the Creative Commons Attribution 4.0 License.
the Creative Commons Attribution 4.0 License.
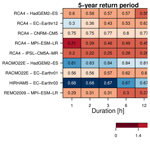
Summertime precipitation extremes in a EURO-CORDEX 0.11° ensemble at an hourly resolution
Ole B. Christensen
Katharina Klehmet
Geert Lenderink
Jonas Olsson
Claas Teichmann
Regional climate model simulations have routinely been applied to assess changes in precipitation extremes at daily time steps. However, shorter sub-daily extremes have not received as much attention. This is likely because of the limited availability of high temporal resolution data, both for observations and for model outputs. Here, summertime depth duration frequencies of a subset of the EURO-CORDEX 0.11∘ ensemble are evaluated with observations for several European countries for durations of 1 to 12 h. Most of the model simulations strongly underestimate 10-year depths for durations up to a few hours but perform better at longer durations. The spatial patterns over Germany are reproduced at least partly at a 12 h duration, but all models fail at shorter durations. Projected changes are assessed by relating relative depth changes to mean temperature changes. A strong relationship with temperature is found across different subregions of Europe, emission scenarios and future time periods. However, the scaling varies considerably between different combinations of global and regional climate models, with a spread in scaling of around 1–10 % K−1 at a 12 h duration and generally higher values at shorter durations.
- Article
(20040 KB) - Full-text XML
-
Supplement
(14016 KB) - BibTeX
- EndNote
Short duration precipitation extremes are the result of enormous quantities of atmospheric water vapour being concentrated to a relatively small area. The natural and societal landscape has large problems with coping with the huge amounts of water with resulting issues of local flooding, damages to infrastructure, landslides, erosion, etc. Theory predicts an intensification of cloudbursts with a warming climate (Trenberth et al., 2003), which makes modelling of future projections important to aid planning of robust infrastructure as well as methods to cope with diversion or delays of water in especially urban settings. Global climate models (GCMs) are generally of too coarse spatio-temporal resolution to allow detailed analysis, but some state-of-the-art regional climate model (RCM) ensemble members provide precipitation output at sufficient resolution for analysis of sub-daily extreme precipitation statistics.
Short duration extremes are often studied from an urban planning perspective, where the consequences of insufficient infrastructure to deal with, for example, cloudbursts, can be catastrophic (Willems et al., 2012). A common analysis approach is to investigate mean intensities or depths, as a function of duration and to perform extreme value analysis to determine depth–duration–frequency (DDF) functions. Mid-latitude cloudbursts have a typical dimension of 10–100 km and a duration of 1 to several hours, which sets the scale of any record for studying these type of events. For example, the highest recorded cloudburst in Sweden (in gauge observations between 1996 and 2017) lasted for 3 h in total but with extreme intensities of about 17 and 40 mm per 15 min for only two consecutive measurements. Still, the event holds the record for durations up to a few hours.
The EURO-CORDEX ensemble of high-resolution 0.11∘ (about 12 km) simulations provide the first larger ensemble with sufficient spatial resolution for studying short duration precipitation extremes (Kotlarski et al., 2014). However, RCMs and GCMs have shown severe problems with their sub-grid scale parameterizations of convective processes, which affect their ability to reproduce, for example, the diurnal cycle of rainfall intensity (Trenberth et al., 2003; Fosser et al., 2015; Prein et al., 2015; Beranová et al., 2018), the peak storm intensities (Kendon et al., 2014), and extreme hourly intensities (Hanel and Buishand, 2010). It is therefore questionable to which extent such RCMs are capable of describing short duration extremes in the present as well as in the future climate.
Olsson et al. (2015) presented increasing agreement of modelled and observed hourly precipitation with higher spatial resolution and found that the 6 km resolution of a parameterized RCM (RCA3) is in approximate agreement with gauge observations in Sweden. Similar results were obtained for Denmark, where future projections were also found to show larger increases in extreme precipitation for higher spatial resolutions and shorter temporal aggregations (Sunyer et al., 2016). Similarly, in the Mediterranean, simulated hourly rainfall has shown stronger increases in future projections than daily or multi-day rainfall (Kyselỳ et al., 2012). Convection-permitting regional models at less than about 5 km resolution have been shown to better simulate the peak structure of extreme events (Kendon et al., 2014), better agreement with observations regarding the diurnal cycle of precipitation intensity (Fosser et al., 2015; Prein et al., 2015), and improved performance of extreme hourly events (Ban et al., 2018; Coppola et al., 2018). Mediterranean heavy precipitation has been shown to be better represented in convection-permitting models, but the same models overestimate moderate to intense hourly precipitation in other regions (Berthou et al., 2018).
The fate of sub-daily precipitation extremes in a warming climate is tied to the availability of atmospheric water vapour. A warmer atmosphere can hold more water, following the Clausius–Clapeyron (CC) equation. At average mid-latitude conditions, the moisture holding capacity of the atmosphere increases at a rate of about 7 % K−1 (CC-rate), and, for example, Trenberth et al. (2003) argue that extreme convective precipitation and can be expected to intensify at or even beyond the CC-rate in a warming climate. Studies of the scaling of sub-daily precipitation extremes with temperature from present-day day-to-day variability have shown increases beyond the CC-rate (e.g. Lenderink and van Meijgaard, 2008; Berg et al., 2013; Westra et al., 2014). How such studies relate to changes in climate is debated (Bao et al., 2017; Barbero et al., 2018), and trend analysis of cloudbursts also suffers from short and non-homogeneous records leaving any potential trends unclear or non-significant (Willems et al., 2012). There are, however, some studies of precipitation extremes that present observational support for the super CC-rate derived from long-term trends in a warming climate (Guerreiro et al., 2018; Westra et al., 2013). Further, data from GCM and RCM data are generally of too coarse spatio-temporal resolution for detailed evaluation of their performance and analysis of their future projections. The scaling of hourly precipitation with increasing temperature in future projections has generally been shown to be constrained to the CC-rate. Some convection-permitting models show stronger (Kendon et al., 2014; Fosser et al., 2017; Ban et al., 2015) and some show weaker scaling compared to coarser parameterized models (Ban et al., 2018). While these high-resolution simulations show increased performance, their availability is still limited outside the research community. Therefore, the current state-of-the-art regional climate model ensemble that is being applied for climate services and local assessments for adaptation is the EURO-CORDEX 0.11∘ ensemble, which we explore here.
In this study, we evaluate the performance of four state-of-the-art regional climate models with hourly output frequency, in their ability to reproduce observed DDF statistics across Europe for the summer half-year. Future projections under the Representative Concentration Pathways (RCPs) RCP4.5 and RCP8.5 emission scenarios are then investigated, and the scaling of extreme precipitation statistics with temperature are explored. The paper starts with a presentation of the data sources (Sect. 2), followed by the applied methodology (Sect. 3), results of the evaluation and future projections (Sect. 4), and ends with a discussion (Sect. 5) and main conclusions (Sect. 6).
2.1 The EURO-CORDEX ensemble
EURO-CORDEX at 0.11∘ spatial resolution is the current state-of-the-art regional climate model ensemble over Europe. The ensemble is the result of the cooperation between many European institutions, and further ensemble members are still being added. Here, we are limited to a subset of the ensemble with members for which we have received precipitation data at a 1 h temporal resolution; see Table 1. This subset is not including the common reanalysis downscaling simulations, and the analysis is therefore of GCM–RCM combinations, which introduce some additional uncertainties (Déqué et al., 2012).
Table 1The RCM–GCM simulations with hourly precipitation output that are included in the analysis. The experiment code (“rip nomenclature”) from CMIP5 indicates the realization (r), the initialization (i), and the physics set-up (p) used. Here, the code is listed due to differences in the realizations of the EC-Earth model.
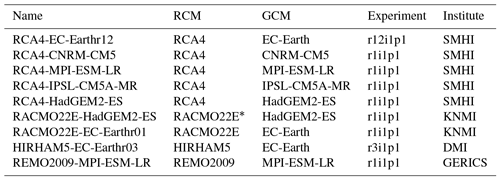
* Version 2 (v2) of the simulation as submitted to the Earth System Grid Federation (ESGF).
Kotlarski et al. (2014) give an overview of the details of the models and applied parameterizations, such as the different convective parameterizations used by the models. In the paper, they also present the performance of the RCMs in reanalysis-driven simulations, mainly discussing average quantities of precipitation and temperature. Focusing on their results for the summer season, the RCMs in the sub-ensemble used here follow the general pattern of a warm summer bias in REMO2009 in continental Europe, whereas RACMO22E has a general cold bias, and RCA4 and HIRHAM5 are too warm in the south and too cold in the north. Bias in precipitation is more scattered but follows a similar structure as the temperature bias for each of the models, indicating a strong dependency of cold and wet conditions, as can be expected for mean quantities. Prein et al. (2016) show that model bias in the EURO-CORDEX 0.11∘ simulations are reduced compared to the earlier 0.44∘ simulations, for both mean and extreme daily and 3-hourly precipitation, especially in local areas. Rajczak and Schär (2017) analysed heavy and extreme daily precipitation intensity and found good performance in RCMs, mostly independent of the driving GCM.
Jacob et al. (2014) investigated end-of-century climate change for the EURO-CORDEX 0.11∘ simulations, with significant changes in both mean precipitation and temperature across Europe for RCP4.5 and RCP8.5. Whereas mean precipitation generally increases in northern Europe and decreases in southern Europe, heavy precipitation shows robust changes across the ensemble, with significant increases in north-eastern Europe in summer, and pan-European increases in winter under RCP8.5. Kjellström et al. (2018) investigated climate change patterns as a function of global mean temperature increases of 1.5 and 2.0 ∘C, with similar results for mean precipitation and temperature as in Jacob et al. (2014). Projected precipitation extremes were investigated by Dosio (2015) and showed general increases in the annual top daily extremes and in the 95th percentile of the precipitation distribution.
The presented analysis makes use of a historical period from 1971 to 2000, as well as future scenario periods 2011–2040, 2041–2070, and 2071–2100. The analysis is restricted to summer half-years (April–September), which constitutes the main convective seasons for large parts of Europe (Berg et al., 2009). Unfortunately, as pointed out in the review process of the current paper, this interferes with the main convective season during autumn in southern France (Berthou et al., 2018) and parts of the Mediterranean. The results for those regions must therefore be handled with caution, especially in a future climate where the seasonality might shift to even later in the year (Marelle et al., 2018). RCP4.5 and RCP8.5 are investigated for all models.
2.2 National DDF data
The model simulations are evaluated against gauge based DDF curves as obtained from countries across Europe, namely Austria, Germany, Sweden, the Netherlands, and France. Much of the information about how the DDFs were calculated is only available in local languages, and the exact procedures are sometimes not clearly or sufficiently explained. Below, we provide a brief introduction to each data set but refer to references for details.
2.2.1 Sweden
The Swedish DDFs statistics were recently updated by Olsson et al. (2018a) and are available as regional tables. The statistics are based on about 125 gauge observations, with a fixed 15 min measurement interval and with data for the period 1996–2017. Durations of 15 min to 12 h were studied, using the block rainfall method, and corrected for underestimations due to the fixed 15 min interval by multiplication by 1.18, 1.08, 1.041, 1.036, and 1.029 for durations of 15 min, 30 min, 45 min, 1 h, and 2 h, respectively. No correction was deemed necessary for longer durations. The coefficients were derived by comparison with additional tipping bucket gauges and agrees approximately with earlier studies (Malitz and Ertel, 2015). Sweden was divided in four subregions, and, for each region, all stations were added to one long time series. From this time series, the POT (peak over threshold) method was applied and set up such that on average one event was selected per station and year. At least a 3 h separation was required between events for a duration of less than 3 h and a separation equal to the duration for longer durations. Then return levels were derived for several return periods, using the generalized Pareto (GP) distribution fitted using the maximum likelihood method.
2.2.2 Germany
The German DDF statistics are described in (Malitz and Ertel, 2015) and are available in the form of high-resolution spatial maps. The statistics were derived from gauge observations throughout Germany in the period May to September 1951–2010. A block rainfall method was applied based on the 5 min base resolution, with adjustments to instantaneous events by multiplication by 1.14, 1.07, 1.04, and 1.03 for 5, 10, 15, and 20 min and no adjustment for longer durations. A precipitation free time period of at least 4 h between events was required for durations below 4 h and a time period equal to the duration for longer durations. POT was applied for sub-daily values, with a threshold dependent on the length of time series such that the threshold is restricted from including more data than the number of years times 2.718. An exponential distribution was then fitted to the data, and the resulting depths were gridded across Germany for each given return period. The method is described in the KOSTRA 2010 report (Malitz and Ertel, 2015).
2.2.3 Austria
The Austrian data set (Kainz et al., 2007) comes from the Ö-KOSTRA programme, which has many similarities with the KOSTRA programme from Germany. However, due to a lower number of gauges, the data set also makes use of a convective precipitation model to support the gauge analysis. The base resolution is 5 min gauge observations with at least 10–20-year long records, and the result is a weighted mean of the gauge and model analyses. A POT approach was applied, and more details can be found in Kainz et al. (2006).
2.2.4 The Netherlands
The DDF statistics from the Netherlands are described in (Beersma et al., 2018) and are available as a country-wide table. The statistics are based on 31 gauge observations with a 10 min resolution and records of approximately 14 years in the period 2003–2016. All data were pooled and used as one long time series (436) of annual maxima. The block rainfall approach was used to find annual maxima for different durations. To accommodate the underestimation introduced when using fixed 10 min intervals rather than instantaneous measurements, a given duration of t min also considered the t+10 min duration. The generalized logistic (GLO) distribution, as an alternative to GEV (generalized extreme value) that has a “fatter” tail, was then fitted to the interval of the data with durations t min and t+10 min. Here, we are using results from Table 2 in STOWA 2018. Since this table lists durations of 1, 2, 4, 8, 12 h and we also require the 3 and 6 h durations, we derive these through a linear interpolation between 2 and 4 h, and 4 and 8 h, respectively.
2.2.5 France
The DDF statistics for France were calculated by applying the method SHYPRE (Simulated Hydrographs for flood Probability Estimation; Arnaud and Lavabre, 2002) to produce rainfall statistics across France (Arnaud et al., 2008) and are available as spatial maps. The SHYPRE method generates data for hourly extremes at a square kilometre scale, from which DDF statistics were derived. This data set is therefore treated a bit differently regarding the reduction factors, as only the spatial reduction factor is applicable; see Sect. 3.3. A complicating factor for the current study is the main convective season occurring in late Autumn in Mediterranean France, which is included in the SHYPRE all-year statistics but not in the analysed RCMs.
3.1 Durations
The DDF statistics are derived in a conventional way by employing a running window with a given duration to arrive at the peak intensity over that window; a so-called “block rain”, which does not reflect the actual event durations. We are confined here to a base resolution of 1 h, which means that the 1-hourly duration is simply taking 1 h steps and that no running mean is possible. This gives an inherent underestimation of the true hourly DDF statistics. For durations above 1 h (2, 3, 6, and 12 h are studied), the running window progresses at 1 h steps, giving a steadily more accurate estimate of the peak intensity.
3.2 Extreme value theory approach
Extreme value theory is applied to study precipitation extremes at various durations. Within extreme value theory, there are two main paths normally taken when it comes to precipitation analyses: annual maxima (AM) or POT (also called partial duration series, PDS) (Coles et al., 2001). With the AM approach (often called block maxima) a single event is selected within a block of data, typically within 1 year for geophysical time series, and with the POT approach a number of events with values greater than a given threshold are selected. The latter allows multiple events in a given year to be selected, and additional choices must be made to assure that the samples are independent and identically distributed (iid). To achieve iid samples, a minimum time separation, ts, is prescribed such that two events cannot occur too close in time. The time separation varies with the duration, d, in hours, such that
A total time range of (d+2ts(d)) h is thereby excluded from further analysis. The selected separation time is set higher than in many studies based on higher temporal resolution data (e.g. Dunkerley, 2008). Further, it is also set conservatively compared to studies based on actual event durations, i.e. defined as periods of hours from increase above a set threshold until below that threshold (Medina-Cobo et al., 2016), in contrast with the block rain approach used here. Other studies using climate model data have used even more conservative de-clustering times of 1 or 2 d (Ban et al., 2018; Chan et al., 2014a). Here, the POT approach is used, mainly because of the 30-year time slices used for the analysis, for which POT allows a more robust sample. Pickands–Balkema–de Haan's theorem (Pickands III, 1975) states that if the samples above the POT threshold are iid, they will follow a GP distribution:
where x>0, ξ is the shape and σ is the scale parameter. We use the maximum likelihood method for fitting parameters, and return values are calculated with the inverse cumulative distribution function of a GP distribution with distribution parameters and probability of exceedance, p:
where N is the number of records, n is the number of exceedances over the selected threshold, and T is the return period.
There is no well-defined method for setting the threshold for POT, but Coles et al. (2001) outlines a method of incrementally lowering the threshold, i.e. increasing the sample size and investigating the impact on the parameter fits. Comparing with a smaller sample, one event per year on average, the parameters of a larger sample must not deviate beyond the uncertainty bounds of the smaller sample. We follow Coles et al. (2001) approach as implemented in the R library “extRemes” (Gilleland and Katz, 2016) and investigate the appropriate threshold for the different durations of one member of the historical period for each RCM and in all subregions. To determine the threshold at a 95 % confidence level, we go through all grid points of each subdomain and find the average number of events per year that is rejected by at most 5 % of the grid points. The results are similar over all models, domains, and durations, and a threshold of on average three events per year was finally adapted to all grid points. This means that a sample size of 90 events is used for each extreme value fit, independent of the time slice and RCP. This amounts to thresholds across all land points ranging from about 1–30 mm h−1 for a 1 h duration, and 0.5–10 mm h−1 for a 12 h duration in the historical period. Comparisons using the Gumbel distribution calculated from annual maxima gave very similar results for the 10-year return values, although with more spatial variability (noise), which is most likely due mainly to the smaller sample size.
3.3 Comparison across spatio-temporal scales
To evaluate the model simulations, DDF statistics were collected from different national authorities across Europe. Most of these data sets are based on gauge data at minute-scale temporal resolution, which are inherently different from the about 12 km and 1-hourly data of the models (e.g. Eggert et al., 2015; Haerter et al., 2015). A direct comparison would reveal a biased comparison where gauge-based data have significantly higher return values due to their better sampling of the peak of a given duration window, as well as the peak within a precipitation area.
To alleviate this bias, we first derive area and time reduction factors that can be applied to each local data set. We make use of the Swedish radar and gauge-based data set HIPRAD (Berg et al., 2016), as well as 15 min resolution gauge records for the same domain, to derive time and areal reduction factors based on annual maxima for the years 2011–2014; see Table 2. Some grid points, primarily in northern mountainous regions of Sweden, were masked out from the analysis due to unrealistic data. In Olsson et al. (2018b), the intensity reduction for hourly aggregations between near instantaneous and 15 min gauge resolution data was studied with Swedish records and found to be about 4 % at the 1-hourly durations and negligible at a 6 h duration.
HIPRAD is originally available at a 2 km grid and 15 min resolution and was used to compare the reduction factors when both time and space coarsening is considered. When coarsening the time and space resolutions from 2 km and 15 min data to 0.11∘ and 60 min data, the reduction is about 16 % at an hourly duration and falls to only about 1 % at a 12 h duration. The final conversion factor to go from a near instantaneous point source rain gauge measurement to the 1 h and 0.11∘ resolution model data becomes the product of the time reduction factor of the gauge data and the space and time reduction factor of HIPRAD, as shown in the last line of Table 2. These factors compare well to previously applied area reduction factors (Sunyer et al., 2016); for example, Wilson (1990) presented a factor 1.279 for hourly precipitation, although at a 24 h duration the factor only decreased to 1.066 indicating a slightly too small factor in our current study. Such differences can be explained by differences in local precipitation climate and is regarded as an inherent uncertainty in this analysis. The factors are applied to the gauge-based local data sets and for the French SHYPRE data set only the space reduction factor for 60 min duration is applied.
4.1 Evaluation
Due to the different methodologies applied in the different national data sets, the evaluation mainly considers the 10-year depths, as this is well within the sample coverage of the data series and is therefore not so sensitive to the choice of method for extreme value calculations, for example, considering the use of AM or POT, or the extreme value distribution applied. The evaluation is therefore qualitative, and we focus only on the main patterns and deviations between the data sets. A general overview of the parameter fits of the extreme value distribution shows minor influence of the driving GCM, but there are differences between the RCMs. At a 12 h duration all RCMs have similar parameter values across Europe (see Figs. S1 and S2 in the Supplement) but at a 1 h duration there are more regional differences, and RACMO22E especially differs with a lower-scale parameter (see Figs. S3 and S4). The differences in the GP parameters indicate differences in the mean and variance of the events in the different RCMs, which might be due to, for example, grid point storms at short durations, as pointed out by Chan et al. (2014b).
When evaluating the DDF statistics, the reduction factors of Table 2 were applied to all national data sets, except for France where the scale gap in time is inherently bridged and only the space scale is adjusted; see Sect. 3. Figure 1 presents the evaluation results for each of the domains with local data. Since only GCM-driven simulations have been analysed, the evaluation is not purely of the RCMs, as would be approximated in reanalysis-driven simulations, but of a mixture between the driving GCM and the RCM response to that forcing. Still, RCM-dependent impacts can be seen in the results. For all domains and most models there is a clear pattern of large dry bias for a 1 h duration, with a clear decrease in bias with longer durations. The main exception from this is the REMO2009 model, which agrees better with observations across all durations. HIRHAM5 also performs better than the RCA4 and RACMO models but has a wetter bias for longer durations. The RACMO22E model produces strong underestimations of extreme intensities, mostly between about −25 % and −50 %.
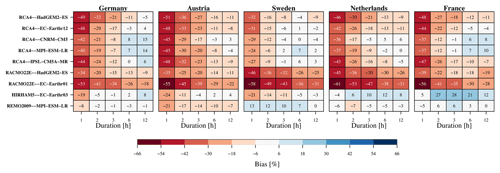
Figure 1Evaluation of model ensemble for selected regions and for the 10-year depths. Gauge-based observations have been adjusted for spatial resolution and time sampling to approximate the statistics of the model resolution and sampling, as explained in the main text. Both colours and numbers indicate the bias.
Observation-based data sets over Germany and France are available as maps, making a visual evaluation possible. Figures 2 and 3 show the 10-year depths for 1 and 12 h durations over Germany, respectively. For both presented durations, the observations show two main high-intensity regions in Germany: one in the pre-Alpine area close to the south-eastern border to Austria and one in the Black forest region oriented in north–south direction in the south-west. Intensities also tend to decrease towards the north. For the hourly duration, all severely underestimate the intensity, except for HIRHAM5 and REMO2009, as also seen in Fig. 1. Here, we see that they also fail in reproducing the spatial pattern, especially for RCA4, which fails to reproduce both the orographic regions in the south or a reversed north–south gradient. Further, the maps for HIRHAM5 and REMO2009 clearly show that although these two simulations perform better in the median intensities in Germany they also fail in reproducing the spatial pattern. The spatial analysis shows that the better performance derived from Fig. 1 is due to generally higher precipitation intensities of the REMO2009 and HIRHAM5 RCMs but not in the right locations. Only when increasing the duration to 12 h do the models start to reproduce the observed spatial patterns; see Fig. 3.
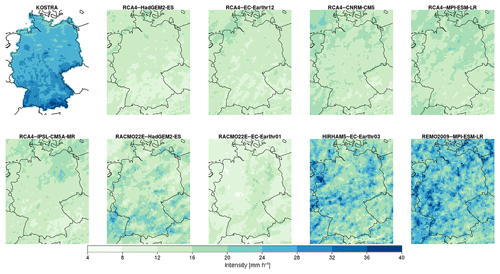
Figure 2Intensity for 10-year return period for 1 h duration of KOSTRA and all models in the RCM ensemble for Germany.
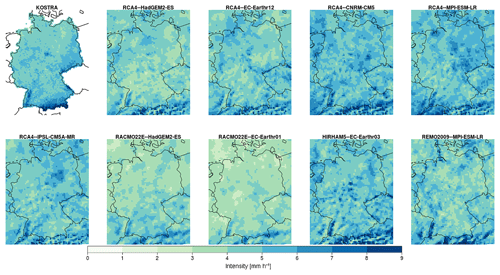
Figure 3Intensity for 10-year return period for 12 h duration of KOSTRA and all models in the RCM ensemble for Germany.
Figures 4 and 5 show similar maps for France and the observation-based data set SHYPRE. SHYPRE shows the highest intensities along the Mediterranean coastline and over the island of Corsica, and intensities decrease gradually towards the north-west. A cautionary note is in place for the comparison of the model analysed summer half-year period to the all-year statistics behind SHYPRE, which can affect conclusions for Mediterranean France with a late autumn convective season. As for Germany, all models but HIRHAM5 and REMO2009 generally underestimate 1-hourly intensities, and the peak intensity region is poorly reproduced in RCA4 and only somewhat better in the RACMO22E simulations. Within the ensemble of each individual RCM, there are variations that are likely due to the driving GCM; however, these variations are small compared to the inter-RCM spread. HIRHAM5 and REMO2009 have clear intensity maxima in the south of France that resemble those of SHYPRE. The 12-hourly durations are better simulated by all models, with the general pattern, at least, being similar to SHYPRE. However, RCA4 and RACMO22E still underestimate intensities, whereas HIRHAM5 and REMO2009 show better agreement regarding intensities.
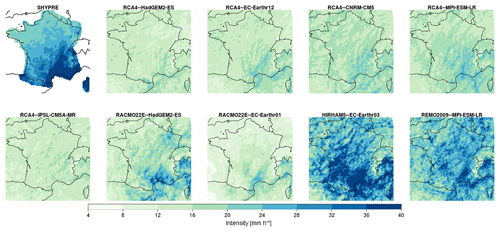
Figure 4Intensity for 10-year return period for 1 h duration of SHYPRE and all models in the RCM ensemble for France.
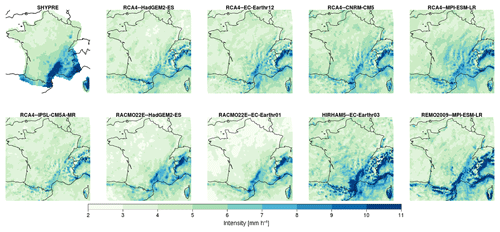
Figure 5Intensity for 10-year return period for 12 h duration of SHYPRE and all models in the RCM ensemble for France.
To complement the evaluation with a pan-European view of modelled extreme intensities, Figs. 6 and 7 show the 10-year depths for 1 and 12 h durations, respectively. At a 1 h duration, all models share a similar structure of higher intensities over the ocean west of France and the Iberian Peninsula and along the northern Mediterranean coastline, although the magnitude differs between the models. The different RCA4 simulations show that the driving GCM has some impact on the pattern across Europe. For example, HadGEM2-ES produces less intense rainfall in southern France, where the MPI-ESM-LR-driven simulation has generally more intense rainfall. However, the driving GCM seems to have less influence than the RCM. At a 12 h duration, the general patterns across Europe converge across all GCM–RCM combinations, although with differences in overall intensities; see Fig. 7. However, it is unclear from this study whether the pattern is correct or not, since observations are lacking. Earlier studies have indicated that the peak of the events is underestimated by the parameterized 0.11∘ simulations (Kendon et al., 2014), but the large bias in the 1 h durations might also indicate that small concentrated events are missing from the parameterized simulations.
The general conclusion is that depths for hourly durations are underestimated in the models, which is a likely consequence of model resolution and deficiencies in convective parameterizations. Longer duration events that also tend to have a larger spatial extent are better captured by the grid resolved component of the model simulations, where orographic effects also become more clear in the spatial patterns, in agreement with observations.
4.2 Future projections
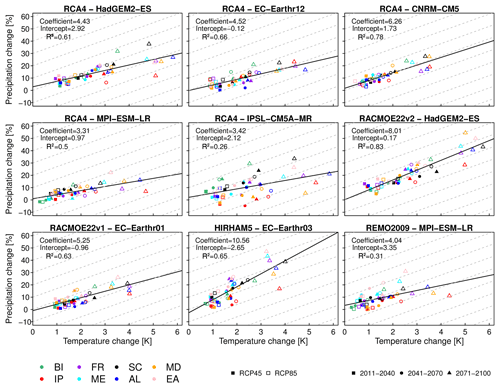
Figure 8Scatterplot of the relative change in 10-year 12 h depths against summertime mean temperature change between future and historical time periods, for different subregions, emission scenarios, and time periods according to the legend. Each panel shows the result for different RCM–GCM combinations. Linear fits to all data are presented in each panel, along with slope and intercept coefficients, as well as the R2 value of the fit. CC-rate changes of 7 % K−1 are shown as grey lines in the plots.
The performance of the RCMs in reproducing observed patterns for 12 h durations is promising enough to promote further analysis of future projections. We also include shorter durations in the analysis, despite their poor evaluation performance. Here, we investigate the response of extreme precipitation as a function of the local summer half-year (April–September) temperature change in three future time slices: 2011–2040, 2041–2070, and 2071–2100. The use of a fixed number of events, rather than setting a threshold for the POT-analysis, means that the effective threshold changes between the time slices. The thresholds are generally increasing by 15 % to 50 % for all durations when comparing the end-of-century RCP8.5 with the historical period. The analysis is performed at land-points for the so-called PRUDENCE regions (BI = British Isles, IP = Iberian Peninsula, FR = France, ME = Mid-Europe, SC = Scandinavia, AL = Alps, MD = Mediterranean, EA = Eastern Europe; Christensen and Christensen, 2007), and the depths are related to the change in mean temperature for each subregion between the future time slices and the historical reference period 1971–2000.
Figure 8 shows scatterplots of the changes in 10-year depths for precipitation of a 12 h duration, with the change in local summertime temperature for each ensemble member. The relative change in precipitation was calculated by first performing a domain average, and then calculating the change between time periods. First, it is clear that the scatterplots have strong linear trends even when considering different subregions, different time slices, and different emission scenarios. This indicates a strong connection between the change in precipitation extremes and the seasonal temperature. Second, the individual RCMs show large differences in their response depending on the driving GCM, but different RCMs also respond differently to the same GCM. Results for a 1 h duration show larger spread but good linear fit and stronger scaling (see Fig. S5).
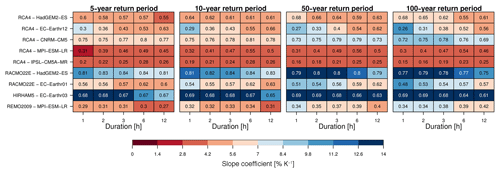
Figure 9Summary of the relative change in precipitation extremes (5, 10, 50, 100-year depths) at various return periods, against summertime temperature change between future and historical time periods for all PRUDENCE regions and RCPs and time slices together. The displayed changes are calculated as the slope coefficient of linear fit, as in Fig. 8. The colour scale is set relative the Clausius–Clapeyron prediction of about 7 % K−1, with red (blue) showing scaling below (above) that rate. The numbers in each box present the R2 value for the individual fits as a measure of the goodness-of-fit.
To further investigate the connection between extreme precipitation and seasonal temperature, we perform linear fits for each RCM–GCM combination; see Fig. 8. The results are summarized for all durations and return periods in Fig. 9, with colour coding such that increases beyond the CC-rate are in shades of blue and below the CC-rate are in shades of red. All model combinations show a positive relationship, i.e. increasing slopes, but the slopes vary from about 1 to over 10 % K−1. Most model combinations show stronger scaling for shorter durations (towards the left in each panel) and an increase in scaling with increasing return period (panels toward the right in Fig. 9). The exceptions are the models RCA4-MPI-ESM-LR and RCA4-IPSL-CM5A-MR, which remain at around 3 % K−1 scaling fairly consistently for all durations and return periods. Comparing the influence of the RCM, it is interesting to see that RCA4 driven with EC-Earth scales stronger than with HadGEM2-ES, whereas the opposite is the case for RACMO22E, although the realization of EC-Earth is different, which might have an influence that we cannot quantify in this study. REMO2009-MPI-ESM-LR has slightly stronger scaling than RCA4-MPI-ESM-LR, and HIRHAM5-EC-Earth scales much stronger than the RACMO22E and RCA4 simulations with the same GCM.
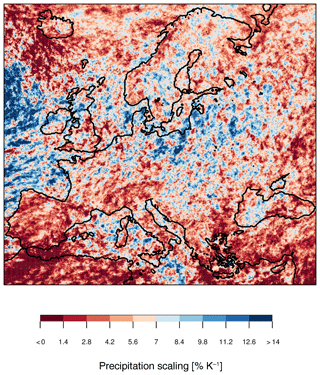
Figure 10Grand ensemble median of scaling factors (% K−1) for 10-year 12 h depths from all models, time slices, and RCPs, calculated separately for each grid point.
Figure 10 shows a grand ensemble median statistic over all models, time slices, and RCPs for each grid point. The weaker than CC temperature scaling in the Mediterranean and Iberian Peninsula land regions is clear and is likely connected to low moisture availability in summer in this region. However, a shift of the main convective season to later in autumn might influence these statistics due to the September cut-off of the investigated summer season. Stronger than CC scaling is seen mainly over water bodies but also in Ireland, the northern UK, and Sweden, which are countries with sufficient atmospheric moisture sources in a future climate as well. However, stronger than CC scaling is also seen in eastern Europe. This feature is prominent in the HIRHAM5 and REMO2009 models but also appears in some other GCM–RCM combinations, such as RACMOE22-HadGEM2-ES and RCA4-CNRM-CM5 (not shown). The regional differences in the scaling seen in Fig. 10 is also apparent on closer inspection of the individual points in Fig. 8.
Sub-daily precipitation measurements are performed throughout Europe: partly organized country-wide by the meteorological offices but frequently by local counties as well. Access to these data is mostly restricted, or simply impractical at larger scales, although initiatives such as the INTENSE project have come a long way in collecting such data (Blenkinsop et al., 2018). National DDF statistics are often available in some form, and a detailed inventory of these data sets would be a valuable first step in collecting a Europe-wide data set for evaluating model simulations. A first step was taken in this study, but a closer involvement of the data providers would be necessary to assess details of the sometimes cryptically explained data processing methods and to start an effort of homogenizing statistical methods across country borders. A further complication is that most national data sets are described only in the local native language.
The national DDF data sets were here employed as qualitative indicators for the performance of RCM simulations. Some challenges with comparing DDF statistics are due to how they were derived: using different methodologies, gauge resolution and record lengths, mixes of observations and model data, etc. The evaluation was therefore restricted to the 10-year return period, which is shorter than the gauge record lengths in all data sets and therefore less dependent on the employed extreme value estimation method. More in-depth analysis would require a larger undertaking in comparing the implications of every choice made in the different data sets and how they affect the final result. A spatial evaluation of the RCMs was performed for the German and French data sets, and here only the main patterns connected to known physical processes are discussed due to large uncertainties.
The four RCMs in the investigated model ensemble show significant differences in the simulations of extreme sub-daily precipitation. This is in spite of the similarities of several of the models. For example, the convective parameterization is similar for HIRHAM5, REMO2009, and RACMO22E, which are all based on Tiedtke (1989) but with differences in their settings and in additions to the parameterizations. Further, HIRHAM5, RACMO22E, and RCA4 share similar dynamical cores (originating from the HIRLAM NWP model). Still their responses are quite different when it comes to extreme precipitation and their response to future emission scenarios. This emphasizes the importance of the complete set of parameterizations and parameter sets in the models.
Differences in settings within the convection schemes, such as the mass flux closure used, can have a significant impact. Other parameterizations, such as turbulence scheme, surface roughness settings, or smoothing of the orography, can also significantly affect the mixing in the lower boundary and thereby affect the sensitivity of convective triggering. The effects of the parameterizations can feedback with the dynamics of the model and produce highly non-linear responses. Thus, reducing the fully three-dimensional processes into simplified one-dimensional or two-dimensional parameterizations is indeed challenging. The separation of the precipitation process into resolved and unresolved (parameterized) components is especially problematic for cloudbursts, where large-scale moisture convergence is present and can lead to positive feedback through latent heat release (Lenderink et al., 2017; Nie et al., 2018).
An important result is the apparently good performance of the RCMs HIRHAM5 and REMO2009 on domain average statistics, whilst a closer look at spatial patterns reveals an actually poor performance. More data of DDF statistics across geographical domains are essential for model evaluation, and we call out for more national institutes to open up their records and share their statistics. For example, domain average DDF statistics over the Alpine region presented in Ban et al. (2018) show fairly equal performance at a 12 and 2 km resolution. However, domain averaging might hide important differences between model simulations, which could inform about the different models' actual performance.
Scaling of precipitation extremes with future projections are studied here by comparing relative changes in precipitation intensities as a function of surface temperature increase. Recently, Ban et al. (2018) performed a similar study relating seasonal mean temperature and precipitation changes, with the result that both the 0.11∘ and 2 km simulations agree on a close to 7 % K−1 scaling. When set into context of the current study, we see that this result might be influenced by both the choice of RCM and GCM, also stressing the importance of ensembles for kilometre-scale studies.
Extreme precipitation at sub-daily timescales in the summer half-year are investigated with a EURO-CORDEX ensemble at 0.11∘ resolution. The extremes are estimated using a POT approach with a GP distribution, and the results are evaluated against national information for several countries across Europe. From the evaluations, we make the following conclusions.
-
All models perform poorly at an hourly duration, with increasing performance for longer durations.
-
Spatial patterns are reasonably well represented only at a 12 h duration, indicating a disconnect between orography and extreme events at shorter durations.
-
Both the GCM and RCM affect both magnitudes and spatial patterns across Europe, but the RCM is most prominent in shaping the spatial structure at short durations.
Future projections are investigated through a connection with summer half-year mean temperature and precipitation change for the time slice periods 2011–2040, 2041–2070, and 2071–2100. The results are presented as % K−1 changes, and we conclude the following.
-
The % K−1-scaling works well across subregions, time slices, and RCP scenarios, such that all aligns practically linearly.
-
The scaling display a large spread between models, with about an equal impact of the GCM and the RCM.
-
Scaling of extreme precipitation with temperature is positive across the model ensemble, resulting in an ensemble mean slightly below the CC-rate but ranging from about half to about 2 times the CC-rate for different ensemble members.
The concept of relating extreme precipitation changes to temperature seems to be a valid and useful approach to predict changes in extreme precipitation. However, this conclusion might be a bit rash since the performance of the models is poor for short durations and does not inspire trust in their application for future projections. The next generation of convection-permitting models might perform better, but their improved performance in reproducing the spatial pattern of extreme precipitation across domains should be investigated. For this, we urge national authorities to openly and transparently share assessments of DDF statistics from their high-resolution observations.
The hourly EURO-CORDEX data are not part of the standard suite of CORDEX and are therefore not available from the ESGF-nodes and are not produced nor shared by all model groups. The existing data can be accessed upon request from each model institute, depending on their good will and capability.
The supplement related to this article is available online at: https://doi.org/10.5194/nhess-19-957-2019-supplement.
PB and JO designed the DDF calculation strategy. WY calculated the DDFs. PB conceptualized the evaluation and future projection analysis and performed the statistical evaluation. KK performed the analysis on future projections. GL, OBC, and CT contributed with model-specific insight. PB prepared the manuscript with contributions from all co-authors.
The authors declare that they have no conflict of interest.
This work was in part funded by the projects SPEX and AQUACLEW, which is part of ERA4CS, an ERA-NET initiated by JPI Climate, and funded by FORMAS (SE), DLR (DE), BMWFW (AT), IFD (DK), MINECO (ES), and ANR (FR) with co-funding by the European Commission (grant no. 690462). We acknowledge the provision of DDF statistics from the various national authorities and our colleagues around Europe that helped with collecting the data sets for us. Further, we acknowledge Grigory Nikuling at the SMHI Rossby Centre for collecting and sharing data, and Kevin Sieck at GERICS for his input during the writing process.
This paper was edited by Joaquim G. Pinto and reviewed by three anonymous referees.
Arnaud, P. and Lavabre, J.: Coupled rainfall model and discharge model for flood frequency estimation, Water Resour. Res., 38, 11-1–11-11, https://doi.org/10.1029/2001WR000474, 2002. a
Arnaud, P., Lavabre, J., Sol, B., and Desouches, C.: Régionalisation d'un générateur de pluies horaires sur la France métropolitaine pour la connaissance de l'aléa pluviographique/Regionalization of an hourly rainfall generating model over metropolitan France for flood hazard estimation, Hydrol. Sci. J., 53, 34–47, 2008. a
Ban, N., Schmidli, J., and Schär, C.: Heavy precipitation in a changing climate: Does short-term summer precipitation increase faster?, Geophys. Res. Lett., 42, 1165–1172, https://doi.org/10.1002/2014GL062588, 2015. a
Ban, N., Rajczak, J., Schmidli, J., and Schär, C.: Analysis of Alpine precipitation extremes using generalized extreme value theory in convection-resolving climate simulations, Clim. Dynam., 1–15, https://doi.org/10.1007/s00382-018-4339-4, 2018. a, b, c, d, e
Bao, J., Sherwood, S. C., Alexander, L. V., and Evans, J. P.: Future increases in extreme precipitation exceed observed scaling rates, Nat. Clim. Change, 7, 128–132, https://doi.org/10.1038/nclimate3201, 2017. a
Barbero, R., Westra, S., Lenderink, G., and Fowler, H. J.: Temperature-extreme precipitation scaling: a two-way causality?, Int. J. Climatol., 38, e1274–e1279, https://doi.org/10.1002/joc.5370, 2018. a
Beersma, J., Versteeg, R., and Hakvoort, H.: Neerslagstatistieken voord korte duren, techreport, STOWA, 2018. a
Beranová, R., Kyselỳ, J., and Hanel, M.: Characteristics of sub-daily precipitation extremes in observed data and regional climate model simulations, Theor. Appl. Climatol., 132, 515–527, https://doi.org/10.1007/s00704-017-2102-0, 2018. a
Berg, P., Haerter, J. O., Thejll, P., Piani, C., Hagemann, S., and Christensen, J. H.: Seasonal characteristics of the relationship between daily precipitation intensity and surface temperature, J. Geophys. Res., 114, D18102, https://doi.org/10.1029/2009JD012008, 2009. a
Berg, P., Moseley, C., and Haerter, J.: Strong increase in convective precipitation in response to higher temperatures, Nat Geosci., 6, 181–185, https://doi.org/10.1038/NGEO1731, 2013. a
Berg, P., Norin, L., and Olsson, J.: Creation of a high resolution precipitation data set by merging gridded gauge data and radar observations for Sweden, J. Hydrol., 541, 6–13, https://doi.org/10.1016/j.jhydrol.2015.11.031, 2016. a
Berthou, S., Kendon, E. J., Chan, S. C., Ban, N., Leutwyler, D., Schär, C., and Fosser, G.: Pan-European climate at convection-permitting scale: a model intercomparison study, Clim. Dynam., 1–25, https://doi.org/10.1007/s00382-018-4114-6, 2018. a, b
Blenkinsop, S., Fowler, H. J., Barbero, R., Chan, S. C., Guerreiro, S. B., Kendon, E., Lenderink, G., Lewis, E., Li, X.-F., Westra, S., Alexander, L., Allan, R. P., Berg, P., Dunn, R. J. H., Ekström, M., Evans, J. P., Holland, G., Jones, R., Kjellström, E., Klein-Tank, A., Lettenmaier, D., Mishra, V., Prein, A. F., Sheffield, J., and Tye, M. R.: The INTENSE project: using observations and models to understand the past, present and future of sub-daily rainfall extremes, Adv. Sci. Res., 15, 117–126, https://doi.org/10.5194/asr-15-117-2018, 2018. a
Chan, S., Kendon, E., Fowler, H., Blenkinsop, S., and Roberts, N.: Projected increases in summer and winter UK sub-daily precipitation extremes from high-resolution regional climate models, Environ. Res. Lett., 9, 084019, https://doi.org/10.1088/1748-9326/9/8/084019, 2014a. a
Chan, S. C., Kendon, E. J., Fowler, H. J., Blenkinsop, S., Roberts, N. M., and Ferro, C. A. T.: The value of high-resolution Met Office regional climate models in the simulation of multihourly precipitation extremes, J. Climate, 27, 6155–6174, https://doi.org/10.1175/JCLI-D-13-00723.1, 2014b. a
Christensen, J. H. and Christensen, O. B.: A summary of the PRUDENCE model projections of changes in European climate by the end of this century, Clim. Change, 81, 7–30, https://doi.org/10.1007/s10584-006-9210-7, 2007. a
Coles, S., Bawa, J., Trenner, L., and Dorazio, P.: An introduction to statistical modeling of extreme values, vol. 208, Springer, 2001. a, b, c
Coppola, E., Sobolowski, S., Pichelli, E., Raffaele, F., Ahrens, B., Anders, I., Ban, N., Bastin, S., Belda, M., Belusic, D., Caldas-Alvarez, A., Cardoso, R. M., Davolio, S., Dobler, A., Fernandez, J., Fita, L., Fumiere, Q., Giorgi, F., Goergen, K., Güttler, I., Halenka, T., Heinzeller, D., Hodnebrog, Ø., Jacob, D., Kartsios, S., Katragkou, E., Kendon, E., Khodayar, S., Kunstmann, H., Knist, S., Lavín-Gullón, A., Lind, P., Lorenz, T., Maraun, D., Marelle, L., van Meijgaard, E., Milovac, J., Myhre, G., Panitz, H.-J., Piazza, M., Raffa, M., Raub, T., Rockel, B., Schär, C., Sieck, K., Soares, P. M. M., Somot, S., Srnec, L., Stocchi, P., Tölle, M. H., Truhetz, H., Vautard, R., de Vries, H., and Warrach-Sagi, K.: A first-of-its-kind multi-model convection permitting ensemble for investigating convective phenomena over Europe and the Mediterranean, Clim. Dynam., 1–32, https://doi.org/10.1007/s00382-018-4521-8, 2018. a
Déqué, M., Somot, S., Sanchez-Gomez, E., Goodess, C., Jacob, D., Lenderink, G., and Christensen, O.: The spread amongst ENSEMBLES regional scenarios: regional climate models, driving general circulation models and interannual variability, Clim. Dynam., 38, 951–964, https://doi.org/10.1007/s00382-011-1053-x, 2012. a
Dosio, A.: Projections of climate change indices of temperature and precipitation from an ensemble of bias-adjusted high-resolution EURO-CORDEX regional climate models, J. Geophys. Res.-Atmos., 121, 5488–5511, https://doi.org/10.1002/2015JD024411, 2015. a
Dunkerley, D.: Identifying individual rain events from pluviograph records: a review with analysis of data from an Australian dryland site, Hydrol. Process., 22, 5024–5036, https://doi.org/10.1002/hyp.7122, 2008. a
Eggert, B., Berg, P., Haerter, J. O., Jacob, D., and Moseley, C.: Temporal and spatial scaling impacts on extreme precipitation, Atmos. Chem. Phys., 15, 5957–5971, https://doi.org/10.5194/acp-15-5957-2015, 2015. a
Fosser, G., Khodayar, S., and Berg, P.: Benefit of convection permitting climate model simulations in the representation of convective precipitation, Clim. Dynam., 44, 45–60, https://doi.org/10.1007/s00382-014-2242-1, 2015. a, b
Fosser, G., Khodayar, S., and Berg, P.: Climate change in the next 30 years: What can a convection-permitting model tell us that we did not already know?, Clim. Dynam., 48, 1987–2003, https://doi.org/10.1007/s00382-016-3186-4, 2017. a
Gilleland, E. and Katz, R. W.: extRemes 2.0: An Extreme Value Analysis Package in R, J. Stat. Softw., 72, 1–39, https://doi.org/10.18637/jss.v072.i08, 2016. a
Guerreiro, S. B., Fowler, H. J., Barbero, R., Westra, S., Lenderink, G., Blenkinsop, S., Lewis, E., and Li, X.-F.: Detection of continental-scale intensification of hourly rainfall extremes, Nat. Clim. Change, 8, 803–807, https://doi.org/10.1038/s41558-018-0245-3, 2018. a
Haerter, J. O., Eggert, B., Moseley, C., Piani, C., and Berg, P.: Statistical precipitation bias correction of gridded model data using point measurements, Geophys. Res. Lett., 42, 1919–1929, https://doi.org/10.1002/2015GL063188, 2015. a
Hanel, M. and Buishand, T. A.: On the value of hourly precipitation extremes in regional climate model simulations, J. Hydrol., 393, 265–273, https://doi.org/10.1016/j.jhydrol.2010.08.024, 2010. a
Jacob, D., Petersen, J., Eggert, B., Alias, A., Christensen, O. B., Bouwer, L. M., Braun, A., Colette, A., Déqué, M., Georgievski, G., Georgopoulou, E., Gobiet, A., Menut, L., Nikulin, G., Haensler, A., Hempelmann, N., Jones, C., Keuler, K., Kovats, S., Kröner, N., Kotlarski, S., Kriegsmann, A., Martin, E., van Meijgaard, E., Moseley, C., Pfeifer, S., Preuschmann, S., Radermacher, C., Radtke, K., Rechid, D., Rounsevell, M., Samuelsson, P., Somot, S., Soussana, J.-F., Teichmann, C., Valentini, R., Vautard, R., Weber, B., and Yiou, P.: EURO-CORDEX: new high-resolution climate change projections for European impact research, Reg. Environ. Change, 14, 536–578, https://doi.org/10.1007/s10113-013-0499-2, 2014. a, b
Kainz, H. et al.: Forschungsprojekt “Bemessungsniederschläge in der Siedlungswasserwirtschaft”, Tech. rep., Lebensministerium, 2006. a
Kainz, H., Beutle, K., Ertl, T., Fenz, R., Flamisch, N., Fritsch, E., Fuchsluger, H., Gruber, G., Hackspiel, A., Hohenauer, R., Klager, F., Lesky, U., Nechansky, N., Nipitsch, M., Pfannhauser, G., Posch, A., Rauch, W., Schaar, W., Schranz, J., Sprung, W., Telegdy, T., and Lehner, F.: Niederschlagsdaten zur Anwendung der ÖWAV-Regelblätter 11 und 19, Tech. rep., ÖWAV, 2007. a
Kendon, E. J., Roberts, N. M., Fowler, H. J., Roberts, M. J., Chan, S. C., and Senior, C. A.: Heavier summer downpours with climate change revealed by weather forecast resolution model, Nat. Clim. Change, 4, 570–576, https://doi.org/10.1038/nclimate2258, 2014. a, b, c, d
Kjellström, E., Nikulin, G., Strandberg, G., Christensen, O. B., Jacob, D., Keuler, K., Lenderink, G., van Meijgaard, E., Schär, C., Somot, S., Sørland, S. L., Teichmann, C., and Vautard, R.: European climate change at global mean temperature increases of 1.5 and 2 ∘C above pre-industrial conditions as simulated by the EURO-CORDEX regional climate models, Earth Syst. Dynam., 9, 459–478, https://doi.org/10.5194/esd-9-459-2018, 2018. a
Kotlarski, S., Keuler, K., Christensen, O. B., Colette, A., Déqué, M., Gobiet, A., Goergen, K., Jacob, D., Lüthi, D., van Meijgaard, E., Nikulin, G., Schär, C., Teichmann, C., Vautard, R., Warrach-Sagi, K., and Wulfmeyer, V.: Regional climate modeling on European scales: a joint standard evaluation of the EURO-CORDEX RCM ensemble, Geosci. Model Dev., 7, 1297–1333, https://doi.org/10.5194/gmd-7-1297-2014, 2014. a, b
Kyselỳ, J., Beguería, S., Beranová, R., Gaál, L., and López-Moreno, J. I.: Different patterns of climate change scenarios for short-term and multi-day precipitation extremes in the Mediterranean, Global Planet. Change, 98, 63–72, https://doi.org/10.1016/j.gloplacha.2012.06.010, 2012. a
Lenderink, G. and van Meijgaard, E.: Increase in hourly precipitation extremes beyond expectations from temperature changes, Nat. Geosci., 1, 511–514, https://doi.org/10.1038/ngeo262, 2008. a
Lenderink, G., Barbero, R., Loriaux, J. M., and Fowler, H. J.: Super-Clausius-Clapeyron scaling of extreme hourly convective precipitation and its relation to large-scale atmospheric conditions, J. Climate, 30, 6037–6052, https://doi.org/10.1175/JCLI-D-16-0808.1, 2017. a
Malitz, G. and Ertel, H.: KOSTRA-DWD2010: Starkniederschalgshöhen für Deutschland (Bezugszeitraum 1951 bis 2010), techreport, Deutscher Wetterdienst, availabel at: https://www.dwd.de/DE/leistungen/kostra_dwd_rasterwerte/download/bericht_kostra_dwd_2010_pdf.html (last access: 29 April 2019), 2015. a, b, c
Marelle, L., Myhre, G., Hodnebrog, Ø., Sillmann, J., and Samset, B. H.: The changing seasonality of extreme daily precipitation, Geophys. Res. Lett., 45, 11–352, https://doi.org/10.1029/2018GL079567, 2018. a
Medina-Cobo, M., García-Marín, A., Estévez, J., and Ayuso-Muñoz, J.: The identification of an appropriate Minimum Inter-event Time (MIT) based on multifractal characterization of rainfall data series, Hydrol. Process., 30, 3507–3517, https://doi.org/10.1002/hyp.10875, 2016. a
Nie, J., Sobel, A. H., Shaevitz, D. A., and Wang, S.: Dynamic amplification of extreme precipitation sensitivity, P. Natl. Acad. Sci. USA, 115, 9467–9472, https://doi.org/10.1073/pnas.1800357115, 2018. a
Olsson, J., Berg, P., and Kawamura, A.: Impact of RCM Spatial Resolution on the Reproduction of Local, Subdaily Precipitation, J. Hydrometeorol., 16, 534–547, https://doi.org/10.1175/JHM-D-14-0007.1, 2015. a
Olsson, J., Berg, P., Eronn, A., Simonsson, L., Södling, J., Wern, L., and Yang, W.: Extremregn i nuvarande och framtida klimat: analyser av observationer och framtidsscenarier, Klimatologi 47, SMHI, 2018a. a
Olsson, J., Södling, J., Berg, P., Wern, L., and Eronn, A.: Short-duration rainfall extremes in Sweden: a regional analysis, Hydrol. Res., 1–16, https://doi.org/10.2166/nh.2019073, 2018b. a
Pickands III, J.: Statistical inference using extreme order statistics, Ann. Stat., 3, 119–131, https://doi.org/10.1214/aos/1176343003, 1975. a
Prein, A. F., Langhans, W., Fosser, G., Ferrone, A., Ban, N., Goergen, K., Keller, M., Tölle, M., Gutjahr, O., Feser, F., Brisson, E., Kollet, S., Schmidli, J., van Lipzig, N. P. M., and Leung, R.: A review on regional convection-permitting climate modeling: Demonstrations, prospects, and challenges, Rev. Geophys., 53, 323–361, https://doi.org/10.1002/2014RG000475, 2015. a, b
Prein, A. F., Gobiet, A., Truhetz, H., Keuler, K., Goergen, K., Teichmann, C., Fox Maule, C., van Meijgaard, E., Déqué, M., Nikulin, G., Vautard, R., Colette, A., Kjellström, E., and Jacob, D.: Precipitation in the EURO-CORDEX 0.11∘ and 0.44∘ simulations: high resolution, high benefits?, Clim. Dynam., 46, 383–412, https://doi.org/10.1007/s00382-015-2589-y, 2016. a
Rajczak, J. and Schär, C.: Projections of future precipitation extremes over Europe: A multimodel assessment of climate simulations, J. Geophys. Res., 122, 10,773–10,800, https://doi.org/10.1002/2017JD027176, 2017. a
Sunyer, M. A., Luchner, J., Onof, C., Madsen, H., and Arnbjerg-Nielsen, K.: Assessing the importance of spatio-temporal RCM resolution when estimating sub-daily extreme precipitation under current and future climate conditions, Int. J. Climatol., 37, 688–705, https://doi.org/10.1002/joc.4733, 2016. a, b
Tiedtke, M.: A comprehensive mass flux scheme for cumulus parameterization in largescale models, Mon. Weather Rev., 117, 1779–1800, 1989. a
Trenberth, K. E., Dai, A., Rasmussen, R. M., and Parsons, D. B.: The changing character of precipitation, B. Am. Meteorol. Soc., 84, 1205–1217, https://doi.org/10.1175/BAMS-84-9-1205, 2003. a, b, c
Westra, S., Alexander, L. V., and Zwiers, F. W.: Global Increasing Trends in Annual Maximum Daily Precipitation, J. Climate, 26, 3904–3918, https://doi.org/10.1175/jcli-d-12-00502.1, 2013. a
Westra, S., Fowler, H., Evans, J., Alexander, L., Berg, P., Johnson, F., Kendon, E., Lenderink, G., and Roberts, N.: Future changes to the intensity and frequency of short-duration extreme rainfall, Rev. Geophys., 52, 522–555, 2014. a
Willems, P., Olsson, J., Arnbjerg-Nielsen, K., Beecham, S., Pathirana, A., Gregersen, I. B., Madsen, H., and Nguyen, V.-T.-V.: Impacts of climate change on rainfall extremes and urban drainage systems, IWA Publishing, 2012. a, b
Wilson, E. M.: Engineering Hydrology, Macmillan Education UK, London, 1–49, https://doi.org/10.1007/978-1-349-11522-8_1, 1990. a