Y. Y., Miralles, D., Mistelbauer, T., Nicolai-Shaw, N., Parinussa, R., Pratola, C., Reimer, C., van der Schalie, R., Seneviratne, S. I., Smolander, T., and Lecomte, P.: ESA CCI Soil Moisture for improved Earth system understanding: State-of-the art and future directions, Remote Sens. Environ., 203, 185–215, https://doi.org/10.1016/j.rse.2017.07.001, 2017.
Dorigo, W. A., Gruber, A., De Jeu, R. A. M., Wagner, W., Stacke, T., Loew, A., Albergel, C., Brocca, L., Chung, D., Parinussa, R. M., and Kidd, R.: Evaluation of the ESA CCI soil moisture product using ground-based observations, Remote Sens. Environ., 162, 380–395, https://doi.org/10.1016/j.rse.2014.07.023, 2015.
El Alaoui El Fels, A., Bachnou, A., and Alaa, N.: Combination of GIS and mathematical modeling to predict floods in semiarid areas: case of Rheraya watershed (Western High Atlas, Morocco), Arab. J. Geosci., 10, 554, https://doi.org/10.1007/s12517-017-3345-x, 2017.
El Khalki, E. M., Tramblay, Y., El Mehdi Saidi, M., Bouvier, C., Hanich, L., Benrhanem, M., and Alaouri, M.: Comparison of modeling approaches for flood forecasting in the High Atlas Mountains of Morocco, Arab. J. Geosci., 11, 410, https://doi.org/10.1007/s12517-018-3752-7, 2018.
Fernandez-Moran, R., Wigneron, J.-P., De Lannoy, G., Lopez-Baeza, E., Parrens, M., Mialon, A., Mahmoodi, A., Al-Yaari, A., Bircher, S., Al Bitar, A., Richaume, P., and Kerr, Y.: A new calibration of the effective scattering albedo and soil roughness parameters in the SMOS SM retrieval algorithm, Int. J. Appl. Earth Obs., 62, 27–38, https://doi.org/10.1016/J.JAG.2017.05.013, 2017.
Gaume, E., Livet, M., Desbordes, M., and Villeneuve, J.-P.: Hydrological analysis of the river Aude, France, flash flood on 12 and 13 November 1999, J. Hydrol., 286, 135–154, https://doi.org/10.1016/J.JHYDROL.2003.09.015, 2004.
Gruber, A., Dorigo, W. A., Crow, W., and Wagner, W.: Triple Collocation-Based Merging of Satellite Soil Moisture Retrievals, IEEE T. Geosci. Remote, 55, 6780–6792, https://doi.org/10.1109/TGRS.2017.2734070, 2017.
Gruber, A., Scanlon, T., van der Schalie, R., Wagner, W., and Dorigo, W.: Evolution of the ESA CCI Soil Moisture climate data records and their underlying merging methodology, Earth Syst. Sci. Data, 11, 717–739, https://doi.org/10.5194/essd-11-717-2019, 2019.
Hajhouji, Y., Simonneaux, V., Gascoin, S., Fakir, Y., Richard, B., Chehbouni, A., and Boudhar, A.: Modélisation pluie-débit et analyse du régime d'un bassin versant semi-aride sous influence nivale. Cas du bassin versant du Rheraya (Haut Atlas, Maroc), La Houille Blanche, 3, 49–62, https://doi.org/10.1051/lhb/2018032, 2018.
Hwang, S. O., Park, J., and Kim, H. M.: Effect of hydrometeor species on very-short-range simulations of precipitation using ERA5, Atmos. Res., 218, 245–256, https://doi.org/10.1016/j.atmosres.2018.12.008, 2019.
Jackson, T. J., Cosh, M. H., Bindlish, R., Starks, P. J., Bosch, D. D., Seyfried, M., Goodrich, D. C., Moran, M. S., and Du, J.: Validation of advanced microwave scanning radiometer soil moisture products, IEEE T. Geosci. Remote, 48, 4256–4272, https://doi.org/10.1109/TGRS.2010.2051035, 2010.
Jarlan, L., Khabba, S., Er-Raki, S., Le Page, M., Hanich, L., Fakir, Y., Merlin, O., Mangiarotti, S., Gascoin, S., Ezzahar, J., Kharrou, M. H., Berjamy, B., Saaïdi, A., Boudhar, A., Benkaddour, A., Laftouhi, N., Abaoui, J., Tavernier, A., Boulet, G., Simonneaux, V., Driouech, F., El Adnani, M., El Fazziki, A., Amenzou, N., Raibi, F., El Mandour, A., Ibouh, H., Le Dantec, V., Habets, F., Tramblay, Y., Mougenot, B., Leblanc, M., El Faïz, M., Drapeau, L., Coudert, B., Hagolle, O., Filali, N., Belaqziz, S., Marchane, A., Szczypta, C., Toumi, J., Diarra, A., Aouade, G., Hajhouji, Y., Nassah, H., Bigeard, G., Chirouze, J., Boukhari, K., Abourida, A., Richard, B., Fanise, P., Kasbani, M., Chakir, A., Zribi, M., Marah, H., Naimi, A., Mokssit, A., Kerr, Y., and Escadafal, R.: Remote Sensing of Water Resources in Semi-Arid Mediterranean Areas: the joint international laboratory TREMA, Int. J. Remote Sens., 36, 4879–4917, https://doi.org/10.1080/01431161.2015.1093198, 2015.
Javelle, P., Fouchier, C., Arnaud, P., and Lavabre, J.: Flash flood warning at ungauged locations using radar rainfall and antecedent soil moisture estimations, J. Hydrol., 394, 267–274, https://doi.org/10.1016/j.jhydrol.2010.03.032, 2010.
Javelle, P., Organde, D., Demargne, J., Saint-Martin, C., Saint-Aubin, C. de, Garandeau, L., and Janet, B.: Setting up a French national flash flood warning system for ungauged catchments based on the AIGA method, E3S Web Conf., 7, 18010, https://doi.org/10.1051/E3SCONF/20160718010, 2016.
Jiang, D. and Wang, K.: The role of satellite-based remote sensing in improving simulated streamflow: A review, Water, 11, 1615, https://doi.org/10.3390/w11081615, 2019.
Kerr, Y. H., Waldteufel, P., Wigneron, J.-P., Martinuzzi, J.-M., Font, J., and Berger, M.: Soil Moisture Retrieval from Space: The Soil Moisture and Ocean Salinity (SMOS) Mission, IEEE T. Geosci. Remote, 39, 1729–1735, https://doi.org/10.1109/36.942551, 2001.
Kerr, Y. H., Waldteufel, P., Richaume, P., Wigneron, J. P., Ferrazzoli, P., Mahmoodi, A., Al Bitar, A., Cabot, F., Gruhier, C., Juglea, S. E., Leroux, D., Mialon, A., and Delwart, S.: The SMOS Soil Moisture Retrieval Algorithm, IEEE T. Geosci. Remote, 50, 1384–1403, https://doi.org/10.1109/TGRS.2012.2184548, 2012.
Khabba, S., Jarlan, L., Er-Raki, S., Le Page, M., Ezzahar, J., Boulet, G., Simonneaux, V., Kharrou, M. H., Hanich, L., and Chehbouni, G.: The SudMed Program and the Joint International Laboratory TREMA: A Decade of Water Transfer Study in the Soil-plant-atmosphere System over Irrigated Crops in Semi-arid Area, Procedia Environ. Sci., 19, 524–533, https://doi.org/10.1016/J.PROENV.2013.06.059, 2013.
Koster, R. D., Guo, Z., Yang, R., Dirmeyer, P. A., Mitchell, K., Puma, M. J., Koster, R. D., Guo, Z., Yang, R., Dirmeyer, P. A., Mitchell, K., and Puma, M. J.: On the Nature of Soil Moisture in Land Surface Models, J. Climate, 22, 4322–4335, https://doi.org/10.1175/2009JCLI2832.1, 2009.
Koster, R. D., Mahanama, S. P. P., Livneh, B., Lettenmaier, D. P., and Reichle, R. H.: Skill in streamflow forecasts derived from large-scale estimates of soil moisture and snow, Nat. Geosci., 3, 613–616, https://doi.org/10.1038/ngeo944, 2010.
Liu, Y. Y., Parinussa, R. M., Dorigo, W. A., De Jeu, R. A. M., Wagner, W., van Dijk, A. I. J. M., McCabe, M. F., and Evans, J. P.: Developing an improved soil moisture dataset by blending passive and active microwave satellite-based retrievals, Hydrol. Earth Syst. Sci., 15, 425–436, https://doi.org/10.5194/hess-15-425-2011, 2011.
Loew, A. and Mauser, W.: On the disaggregation of passive microwave soil moisture data using A Priori knowledge of temporally persistent soil moisture fields, IEEE T. Geosci. Remote, 46, 819–834, https://doi.org/10.1109/TGRS.2007.914800, 2008.
Loew, A. and Schlenz, F.: A dynamic approach for evaluating coarse scale satellite soil moisture products, Hydrol. Earth Syst. Sci., 15, 75–90, https://doi.org/10.5194/hess-15-75-2011, 2011.
Ma, H., Zeng, J., Chen, N., Zhang, X., Cosh, M. H., and Wang, W.: Satellite surface soil moisture from SMAP, SMOS, AMSR2 and ESA CCI: A comprehensive assessment using global ground-based observations, Remote Sens. Environ., 231, 111215, https://doi.org/10.1016/j.rse.2019.111215, 2019.
Mahto, S. S. and Mishra, V.: Does ERA-5 Outperform Other Reanalysis Products for Hydrologic Applications in India?, J. Geophys. Res.-Atmos., 124, 9423–9441, https://doi.org/10.1029/2019JD031155, 2019.
Marchandise, A. and Viel, C.: Utilisation des indices d'humidité de la chaîne Safran-Isba-Modcou de Météo-France pour la vigilance et la prévision opérationnelle des crues, La Houille Blanche, 6, 35–41, https://doi.org/10.1051/lhb/2009075, 2010.
Marchane, A., Tramblay, Y., Hanich, L., Ruelland, D., and Jarlan, L.: Climate change impacts on surface water resources in the Rheraya catchment (High Atlas, Morocco), Hydrolog. Sci. J., 62, 979–995, https://doi.org/10.1080/02626667.2017.1283042, 2017.
Martínez-Fernández, J. and Ceballos, A.: Mean soil moisture estimation using temporal stability analysis, J. Hydrol., 312, 28–38, https://doi.org/10.1016/j.jhydrol.2005.02.007, 2005.
Massari, C., Brocca, L., Moramarco, T., Tramblay, Y., and Didon Lescot, J.-F.: Potential of soil moisture observations in flood modelling: estimating initial conditions and correcting rainfall, Adv. Water Resour., 74, 44–53, https://doi.org/10.1016/j.advwatres.2014.08.004, 2014.
Massari, C., Brocca, L., Ciabatta, L., Moramarco, T., Gabellani, S., Albergel, C., De Rosnay, P., Puca, S., and Wagner, W.: The Use of H-SAF Soil Moisture Products for Operational Hydrology: Flood Modelling over Italy, Hydrology, 2, 2–22, https://doi.org/10.3390/hydrology2010002, 2015.
Merheb, M., Moussa, R., Abdallah, C., Colin, F., Perrin, C., and Baghdadi, N.: Hydrological response characteristics of Mediterranean catchments at different time scales: a meta-analysis, Hydrolog. Sci. J., 61, 2520–2539, https://doi.org/10.1080/02626667.2016.1140174, 2016.
Miliani, F., Ravazzani, G., and Mancini, M.: Adaptation of Precipitation Index for the Estimation of Antecedent Moisture Condition in Large Mountainous Basins, J. Hydrol. Eng., 16, 218–227, https://doi.org/10.1061/(ASCE)HE.1943-5584.0000307, 2011.
Miralles, D. G., Crow, W. T., and Cosh, M. H.: Estimating Spatial Sampling Errors in Coarse-Scale Soil Moisture Estimates Derived from Point-Scale Observations, J. Hydrometeorol., 11, 1423–1429, https://doi.org/10.1175/2010JHM1285.1, 2010.
Nash, J. E. and Sutcliffe, J. V.: River flow forecasting through conceptual models part I – A discussion of principles, J. Hydrol., 10, 282–290, https://doi.org/10.1016/0022-1694(70)90255-6, 1970.
Norbiato, D., Borga, M., Degli Esposti, S., Anquetin, S., and Gaume, E.: Flash flood warning based on rainfall thresholds and soil moisture conditions: An assessment for gauged and ungauged basins, J. Hydrol., 362, 274–290, https://doi.org/10.1016/j.jhydrol.2008.08.023, 2008.
Ochsner, T. E., Cosh, M. H., Cuenca, R. H., Dorigo, W. A., Draper, C. S., Hagimoto, Y., Kerr, Y. H., Njoku, E. G., Small, E. E., Zreda, M., and Larson, K. M.: State of the Art in Large-Scale Soil Moisture Monitoring, Soil Sci. Soc. Am. J., 77, 1888, https://doi.org/10.2136/sssaj2013.03.0093, 2013.
Olauson, J.: ERA5: The new champion of wind power modelling?, Renew. Energ., 126, 322–331, https://doi.org/10.1016/j.renene.2018.03.056, 2018.
Oudin, L., Michel, C., and Anctil, F.: Which potential evapotranspiration input for a lumped rainfall-runoff model?: Part 1 – Can rainfall-runoff models effectively handle detailed potential evapotranspiration inputs?, J. Hydrol., 303, 275–289, https://doi.org/10.1016/J.JHYDROL.2004.08.025, 2005.
Pérennès, J. J.: L'eau et les hommes au Maghreb. Contribution à une politique de l'eau en Méditerranée, Revue Tiers Monde, 35, 231–232, available at: https://www.persee.fr/doc/tiers_0040-7356_1994_num_35_137_4870_t1_0231_0000_5 (last access: 7 October 2019), 1994.
Saidi, M. E. M., Daoudi, L., Aresmouk, M. E. H., and Blali, A.: Rôle du milieu physique dans l'amplification des crues en milieu montagnard: exemple de la crue du 17 août 1995 dans la vallée de l'Ourika (Haut-Atlas, Maroc), Sécheresse, 14, 107–114, 2003.
Schulte, R. P. O., Diamond, J., Finkele, K., Holden, N. M., and Brereton, A. J.: Predicting the Soil Moisture Conditions of Irish Grasslands, Irish J. Agr. Food Res., 44, 95–110, 2005.
Tramblay, Y., Bouvier, C., Crespy, A., and Marchandise, A.: Improvement of flash flood modelling using spatial patterns of rainfall: a case study in southern France, in: Global Change: Facing Risks and Threats to Water Resources, Proc. of the Sixth World FRIEND Conference, Fez, Morocco, October 2010, IAHS Publ. 340, 172–178, 2010.
Tramblay, Y., Bouvier, C., Ayral, P.-A., and Marchandise, A.: Impact of rainfall spatial distribution on rainfall-runoff modelling efficiency and initial soil moisture conditions estimation, Nat. Hazards Earth Syst. Sci., 11, 157–170, https://doi.org/10.5194/nhess-11-157-2011, 2011.
Tramblay, Y., Bouaicha, R., Brocca, L., Dorigo, W., Bouvier, C., Camici, S., and Servat, E.: Estimation of antecedent wetness conditions for flood modelling in northern Morocco, Hydrol. Earth Syst. Sci., 16, 4375–4386, https://doi.org/10.5194/hess-16-4375-2012, 2012.
Tramblay, Y., Ruelland, D., Somot, S., Bouaicha, R., and Servat, E.: High-resolution Med-CORDEX regional climate model simulations for hydrological impact studies: a first evaluation of the ALADIN-Climate model in Morocco, Hydrol. Earth Syst. Sci., 17, 3721–3739, https://doi.org/10.5194/hess-17-3721-2013, 2013.
Tuttle, S. E. and Salvucci, G. D.: A new approach for validating satellite estimates of soil moisture using large-scale precipitation: Comparing AMSR-E products, Remote Sens. Environ., 142, 207–222, https://doi.org/10.1016/j.rse.2013.12.002, 2014.
US Army Corps of Engineers: Hydrologic Modelling System HEC-HMS, Applications Guide, Hydrologic Engineering Center, Davis, CA, Report Number CPD-74C, 167 pp., 2015.
Vachaud, G., Passerat de Silans, A., Balabanis, P., and Vauclin, M.: Temporal stability of spatially measured soil water probability density function, Soil Sci. Soc. Am. J., 49, 822–828, 1985.
Van doninck, J., Peters, J., Lievens, H., De Baets, B., and Verhoest, N. E. C.: Accounting for seasonality in a soil moisture change detection algorithm for ASAR Wide Swath time series, Hydrol. Earth Syst. Sci., 16, 773–786, https://doi.org/10.5194/hess-16-773-2012, 2012.
Vinet, F., El Mehdi Saidi, M., Douvinet, J., Fehri, N., Nasrallah, W., Menad, W., and Mellas, S.: Urbanization and land use as a driver of flood risk, sub-chapter 3.4.1, in: The Mediterranean region under climate change, IRD Éditions, Marseille, France, 563–575, 2016.
Wagner, W., Pathe, C., Doubkova, M., Sabel, D., Bartsch, A., Hasenauer, S., Blöschl, G., Scipal, K., Martínez-Fernández, J., and Löw, A.: Temporal Stability of Soil Moisture and Radar Backscatter Observed by the Advanced Synthetic Aperture Radar (ASAR), Sensors, 8, 1174–1197, https://doi.org/10.3390/s80201174, 2008.
Wagner, W., Hahn, S., Kidd, R., Melzer, T., Bartalis, Z., Hasenauer, S., Figa-Saldaña, J., de Rosnay, P., Jann, A., Schneider, S., Komma, J., Kubu, G., Brugger, K., Aubrecht, C., Züger, J., Gangkofner, U., Kienberger, S., Brocca, L., Wang, Y., Blöschl, G., Eitzinger, J., and Steinnocher, K.: The ASCAT Soil Moisture Product: A Review of its Specifications, Validation Results, and Emerging Applications, Meteorol. Z., 22, 5–33, https://doi.org/10.1127/0941-2948/2013/0399, 2013.
Western, A. W. and Blöschl, G.: On the spatial scaling of soil moisture, J. Hydrol., 217, 203–224, https://doi.org/10.1016/S0022-1694(98)00232-7, 1999.
Wigneron, J.-P., Kerr, Y., Waldteufel, P., Saleh, K., Escorihuela, M.-J., Richaume, P., Ferrazzoli, P., de Rosnay, P., Gurney, R., Calvet, J.-C., Grant, J. P., Guglielmetti, M., Hornbuckle, B., Mätzler, C., Pellarin, T. and Schwank, M.: L-band Microwave Emission of the Biosphere (L-MEB) Model: Description and calibration against experimental data sets over crop fields, Remote Sens. Environ., 107, 639–655, https://doi.org/10.1016/J.RSE.2006.10.014, 2007.
Zema, D. A., Labate, A., Martino, D., and Zimbone, S. M.: Comparing Different Infiltration Methods of the HEC-HMS Model: The Case Study of the Mésima Torrent (Southern Italy), Land Degrad. Dev., 28, 294–308, https://doi.org/10.1002/ldr.2591, 2017.
Zeng, J., Li, Z., Chen, Q., Bi, H., Qiu, J., and Zou, P.: Evaluation of remotely sensed and reanalysis soil moisture products over the Tibetan Plateau using in-situ observations, Remote Sens. Environ., 163, 91–110, https://doi.org/10.1016/j.rse.2015.03.008, 2015.