the Creative Commons Attribution 4.0 License.
the Creative Commons Attribution 4.0 License.
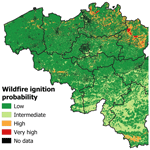
Wildfire ignition probability in Belgium
Arthur Depicker
Bernard De Baets
Jan Marcel Baetens
In recent decades, large wildfires have inflicted considerable damage on valuable Natura 2000 regions in Belgium. Despite these events and the general perception that global change will exacerbate wildfire prevalence, this has not been studied yet in the Belgian context. Therefore, the national government initiated the national action plan on wildfires in order to evaluate the wildfire risk, on the one hand, and the materials, procedures, and training of fire services, on the other hand.
This study focuses on the spatial distribution of the ignition probability, a component of the wildfire risk framework. In a first stage, we compile a historical wildfire database using (i) newspaper articles between 1994 and 2016 and (ii) a list of wildfire interventions between 2010 and 2013, provided by the government. In a second stage, we use a straightforward method relying on Bayes' rule and a limited number of covariates to calculate the ignition probability.
It appears that most wildfire-prone areas in Belgium are located in heathland where military exercises are held. The provinces that have the largest relative areas with a high or very high wildfire risk are Limburg and Antwerp. Our study also revealed that most wildfire ignitions in Belgium are caused by humans (both arson and negligence) and that natural causes such as lightning are rather scarce. Wildfire prevention can be improved by (i) excluding military activity in fire-prone areas during the fire season, (ii) improving collaboration with foreign emergency services, (iii) concentrating the dedicated resources in the areas that display the highest ignition probabilities, (iv) improving fire detection methods, and (v) raising more awareness among the public.
- Article
(13487 KB) - Full-text XML
- BibTeX
- EndNote
Every year, wildfires burn an astonishing 350–450 million ha of forest and grassland globally, an area corresponding to approximately 4 % of earth's land surface, Antarctica and Greenland not taken into account (Randerson et al., 2012; Giglio et al., 2010). The general perception is that wildfire frequency and damage are increasing due to more extreme weather events and altered precipitation and temperature patterns (National Wildlife Federation, 2008; IPCC, 2014; North et al., 2015; Doerr and Santin, 2016). Wildfires inflict physical and mental harm (Liu et al., 2014; Youssouf et al., 2014; Eisenman et al., 2015; Navarro et al., 2018) and damage infrastructure (Syphard et al., 2013; Penman et al., 2015).
Despite their increasing threat, wildfires in Belgium have not received any attention in literature. On the one hand, this gap can be justified by the lack of casualties and the low wildfire frequency, but, on the other hand, fires have been inflicting considerable damage to valuable nature areas (San-Miguel-Ayanz et al., 2012a). The latter has prompted the federal government to initiate a national action plan on wildfires, for which one of the objectives is to perform a wildfire risk assessment.
However, there is no unambiguous framework for assessing wildfire risk (Hardy, 2005; Miller and Ager, 2013; San-Miguel-Ayanz et al., 2017). Following the IPCC framework of natural hazard risk, the European Commission (EC) defines wildfire risk as a function of (i) hazard and (ii) vulnerability. The former refers to the occurrence of an incident and is a combination of fire ignition and spread. The second component, wildfire vulnerability, is a measure of the presence of ecological and socioeconomic assets that can be damaged by fire, and the extent to which one can anticipate, resist, cope with, or recover from this damage (IPCC, 2012; San-Miguel-Ayanz et al., 2017).
Within this study, we focus on the wildfire ignition probability and its spatial distribution. First, the study area is presented together with the spatial data, necessary for the assessment. Second, we introduce a method that relies on Bayes' rule and a limited number of covariates to assess the probability. In Sect. 3, the resulting ignition probability map (IPM) is presented, and lastly we discuss the results and include some recommendations for future wildfire management in Belgium.
2.1 Study area: Belgium
Belgium is a western European country and a member state of the European Union. It is bordered by France to the south, Luxembourg and Germany to the east, the Netherlands to the north, and the North Sea to the west. Belgium has a temperate maritime climate that is characterized by four distinct seasons: spring, summer, fall, and winter. It has a total area of approximately 30 528 km2 and a population of more than 11.2 million. The average population density is 363 inhabitants km−2, though the northern region, Flanders, is much more densely populated than the southern region, Wallonia (Fig. 1, 562 inh. km−2 versus 214 inh. km−2) (Belgian Federal Government, 2016).
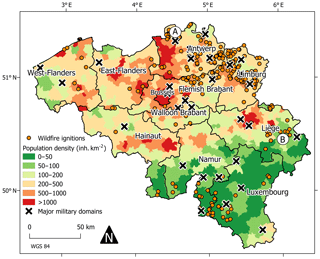
Figure 1Belgium, its 10 provinces, and the Brussels Capital Region. The map displays the wildfire ignitions in Belgium between 1994 and 2016 and the major military domains (Sect. 3.1). The population densities were provided by the NGI (http://www.ngi.be/NL/NL1-5-2.shtm, last access: 11 October 2017). A: “de Kalmthoutse Heide”; B: “les Hautes Fagnes”.
Within wildfire literature, this region has not received any attention. Therefore, in the following paragraphs, we will discuss the (i) prevalence, (ii) damage, (iii) detection and suppression, and (iv) prevention of wildfires in Belgium, and (v) the national action plan on wildfires, which was introduced by the Federal Public Service Interior (2013) to improve the aforementioned management aspects.
- i.
The prevalence of wildfires in Belgium is rather limited. The annual burnt area rarely exceeds 40 ha, but depending on the meteorological conditions relatively large areas – in a Belgian context – can be affected. Unfortunately, these fires often occur in biologically valuable nature areas. In 2011, a year with an exceptionally dry spring characterized by 70 % less precipitation than usual (KMI, 2011), more than 2360 ha of land was affected by wildfires, of which 2144 ha burned within the Natura 2000 network. This network consists of protected nature areas throughout the European Union (San-Miguel-Ayanz et al., 2012a). The largest damage occurred in the Kalmthoutse Heide on 25 May (600 ha) and in “les Hautes Fagnes” on 25 April (1400 ha), letters A and B in Fig. 1. These two wildfires are the largest and second-largest documented wildfires in Belgium. The les Hautes Fagnes wildfire was initiated in the Baelen municipality territory (50.5407∘ N, 6.1082∘ E) on 25 April, at 17:30 CEST, and was under control by emergency services on 26 April, at 17:30 CEST. The cause has not been determined, yet the vicinity of walking trails near the ignition point supports the hypothesis of either negligence or arson. In this paper, a more detailed assessment of wildfire prevalence in Belgium is performed. The results are presented in Sect. 3.1.
- ii.
Since even the vaster wildfires in Belgium did not damage infrastructure or housing, while there have been no human casualties up to this day, it may be concluded that the damage cost of wildfires in Belgium is very limited. Essentially, wildfire damage occurs most frequently in natural areas, where wildfires might jeopardize the survival of vulnerable species like Lyrurus tetrix (Jacob and Paquet, 2011) or promote the growth of undesired plant species such as competitive grasses (e.g., Molinia caerulea) that suppress the presence of characteristic plant species, such as Calluna vulgaris and Erica tetralix (Marrs et al., 2004; Jacquemyn et al., 2005; Schepers et al., 2014). Hence, wildfire research in Belgium is important from a biological, ecological, and nature conservation perspective. In that respect, it is important to estimate the monetary value of nature in Belgium. Focusing on Flanders, Liekens et al. (2013) did this on the basis of a large-scale choice experiment to determine the willingness of households to pay for nature (EUR per household per year). These authors rank forest as the most valuable (EUR 182), followed by heathland and inland dunes (EUR 159), grassland (EUR 158), open reed and swamp (EUR 146), pioneer vegetation (EUR 119), and marshes (EUR 117). These monetary values should not be used to determine the value of nature areas, but rather to compare the value of different types of nature. It should also be noted that the monetary value of a burnt nature area is not necessarily affected in the long run since regeneration of the vegetation will often occur. Still, wildfires can alter the monetary value of an area if its cover changes from one type of nature to another. Even so, monetary value does not necessarily reflect ecological value.
- iii.
As a consequence of the high population density, wildfires in Belgium are rapidly detected and reported to the emergency services. Moreover, in some valuable nature areas extra efforts are made for an even more rapid detection. For instance, on days with a (very) high wildfire risk, in one of Flanders' vastest nature areas (“de Kalmthoutse Heide”) predominantly consisting of heathland, volunteers man a fire watch tower – a building structure that offers a clear view of the area – and immediately report any detected smoke or flames to the emergency services. Currently, this is the only wildfire detection method in use. Wildfires are suppressed by ordinary firefighters using their standard equipment, which is complemented with dedicated terrain vehicles to gain access to rough terrain, and some firefighters received a specific training in France (Federal Public Service Interior, 2013). Belgium also lacks planes or helicopters that can be deployed in the case of wildfires, though in 2015 a bilateral agreement between Belgium and the Netherlands was signed to deploy a dedicated helicopter from the Netherlands in the case of major events (Ministry of Justice and Security, 2015). Also in the past, aerial means from neighboring countries were deployed in large-scale exercises in les Hautes Fagnes to fight the largest wildfires (Belga, 2013). Since wildfires are rather rare and mostly ordinary firefighting equipment is used, the suppression cost of wildfires in Belgium is expected to be a limited portion of the total budget spent on its emergency services.
- iv.
The main prevention strategy in nature areas is to assign a color code reflecting the wildfire risk. The exact procedure is defined at the provincial level, and it is determined by the terrain manager and local experts by combing information from three sources: (1) field assessments, (2) consultation of the European Forest Fire Information System (EFFIS) fire danger forecast, and (3) consultation of the Brand Waarschuwings Index (BWI, “fire warning index”), a national index developed by the Belgian Air Force. These color codes come with specific guidelines for visitors and firefighters. “Code green” means that there is a low wildfire risk, and in the unlikely event of a wildfire, the fire brigade follows the standard procedure in terms of the number of men. “Code yellow” is associated with an elevated risk. For instance, in de Kalmthoutse Heide the watch tower is manned on such days. If a wildfire is detected in a region with “code orange”, the fire brigade will deploy extra men and equipment. Moreover, the fire watch tower is permanently manned and children can only play under parental supervision. Finally, “code red” means that the wildfire risk is very high and access to such areas is discouraged (ANB, 2017). In the case of the 2011 wildfires in de Kalmthoutse Heide and les Hautes Fagnes, the wildfire risk for both areas was classified as code red. Another form of prevention is the construction or repair of firebreaks, as illustrated in the management plans for military domains (e.g., Vandenberghe et al., 2009; Waumans et al., 2009).
- v.
In the aftermath of the 2011 wildfires (San-Miguel-Ayanz et al., 2012a), and largely motivated by the shortcomings and problems detected while being faced with fighting relatively vast wildfires (up to 1000 ha), the national action plan on wildfires was compiled by the directorate general of the Federal Public Service Interior in order to evaluate and improve the risk analysis and cartography, materials, procedures and training, emergency planning, and exercises related to the outbreak of wildfires (Federal Public Service Internal Affairs, 2013). Although a preliminary risk map was constructed based on the qualitative feedback from emergency planning services and province governors, EU legislation dictates that a more scientifically sound approach should be used. This is important because the law states that forest areas classified as medium to high forest fire risk are eligible for financial support from the European Regional Development Fund. However, such a wildfire risk map must be backed up by scientific evidence and acknowledged by scientific public organizations, in agreement with Article 24 of Regulation (EU) No. 1305/2013 of the European Parliament and of the Council of 17 December 2013 (European Parliament and European Council, 2013). In order to support the EU member states in arriving at such a map and to harmonize the used methodology across the EU member states, the European Commission has consulted the EU member states on how the JRC should proceed during the 2017 meeting of the Commission Expert Group on Forest Fires. Moreover, the preliminary risk map included in the national action plan on wildfires did not account for how “high risk” is perceived differently by the consulted parties across the country.
2.2 Wildfire inventory for Belgium
In order to develop a wildfire ignition probability map (IPM) for Belgium, data on historical wildfire ignitions were needed. These data were collected in two ways. Firstly, a list of all wildfire interventions between 2010 and 2013 was provided by the directorate general of the Federal Public Service Interior. The ignition location was identified by means of (i) a residential address, (ii) personal communication with the firefighting services, and/or (iii) topographic features. Secondly, the digital archives of several newspapers were searched through. These archives covered the period 1985–2016, though relevant data were retrieved for the period 1994–2016 only. The following newspapers were searched: Gazet van Antwerpen, Het Laatste Nieuws, Het Belang van Limburg, Le Soir, L'Echo, La Dernière Heure, La Meuse, La Nouvelle Gazet, Metro, and L'Avenir, thereby ensuring that most news items on wildfires throughout the country would be retrieved. For these instances, the location of the wildfire ignition was assessed through (i) the description of topographic features and (ii) communications with the relevant firefighting services. This way, we assumed that the remaining uncertainty on the location of the registered wildfires was higher than the chosen 100 m spatial resolution.
2.3 Modeling ignition probability
The definition of wildfire risk varies greatly within literature (Miller and Ager, 2013; San-Miguel-Ayanz et al., 2017). In the past, many authors described risk as the probability of wildfire occurrence (e.g., Hardy, 2005; Catry et al., 2009). As a consequence, many wildfire risk assessments are, following the wildfire framework of the European Commission, in fact an assessment of the ignition probability. Common approaches for such an assessment involve data-driven methods such as logistic regression (e.g., Martinez et al., 2008; Catry et al., 2009; Vilar del Hoyo et al., 2011; Preisler et al., 2004), machine learning (e.g., Massada et al., 2012; Rodrigues and de la Riva, 2014), and a Bayesian weights-of-evidence modeling approach (e.g., Kolden and Weigel, 2007; Dickson et al., 2006). The last method involves the use of Bayes' rule to calculate weights for the different classes of input maps. These weights are then integrated per grid cell in a logit equation to obtain a probability (Dickson et al., 2006).
However, we consider there are some limitations towards the interpretation of the probabilities obtained with these aforementioned methods. First, the increase in ignition probability is not proportional to the actual increase in the occurrence of ignitions. More concretely, a doubling of the ignition probability may not be interpreted as a doubling of the number of wildfire occurrences. Second, the probabilities do not have a time dimension: for which period is this probability valid? If the ignition probability in a grid cell equals 0.8, then how should this value be interpreted? Clearly, we cannot interpret it so that the chance of ignition for such a cell equals 80 % in a given year. In this paper, we use a straightforward application of Bayes' rule to tackle the issues of proportionality and time specificity. The ignition probability in this paper is defined as the average probability that an ignition will occur during the course of 1 calendar year within a grid cell (Dawid et al., 2005):
where I indicates an ignition event and Ci contains the features that characterize the environment of cell i. Such an environment is defined as the specific combination of predictor classes.
In Eq. (1), the probability that a randomly selected cell belongs to class Ci is equal to
P(Ci|I) is the probability that, given that an ignition took place in cell i, this cell belongs to class Ci, and was computed as
with the total number of ignitions determined by the number of ignitions used for the construction of the IPM. Finally, the probability that an ignition occurs in a random cell within the time span of 1 year was calculated as
Due to the low number of wildfire occurrences in Belgium, the size of the wildfire inventory is expected to be rather limited, with only a few hundred registered wildfires. Therefore, the number of possible environments had to be kept relatively small, otherwise, too many environments without any recorded wildfires would be created. In this paper, the maximum number of environments was arbitrarily set at 20. An overview of the complete methodology is given in Fig. 2.
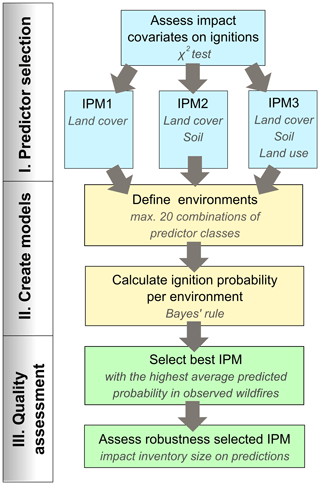
Figure 2A schematic representation of the methodology used in this paper to calculate the wildfire ignition probability. In stage I, we assess the significance of the impact on wildfire ignition of the three predictors, and we outline three models, each with a different parameter set. In stage II, no more than 20 unique environments are created per model through the combination of different predictor classes. We then use Bayes' rule to calculate the ignition probability observed in each environment. Stage III comprises the selection of the best model and assessing its robustness, or, in other words, the impact of the inventory size on the model's prediction average and variance.
The annual ignition probabilities, which are calculated per grid cell, can be merged for larger areas using Eq. (5):
where PA is the probability that a certain area A containing n environments will be affected by a wildfire in the span of 1 year, pi is the probability that a grid cell of environment i will burn within 1 year (Eq. 1), and Ni is the number of grid cells of environment i within area A. Note that for the application of Eq. (5), we assume that the ignition probabilities in neighboring pixels are independent. In reality, however, this will not be the case. An ignition might give rise to significant wildfire spread. In the short term, this might lead to a decrease in the ignition probabilities of the neighboring burnt pixels because of the removal of fuel. In the long term, burnt pixels might display a transition to more fire-prone vegetation, thus increasing the ignition probability (e.g., Jacquemyn et al., 2005).
2.4 Predictors
We considered three categorical covariates: (i) land cover, (ii) soil, and (iii) land use (Fig. 3). Given the nature of the applied methodology (Sect. 3.1), the number of spatial layers was restricted to three. Due to this restriction, we did not integrate data on population density, precipitation, and distance to roads (e.g., Dickson et al., 2006) in the analysis. We used the χ2 test of independence to determine whether there was a significant impact of each variable on the wildfire occurrence (McDonald, 2014). Due to the spatial scale at which the wildfire data are reliable, all data layers were resampled to a 100 m resolution.
The land cover vector dataset, dating from 2011 and originally provided at a 10 m resolution, was obtained from the Belgian National Geographic Institute (NGI) and rasterized. This variable contains the following 11 classes: coniferous forest, deciduous forest, mixed forest, heathland, mixed heathland coniferous forest, mixed heathland deciduous forest, agricultural land, reed land, shrubland, urban land, and other. Different vegetation types can display a different wildfire susceptibility (Bond and van Wilgen, 1996). More in particular, in the context of Belgium, coniferous forests and heathland are more sensitive to wildfires than other vegetation types (Goldammer and Furyaev, 2013; Log et al., 2017).
The soil vector data were constructed for Flanders in 2016 by the Flemish Soil Database (DOV) and for Wallonia in 2007 by the Walloon Public Service (SPW). Both datasets are applicable at a 1 : 20 000 map scale. Six different classes are distinguished: rock, clay, loam, sand, fen/wetland, and other. The different soil types are mainly based upon particle size (sand, loam, and clay), which is negatively correlated with soil moisture and water retention (Kaleita et al., 2005). The availability of soil moisture to vegetation influences the fuel condition and hence the ignition probability (Chuvieco et al., 2004; Chaparro et al., 2015).
The land use vector data were developed at a 1 : 10 000 scale for Flanders in 2014 by the DOV and for Wallonia in 2016 by the SPW. Land use data provide information on how people behave in a certain region and hence serve as a proxy for human impact on wildfires. In Belgium, for example, military exercises are a known cause of wildfire ignitions as a consequence of the use of explosives. In addition to its impact on fire ignitions, land use can also have an effect on fuel loads (Van Butsic and Moritz, 2015). We distinguished seven different land use classes: habitat, agriculture, military, economy/industry, recreation, nature conservation areas, and other.
The average population density in Belgium (363 inh. km−2) is much higher than in the Mediterranean countries where wildfires are much more rampant: Spain (93 inh. km−2), Portugal (115 inh. km−2), France (118 inh. km−2), Greece (84 inh. km−2), and Italy (203 inh. km−2) (United Nations, 2015). Conversely to these countries, Belgium has few remote areas with low population densities that are not urbanized in one way or another. Moreover, the highest densities are to be found in urbanized areas where we do not expect wildfires.
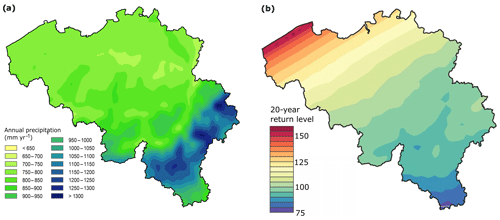
Figure 4(a) The average annual rainfall in Belgium (Meersmans et al., 2016), and (b) the 20-year return level of a precipitation deficit expressed in days, and calculated in reference to the evapotranspiration rates of coniferous forests (Zamani et al., 2016).
Precipitation in Belgium varies roughly between 700 and 1000 mm yr−1, with peaks of up to 1300 mm yr−1 in the southeastern regions of the country like les Hautes Fagnes (Fig. 4a) (Meersmans et al., 2016). Despite the high precipitation rates in this area, les Hautes Fagnes is known for its many and vast wildfires (e.g., San-Miguel-Ayanz et al., 2012a). Hence, rather than looking at the mean annual rainfall, it would be more appropriate to use data on drought sensitivity, for example based on the precipitation deficit (Zamani et al., 2016). Figure 4b shows the extent (days) of the most severe drought expected in a period of 20 years. There is a clear gradient from west to southeast, inferring that the coastal areas are most sensitive to precipitation deficits. However, it is known that most fires occur in the east of the country (Federal Public Service Interior, 2013). Therefore, we concluded that both the available annual rainfall and drought sensitivity map were not suitable for modeling the ignition probability. Given the fact that most anthropogenic wildfires are controlled by drought (Burk, 2005), future research should aim at the development of more suitable drought covariates for Belgium that reflect the different responses of different plant communities and soil types to precipitation deficits.
The road network is very dense across the entire country. In fact, the road density in Belgium is 5 times as high as the average for the European Union (5.1 km km−2 versus 1.1 km km−2) (European Union Road Federation, 2016). Furthermore, in most cases the location of wildfire interventions by firefighters is identified by means of a residential address, i.e., municipality, street name, and number, possibly biasing the perception of wildfire as occurring closer to roads.
2.5 Quality assessment
In total, three wildfire IPMs were constructed. The first (IPM1) is solely based on land cover class, the second one (IPM2) on land cover class and soil type, and the third one (IPM3) on land cover class, soil type, and land use class. For each IPM, the number of environments was kept lower than or equal to 20.
In order to compare the quality of these three different IPMs, each one was constructed 23-fold, every time leaving out the wildfire data for 1 year. The average ignition probability at the wildfire locations of the discarded year served as a measure for model quality. For example, for the first of the 23 IPMs, we used the data between 1994 and 2015 for training and the data of 2016 to validate whether the IPM predicts a high wildfire ignition probability at those locations where wildfires occurred in 2016. As such, an indication was obtained of how reliably the map reflected the ignition probability at locations that were effectively affected in the course of history. The IPM resulting in the highest average predicted ignition probabilities was considered to be the most accurate. We relied on the nonparametric Mann–Whitney U test to identify this IPM, with a 5 % level of significance (McDonald, 2014).
Next, the robustness of the best IPM was investigated. We assessed the influence of the inventory size on the model quality by constructing the IPM several times with datasets of increasing size. The first map was constructed with data from the period 1994–2004. Subsequently, we incrementally increased the length of the period from which data were used in the IPM construction stage with 1 year. As such, we constructed 13 IPMs and the first one with data from the period 1994–2004, the last one with data from the period 1994–2016. For each IPM, we randomly selected 90 % of the data for calibration, while the remaining 10 % of the instances were used to assess the quality, i.e., the average predicted probability within observed ignition points. The robustness of each of the 13 IPMs was tested by calibrating each of the IPMs 100 times. This approach allowed us to construct a boxplot of the corresponding average ignition probabilities in the 13 IPMs. The range of each of these 13 probabilities is a proxy for the robustness of the IPMs.
3.1 Wildfire inventory for Belgium
3.1.1 Spatial distribution
In total, 385 wildfires were recorded, from which 273 were assigned GPS coordinates. The wildfire locations are displayed in Fig. 1. In Flanders, the northern half of Belgium, the eastern provinces of Antwerp and Limburg clearly show a higher wildfire ignition probability and prevalence than the other provinces. In Wallonia, the southern part of Belgium, wildfires seem to be less rampant and occur mainly in the east and southwest parts of the region. An explanation for the distribution of these wildfires can be found in the social, economical, and technological shifts of the 19th century and their impact on land use and cover (Buis, 1985).
In Flanders, the omnipresent heathland, characterized by poor, sandy soils, was afforested in the eastern provinces with Pinus sylvestris, while the forests on the rich soils in the west were cleared for agricultural practices (den Ouden et al., 2010). At present, both forests and heathland are relatively more common in Limburg and Antwerp than in the rest of Flanders (Hermy et al., 2004); thus it is expected that the average wildfire ignition probability in these two provinces is higher than in the other Flemish provinces.
In Wallonia, the relative forested area is 3 times as high as the one in Flanders, 32.0 % versus 11.4 % (Walloon Government and European Commission, 2015; Stevens et al., 2015). The forested areas are mainly concentrated in the eastern provinces of Liège and Luxembourg. The typical tree species used for afforestation in this region is Picea abies, a coniferous species associated with a very high wildfire sensitivity (Goldammer and Furyaev, 2013), which would explain a relatively high number of wildfire occurrences in the Liège and Luxembourg. As expected, the nature reserve “les Hautes Fagnes” (in the eastern part of Liège) and its surrounding area show a higher prevalence because of its fens, which easily dry out in the absence of rain.
Unfortunately, precise data on the size of wildfires were very scarce. Most wildfires covered small areas (<1 ha), though for some major events, relatively accurate estimates of the burnt area could be provided (San-Miguel-Ayanz et al., 2012a). An interesting observation is that major events occurred in heathland or fen. It seems that wildfires in such land cover are less controllable than those in coniferous or deciduous forests. This can be understood by the fact that heathlands and fens are largely covered with shrubs and grass that ignite easily and hence allow wildfires to propagate rapidly. In 2011, a series of wildfires raged through three nature areas: les Hautes Fagnes, de Kalmthoutse Heide (heathland), and the military domain in Meeuwen, destroying respectively 1400, 600, and 360 ha. In total, more than 2360 ha of land was burnt that year, mainly Natura 2000 sites (Schmuck et al., 2012).
3.1.2 Temporal distribution
Contrary to the statement of the Federal Public Service Interior (2013) that there are two periods with an elevated wildfire occurrence (April–May and August), the data displayed in Fig. 5a indicate that the number of ignitions peaks in April. This can be explained by the seasonal rainfall pattern, which shows that April is the month with the lowest precipitation (Journée et al., 2015). The frequency drops rapidly in May and June and remains stable in July and August, despite the fact that these months display the highest average temperatures (Federal Public Service Interior, 2013). This observation confirms the hypothesis of Burk (2005) that human-induced wildfires are more controlled by precipitation than temperature. Outside the period April–August, wildfires are rather scarce. To visualize how this seasonal pattern was impacted by years with many wildfire ignitions, the frequency for each month was calculated 21 times, alternately leaving out the data for 1 year. The obtained difference between the minimal and maximal monthly frequency appeared to be small. Hence, the seasonal pattern seems not to be sensitive to years with many fires, such as the period between 2010 and 2013.
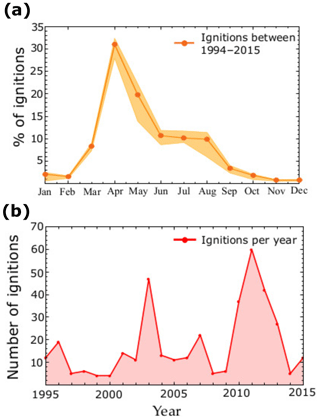
Figure 5(a) The monthly relative ignition frequency between 1994 and 2015, and (b) the number of ignitions per year.
Figure 5b shows the number of wildfires per year for the period 1995–2015. The data for 1994 were omitted because almost no newspapers were digitized for this period, and the wildfires for 2016 were not included in the figure because, at the time this research was conducted, the year had not yet passed. The figure shows clearly that there is a great variability in the number of wildfires between different years. A critical note is that for the period 2010–2013 the data were more complete (because a list with wildfire interventions was provided by the government), possibly explaining the higher number of wildfires in these years. Due to this lack of a standardized registration approach, it was not possible to compare the number of ignitions to climatic data and derive reliable relationships. Nonetheless, in 2003, the number of wildfires was extremely high as a consequence of the extremely warm and dry summer (Eysker et al., 2005).
3.1.3 Ignition sources
This research made it clear that negligence (e.g., ignitions due to cigarettes or campfires), arson, and military exercises were major drivers of ignition; records have even been found that support the hypothesis that pieces of glass can trigger a fire through the redirection and focusing of sunlight (Timperman and Willekens, 1999). No reports were found of natural ignition causes such as lightning. In other words, humans are the main driver of wildfires in Belgium. This is consistent with other regions in Europe, e.g., the Mediterranean area, where 95 % of the ignitions can be attributed to human causes (San-Miguel-Ayanz et al., 2012b).
3.2 Creating environments
Figure 6 shows the comparison between the observed and expected ignition frequencies for each variable, where the expected ignition frequency was calculated as the proportion of the total study area of each category of that specific variable. As the nonparametric χ2 test of independence proved, the land cover class clearly influenced the wildfire ignition probability (χ2=206.4, p<0.05). Likewise, soil type had a significant impact on the prevalence of wildfire ignitions (χ2=100.4, p<0.05), as did land use class (χ2=198.2, p<0.05).
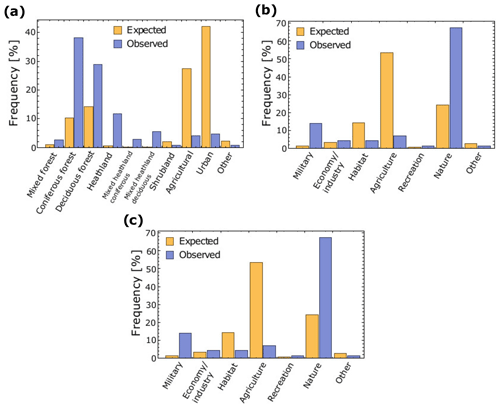
Figure 6The expected and observed ignition frequency in relation to the distribution of the (a) land cover, (b) soil, and (c) land use classes.
The first IPM was constructed by taking into account land cover classes, which gave us 11 possible environments. These are displayed in Fig. 3a. For the second IPM, we simplified the land cover map by reclassifying it into three classes, guided by the frequency discrepancies between the observed and expected number of wildfires (Fig. 6): (i) forests (covering 25.44 % of the area), by merging deciduous, mixed, and coniferous forests; (ii) shrubland (2.84 %), by grouping heathland and shrubland; and (iii) a third class containing the remaining land cover classes (71.72 %). In total, 18 environments remained for the second IPM.
The third IPM was based on the three land cover classes, soil, and land use maps. The soil map was composed of (i) sand (21.35 %), (ii) wetlands/fens (0.48 %), and (iii) a class that contained the remaining soil types (78.17 %). The land use map distinguished between three classes: (i) military domains (1.18 %), (ii) nature areas (25.43 %), and (iii) the remaining land use classes (73.39 %). Hence, in total, 27 possible environments were defined for the third IPM. However, this procedure led to environments with a very small spatial extent. Therefore, such environments were merged into two new environments: first, we merged all the military domains with a soil type different from sand. Second, within the “other” land use class, all environments with wetland or fen land cover were merged. As such, 20 environments remained for which the ignition probability was assessed.
3.3 Ignition probability maps
Figure 7 shows, for each of the three IPMs, the 23 different average wildfire ignition probabilities observed at the wildfire locations that were not used for the IPM construction. The Mann–Whitney U test showed that there was no significant difference in the medians of IPM1 and IPM2 (p=0.561). However, IPM3 had a significantly higher median than IPM1 (p=0.020) and IPM2 (p=0.003). Hence, IPM3, based on three covariates, was considered the best wildfire ignition probability model.
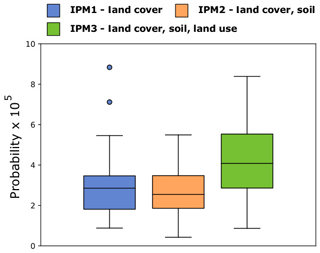
Figure 7The average ignition probability observed in the data points that were not used for the construction of the IPM.
From Fig. 8, we infer that the quality of the IPM, expressed as the predicted probability in observed ignition points, remains stable for an increasing inventory. It can also be observed that the robustness of the IPM increases substantially for the smaller datasets, while, for datasets larger than the one that contains the data from the period 1994–2011 (219 ignitions), the quartiles of the boxplots appear at more or less the same values.
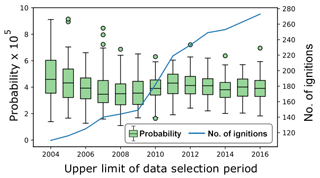
Figure 8An illustration of the dependency on the number of data points of the robustness of the ignition probability map. The boxplots show the robustness of the ignition probability map as a function of the data period that was used for construction, from 1994 to the upper limit. The line shows the actual number of data points used for model training.
The final IPMs were constructed with all 273 data points. We defined four probability classes guided by three principles: (i) the highest class should cover the smallest part of the study area and vice versa; (ii) the visible gaps, which might be an artifact of the small number of environments, should be used to identify natural breaks where possible; and (iii) the probability classes must be equal for all three IPMs, without violating the first principle (Fig. 9 and Table 1).
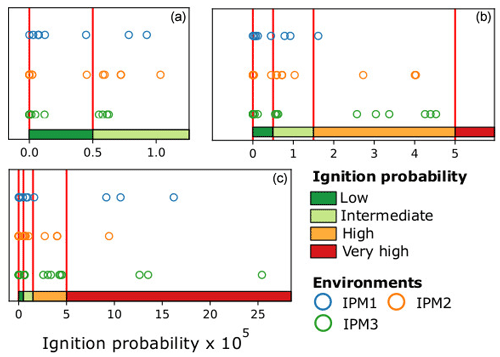
Figure 9(a) Frequency of the calculated probabilities in the ignition probability maps constructed with land cover class; (b) land cover class and soil type; and (c) land cover class, soil type, and land use class. The four probability class intervals are indicated by red lines.
Table 1Relative areas (%) per ignition probability class for the three IPMs and the average probability assigned to the ignition points.
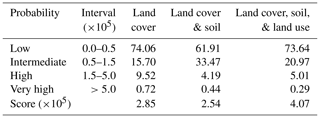
Table 2The relative area (%) per ignition probability class for the Belgian provinces and the capital region of Brussels.
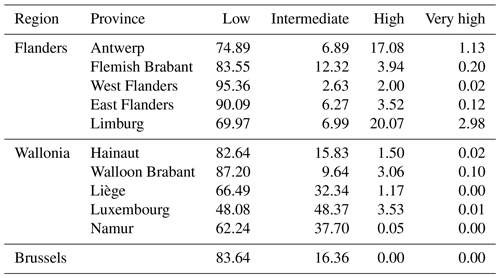
The IPM leading to the highest probabilities assigned to the wildfire ignition points is the one that considers land cover class, soil type, and land use class; hence, such an IPM was constructed with all 273 ignition points (Fig. 10). The average ignition probability assigned to all data points was wildfire ignitions per year and per 100 m × 100 m grid cell. The relative area per ignition probability class for each province is presented in Table 2. As expected for Flanders, the provinces of Antwerp and Limburg have the largest high-probability area. In Wallonia, the provinces of Liège and Luxembourg appear to be most sensitive to wildfires.
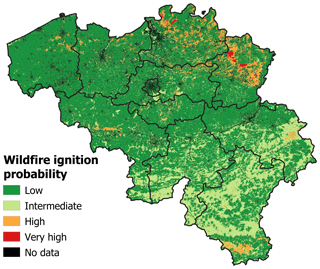
Figure 10The ignition probability map constructed with land cover class, soil type, and land use class.
The maximum calculated probability for the final IPM was . According to Eq. (5), this means that within such an area of 1000 ha, the annual ignition probability is 22.4 %. The section of les Hautes Fagnes where the 2011 wildfire occurred has a total area of 2091 ha. Here, the annual ignition probability is 4.3 %. Note that the maximum calibrated probability is extremely low compared to the results obtained with logistic regression or machine learning. Using these techniques, probabilities as high as 80 % were observed for a significant portion of the study area (e.g., Martinez et al., 2008; Catry et al., 2009; Massada et al., 2012). However, these values cannot be interpreted as ignition probabilities in the sense of an annual chance that a certain pixel will burn, but rather as the similarity between the spatial characteristics of a given pixel and the average spatial characteristics of historical wildfires.
It should be underlined that this study is a very first assessment of the wildfire ignition probability in Belgium, which is a determinant of wildfire hazard, and hence of wildfire risk (IPCC, 2012; San-Miguel-Ayanz et al., 2017). The study was complicated by (i) the lack of literature on wildfires in Belgium, (ii) the limited number of ignitions, and (iii) the uncertainty of the ignition locations. The latter was a decisive factor in determining the optimal spatial resolution of the model, i.e., sufficiently low on the one hand to capture the uncertainty on the ignition data and sufficiently high on the other hand to allow for the application of our model at a provincial or municipal scale.
Existing wildfire literature is often limited to a description of the wildfire impact on ecosystems (e.g., Marrs et al., 2004; Jacquemyn et al., 2005; Schepers et al., 2014). The only well-described wildfire damage occurred in natural areas, like in 2011, when 2144 ha of natural areas was consumed by flames within the Natura 2000 network (San-Miguel-Ayanz et al., 2012a). The lack of literature on the damage to properties and human livelihoods is understandable, as no evidence of such events could be produced.
Not surprisingly, given the fact that wildfire occurrence and damage are rare in Belgium, the number of instances included in the used wildfire database was relatively low. The database compilation was even further complicated by the lack of a standardized registration procedure for interventions of emergency services in the case of wildfires. However, It can be expected that more data will become available in the near future, (i) due to an increased interest of policy makers in wildfires motivated by the fact that wildfires might occur more frequently in the future (Federal Public Service Interior, 2013) and (ii) because of a standardization of wildfire registration by fire brigade interventions.
In order to calculate the ignition probability, we used a straightforward data-driven approach relying on Bayes' rule. Contrary to other approaches (e.g., Martinez et al., 2008; Catry et al., 2009; Massada et al., 2012), the resulting map provides a tangible estimation of the annual probability that a wildfire will ignite in a certain region. Moreover, we demonstrated that this approach can be used to obtain an estimate of the average annual ignition probability in a certain area. Our method involved the delineation of environments through the combination of predictor classes. Because of the limited number of wildfires in Belgium, it was necessary to limit the number of environments to 20 and hence the number of covariates to three. To allow for more covariates, the ignition database should be larger. It could be concluded that the approach relying on exactly three covariates (land cover, soil, and land use) led to the most reliable wildfire ignition probability map, which is, moreover, robust to an increase in the number of wildfires in the underlying database. We assume that our model could be substantially improved through the inclusion of more covariates, preferably a drought index for Belgium that reflects plant moisture sensitivity to precipitation deficits.
In line with the spatial wildfire distribution (Fig. 1), the provinces of Limburg and Antwerp display the highest probabilities (Table 2), which can be explained by the relatively large areas covered by heathland and coniferous forest, and the presence of military training areas. As such, these provinces should receive a proportionally higher share of the available means for wildfire prevention and suppression.
A final remark is that most causative factors are human. Anthropogenic ignition causes such as military explosions, arson, cigarettes, campfires, and broken glass have been reported, while natural ignitions such as lightning strikes appear to be exceptional. It seems that the best way of preventing wildfires is perhaps to exclude military exercises in fire-prone areas during the months of April to August. Furthermore, improvement in fire detection methods could be made (e.g., the use of drones), the lack of heavy firefighting equipment such as planes should be compensated through an increased cooperation with foreign emergency services, the available resources should be located in the most fire-prone areas, and the awareness of the general public could be raised, so that people become more aware of the danger they pose to the natural environment. In the context of global change and the expected increase in extreme weather events such as dry spells and heat waves, well-considered and elaborate wildfire management will gain more and more importance in Belgium.
The wildfire ignition data and probability map for Belgium can be requested from the authors (arthur.depicker@kuleuven.be).
AD conducted the formal analysis and investigation, helped develop the methodology, and was in charge of writing the original draft and the reviewed/edited drafts. BDB helped develop the applied methodology and helped write and edit the paper. JMB was responsible for the conceptualization of this paper and helped develop the applied methodology and write and edit the paper.
The authors declare that they have no conflict of interest.
We are grateful for the wildfire data provided by the directorate general of the Federal Public Service Interior.
This paper was edited by Ricardo Trigo and reviewed by three anonymous referees.
ANB: Bos en heide brandbestrijding, available at: http://www.kalmthout.be/brandweer-bos-en-heide-brandbestrijding.html, last access: 15 October 2017.
Belga: Franse blusvliegtuigen oefenen bestrijding van bosbrand in Hoge Venen, available at: https://www.knack.be/nieuws/belgie/franse-blusvliegtuigen-oefenen-bestrijding-van-bosbrand-in-hoge-venen/article-normal-93149.html (last access: 20 November 2017), 2013.
Belgian Federal Government: Over België, available at: http://www.belgium.be/nl/over_belgie/land, last access: 11 August 2016.
Bond, W. J. and van Wilgen, B. W.: Fire and Plants, Chapman & Hall, London, UK, 1996.
Buis, J.: Historia Forest: Nederlandse Bosgeschiedenis, HES Uitgevers, 't Goy, the Netherlands, 1985.
Burk, A. R. (Ed.): New research on Forest Ecosystems, Nova Science Pub Inc, Happauge, NY, USA, 2005.
Catry, F. X., Rego, F. C., Bacao, F. L., and Moreira, F.: Modeling and mapping wildfire ignition risk in Portugal, Int. J. Wildland Fire, 18, 1–11, https://doi.org/10.1071/WF07123, 2009.
Chaparro, D., Vall-Ilosera, M., Piles, M., Camps, A., and Rudiger, C.: Low soil moisture and high temperatures as indicators for forest fire occurrence and extent across the Iberian Peninsula, in: 2015 IEEE International Geoscience and Remote Sensing Symposium, 13–18 July 2015, Milan, Italy, 3325–3328, https://doi.org/10.1109/IGARSS.2015.7326530, 2015.
Chuvieco, E., Cocero, D., Riano, D., Martin, P., Martinez-Vega, J., de la Riva, J., and Perez, F.: Combining ndvi and surface temperature for the estimation of live fuel moisture content in forest fire danger rating, Remote Sens. Environ., 92, 322–331, 2004.
Dawid, P., Earman, J., Howson, C., Miller, D., and Sober, E.: Bayes's theorem, Oxford university press, Oxford, UK, https://doi.org/10.5871/bacad/9780197263419.001.0001, 2005.
den Ouden, J., Muys, B., Mohren, F., and Verheyen, K. (Eds.): Bosecologie en Bosbeheer, Acco, Leuven, Belgium, 2010.
Dickson, B. G., Prather, J. W., Xu, Y., Hampton, H. M., Aumack, E. N., and Sisk, T. D.: Mapping the probability of large fire occurrence in northern Arizona, USA, Landscape Ecol., 21, 747–761, https://doi.org/10.1007/s10980-005-5475-x, 2006.
Doerr, S. H. and Santin, C.: Global trends in wildfire and its impacts: perceptions versus realities in a changing world, Philos. T. Roy. Soc. B, 371, 1–10, https://doi.org/10.1098/rstb.2015.0345, 2016.
Eisenman, D., McCaffrey, S., Donatello, I., and Marshal, G.: An ecosystems and vulnerable populations perspective on solastalgia and psychological distress after a wildfire, EcoHealth, 12, 602–610, 2015.
European Parliament, the European Counsil: Regulation (eu) no 1305/2013 of the european parliament and of the council of 17 december 2013 on support for rural development by the european agricultural fund for rural development (eafrd) and repealing council regulation (ec) no. 1698/2005, Official Journal of the European Union, 56, 487–548, 2013.
European Union Road Federation: Road Statistics, Yearbook 2016, Brussels, Belgium, 2016.
Eysker, M., Bakker, N., Kooyman, F. N., van der Linden, D., Schrama, C., and Ploeger, H. W.: Consequences of the unusually warm and dry summer of 2003 in The Netherlands: Poor development of free living stages, normal survival of infective larvae and long survival of adult gastrointestinal nematodes of sheep, Vet. Parasitol., 133, 313–321, https://doi.org/10.1016/j.vetpar.2005.05.058, 2005.
Federal Public Service Interior: Nationaal Actieplan Natuurbranden, Brussels, Belgium, 2013.
Giglio, L., Randerson, J. T., van der Werf, G. R., Kasibhatla, P. S., Collatz, G. J., Morton, D. C., and DeFries, R. S.: Assessing variability and long-term trends in burned area by merging multiple satellite fire products, Biogeosciences, 7, 1171–1186, https://doi.org/10.5194/bg-7-1171-2010, 2010.
Goldammer, J. G. and Furyaev, V.: Fire Ecosystems of Boreal Eurasia, Springer Science & Business Media, Dordrecht, the Netherlands, 2013.
Hardy, C. C.: Wildland fire hazard and risk: problems, definitions, and context, Forest Ecol. Manage., 15, 2097–2108, https://doi.org/10.1016/j.foreco.2005.01.029, 2005.
Hermy, M., de Blust, G., and Slootmaekers, M.: Natuurbeheer, Davidsfonds, Leuven, Belgium, 2004.
IPCC: Managing the Risks of Extreme Events and Disasters to Advance Climate Change Adaptation. Special Report of the Intergovernmental Panel on Climate Change, edited by: Field, C. B., Barros, V., Stocker, T. F., Dahe, Q., Dokken, D. J., Plattner, G.-K., Ebi, K. L., Allen, S. K., Mastrandrea, M. D., Tignor, M., Mach, K. J., and Midgley, P. M., Cambridge University Press, Cambridge, UK, 2012.
IPCC: Climate Change 2014: Synthesis Report. Contribution of Working Groups I, II and III to the Fifth Assessment Report of the Intergovernmental Panel on Climate Change, IPCC, edited by: Pachauri, R. K. and Meyer, L. A., Geneva, Switzerland, 2014.
Jacob, J. P. and Paquet, J. Y.: Nieuwe waalse broedvogelatlas 2001–2007, Natuur oriolus, 77, 39–47, 2011.
Jacquemyn, H., Brys, R., and Neubert, M. G.: Fire increases invasive spread of Molinia caerulea mainly through changes in demograhic parameters, Ecol. Appl., 15, 2097–2108, https://doi.org/10.1890/04-1762, 2005.
Journée, M., Delvaux, C., and Bertrand, C.: Precipitation climate maps of Belgium, Adv. Sci. Res., 12, 73–78, 2015.
Kaleita, A. M., Tian, L. F., and Hirschi, M. C.: Relationship between soil moisture content and soil surface reflectance, T. ASAE, 48, 1979–1986, 2005.
KMI: Jaarverslag KMI 2011, Brussels, Belgium, 2011.
Kolden, C. A. and Weigel, T. J.: Fire risk in San Diego County, California: a weighted Bayesian model approach, Californian Geographer, 47, 42–60, 2007.
Liekens, I., Schaafsma, M., De Nocker, L., Steven, B., Staest, J., Aertsens, J., and Brouwer, R.: Developing a value function for nature development and land use policy in Flanders, Belgium, Land Use Policy, 30, 549–559, https://doi.org/10.1016/j.landusepol.2012.04.008, 2013.
Liu, G. C., Pereira, G., Uhl, S. A., Bravo, M. A., and Bell, M. L.: A systematic review of the physical health impacts from non-occupational exposure to wildfire smoke, Environ. Res., 136, 120–132, 2014.
Log, T., Thuestad, G., Velle, L. G., Khattri, S. K., and Kleppe, G.: Unmanaged heathland a fire risk in subzero temperatures?, Fire Safety Journal, 90, 62–71, https://doi.org/10.1016/j.firesaf.2017.04.017, 2017.
Marrs, R. H., Phillips, J. D., Todd, P. A., Ghorbani, J., and Le Duc, M. G.: Control of Molinia caerulea on upland moors, J. Appl. Ecol., 41, 398–411, https://doi.org/10.1111/j.0021-8901.2004.00901.x, 2004.
Martinez, J., Chuvieco, E., Martin, P., and Gonzalez-Caban, A.: Estimation of Risk Factors of Human Ignition of Fires in Spain by Means of Logistic Regression, in: Second International Symposium on Fire Economics, Planning and Policy: A Global View. Albany, https://doi.org/10.2737/PSW-GTR-208, 2008.
Massada, A., Syphard, A., Stewart, S., and Radeloff, V.: Wildfire ignition-distribution modelling: A comparative study in the Huron-Manistee National Forest, Michigan, USA, Int. J. Wildland Fire, 22, 174–183, https://doi.org/10.1071/WF11178, 2012.
McDonald, J.: Handbook of Biological Statistics, 3rd edn., Sparky House Publishing, Baltimore, MD, USA, 2014.
Meersmans, J., Van Weverberg, K., De Baets, S., De Ridder, F., Palmer, S. J., van Wesemael, B., and Quine, T. A.: Mapping mean total annual precipitation in Belgium, by investigating the scale of topographic control at the regional scale, J. Hydrol., 540, 96–105, https://doi.org/10.1016/j.jhydrol.2016.06.013, 2016.
Miller, C. and Ager, A. A.: A review of recent advances in risk analysis for wildfire management, Int. J. Wildland Fire, 22, 1–14, https://doi.org/10.1071/WF11114, 2013.
Ministry of Justice and Security: Memorandum van overeenstemming over de uitvoering van fire bucket operations, Staatscourant 7003, Brussels, Belgium, 2015.
National Wildlife Federation: Increased Risk of Catastrophic Wildfires: Global Warmings Wake-Up Call for the Western United States, National Wildlife Federation Report, Reston, VA, USA, 2008.
Navarro, K. M., Schweizer, D., Balmes, R. J., and Cisneros, R.: A review of community smoke exposure from wildfire compared to prescribed fire in the United States, Atmosphere, 9, 185, https://doi.org/10.3390/atmos9050185, 2018.
North, B. M., Stephens, S. L., Collins, B. M., Agee, J. K., Aplet, G., Franklin, J. F., and Fulé, P. Z.: Reform forest fire management, Science, 349, 1280–1281, https://doi.org/10.1126/science.aab2356, 2015.
Penman, T. D., Nicholson, A. E., Bradstock, R. A., Collins, L., Penman, S. H., and Price, O. F.: Reducing the risk of house loss due to wildfires, Environ. Modell. Softw., 67, 12–25, 2015.
Preisler, H., Brillinger, D., Burgan, R., and Benoit, J.: Probability based models for estimation of wildfire risk, Int. J. Wildland Fire, 13, 133–142, https://doi.org/10.1071/WF02061, 2004.
Randerson, J. T., Chen, Y., van der Werf, G. R., Rogers, B. M., and Morton, D. C.: Global burned area and biomass burning emissions from small fires, J. Geophys. Res., 117, G04012, https://doi.org/10.1029/2012JG002128, 2012.
Rodrigues, M. and de la Riva, J.: An insight into machine-learning algorithms to model human-caused wildfire occurrence, Environ. Modell. Softw., 57, 201, https://doi.org/10.1016/j.envsoft.2014.03.003, 2014.
San-Miguel-Ayanz, J., Durrant, T., Boca, R., and Camia, A.: Forest Fire Damage in Natura 2000 sites 2000–2012, European Commission and Joint Research Centre Scientific and Technical Research Report, Ispra, Italy, https://doi.org/10.2788/58397, 2012a.
San-Miguel-Ayanz, J., Schulte, E., Schmuck, G., Camia, A., Strobl, P., Liberta, G., Giovando, C., Boca, R., Sedano, F., Kempeneers, P., McInerney, D., Withmore, C., de Oliveira, S. S., Rodrigues, M., Durrant, T., Corti, P., Vilar, F. O. L., and Amatulli, G.: Comprehensive Monitoring of Wildfires in Europe: The European Forest Fire Information System (EFFIS), European Commission, Joint Research Centre and Directorate-General Environment Joint Report, Ispra, Italy, 2012b.
San-Miguel-Ayanz, J., Chuvieco, E., Handmer, J., Moffat, A., Montiel-Molina, C., Sandahl, L., and Viegas, D.: Climatological Risk, European Commission, Ispra, Italy, 294–305, 2017.
Schepers, L., Haest, B., Veraverbeke, S., Spanhove, T., Vanden Borre, J., and Goossens, R.: Burned area detection and burn severity assessment of a heathland fire in belgium using airborne imaging spectroscopy (apex), Remote Sens., 6, 1803–1826, https://doi.org/10.3390/rs6031803, 2014.
Schmuck, G., San-Miguel-Ayanz, J., Camia, A., Durrant, T., Boca, R., Libertà, G., Petroliagkis, T., Di Leo, M., Rodrigues, D., Boccacci, F., and Schulte, E.: Forest Fires in Europe, Middle East and North Africa 2011, European Commission, Joint Research Centre and Directorate-General Environment Joint Report, Ispra, Italy, https://doi.org/10.2788/44558, 2012.
Stevens, M., Demolder, H., Jacobs, S., Schneiders, A., Simoens, I., Spanhove, T., Van Gossum, P., Van Reeth, W., Michels, H., and Peymen, J.: Flanders Regional Ecosystem Assessment: State and trends of ecosystems and their services in Flanders, Research Institute for Nature and Forest, Communications of the Research Institute for Nature and Forest, Brussels, Belgium, 2015.
Syphard, A. D., Bar Massada, A., Butsic, V., and Keeley, J. E.: Land use planning and wildfire: development policies influence future probability of housing loss, Plos ONE, 8, e71708, https://doi.org/10.1371/journal.pone.0071708, 2013.
Timperman, B. and Willekens, G.: Brandgevaar dreigt in bossen – snel ingrijpen van Geelse, Molse en Grobbendonkse spuitgasten voorkomt erger, 30 July 1999, Gazet Van Antwerpen, Antwerp, Belgium, 1999.
United Nations: List of countries by population density, available at: http://statisticstimes.com/population/countries-by-population-density.php (last access: 24 August 2016), 2015.
Van Butsic, M. K. and Moritz, M. A.: Land use and wildfire: A review of local interactions and teleconnections, Land, 4, 140–156, https://doi.org/10.3390/land4010140, 2015.
Vandenberghe, R., Laurijssens, G., Vandekerkhove, K., and De Blust, G.: Geïntegreerd bos- en natuurbeheerplan voor het Schietveld Helchteren, INBO Nature Management Plan, Brussels, Belgium, 2009.
Vilar del Hoyo, L., Isabel, M., and Vega, F.: Logistic regression models for human-caused wildfire risk estimation: analysing the effect of the spatial accuracy in fire occurrence data, Eur. J. For. Res., 130, 983–996, https://doi.org/10.1007/s10342-011-0488-2, 2011.
Walloon Government, the European Commission: Programme Wallon de Développement Rural 2007–2013, Service Public de Wallonie, Jambes, Belgium, 2015.
Waumans, F., Geudens, G., and Wijns, K.: Beheerplan militair domein Vrijbos Houthulst, ANB Nature Management Plan, Holsbeek, Belgium, 2009.
Youssouf, H., Liousse, C., Roblou, L., Assamoi, E., Salonen, R. O., Maesano, C., Banerjee, S., and Annesi-Maesano, I.: Non-accidental health impacts of wildfire smoke, Int. J. Env. Res. Pub. He., 11, 11772–11804, 2014.
Zamani, S., Gobin, A., Van de Vyver, H., and Gerlo, J.: Atmospheric drought in Belgium – statistical analysis of precipitation deficit, Int. J. Climatol., 36, 3056–3071, https://doi.org/10.1002/joc.4536, 2016.