the Creative Commons Attribution 4.0 License.
the Creative Commons Attribution 4.0 License.
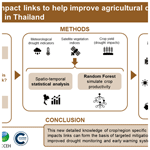
Indicator-to-impact links to help improve agricultural drought preparedness in Thailand
Michael Eastman
Eugene Magee
Lucy J. Barker
Thomas Chitson
Chaiwat Ekkawatpanit
Daniel Goodwin
Jamie Hannaford
Ian Holman
Liwa Pardthaisong
Simon Parry
Dolores Rey Vicario
Supattra Visessri
Droughts in Thailand are becoming more severe due to climate change. Developing a reliable drought monitoring and early warning system (DMEWS) is essential to strengthen a country's resilience to droughts. However, for a DMEWS to be valuable, the drought indicators provided to stakeholders must have relevance to tangible impacts on the ground. Here, we analyse drought indicator-to-impact relationships in Thailand, using a combination of correlation analysis and machine learning techniques (random forest). In the correlation analysis, we study the link between meteorological drought indicators and high-resolution remote sensing vegetation indices used as proxies for crop yield and forest growth impacts. Our analysis shows that this link varies depending on land use, season and region. The random forest models built to estimate regional crop productivity allow a more in-depth analysis of the crop- and region-specific importance of different drought indicators. The results highlight seasonal patterns of drought vulnerability for individual crops, usually linked to their growing season, although the effects are somewhat attenuated in irrigated regions. Integration of the approaches provides new, detailed knowledge of crop- and region-specific indicator-to-impact links, which can form the basis of targeted mitigation actions in an improved DMEWS in Thailand and could be applied to other parts of Southeast Asia and beyond.
- Article
(9609 KB) - Full-text XML
-
Supplement
(1886 KB) - BibTeX
- EndNote
Droughts are one of the costliest natural hazards worldwide (FAO, 2021). Their frequency and duration are expected to increase in many parts of the world due to climate change (IPCC, 2021, 2022; WBG and ADB, 2021). Over the past few decades, Thailand has already seen a rise in impacts from a warming world, experiencing increasingly unpredictable weather, with an alternation of droughts and floods on a 2- to 3-year cycle (Ikeda and Palakhamarn, 2020), causing a wide range of impacts. This trend is expected to intensify further in the near future in Southeast Asia, as highlighted by Hariadi et al. (2023).
One notable recent example is the severe 2020 drought, which was driven by a shorter monsoon period and a strong El Niño event (CFE-DMHA, 2022). The drought caused impacts in water supply, water quality, crop production and the economy, with an economic loss of THB 46 billion (USD 1.4 billion, GBP 1.1 billion; Sowcharoensuk and Marknual, 2020). Other notable recent droughts include the 2005 event, in which 11 million people in 71 (out of 77) provinces were affected by water shortages; the 2008 event where over 10 million people in rural areas were affected (Ikeda and Palakhamarn, 2020); and the 2015–2016 event, which affected the upper-middle part of the country the most, and was so severe that at the Ubol Ratana Dam, in northeastern Thailand, steps were taken to use “dead storage” (i.e. the last 1 % in the bottom of the reservoir; CFE-DMHA, 2022). Overall, the National Disaster Relief Centre estimates that drought events between 1989 and 2017 caused more than THB 19.1 billion (USD 0.6 billion, GBP 0.5 billion) of damage to the Thai economy, with average annual economic damages of almost THB 0.6 billion per year (USD 20 million, GBP 16 million; NESDC, 2021).
One sector particularly affected by droughts in Thailand is agriculture (Yoshida et al., 2019); in particular, rice, corn and other cash crops periodically suffer economic losses (Ikeda and Palakhamarn, 2020). Thailand is currently the second largest rice exporter in the world (OECD, 2020), and rice fields utilise 70 % of Thailand's total water supply (ICID, 2020). Thailand is also the second biggest sugar exporter, and the 2020 drought resulted in a 28 % fall in production (Thammachote and Trichim, 2021). However, drought risk is also moderated or exacerbated by human activities. Areas with water reservoirs and extensive irrigation facilities are more resilient and are impacted less by droughts than rainfed agriculture and areas without reservoir storage. In the northeast, higher water demand for rice cultivation during the dry seasons, combined with limited irrigation infrastructure, exacerbates water scarcity (CFE-DMHA, 2022).
Thirty percent of Thailand's population work in agriculture, and drought threatens their income and poses food security issues. Given this considerable impact that droughts have on Thai society and the expectation of it worsening in the coming years and decades, there is an urgent need to improve preparedness for and resilience to droughts in the country (UNDRR and ADCP, 2020). This also aligns with the priorities of the UNDRR's (United Nations Office for Disaster Risk Reduction) Sendai Framework for Disaster Risk Reduction 2015–2030, which aims to achieve a substantial reduction in disaster risk and the loss of lives, livelihoods and health, as well as economic, physical, social, cultural and environmental assets of persons, businesses, communities and countries over the next 15 years (UNDRR, 2015). One important aspect of improving drought resilience lies in enhancing the drought monitoring and early warning (DMEW) capabilities of a country, in order to detect droughts in their early stages such that proactive mitigation strategies can be implemented (Bachmair et al., 2016a).
According to the World Meteorological Organization (WMO), drought can be defined as a prolonged dry period in the natural climate cycle (WMO, 2014). Since Wilhite and Glantz's study (1985), drought has commonly been categorised into various types, often differentiating between meteorological, hydrological and soil moisture (or agricultural) droughts, alongside various others. Many drought indices have been developed for drought monitoring purposes for these different types of drought over the past few decades (Lloyd-Hughes, 2014). In this paper, we consider a drought to be a period drier than normal for that time of year at a given location and distinct from the impacts it causes. Droughts can occur without causing any impacts, in which case they are not a concern for water managers or water users.
Drought impacts (e.g. crop failure, water quality issues, etc.) are what stakeholders are interested in from a DMEW point of view. However, impact data are scarce and generally not routinely monitored. There are significant challenges in collecting and monitoring drought impacts, including the visibility of drought impacts, which can be diffuse, delayed and non-structural (e.g. in comparison to the impacts of flooding). Nevertheless, while impact research is inherently challenging, it is also pivotal to drought management. While rainfall or river flow deficits can help track drought evolution, ultimately it is the impacts of drought which are of greatest importance for water managers and other stakeholders. Numerous international initiatives have highlighted that information on drought impacts is the key “missing piece” of drought monitoring and forecasting (e.g. Bachmair et al., 2016a), and some effort has been invested in collating drought impact data at national or international scales (e.g. in Europe: European Drought Impact report Inventory (EDII), Stahl et al., 2016; in the US: Drought Impact Reporter (DIR), Smith et al., 2014). In an age where there have been huge advances in real-time hydrometeorological monitoring, better prediction of impacts would be the single greatest practical advance in paving the way for improved drought resilience. Understanding the link between drought indicators and impacts is an essential first step to achieve this goal (Bachmair et al., 2016a).
Some of the most commonly used indices in operational DMEWS are the meteorological standardised indices such as the Standardised Precipitation Index (SPI; McKee et al., 1993) and Standardised Precipitation Evapotranspiration Index (SPEI; Vicente-Serrano et al., 2010). However, these indicators based purely on meteorological status are not always well correlated with drought impacts (Bachmair et al., 2018), as impacts often occur when precipitation deficits have propagated through the hydrological cycle to deficits in soil moisture or river flows, for example. Moreover, precipitation deficit is likely to cause more impacts in water-limited regions than in regions with abundant water, though water management practices can counteract this effect to a certain extent. Drought indices are only meaningful to decision-makers if the relationship to drought impacts is known, i.e. understanding the type and magnitude of impacts that can be expected for different drought index values. For regions where drought impact data are available, the relationship between drought indices to drought impacts can be studied (e.g. Bachmair et al., 2016b; Parsons et al., 2019; Wang et al., 2020). Where drought impact data are not readily available, remote sensing vegetation indices (VIs) can provide a proxy for drought impacts on vegetation.
VIs are commonly used to monitor the impacts of drought on vegetation. The Normalised Difference Vegetation Index (NDVI) is one of the most established and widely used VIs (Tucker, 1979). It exploits the sharp increase in vegetation reflectance across the red and near-infrared (NIR) regions of the electromagnetic spectrum, known as the “red edge”, to detect photosynthetically active plant material and infer plant stress. However, the Vegetation Condition Index (VCI), a pixel-based normalisation of NDVI, offers a more robust indicator for seasonal droughts by minimising spurious or short-term signals and amplifying long-term trends (Anyamba and Tucker, 2012; Liu and Kogan, 1996). VCI has been widely used and has proved to be effective in monitoring vegetation change and signalling agricultural drought (e.g. Jiao et al., 2016). The Vegetation Health Index (VHI) is a composite index that combines the VCI and Temperature Condition Index (TCI) – a pixel-based normalisation of the land surface temperature (LST) – and is also commonly used to monitor vegetation stress and drought conditions (Kogan, 1997). VHI incorporates the effect of temperature and is therefore more suitable for monitoring the effect of drought in species more sensitive to concurrent water and heat stress. VHI has successfully been used worldwide to monitor vegetation stress and drought conditions (e.g. Jain et al., 2009; Singh et al., 2003; Unganai and Kogan, 1998). Note that these VIs are relative indices that compare current conditions to the long-term average to measure vegetation health and therefore are dependent on the environmental and climatic conditions of the study area. As such, they should be used in conjunction with information on the drought hazard situation to distinguish between drought and different hazards on vegetation (e.g. disease, floods, anthropogenic impacts, etc.).
In addition to their use as drought indicators as discussed above, VIs are often used as proxies for agricultural drought impacts. The relationship between crop yield and VIs varies by crop type and location but has been shown to be strong in many locations. For example, strong correlations were found between VIs and crop yield in North America (e.g. maize in Bolton and Friedl, 2013; winter wheat, sorghum and corn in Kogan et al., 2012), South America (e.g. white oat in Brazil in Coelho et al., 2020), Europe (e.g. maize in Germany in Bachmair et al., 2018; cereals in Spain in García-León et al., 2019), Asia (e.g. sugarcane in India in Dubey et al., 2018), the Middle East (e.g. paddy rice in Iran in Shams Esfandabadi et al., 2022), Africa (e.g. millet and sorghum in the Sahelian region in Maselli et al., 2000) and Australia (e.g. wheat in Smith et al., 1995).
Data science and machine learning are fast-moving fields and are increasingly being used for the study of environmental science, though still in its infancy (Blair et al., 2019). Random forest (RF) models have been used to link drought indicators to drought impacts (e.g. Bachmair et al., 2016b), including drought impact forecasting with relative success (Hobeichi et al., 2022; Sutanto et al., 2019). These emerging techniques within the field of DMEW offer great potential to move from simply monitoring droughts using indices to drought impact estimation, which would revolutionise the early warning aspect of drought mitigation, enabling action to be taken before impacts occur.
Despite the significance of droughts in Thailand, few previous studies have analysed the link between drought indices and drought impacts in the country. Thavorntam et al. (2015) and Thavorntam and Shahnawaz (2022) looked at links between SPI and VIs but only at four test sites in the northeast of Thailand. Prabnakorn et al. (2018) and Khadka et al. (2021) have both focused on the drought-prone Mun River basin situated in the northeast of Thailand; both studies have found that SPEI shows a good correlation with crop yield. However, no previous study has looked comprehensively at drought indicator-to-impact links at a national scale in Thailand, and to our knowledge none has used machine learning techniques to estimate drought impacts in the country.
The ambition of this paper was to fill the gap in the literature on studies investigating the links between drought indicators and impacts at a national scale in Thailand. Specifically, we focused on agricultural drought impacts, considering different crops and seasons, and compared the relative utility of traditional statistical methods at high-resolution (i.e. remote sensing data at provincial scale) vs. lower-resolution sectoral-specific analyses (i.e. applying machine learning approaches to regional/provincial yield data) to inform improved approaches for national DMEW. The overall aim was to support agricultural drought management and inform targeted action and policy by water resource managers. To that end, this paper evaluates how relationships between drought indices and impacts vary according to the time of year, index, accumulation period length and location in Thailand. The approach presented is relevant internationally and could be replicated in other parts of the world to improve the management of agricultural droughts and their impacts.
2.1 Study area
Thailand is located between 5∘30′ and 20∘30′ N latitudes and between 97∘30′ and 105∘30′ E longitudes and has an area of 51 million ha, from which 46.5 % is agricultural area (77 % of which is rainfed). Paddy fields cover 46 % of that cultivated area, with around 30 % being irrigated (OAE, 2022).
Most of the country experiences distinct wet and dry seasons, except some parts of the southern region, which experience a wet and humid climate throughout the year. The average annual rainfall of the whole country is about 1700 mm, ranging from 1200 mm in the north and central plain up to 2000–2700 mm in the western part of the south and the eastern part of the country (ICID, 2020).
Droughts often occur in two distinct periods: between June and September, as a consequence of the delay in the onset of rainfall or due to low precipitation during the dry season between October and May. The occurrence of drought in Thailand is increasingly associated with the El Niño–Southern Oscillation (ENSO) cycle, which brings drier-than-average rainfall conditions (UNDRR and ADCP, 2020).
Figure 1 shows the six regions and 77 provinces that were used in our analysis. Provinces (changwat) are the primary local government unit in Thailand. The regions do not have an administrative character but are commonly used for geographical and scientific purposes (e.g. Martin and Ritchie, 2020; Sanoamuang and Dabseepai, 2021). The dominant land cover for each province is shown in Fig. S3 in the Supplement.
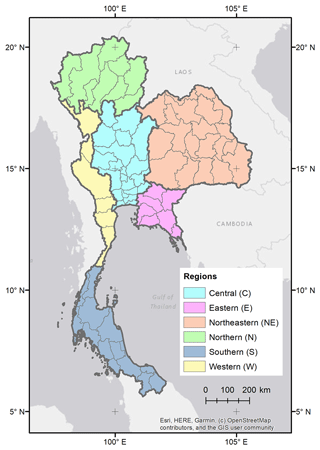
Figure 1Map of Thailand showing provinces and regions used in this study. The provinces are the smaller areas shown within each of the coloured regions.
In addition to these regions and provinces, in the Results and Discussion sections, we use “the north” to refer to the area encompassing regions N, NE, C, W and E, as opposed to “the south”, comprising only region S.
Regional differences
The northeastern region (NE) consists mainly of the dry Khorat Plateau. Unlike the more fertile areas of Thailand, the NE has a long dry season, and much of the land is covered by sparse grasses. The main crops cultivated in this region are glutinous rice (two harvests), cash crops such as sugar cane and cassava, and to a lesser extent rubber. This region is the most prone to drought (LePoer, 1987) and as such is particularly vulnerable to agricultural droughts, as highlighted by several studies (e.g. Mongkolsawat et al., 2001; Sa-nguansilp et al., 2017; Wijitkosum, 2018).
The northern region (N) is a mountainous region and the most forested region of Thailand. Although it has suffered from extensive deforestation due to agricultural expansion over the past few decades, there has been some reforestation in recent years (RFD, 2022). Many dams and irrigated croplands are situated in this region.
The western (W) region is characterised by high mountains and steep river valleys. Western Thailand hosts much of Thailand's less-disturbed forest areas. The region is home to many of the country's major dams.
The eastern (E) region is characterised by short mountain ranges alternating with small basins of short rivers which drain into the Gulf of Thailand; fruit is a major component of agriculture in the area.
The central (C) region is a natural self-contained basin often termed “the rice bowl of Asia”. A complex irrigation system and fertile soil support the cultivation of rice paddies. It is the most densely populated region of Thailand, with metropolitan Bangkok on its southern edge (LePoer, 1987).
The southern region (S) is part of a narrow peninsula and is distinctive in climate, terrain and resources. This region is characterised by north–south mountain barriers, tropical forest and the absence of large rivers. It is the wettest region in Thailand and is not generally considered to suffer from drought impacts (LePoer, 1987).
2.2 Data
Table 1 lists the data used in this study, with details on the type of data, spatial resolutions, temporal resolutions, periods available, post-processing applied in this study and references.
From these datasets, the following drought indicators were calculated.
-
Standardised meteorological indicators. This includes the Standardised Precipitation Index (SPI; McKee et al., 1993) and Standardised Precipitation Evapotranspiration Index (SPEI; Vicente-Serrano et al., 2010) for accumulation periods of 1–6, 9, 12, 18 and 24 months. For the SPI, the data were fitted to a gamma distribution, whereas for SPEI, a generalised logistic distribution was used, as recommended by the original authors.
-
Vegetation indices (VIs) from remote sensing. The Vegetation Condition Index (VCI), Temperature Condition Index (TCI) and Vegetation Health Index (VHI) were calculated on a monthly time step following Bachmair et al.'s (2018) methodology, which is detailed in Sect. S1 in the Supplement.
In this study, when the word “indicators” is used on its own, we refer to both meteorological indicators (SPI and SPEI) and VIs (VCI, TCI and VHI).
Annual crop yield data (OAE, 2021) are used as a measure of agricultural drought impacts. Although drought is not the only factor that can cause crop yield departure, Venkatappa et al. (2021) have shown that it is the main driver of crop loss in Thailand.
Spatial and temporal aggregation
To derive the meteorological indicators, we first averaged the meteorological variables (precipitation and PET) for each province and then calculated the standardised indicators based on the province-averaged time series. For VIs, we first derived them at the pixel level for the entire country and then used a land cover map to differentiate between forest- and crop-covered pixels. We then calculated province-level VI averages separately for forests and crops, using the corresponding land cover mask. We used monthly time series for most of our analysis, with the exception of the comparative analysis between VIs and crop yield (described further in Sect. 2.3.1) where VIs were averaged over the growing season for each crop.
2.3 Methods
Figure 2 shows a schematic representation of the methodological steps involved in this study.
2.3.1 Indicator-to-indicator correlation analyses
For the correlation analysis, data were used at the finer province resolution.
VIs vs. crop yield
Firstly, a correlation analysis was performed between the vegetation indices (VIs) and the crop yield data. This was done to investigate whether the VIs could be used as a proxy for agricultural impacts, as spatially distributed data on crop areas were unavailable to accompany the yield data. VIs were masked using the land cover data to ensure that only areas covered by cropland were considered. The cropland-masked monthly VIs were then averaged to the province level, the time series were filtered to only include the growing season of the spatially dominant crop within each province, and an annual average was taken. The growing season was taken from Lacombe et al. (2017) for Cassava, the FAO GIEWS (Global Information and Early Warning System on Food and Agriculture) Country Briefs (FAO, 2021) for paddy rice, Arunrat et al. (2022) for corn and the FFTC (2015) for longan. The annual time series for the VIs for each province was correlated with the yield of the dominant crop for that province using a Pearson correlation (Pearson, 1920). The Pearson correlation was selected since it estimates the strength of normalised covariance between two variables, allowing for insight into how closely related the two variables are.
Meteorological drought indicators vs. VIs
The Pearson correlation was also used to compare the standardised indicators (SPI and SPEI) and vegetation indices (VCI and VHI) for both forest areas and cropland, where the crop-masked vegetation indices were treated as a proxy for the agricultural impact. This approach was used to investigate the effect of meteorological conditions on crops and forests and identify the most relevant indicators from a drought monitoring perspective. Monthly crop-masked VCI values were regressed against time using linear regression, and the residuals were used to remove linear trends, accounting for increased biomass from developments in agricultural technology and practices. The analysis was done spatially, making use of province-averaged indicators, and temporally, by splitting the time series into wet and dry seasons. While the specific months of these seasons vary across the country, a general approach was taken with the wet season being May to October and the dry season November to April, inclusive. Correlation coefficients were calculated between standardised meteorological indicators for all given accumulation periods and the VIs. For each VI and province, the standardised meteorological indicator with the largest-magnitude correlation was identified, and critical values were calculated by accounting for autocorrelation using Pyper and Peterman's (1998) methodology.
To check how much difference there is between SPI and SPEI and verify that they are different enough to justify using both indices in our analysis, we compared the two indicators to determine how much of SPEI can be explained by SPI over the whole period, each season and each accumulation period, details of which are given in Sect. S2 of the Supplement.
2.3.2 Simulating crop productivity
Regional random forest (RF) models were used to predict agricultural impacts (crop yield). RF regression is a machine learning algorithm that combines predictions from multiple decision trees to make a more accurate prediction than a single tree. The analysis was carried out at regional level by aggregating all provincial data to the regional level, as data were too scarce at the provincial level to be able to train the models at that higher resolution.
As input data to the models, we used SPI, SPEI, VCI and TCI for each individual month separately. Note that VHI was not used here, as it is a combination of VCI and TCI and is therefore strongly correlated to both. Accumulation periods of 1–6, 9, 12, 18 and 24 months were used for SPI and SPEI. All input data were first regressed against time, and the residuals were used as input to the random forest models to account for linear trends. Annual crop yield data for a range of individual crops were used to train the models and evaluate them.
First, a correlation analysis of all input data against each crop's annual yield was carried out with the objective of ranking the indicators in the order of the highest to lowest correlation with crop yield. All indicators were split by month (i.e. all the Januaries lumped together, all the Februaries, and so on), and the correlations were ranked by p value.
In a second step, we built the feature set by adding features (i.e. indicators) in the order of ascending correlation p value, while maintaining all variance inflation factor (VIF) values below 5 (to minimise multicollinearity). This means that for strongly correlated input variables, only the variable with the strongest correlation with crop yield was used to build the model.
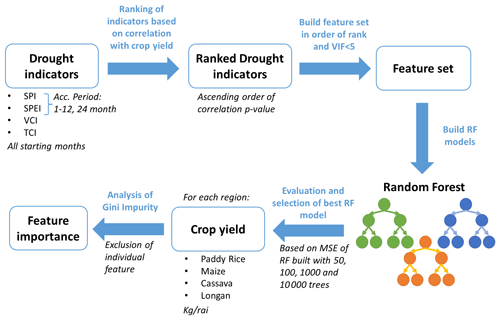
Figure 3Schematic representation of the steps involved to build RF models and associated analysis in this study.
In the final step, we built the forests to predict crop yield. A total of 38 individual RFs were built for each combination of crop and region using the six regions shown in Fig. 1 and seven crops – cassava, corn S1 (March–October), corn S2 (November–February), mixed corn (corn S1 + corn S2), paddy rice, second rice and longan. Only combinations that had more than 50 samples (province yield–year combinations) were used, and as a result, corn S1, corn S2 and mixed corn were removed from region S, and corn S2 was removed from region E. Figure 3 shows a schematic representation of the steps involved to build the RF models.
Due to the considerable number of RFs trained and evaluated, the number of trees within each RF was selected using an automated process by evaluating the mean squared error for RFs consisting of 50, 100, 1000 and 10 000 trees. The number of trees that resulted in the lowest mean squared error was used to train the final model for each region–crop combination. To enable parameters to be estimated on the full dataset, estimation of the optimal number of trees and training of the final model were performed using 5-fold cross validation.
Finally, using these models, we investigated the relative importance of features in explaining the variance in crop yield. The average decrease in Gini impurity resulting from the exclusion of a certain feature can provide insights into its relative importance for simulating the target variable. In this case, indicators with relatively high decreases in Gini impurity resulting from their exclusion were considered important for the simulation of the productivity of the crop in question. While RFs were built to predict crop yields, the main focus of our study was their use to study feature importance to identify monitoring priorities for different regions and crops.
3.1 Correlation analysis: indicator-to-indicator
3.1.1 VIs vs. crop yield
In most provinces, we found that VCI is positively correlated with crop yield for the dominant crop in that province, and in the majority of cases that correlation is statistically significant (p ≤ 0.05) (See Fig. 4a). In 13 provinces (out of 77), VCI is negatively correlated with crop yield (provinces in blue in Fig. 4a), which suggests VCI is not directly linked to crop yields in these provinces and may not be suitable as a proxy for agricultural impacts. In the most northern provinces, the land cover is highly dominated by dense forest (Fig. S3), and the limited crop area has a mixture of crops, which might explain these poor relationships.
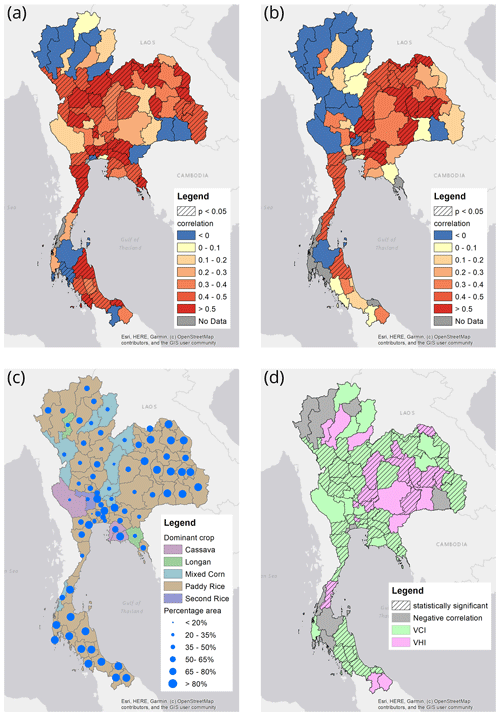
Figure 4(a) Correlation between VCI and crop yield for the dominant crop in each province, (b) correlation between VHI and crop yield for the dominant crop in each province, (c) map of the dominant crop in each province and the percentage area of said crop over the total crop area in each province, and (d) map of VI best correlated with crop yield for the dominant crop in each province.
VHI is negatively correlated with yields in more provinces than VCI but has stronger correlation than VCI in some provinces (Fig. 4b). Figure 4d shows the VI best correlated with crop yield (for dominant crop) in each province. For more than 90 % of the provinces, at least one of the VIs is positively correlated with crop yield. Note that in some provinces the dominant crop – especially provinces in W, C and E regions – accounts for less than 50 % of the total cultivated area (Fig. 4c). This can introduce significant noise in the data, and therefore these results should be treated with caution and be regarded as a general indication that VIs are a reasonable proxy for crop yield, rather than an absolute validation. In some cases, there is no obvious reason as to why the correlation is very different between two neighbouring provinces which share a similar topography, land cover, climatology and dominant crop type. However, differences in irrigation or agricultural practices or in the outbreak of pests and diseases could be contributing factors. Exploring these factors in future research may provide insights into the observed differences in correlations. Crop yield at field scale or a high-resolution land cover map, which includes information on crop type, would be needed to carry out a robust validation, but in the absence of such data, we consider that the strong correlation between VIs and crop yield found in most provinces provides enough confidence to utilise VIs as a reasonable proxy for crop yield in subsequent analysis in this paper.
The following analysis focusses on VCI to simplify the messaging, but the equivalent plots for VHI can be found in the Supplement. Note that for this analysis, TCI was not considered, as its effect is implicit within VHI as described in Sect. 2.2 above.
Note that we also use VIs as proxy for forest growth in the following analysis, but we had no verification data to validate this assumption. However, VIs have shown strong links to forest health and drought impacts in previous studies (e.g. Byer and Jin, 2017; Torres et al., 2021). Therefore, we consider the assumption that VIs are good proxies for drought impacts on forests to be reasonable.
3.1.2 Meteorological indicators vs. VIs
Meteorological drought indicators were then correlated with VIs to assess the effect of meteorological conditions on crops and forests and identify the most relevant drought indicator for impacts on crops and forests. The analysis was divided between dry and wet seasons.
Dry season
Figure 5 shows the strongest correlation for all the combinations of meteorological indicators vs. VCI for the dry season (Fig. 5a and b) with the corresponding meteorological indicator (Fig. 5c and d) for crops (Fig. 5a and c) and for forests (Fig. 5b and d). Strong and statistically significant correlations can be seen for most provinces in the north. Correlations are higher for crops than for forests.
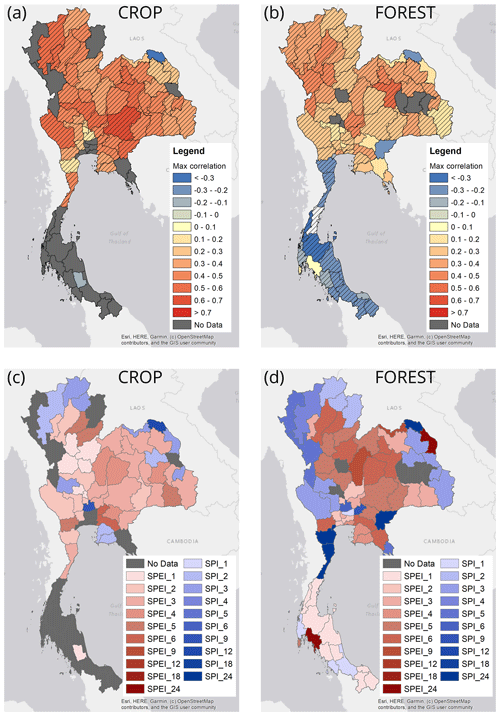
Figure 5For the dry season, maximum correlation (all combinations of meteorological indicators with VCI) for each province for (a) crops and (b) forests and the corresponding meteorological indicator and accumulation period for each province for (c) crops and (d) forests.
For crops, we find high correlations between VCI and SPEI of a relatively short accumulation period during the dry season, suggesting that short droughts affect crops the most. The fact that SPEI is generally more highly correlated with crop production than SPI highlights the important link between the evaporative demand and impact on crops.
For forests, we observe a very clear north–south split, with positive correlations in the north and negative in the south. A positive correlation between VCI and the meteorological indicators suggests that a deficit in water availability (as indicated by negative SPI or SPEI) leads to a decline in vegetation growth (reduced VCI). In contrast, a negative correlation suggests that such a deficit leads to an increase in vegetation growth. This second scenario may seem counterintuitive, but it can occur in energy-limited environments where water is not the limiting factor. In such cases, short-duration droughts (i.e. periods drier than usual for the time of year) can stimulate increased vegetation growth, as droughts in energy-limited environments are often associated with increased radiation (i.e. energy) due to decreased cloud cover. This is discussed further in Sect. 4.3.
Except in the south, the best-correlated accumulation period is generally longer for forests than for crops. Forests are impacted by droughts that are longer in duration than those affecting crops.
Wet season
Figure 6 shows the highest correlation for all the combinations of meteorological indicators with VCI for the wet season (Fig. 6a and b) with the corresponding meteorological indicators (Fig. 6c and d) for crops (Fig. 6a and c) and for forests (Fig. 6b and d). The maximum correlation is, in general, lower for the wet season than for the dry season, which indicates that the impact of meteorological droughts on crops and forests is less severe during the wet season.
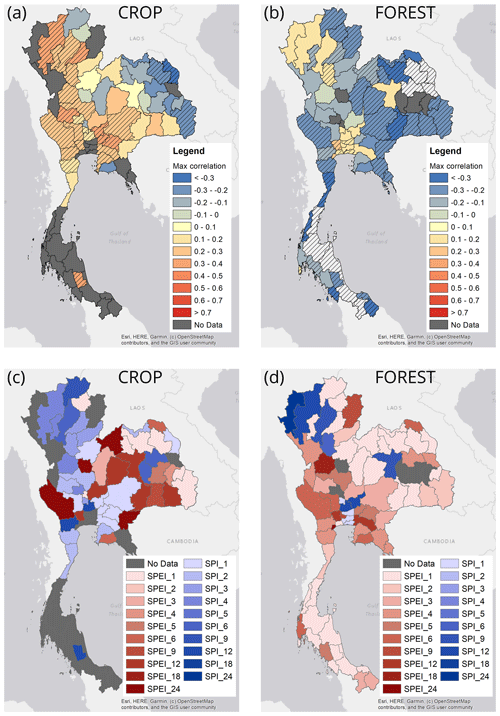
Figure 6For the wet season, maximum correlation (all combinations of meteorological indicators with VCI) for each province for (a) crops and (b) forests and the corresponding meteorological indicator and accumulation period for each province for (c) crops and (d) forests.
A clear difference between crops and forests can be observed. Whereas crops suffer some negative impact from meteorological drought (positive correlations), even during the wet season, forest growth seems to benefit from short droughts (negative correlations in most provinces, with short accumulation periods ranking first in many provinces).
The figures corresponding to Figs. 5 and 6 for VHI can be found in the Supplement (Figs. S4 and S5).
3.2 Simulating crop productivity
3.2.1 Model performance
The ability of RFs to simulate crop yield in Thailand varied across the country and between different crops. Cassava productivity was simulated well, and RFs were able to explain variance in the data across the country, apart from in the S region where the lack of cassava being grown in this region made approximating the relationships between indicators and impacts difficult (Fig. 7a). The variance in cassava yield data explained by the indicators also varied across the country, with more variance explained in the E and NE regions than the W region.
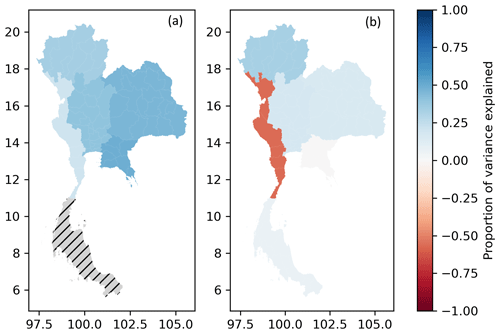
Figure 7Amount of variance explained in cassava (a) and paddy rice (b) productivity data by the random forest models.
Indicators were also able to explain more than 33 % of the observed variance in corn (S1, S2 and mixed) yield in the N region and S region for corn S2 (Fig. S6). Furthermore, the amount of variance in paddy rice yield data explained by the RF models only exceeded 33 % in the N region (Fig. 7b). In total, variance explained exceeded 33 % for five crops in the N region, one crop (cassava) in the NE region, no crops in the W region, one crop (cassava) in the C region, two crops in the E region and no crops in the S region.
3.2.2 Feature importance
RF models also allow us to investigate the relative importance of features (i.e. indicators) in explaining the variance in crop yield by calculating the average decrease in Gini impurity from the exclusion of individual features, as described in Sect. 2.3.2. To ensure the feature importance were representative of the variation observed within the crop yield data, only RFs that explained > 33 % of the variance observed in the crop productivity data were selected for analysis. This resulted in the feature importance of nine RFs being presented here.
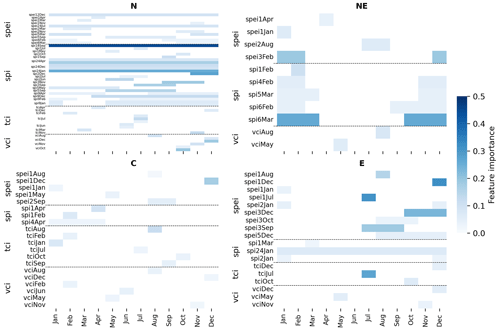
Figure 8Heatmap displaying the relative feature importance (impurity decrease) of each indicator used in the random forest models (for all crops) for each region. Each row corresponds to a different indicator, with the y axis representing the indicator and the length of the bar representing the accumulation period. The x axis indicates the time of year (month) when the indicator is the most relevant for predicting crop yield. For instance, spi6Mar in the NE region represents SPI with a 6-month accumulation period for March, and the bar covers October to March (i.e. the 6-month period ending in March). The bars are shaded darker for indicators that are more important in the models. Unlike Figs. 9 and 10, which show only one crop per subplot, this figure includes all crops that can be modelled in each region. Region N has five models (cassava, corn S1, corn S2, mixed corn, and paddy rice models), while Region NE, region C and region E have one, one and two models, respectively. The number of rows (i.e. indicators) in each subplot is a consequence of the number of models in each region and the number of variables in each model. The thickness of the lines is a result of the number of indicators displayed for each region and has no meaning attached to it. Finally, note that different crop models within a region can use the same indicators, leading to some indicators being repeated and having multiple rows within the same region (e.g. vciDec in region N).
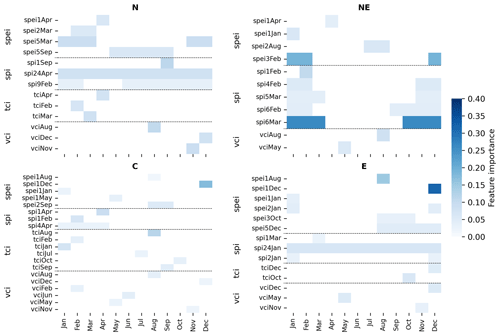
Figure 9Heatmap displaying the relative feature importance (impurity decrease) of each indicator used in the random forest models for cassava for each region. Each row corresponds to a different indicator, with the y axis representing the indicator and the length of the bar representing the accumulation period. The x axis indicates the time of year (month) when the indicator is the most relevant for predicting crop yield. For instance, spi6Mar in region NE represents SPI with a 6-month accumulation period for March, and the bar covers October to March (i.e. the 6-month period ending in March). The bars are shaded darker for indicators that are more important in the models. Unlike Fig. 8, each subplot here shows only cassava models for each region. However, the number of indicators can still differ between models due to the feature selection process that eliminates highly correlated indicators, which may vary between regions. The number of rows (i.e. indicators) in each subplot reflects the number of variables in each model, and the thickness of the lines is a result of the number of indicators displayed for each region and has no meaning attached to it.
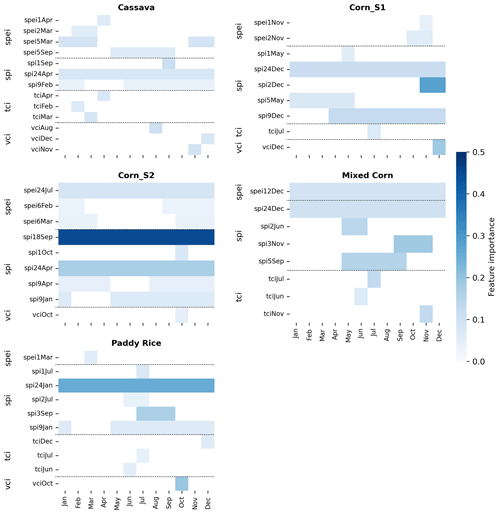
Figure 10Heatmap displaying the relative feature importance (impurity decrease) of each indicator used in the random forest models for five different crops in region N. Each row corresponds to a different indicator, with the y axis representing the indicator and the length of the bar representing the accumulation period. The x axis indicates the time of year (month) when the indicator is the most relevant for predicting crop yield. For instance, spi5Sep for mixed corn represents SPI with a 5-month accumulation period for September, and the bar covers May to September (i.e. the 5-month period ending in September). The bars are shaded darker for indicators that are more important in the models. Unlike Fig. 8, each subplot here only shows the model for a single crop in region N. The number of rows (i.e. indicators) in each subplot reflects the number of variables in each model, and the thickness of the lines is a result of the number of indicators displayed and has no meaning attached to it.
Figure 8 shows feature importance in each region for all available crops aggregated, whereas Fig. 9 presents the feature importance in each region for a single crop (cassava), and finally Fig. 10 shows feature importance for five distinct crops in region N only. Figure S6 in the Supplement also shows the feature importance in two regions for the various types of corn.
Long accumulation periods are assigned relatively high importance in the N region compared to other regions (Fig. 8). This agrees with regional differences observed in cassava feature importance, where SPI24 had the highest mean decrease in impurity (i.e. feature importance) in the cassava model for region N (Fig. 9). Furthermore, 22 SPI indicators (different accumulation periods and different times of year) were used in the N region RFs, more than any other indicator (11 SPEI, 10 VCI, 6 TCI; Fig. 10). For corn S1, S2 and mixed corn, SPI consisted of , and of the indicators used to simulate crop yield, respectively. In contrast, just features used in the cassava model were SPIs; the remaining features were a combination of SPEI, TCI and VCI. Differences in accumulation periods of the most important indicators were also observed between crops. Whereas the corn models (all types) had many long-accumulation-period indicators, cassava included eight indicators covering just 1 month, demonstrating the importance of short-term effects on cassava production. Paddy rice also exhibits high importance for several indicators of short accumulation periods. However, in contrast to cassava, features selected by the model for paddy rice yield simulation were SPIs.
Mean decreases in impurity for cassava models appear to exhibit seasonality in the NE and E regions, with a focus on October–March in the NE region, and August–January in the E region (Figs. 9 and S6). However, seasonality is less evident in the N and C regions. Seasonality is also observed in the correlation coefficients between cassava yields and meteorological indicators in the NE and E regions (Fig. S7). Figure S7 shows that meteorological indicators accumulated between October and April exhibit the highest correlation coefficients in the NE region, agreeing with the results from the RF analysis. In contrast, the highest correlation coefficients occur for indicators calculated from data in February–July in the E region. This period is only covered by a single indicator in the RF model, assigning relatively low importance for estimating cassava yields. This may be due to crop yields being more sensitive to floods in region E, supported by the strong negative correlations between meteorological indicators and cassava yields during August–December (Fig. S7). This is also consistent with findings from Venkatappa et al. (2021), who found that floods caused more damage than droughts to crops in the eastern region.
4.1 Spatial variation in indicator-to-impact relationships
The correlation analysis showed that in most of the provinces in region N (Figs. 5c and 6c), SPI is more correlated with crop yield than SPEI, whereas SPEI dominates in the rest of the country during both the wet and dry seasons. This, combined with the high importance placed on SPI features by the RF models in region N (Figs. 8 and 9), demonstrates the strong relationship between SPI and crop yield, particularly compared to other parts of the country. Region N is one of the most irrigated areas of Thailand together with region C (FAO, 1999; Varawoot, 2016). A region's dependence on irrigation (and therefore water storage) seems to result in (i) a lack of variation in indicator importance across different seasons, (ii) an importance of long accumulation periods (when storage gets depleted) and (iii) SPI being more important than SPEI to explain drought impacts. This last point could be explained by the fact that low precipitation in region N leads to the actual evapotranspiration (AET) to be water limited (i.e. AET < PET), meaning SPEI may be less closely linked to agrometeorological conditions. However, this is also the case for region W and C, and to lesser extent NE as well, where precipitation is also low, but in these other regions, SPEI's importance is generally dominant (Fig. 5c). Therefore, it is likely that the dominant importance of SPI in region N is linked to the reliance of agriculture on water storage for irrigation in this region, particularly for corn S2, which is planted in the dry season and relies heavily on irrigation (Fig. 10). Therefore, a deficit in rainfall (and consequent depleted storage) will have a strong impact on crop yield. For regions where rainfed crops dominate, shorter droughts can cause impacts, and SPEI becomes a stronger explanatory variable, given the effect of the increased evaporative demand. This was observed in regions E and NE, where SPEI indicators had high importance, whereas in regions N and C, SPI and TCI were more important for understanding the impacts of droughts on cassava yield. These results agree with previous studies which found strong relationships between SPEI and crop yield in the Mun River basin located in the NE region (Khadka et al., 2021; Prabnakorn et al., 2018).
Region N was the only region where we successfully built RF models for five crops (cassava, corn S1, corn S2, mixed corn, paddy rice). This was due to a combination of a lack and/or low quality of yield data, limited crop area, relationships being too complicated (with factors other than drought affecting crop yield), or indicators not being related to impacts in other regions. The comparison of the feature importance for these five models in the N region provides insight into the differences between crops. Both short- and long-duration droughts are important for all the crops simulated, demonstrated by the presence of indicators with 1- to 12-month accumulation periods for each crop. However, there was a higher prominence of short-accumulation-period indicators for cassava than other crops, particularly corn (all types). Cassava has the longest crop calendar (12 months), therefore having a higher chance of experiencing drought during the growing period. Other crops have shorter cycles compared to cassava (4–5.5 months for paddy rice, 4 months for both corn S1 and S2). Also, cassava is the least water-demanding crop of the list (irrigation requirement of around 20 m3 t−1 in the wet season and 65 m3 t−1 in the dry season, Gheewala et al., 2014). This explains the comparatively lower importance of long-accumulation-period indicators for cassava (Fig. 10), given the decreased reliance on water storage, especially compared with the most water-intensive crops, such as paddy rice (irrigation requirement of 520 m3 t−1 during the wet season and 1140 m3 t−1 in the dry season) and corn S2 (irrigation requirement of 850 m3 t−1 in the dry season). Whereas SPI were important for simulating productivity of corn and paddy rice in the N region, SPEI, TCI and VCI were more important in simulating cassava productivity than in other crops. These differences in results between regions and crops demonstrate the importance of having region- and crop-specific policies, actions and indicators for drought monitoring.
4.2 Temporal- and crop-specific variation in indicator-to-impact relationships
When we look at seasonal differences, we observe that SPI a has higher correlation with yield during the wet season, whereas SPEI is generally more correlated during the dry season (Figs. 5c and 6c). This suggests the importance of accounting for both temperature and evapotranspiration in dry season monitoring. We also observe that the highest correlations are for longer accumulation periods in the wet season compared to the dry season. This might be because longer wet season accumulation periods also include the information of the preceding dry season. The N region paddy rice RF model exhibited the high importance of indicators accumulated for the second half of the year (Fig. 10). Rice can be harvested twice or even three times per year in certain regions. The main rice crop cycle has its growing season between June and December, a large part of which occurs during the wet season. The RF model for paddy rice in region N exhibits high importance during this period (Fig. 10).
The RF models show spatial variations in the strength of relationships between crop yields and different accumulation periods, with longer accumulations assigned higher importance in region N, compared to other regions (Figs. 8 and 9), and this is also evident in the correlation analysis plot (Fig. S7). This might partly be explained by the presence of major dams in that region, which can mitigate the effect of short droughts (LePoer, 1987). The comparison of feature importance for cassava and paddy in region N (Fig. 9) supports the idea that different indicators and periods are important for each crop.
Cassava models for regions NE and E exhibit importance during October–March (Fig. 9), which overlaps with the dry season. Cassava is usually planted in April–June, and its yield is known to be sensitive to water stress in early stages of growth, corresponding to root initiation and bulking (Connor et al., 1981; Okogbenin et al., 2013; Oliveira et al., 1982). This seasonal pattern is not seen as clearly for regions N and C and is most probably explained by the fact that these two regions rely heavily on irrigation as opposed to regions NE and E, which are mainly rainfed.
Paddy rice's critical period for drought stress – which will have a severe effect on crop yield – is the early stages of germination, the seedling stage and also the flowering period (Farooq et al., 2012; Kadam et al., 2017; Mishra and Panda, 2017; Yang et al., 2019). However, it should be noted that this effect varies significantly depending on the specific crop variety, and the increasing adoption of drought-resistant varieties mitigates the impacts. The RF model for paddy rice in region N (Fig. 10) shows important features overlapping with these critical periods, in particular the early stage of the germination and seedling.
The critical period for drought for corn (all types) is in the early period of the growing season, with water stress after the anthesis (flowering) having no significant impact on crop yield (Pradawet et al., 2023). However, no distinct seasonality is observed in feature importance for corn in region N (Fig. S6), with a high prominence of long accumulation periods, despite corn being grown within mostly one season. This most likely reflects the dependence on irrigation of this crop in region N. However, in region E, which is dominated by rainfed crops, feature importance is concentrated around this critical period (June–October, Fig. S6).
We were not able to build RF models able to simulate longan productivity. The lack of model skill could be due to several factors. Firstly, unlike the other crops studied here, longan is a tree, and the effect of drought might be more complex. Secondly, longan is not grown extensively in comparison to the other crops, and the resulting lack of data might make it hard to identify patterns. And finally, longan trees are particularly sensitive to drought during the flowering and early fruit development stages (Menzel and Waite, 2005), which coincides with the dry season in Thailand, making this crop completely reliant on irrigation for production (Spreer et al., 2013). Irrigation mitigates the effects of drought, making it more difficult to model direct effects of meteorological droughts on crop production.
For the crops where it was possible to build an RF model, the analysis of the temporal variation in feature importance and the indicator-to-impact relationships provide insights into critical periods of the year for early warning of impacts and relevant accumulation periods. Specifically, these are periods of interest when dry conditions could lead to impacts.
4.3 Crop vs. forest
Clear differences in how drought impacts crops versus natural vegetation (forest) were observed. Firstly, a geographic distinction between the north (regions N, NE, W, C and E) and the south (region S) and secondly, temporal differences between wet and dry seasons suggest that drought management will be different between regions and the time of year. The summary of these differences can be found in Table 2, which also indicates the most relevant drought indicator to be used in a DMEW context for each land use type, season and region.
Table 2Summary of main findings on the relationship between drought indicators and drought impacts, as well as differences between land cover, region and season. North: regions N, NE, W, C and E; south: region S.
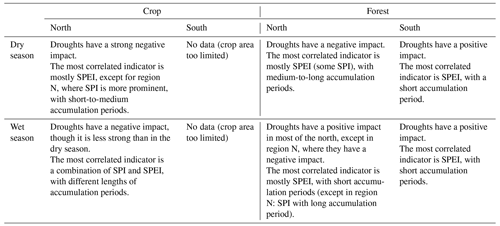
For forests in the north, droughts during the dry season will limit vegetation growth. This is in line with previous studies on dendrochronology, which have found that moisture availability in the pre-monsoon season is the predominant climatic factor controlling vegetation growth of tree species in tropical Southeast Asia, though there are important differences between species (e.g. Buckley et al., 1995; Rakthai et al., 2020; Sano et al., 2008). Generally, the indicator showing the highest correlation with impacts is for the longer accumulation period for forests than for crops, suggesting that shorter droughts will have impacts on crops, whereas only longer droughts will affect forests. The higher resilience to droughts of forests compared to crops is at least partially explained by the deeper root systems of forest trees, allowing them to extract water from deeper layers of the soil (Bréda et al., 2006; Schenk and Jackson, 2002).
During the wet season in the north and year round in the south, forests do not suffer from drought (negative correlation), and short droughts might even contribute positively to vegetation growth. Roebroek et al. (2020) produced a global distribution of hydrologic controls on forest growth. For Thailand, the forest growth in the south is mainly energy limited (solar radiation), rather than water limited. Hence short droughts, which are associated with increased radiation (due to decreased cloud cover), can have a beneficial effect on the forest.
The north of Thailand is a mixture of water-limited areas (water is the limiting factor for vegetation growth) – which explains the negative effect of droughts during the dry season – and oxygen-limited areas (when growth is limited by the availability of oxygen by the root, often due to flooding), which in Thailand is typical during the wet season. This explains the positive effect that short droughts have on forests during the wet season in the north (less flooding).
For crops, droughts have a negative impact both in the dry and wet seasons, though the effects during the dry season are stronger. The correlation could only be derived in the north, as the south is dominated by forested areas. Although SPEI shows the highest correlation with crop yields in most cases in the north, SPI is more prominent in region N. This, combined with the relatively high correlation between SPI and crop yields in region N during the wet season (Fig. S7), explains the higher importance placed on SPI compared to SPEI in the RF results. This can be explained by the higher dependency on storage and irrigation in region N than in the rest of the country.
4.4 Limitations
Though this study provides important new insight into the relationship between drought indicators and drought impacts in Thailand, some limitations should be acknowledged.
Firstly, there are limitations due to the imperfect nature of the data. By averaging VIs at a province level and correlating these values with crop yield from the dominant crop within that region, we inevitably introduce some noise, especially in provinces with a varied range of cultivated crops. However, a detailed map of crop distribution was not available. Therefore, the simplified approach taken here of using a land cover mask differentiating cropland from forests (but with no distinction between crops) was the best approach possible with the available data.
In addition, the time series available to carry out our analysis are short (15 years), which makes them more susceptible to noise, and also means that fewer drought events are available to learn from. Nevertheless, the study period (1984–2019) does include some of the major recent drought events such as 1990–1993, 1997, 2005, 2008 and 2015–2016.
Secondly, some limitations come from the methods we have used. Different factors can cause a trend (e.g. climate change, policy change, improvement in agricultural practice, etc.). However, we have applied a simple detrending approach (linear regression), which assumes that the trend is linear. In addition, the short length of the record makes it difficult to identify any trend. Another methodological limitation is our use of correlations to link drought indicators to drought impacts. Correlations can only explain linear relationships. However, the reality can be more complex, especially when looking at precipitation, where both extremes (droughts and floods) can have similar effects on crop yield loss. RFs are powerful tools for producing predictive models from data, but they are considered “black boxes”, since they do not explicitly extract the relationships between input features and the predicted outcomes. However, RFs can aid in the interpretation of the model through the analysis of feature importance, which identifies the most influential variables in making predictions. In addition, we only consider drought indicators and VIs as input variables, but many other factors can influence crop yield, such as floods, low temperature, disease, policy changes, farming practices, etc. This might also partially explain the relatively low performance of some of the RF models. Lastly, another limitation of using data-driven models such as RFs is the need for a large amount of data needed to train the model effectively. In our study, we had a relatively short period of data available, which limited the amount of data available for training the models. As a result, the models may not have been able to accurately capture the full range of conditions that could occur in the real world. For example, for species such as longan, which are more susceptible to long drought events, the limited instances of these events in our training data may have affected the model's ability to accurately predict impacts.
4.5 Future work
In this study, we used RF models primarily to analyse the relationships between drought indicators and impacts and to identify the relative importance and timing of relevant indicators for impacts on crops and forests. While the main focus of our analysis was on feature importance, our analysis also demonstrated the potential of RFs to simulate unseen data, which suggests they could be used for impact prediction. With further work, such as addressing the limitations discussed above, these models could be used for DMEWSs, support and compensation schemes, long-term planning, etc.
Furthermore, alternative methods could be explored and compared with the ones used here. Simpler approaches could provide simpler interpretation, such as the logistic regression (model diagnosis and equifinality/extrapolation). Due to the linear additive nature of logistic regression, it can be used to identify thresholds at which drought impacts are expected (Bachmair et al., 2016b; Parsons et al., 2019). However, for that same reason, it can only account for the probability of impacts increasing as conditions get drier or wetter, not both. Given that Thailand suffers from both floods and droughts, more complex models capable of capturing this non-linearity would be more suitable.
The RF models developed here offer promising results but could be compared to more sophisticated approaches. Machine learning/deep learning algorithms (artificial intelligence, AI) and Bayesian inference techniques are currently two rapidly developing areas of research and are increasingly used in environmental science. AI is very effective in finding patterns and connections within large volumes of multi-source spatio-temporal information, while Bayesian models are well suited for modelling complex spatio-temporal variations and capturing uncertainties. Shen et al. (2019) used a deep learning technique (artificial neural network) to build a drought monitoring model in China, whereas Bouras et al. (2021) developed a crop yield forecasting tool based on eXtreme Gradient Boost (XGBoost) in Morocco. Salakpi et al. (2022), on the other hand, used a dynamic hierarchical Bayesian approach for forecasting vegetation conditions in Kenya. With the burgeoning of new and increasingly complex methods, an assessment of the most suited approach in the context of DMEWS would be highly valuable.
In this study, we used a combination of traditional statistical approaches and machine learning techniques to analyse the relationship between drought indicators and drought impacts on vegetation and crops. These approaches are relatively novel in environmental science, particularly in the Southeast Asian context, bridging the gap between hazard and vulnerability by incorporating observed drought impact data.
Firstly, we carried out a correlation analysis to study the link between meteorological drought indicators (SPI and SPEI at different accumulation periods) and remote sensing vegetation indices (VCI and VHI) used as a proxy for crop yield and forest growth. Our analysis shows that these links in Thailand vary greatly depending on the land use (crops vs. forests), season (wet vs. dry) and geographical region, as does the type of droughts (short vs. long duration, with or without high temperature), which causes the most damaging impacts. Some of the main findings are that droughts have a negative effect on crops during both wet and dry seasons, though the lengths of the droughts having the most impact differ between seasons (shorter droughts during the dry season). Results also highlighted that short droughts can have a beneficial effect on forest growth in the wettest areas of the country and during the wet season.
Secondly, we built a series of random forest models to estimate crop productivity for each crop and region separately. This allowed a more in-depth analysis of the importance of the different drought indicators in a crop-specific way. The analysis of feature importance has teased out seasonal patterns of feature importance for individual crops, often linked to their growing season, though the presence of irrigation systems in some of the regions (regions N and C) removes some of that seasonality. This new knowledge about the importance of specific drought indicators to predict drought impacts for targeted crops and regions could be used to improve drought monitoring and early warning systems in Thailand, particularly for the agricultural sector, which is both economically important to Thailand as well as vulnerable to the drought, as it will allow tailored monitoring of the most relevant indicators for individual crops/regions. The best indicators to monitor vary in space and time, as well as by land use and crop type. The work presented in this paper can provide guidance and inform water managers of the best indicator to use spatio-temporally, which ultimately will contribute to increasing Thailand's resilience to and preparedness for droughts. Furthermore, the methodology can be replicated in other areas of the world to help build this knowledge in other countries aiming to increase their resilience to droughts.
All code and data are available upon request. Open data used in this study are listed in Table 1.
The supplement related to this article is available online at: https://doi.org/10.5194/nhess-23-2419-2023-supplement.
MT: conceptualisation, formal analysis, investigation, methodology, supervision, validation, visualisation, writing (original draft), writing (review and editing). ME: conceptualisation, formal analysis, investigation, methodology, validation, visualisation, writing (original draft). EM: data curation, formal analysis, investigation, methodology, validation, visualisation, writing (original draft). LJB: conceptualisation, writing (review and editing). TC: data curation. CE: funding acquisition, writing (review and editing). DG: conceptualisation, methodology, writing (review and editing). JH: conceptualisation, funding acquisition, supervision, writing (review and editing). IH: conceptualisation, funding acquisition, writing (review and editing). LP: funding acquisition, writing (review and editing). SP: conceptualisation, funding acquisition, project administration, resources, writing (review and editing). DRV: funding acquisition, writing (review and editing). SV: funding acquisition, project administration, writing (review and editing).
The contact author has declared that none of the authors has any competing interests.
Publisher’s note: Copernicus Publications remains neutral with regard to jurisdictional claims in published maps and institutional affiliations.
This article is part of the special issue “Drought, society, and ecosystems (NHESS/BG/GC/HESS inter-journal SI)”. It is a result of the Panta Rhei Drought in the Anthropocene workshop 2022, Uppsala, Sweden, 29–30 August 2022.
This project was funded through the Cranfield University Global Challenges Research Fund (grant no. QR 2020/21) and a joint grant from NERC (Natural Environment Research Council, UK), grant number NE/S003223/1, and TSRI (Thailand Science Research and Innovation), grant number RDG6130017, for the STAR project (Strengthening Thailand's Agricultural drought Resilience). The funding was also complemented by UKCEH's Land Air Water International Science (LAWIS) and NC international programmes (NE/X006247/1) delivering national capability, both funded by NERC. The authors would also like to express their gratitude to the two reviewers, Samuel Jonson Sutanto and Veit Blauhut, for their valuable feedback which has contributed to improve the quality of the paper.
This research has been supported by the Natural Environment Research Council (grant nos. NE/S003223/1 and NE/X006247/1), the Global Challenges Research Fund (grant no. QR 2020/21), and the Thailand Science Research and Innovation (grant no. RDG6130017).
This paper was edited by Anne Van Loon and reviewed by Samuel Jonson Sutanto and Veit Blauhut.
Anyamba, A. and Tucker, C. J.: Historical perspectives on AVHRR NDVI and vegetation drought monitoring, Remote Sensing of Drought: Innovative Monitoring Approaches, edited by: Wardlow, B. D., Anderson, M. C., Verdin, J. P., CRC Press, New York, United States of America, 2023–2051, https://doi.org/10.1201/b11863, 2012.
Arunrat, N., Sereenonchai, S., Chaowiwat, W., and Wang, C.: Climate change impact on major crop yield and water footprint under CMIP6 climate projections in repeated drought and flood areas in Thailand, Sci. Total Environ., 807, 150741, https://doi.org/10.1016/j.scitotenv.2021.150741, 2022.
Bachmair, S., Stahl, K., Collins, K., Hannaford, J., Acreman, M., Svoboda, M., Knutson, C., Smith, K. H., Wall, N., Fuchs, B., Crossman, N. D., and Overton, I. C.: Drought indicators revisited: the need for a wider consideration of environment and society, WIREs Water, 3, 516–536, https://doi.org/10.1002/wat2.1154, 2016a.
Bachmair, S., Svensson, C., Hannaford, J., Barker, L. J., and Stahl, K.: A quantitative analysis to objectively appraise drought indicators and model drought impacts, Hydrol. Earth Syst. Sci., 20, 2589–2609, https://doi.org/10.5194/hess-20-2589-2016, 2016b.
Bachmair, S., Tanguy, M., Hannaford, J., and Stahl, K.: How well do meteorological indicators represent agricultural and forest drought across Europe?, Environ. Res. Lett., 13, 034042, https://doi.org/10.1088/1748-9326/aaafda, 2018.
Blair, G. S., Henrys, P., Leeson, A., Watkins, J., Eastoe, E., Jarvis, S., and Young, P. J.: Data Science of the Natural Environment: A Research Roadmap, Front. Environ. Sci., 7, 121, https://doi.org/10.3389/fenvs.2019.00121, 2019.
Bolton, D. K. and Friedl, M. A.: Forecasting crop yield using remotely sensed vegetation indices and crop phenology metrics, Agr. Forest Meteorol., 173, 74–84, https://doi.org/10.1016/j.agrformet.2013.01.007, 2013.
Bouras, E. H., Jarlan, L., Er-Raki, S., Balaghi, R., Amazirh, A., Richard, B., and Khabba, S.: Cereal Yield Forecasting with Satellite Drought-Based Indices, Weather Data and Regional Climate Indices Using Machine Learning in Morocco, Remote Sens., 13, 3101, https://doi.org/10.3390/rs13163101, 2021.
Bréda, N., Huc, R., Granier, A., and Dreyer, E.: Temperate forest trees and stands under severe drought: a review of ecophysiological responses, adaptation processes and long-term consequences, Ann. For. Sci., 63, 625–644, 2006.
Buckley, B. M., Barbetti, M., Watanasak, M., Arrigo, R. D., Boonchirdchoo, S., and Sarutanon, S.: Dendrochronological Investigations in Thailand, IAWA J., 16, 393–409, https://doi.org/10.1163/22941932-90001429, 1995.
Byer, S. and Jin, Y.: Detecting Drought-Induced Tree Mortality in Sierra Nevada Forests with Time Series of Satellite Data, Remote Sens., 9, 929, https://doi.org/10.3390/rs9090929, 2017.
CFE-DMHA: THAILAND Disaster Management Reference Handbook, Center for Excellence in Disaster Management & Humanitarian Assistance, ISBN 978-971-955429-955433-955427, https://reliefweb.int/report/thailand/disaster-management-reference-handbook-thailand-january-2022 (last access: 22 June 2023), 2022.
Coelho, A. P., de Faria, R. T., Leal, F. T., Barbosa, J. D. A., and Rosalen, D. L.: Validation of white oat yield estimation models using vegetation indices. Basic areas, Bragantia, 79, 2, https://doi.org/10.1590/1678-4499.20190387, 2020.
Connor, D. J., Cock, J. H., and Parra, G. E.: Response of cassava to water shortage I. Growth and yield, Field Crop. Res., 4, 181–200, https://doi.org/10.1016/0378-4290(81)90071-X, 1981.
Didan, K.: MYD13A1 MODIS/Aqua Vegetation Indices 16-day L3 Global 500m SIN Grid V006, NASA EOSDIS Land Processes DAAC [data set], https://doi.org/10.5067/MODIS/MYD13A1.006, 2015a.
Didan, K.: MOD13A1 MODIS/Terra Vegetation Indices 16-Day L3 Global 500m SIN Grid V006, NASA EOSDIS Land Processes DAAC [data set], https://doi.org/10.5067/MODIS/MOD13A1.006, 2015b.
Dubey, S. K., Gavli, A. S., Yadav, S. K., Sehgal, S., and Ray, S. S.: Remote Sensing-Based Yield Forecasting for Sugarcane (Saccharum officinarum L.) Crop in India, J. Indian Soc. Remot., 46, 1823–1833, https://doi.org/10.1007/s12524-018-0839-2, 2018.
FAO: Irrigation in Asia in Figures, Water reports, Food and Agriculture Organization of the United Nations, https://www.fao.org/1023/I9275EN/i9275en.pdf (last access: 5 January 2023), 1999.
FAO: The impact of disasters and crises on agriculture and food security: 2021, Food and Agriculture Association of the United Nations, Rome, https://doi.org/10.4060/cb3673en, 2021.
Farooq, M., Hussain, M., Wahid, A., and Siddique, K. H. M.: Drought Stress in Plants: An Overview, in: Plant Responses to Drought Stress: From Morphological to Molecular Features, edited by: Aroca, R., Springer Berlin Heidelberg, Berlin, Heidelberg, 1–33, https://doi.org/10.1007/978-3-642-32653-0_1, 2012.
FFTC: Fruit Production, Marketing and Research and Development System in Thailand, Food and Fertilizer Technology Cente for the Asian and Pacific Region, https://www.fftc.org.tw/en/publications/main/1912 (last access: 19 January 2023), 2015.
Friedl, M. and Sulla-Menashe, D.: MCD12Q1 MODIS/Terra+Aqua Land Cover Type Yearly L3 Global 500m SIN Grid V006, NASA EOSDIS Land Processes DAAC [data set], https://doi.org/10.5067/MODIS/MCD12Q1.006, 2019.
García-León, D., Contreras, S., and Hunink, J.: Comparison of meteorological and satellite-based drought indices as yield predictors of Spanish cereals, Agr. Water Manage., 213, 388–396, https://doi.org/10.1016/j.agwat.2018.10.030, 2019.
Gheewala, S. H., Silalertruksa, T., Nilsalab, P., Mungkung, R., Perret, S. R., and Chaiyawannakarn, N.: Water Footprint and Impact of Water Consumption for Food, Feed, Fuel Crops Production in Thailand, Water, 6, 1698–1718, 2014.
Hariadi, M. H., van der Schrier, G., Steeneveld, G.-J., Sutanto, S., Sutanudjaja, E., Ratri, D. N., Sopaheluwakan, A., and Klein Tank, A.: A high-resolution perspective of extreme rainfall and river flow under extreme climate change in Southeast Asia, Hydrol. Earth Syst. Sci. Discuss. [preprint], https://doi.org/10.5194/hess-2023-14, in review, 2023.
Hobeichi, S., Abramowitz, G., Evans, J. P., and Ukkola, A.: Toward a Robust, Impact-Based, Predictive Drought Metric, Water Resour. Res., 58, e2021WR031829, https://doi.org/10.1029/2021WR031829, 2022.
ICID: Thailand, International Commission on Irrigation & Drainage, Thai National Committee on Irrigation and Drainage (THAICID), https://www.icid.org/v_thailand.pdf (last access: 5 June 2022), 2020.
Ikeda, M. and Palakhamarn, T.: Economic Damage from Natural Hazards and Local Disaster Management Plans in Japan and Thailand, ERIA Discussion Paper Series, No. 346, ERIA-DP-2020-2019, Economic Research Institute for ASEAN and East Asia, https://www.eria.org/research/economic-damage-from-natural-hazards-and-local-disaster-management-plans-in-japan-and-thailand/ (last access: 22 June 2023), 2020.
IPCC: Climate Change 2021: The Physical Science Basis. Contribution of Working Group I to the Sixth Assessment Report of the Intergovernmental Panel on Climate Change, edited by: Masson-Delmotte, V., Zhai, P., Pirani, A., Connors, S. L., Péan, C., Berger, S., Caud, N., Chen, Y., Goldfarb, L., Gomis, M. I., Huang, M., Leitzell, K., Lonnoy, E., Matthews, J. B. R., Maycock, T. K., Waterfield, T., Yelekçi, O., Yu, R., and Zhou, B., Cambridge University Press, Cambridge, United Kingdom and New York, NY, USA, 2391 pp., https://report.ipcc.ch/ar6/wg1/IPCC_AR6_WGI_FullReport.pdf (last access: 4 July 2023), 2021.
IPCC: Climate Change 2022: Impacts, Adaptation and Vulnerability. Contribution of Working Group II to the Sixth Assessment Report of the Intergovernmental Panel on Climate Change, edited by: Pörtner, H.-O., Roberts, D. C., Tignor, M., Poloczanska, E. S., Mintenbeck, K., Alegría, A., Craig, M., Langsdorf, S., Löschke, S., Möller, V., Okem, A., and Rama, B., Cambridge University Press. Cambridge University Press, Cambridge, UK and New York, NY, USA, 3056 pp., https://doi.org/10.1017/9781009325844, 2022.
Jain, S. K., Keshri, R., Goswami, A., Sarkar, A., and Chaudhry, A.: Identification of drought-vulnerable areas using NOAA AVHRR data, Int. J. Remote Sens., 30, 2653–2668, https://doi.org/10.1080/01431160802555788, 2009.
Jiao, W., Zhang, L., Chang, Q., Fu, D., Cen, Y., and Tong, Q.: Evaluating an Enhanced Vegetation Condition Index (VCI) Based on VIUPD for Drought Monitoring in the Continental United States, Remote Sens., 8, 224, https://doi.org/10.3390/rs8030224, 2016.
Kadam, N. N., Tamilselvan, A., Lawas, L. M. F., Quinones, C., Bahuguna, R. N., Thomson, M. J., Dingkuhn, M., Muthurajan, R., Struik, P. C., Yin, X., and Jagadish, S. V. K.: Genetic Control of Plasticity in Root Morphology and Anatomy of Rice in Response to Water Deficit, Plant Physiol., 174, 2302–2315, https://doi.org/10.1104/pp.17.00500, 2017.
Khadka, D., Babel, M. S., Shrestha, S., Virdis, S. G. P., and Collins, M.: Multivariate and multi-temporal analysis of meteorological drought in the northeast of Thailand, Weather and Climate Extremes, 34, 100399, https://doi.org/10.1016/j.wace.2021.100399, 2021.
Kogan, F., Salazar, L., and Roytman, L.: Forecasting crop production using satellite-based vegetation health indices in Kansas, USA, Int. J. Remote Sens., 33, 2798–2814, https://doi.org/10.1080/01431161.2011.621464, 2012.
Kogan, F. N.: Global Drought Watch from Space, B. Am. Meteorol. Soc., 78, 621–636, https://doi.org/10.1175/1520-0477(1997)078<0621:GDWFS>2.0.CO;2, 1997.
Lacombe, G., Polthanee, A., and Trébuil, G.: Long-term change in rainfall distribution in Northeast Thailand: will cropping systems be able to adapt?, Cah. Agric., 26, 25001, https://doi.org/10.1051/cagri/2017006, 2017.
LePoer, B. L.: Thailand: a country study, Federal Research Division, Washington, D.C., USA, 60–65, OCLC 44366465, https://www.loc.gov/item/88600485/ (last access: 4 July 2023), 1987.
Liu, W. T. and Kogan, F. N.: Monitoring regional drought using the Vegetation Condition Index, Int. J. Remote Sens., 17, 2761–2782, https://doi.org/10.1080/01431169608949106, 1996.
Lloyd-Hughes, B.: The impracticality of a universal drought definition, Theor. Appl. Climatol., 117, 607–611, https://doi.org/10.1007/s00704-013-1025-7, 2014.
Martin, S. A. and Ritchie, R. J.: Sourcing Thai geography literature for ASEAN and international education, Singapore J. Trop. Geo., 41, 61–85, https://doi.org/10.1111/sjtg.12296, 2020.
Maselli, F., Romanelli, S., Bottai, L., and Maracchi, G.: Processing of GAC NDVI data for yield forecasting in the Sahelian region, Int. J. Remote Sens., 21, 3509–3523, https://doi.org/10.1080/014311600750037525, 2000.
McKee, T. B., Doesken, N. J., and Kleist, J.: The relationship of drought frequency and duration to time scales, Eighth Conference on Applied Climatology, 17–22 January 1993, Anaheim, California, American Meteorological Society, https://www.droughtmanagement.info/literature/AMS_Relationship_Drought_Frequency_Duration_Time_Scales_1993.pdf (last access: 4 July 2023), 1993.
Menzel, C. M. and Waite, G. K.: Litchi and longan, botany, production and uses, CABI Publishing, Oxfordshire/Cambridge, MA, ISBN 9780851996967, 2005.
Mishra, S. S. and Panda, D.: Leaf Traits and Antioxidant Defense for Drought Tolerance During Early Growth Stage in Some Popular Traditional Rice Landraces from Koraput, India, Rice Science, 24, 207–217, https://doi.org/10.1016/j.rsci.2017.04.001, 2017.
Mongkolsawat, C., Thirangoon, P., Suwanwerakamtorn, R., Karladee, N., Paiboonsak, S., and Champathet, P.: An evaluation of drought risk area in Northeast Thailand using remotely sensed data and GIS, Asian Journal of Geoinformatics, 1, 33–43, 2001.
NESDC: Statistics on Thailand's drought situation for the period 1989–2021, National Economic and Social Development Council, Data collated from annual report of the Disaster Data Center DDPM, https://www.nesdc.go.th/ewt_dl_link.php?nid=9787 (last access: 23 June 2023), 2021.
OAE: Agricultural production data, Office of Agricultural Economics, https://www.ceicdata.com/en/thailand/agricultural-production-index-office-of-agricultural-economics (last access: 23 June 2023), 2021.
OAE: Agricultural Statistics of Thailand 2021, Office of Agricultural Economics, Ministry of Agriculture and Cooperatives, Bangkok, Thailand, https://www.oae.go.th/assets/portals/1/files/jounal/2565/yearbook2564.pdf (last access: 23 June 2023), 2022.
OECD: OECD Economic Surveys Economic Assessment: Thailand, Organisation for Economic Co-operation and Development, https://www.oecd.org/economy/thailand-economic-snapshot/ (last access: 23 June 2023), 2020.
Okogbenin, E., Setter, T., Ferguson, M., Mutegi, R., Ceballos, H., Olasanmi, B., and Fregene, M.: Phenotypic approaches to drought in cassava: review, Front. Physiol., 4, https://doi.org/10.3389/fphys.2013.00093, 2013.
Oliveira, S. L., Macedo, M. M. C., and Porto, M. C. M.: Effects of water stress on cassava root production, Pesquia Agropecuria Brasil, 17, 121–124, https://agris.fao.org/agris-search/search.do?recordID=US201302182137 (last access: 31 January 2023), 1982.
Parsons, D. J., Rey, D., Tanguy, M., and Holman, I. P.: Regional variations in the link between drought indices and reported agricultural impacts of drought, Agr. Syst., 173, 119–129, https://doi.org/10.1016/j.agsy.2019.02.015, 2019.
Pearson, K.: Notes on the history of correlation, Biometrika, 13, 25–45, https://doi.org/10.1093/biomet/13.1.25, 1920.
Prabnakorn, S., Maskey, S., Suryadi, F. X., and de Fraiture, C.: Rice yield in response to climate trends and drought index in the Mun River Basin, Thailand, Sci. Total Environ., 621, 108–119, https://doi.org/10.1016/j.scitotenv.2017.11.136, 2018.
Pradawet, C., Khongdee, N., Pansak, W., Spreer, W., Hilger, T., and Cadisch, G.: Thermal imaging for assessment of maize water stress and yield prediction under drought conditions, J. Agron. Crop Sci., 209, 56–70, https://doi.org/10.1111/jac.12582, 2023.
Pyper, B. J. and Peterman, R. M.: Comparison of methods to account for autocorrelation in correlation analyses of fish data, Can. J. Fish. Aquat. Sci., 55, 2127–2140, https://doi.org/10.1139/f98-104, 1998.
Rakthai, S., Fu, P.-L., Fan, Z.-X., Gaire, N. P., Pumijumnong, N., Eiadthong, W., and Tangmitcharoen, S.: Increased Drought Sensitivity Results in a Declining Tree Growth of Pinus latteri in Northeastern Thailand, Forests, 11, 361, https://doi.org/10.3390/f11030361, 2020.
RFD: Forest area of Thailand, 1973–2018, Royal Forest Department (RFD), http://forestinfo.forest.go.th/Content.aspx?id=72 (last access: 5 June 2022), 2022.
Roebroek, C. T. J., Melsen, L. A., Hoek van Dijke, A. J., Fan, Y., and Teuling, A. J.: Global distribution of hydrologic controls on forest growth, Hydrol. Earth Syst. Sci., 24, 4625–4639, https://doi.org/10.5194/hess-24-4625-2020, 2020.
Running, S., Mu, Q., and Zhao, M.: MOD16A2 MODIS/Terra Net Evapotranspiration 8-Day L4 Global 500m SIN Grid V006, NASA EOSDIS Land Processes DAAC [data set], https://doi.org/10.5067/MODIS/MOD16A2.006, 2017.
Salakpi, E. E., Hurley, P. D., Muthoka, J. M., Bowell, A., Oliver, S., and Rowhani, P.: A dynamic hierarchical Bayesian approach for forecasting vegetation condition, Nat. Hazards Earth Syst. Sci., 22, 2725–2749, https://doi.org/10.5194/nhess-22-2725-2022, 2022.
Sa-nguansilp, C., Wijitkosum, S., and Sriprachote, A.: Agricultural Drought Risk Assessment in Lam Ta Kong Watershed, International Journal of Geoinformatics, 13, 46, https://journals.sfu.ca/ijg/index.php/journal/article/view/1090 (last access: 23 June 2023), 2017.
Sano, M., Buckley, B. M., and Sweda, T.: Tree-ring based hydroclimate reconstruction over northern Vietnam from Fokienia hodginsii: eighteenth century mega-drought and tropical Pacific influence, Clim. Dynam., 33, 331–340, https://doi.org/10.1007/s00382-008-0454-y, 2008.
Sanoamuang, L. and Dabseepai, P.: Diversity, Distribution, and Habitat Occurrence of the Diaptomid Copepods (Crustacea: Copepoda: Diaptomidae) in Freshwater Ecosystems of Thailand, Water, 13, 2381, https://doi.org/10.3390/w13172381, 2021.
Schenk, H. J. and Jackson, R. B.: The Global Biogeography Of Roots, Ecol. Monogr., 72, 311–328, https://doi.org/10.1890/0012-9615(2002)072[0311:TGBOR]2.0.CO;2, 2002.
Shams Esfandabadi, H., Ghamary Asl, M., Shams Esfandabadi, Z., Gautam, S., and Ranjbari, M.: Drought assessment in paddy rice fields using remote sensing technology towards achieving food security and SDG2, Brit. Food J., 124, 4219–4233, https://doi.org/10.1108/BFJ-08-2021-0872, 2022.
Shen, R., Huang, A., Li, B., and Guo, J.: Construction of a drought monitoring model using deep learning based on multi-source remote sensing data, Int. J. Appl. Earth Obs., 79, 48–57, https://doi.org/10.1016/j.jag.2019.03.006, 2019.
Singh, R. P., Roy, S., and Kogan, F.: Vegetation and temperature condition indices from NOAA AVHRR data for drought monitoring over India, Int. J. Remote Sens., 24, 4393–4402, https://doi.org/10.1080/0143116031000084323, 2003.
Smith, K. H., Svoboda, M., Hayes, M., Reges, H., Doesken, N., Lackstrom, K., Dow, K., and Brennan, A.: Local Observers Fill In the Details on Drought Impact Reporter Maps, B. Am. Meteorol. Soc., 95, 1659–1662, https://doi.org/10.1175/1520-0477-95.11.1659, 2014.
Smith, R., Adams, J., Stephens, D., and Hick, P.: Forecasting wheat yield in a Mediterranean-type environment from the NOAA satellite, Aust. J. Agr. Res., 46, 113–125, https://doi.org/10.1071/AR9950113, 1995.
Sowcharoensuk, C. and Marknual, C.: Severe drought: Agriculture sector takes direct hit and spillover effects on manufacturing supply chains, Bank of Ayudhya's Krungsri Research Intellingence Report, https://www.krungsri.com/getmedia/dc6db8a2-00d2-4c3b-bbd4-ebad9275193b/RI_Drought_200207_EN.pdf.aspx (last access: 23 June 2023), 2020.
Spreer, W., Schulze, K., Ongprasert, S., Wiriya-Alongkorn, W., and Müller, J.: Mango and Longan Production in Northern Thailand: The Role of Water Saving Irrigation and Water Stress Monitoring, in: Sustainable Land Use and Rural Development in Southeast Asia: Innovations and Policies for Mountainous Areas, edited by: Fröhlich, H. L., Schreinemachers, P., Stahr, K., and Clemens, G., Springer Berlin Heidelberg, Berlin, Heidelberg, 215–228, https://doi.org/10.1007/978-3-642-33377-4_6, 2013.
Stahl, K., Kohn, I., Blauhut, V., Urquijo, J., De Stefano, L., Acácio, V., Dias, S., Stagge, J. H., Tallaksen, L. M., Kampragou, E., Van Loon, A. F., Barker, L. J., Melsen, L. A., Bifulco, C., Musolino, D., de Carli, A., Massarutto, A., Assimacopoulos, D., and Van Lanen, H. A. J.: Impacts of European drought events: insights from an international database of text-based reports, Nat. Hazards Earth Syst. Sci., 16, 801–819, https://doi.org/10.5194/nhess-16-801-2016, 2016.
Sutanto, S. J., van der Weert, M., Wanders, N., Blauhut, V., and Van Lanen, H. A. J.: Moving from drought hazard to impact forecasts, Nat. Commun., 10, 4945, https://doi.org/10.1038/s41467-019-12840-z, 2019.
Thammachote, P. and Trichim, J. I.: The Impact of the COVID-19 Pandemic on Thailand's Agricultural Export Flows, Feed the Future report, The U.S. Government's Global Hunger & Food Security Initiative, https://www.canr.msu.edu/prci/PRCI-Research-Paper-4-Thailand_updated.pdf (last access: 29 January 2023), 2021.
Thavorntam, W. and Shahnawaz, S.: Evaluation of Drought in the North of Thailand using Meteorological and Satellite-Based Drought Indices, International Journal of Geoinformatics, 18, 13–26, https://doi.org/10.52939/ijg.v18i5.2367, 2022.
Thavorntam, W., Tantemsapya, N., and Armstrong, L.: A combination of meteorological and satellite-based drought indices in a better drought assessment and forecasting in Northeast Thailand, Nat. Hazards, 77, 1453–1474, https://doi.org/10.1007/s11069-014-1501-0, 2015.
Torres, P., Rodes-Blanco, M., Viana-Soto, A., Nieto, H., and García, M.: The Role of Remote Sensing for the Assessment and Monitoring of Forest Health: A Systematic Evidence Synthesis, Forests, 12, 1134, https://doi.org/10.3390/f12081134, 2021.
Tucker, C. J.: Red and photographic infrared linear combinations for monitoring vegetation, Remote Sens. Environ., 8, 127–150, https://doi.org/10.1016/0034-4257(79)90013-0, 1979.
UNDRR: The Sendai Framework for Disaster Risk Reduction 2015–2030, United Nations Office for Disaster Risk Reduction, 32 pp., https://www.undrr.org/publication/sendai-framework-disaster-risk-reduction-2015-2030 (last access: 23 June 2023), 2015.
UNDRR and ADCP: Disaster Risk Reduction in Thailand: Status Report 2020, UNDRR (United Nations Office for Disaster Risk Reduction) and ADCP (Asian Disaster Preparedness Center), Climate Change and Climate Risk Management, DRR Report, http://www.adpc.net/Igo/contents/Publications/publications-Details.asp?pid=1681#sthash.JBSoZQWU.dpbs (last access: 23 June 2023), 2020.
Unganai, L. S. and Kogan, F. N.: Southern Africa's recent droughts from space, Adv. Space Res.-Series, 21, 507–511, https://doi.org/10.1016/S0273-1177(97)00888-0, 1998.
Varawoot, V.: Historical Irrigation Development of Thailand, Irrigation Technology Research, Development Laboratory, Department of Irrigation Engineering, Kasetsart University, Kamphaengsaen campus, https://eng.kps.ku.ac.th/irre/slideshow/pdf/4.pdf (last access: 18 February 2023), 2016.
Venkatappa, M., Sasaki, N., Han, P., and Abe, I.: Impacts of droughts and floods on croplands and crop production in Southeast Asia – An application of Google Earth Engine, Sci. Total Environ., 795, 148829, https://doi.org/10.1016/j.scitotenv.2021.148829, 2021.
Vicente-Serrano, S. M., Beguería, S., and López-Moreno, J. I.: A Multiscalar Drought Index Sensitive to Global Warming: The Standardized Precipitation Evapotranspiration Index, J. Climate, 23, 1696–1718, https://doi.org/10.1175/2009jcli2909.1, 2010.
Wan, Z., Hook, S., and Hulley, G.: MOD11A2 MODIS/Terra Land Surface Temperature/Emissivity 8-Day L3 Global 1km SIN Grid V006, NASA EOSDIS Land Processes DAAC [data set], https://doi.org/10.5067/MODIS/MOD11A2.061, 2015.
Wang, Y., Lv, J., Hannaford, J., Wang, Y., Sun, H., Barker, L. J., Ma, M., Su, Z., and Eastman, M.: Linking drought indices to impacts to support drought risk assessment in Liaoning province, China, Nat. Hazards Earth Syst. Sci., 20, 889–906, https://doi.org/10.5194/nhess-20-889-2020, 2020.
WBG and ADB: Climate Risk Country Profile: Thailand (2021), The World Bank Group and the Asian Development Bank, https://www.adb.org/sites/default/files/publication/722251/climate-risk-country-profile-thailand.pdf (last access: 23 June 2023), 2021.
Wijitkosum, S.: Fuzzy AHP for drought risk assessment in Lam Ta Kong watershed, the north-eastern region of Thailand, Soil Water Res., 13, 218–225, 2018.
Wilhite, D. A. and Glantz, M. H.: Understanding: the Drought Phenomenon: The Role of Definitions, Water Int., 10, 111–120, https://doi.org/10.1080/02508068508686328, 1985.
WMO: WMO Atlas of Mortality and Economic Losses from Weather, Climate and Water Extremes (1970–2019), WMO-No. 1267, World Meteorological Organization (WMO), ISBN 978-992-963-11267-11265, https://library.wmo.int/index.php?lvl=notice_display&id=21930#.Y11294o_11263bP11262Ul (last access: 23 June 2023), 2014.
Yang, X., Wang, B., Chen, L., Li, P., and Cao, C.: The different influences of drought stress at the flowering stage on rice physiological traits, grain yield, and quality, Sci. Rep., 9, 3742, https://doi.org/10.1038/s41598-019-40161-0, 2019.
Yatagai, A., Kamiguchi, K., Arakawa, O., Hamada, A., Yasutomi, N., and Kitoh, A.: APHRODITE: Constructing a Long-Term Daily Gridded Precipitation Dataset for Asia Based on a Dense Network of Rain Gauges, B. Am. Meteorol. Soc., 93, 1401–1415, https://doi.org/10.1175/bams-d-11-00122.1, 2012.
Yoshida, K., Srisutham, M., Sritumboon, S., Suanburi, D., and Janjirauttikul, N.: Weather-induced economic damage to upland crops and the impact on farmer household income in Northeast Thailand, Paddy Water Environ., 17, 341–349, https://doi.org/10.1007/s10333-019-00729-y, 2019.