the Creative Commons Attribution 4.0 License.
the Creative Commons Attribution 4.0 License.
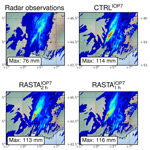
Assimilation of wind data from airborne Doppler cloud-profiling radar in a kilometre-scale NWP system
Mary Borderies
Olivier Caumont
Julien Delanoë
Véronique Ducrocq
Nadia Fourrié
The article reports on the impact of the assimilation of wind vertical profile data in a kilometre-scale NWP system on predicting heavy precipitation events in the north-western Mediterranean area. The data collected in diverse conditions by the airborne W-band radar RASTA (Radar Airborne System Tool for Atmosphere) during a 45-day period are assimilated in the 3 h 3DVAR assimilation system of AROME. The impact of the length of the assimilation window is investigated. The data assimilation experiments are performed for a heavy rainfall event, which occurred over south-eastern France on 26 September 2012 (IOP7a) and over a 45-day cycled period. Results indicate that the quality of the rainfall accumulation forecasts increases with the length of the assimilation window, which recommends using observations with a large period centred on the assimilation time. The positive impact of the assimilation of RASTA wind data is particularly evidenced for the IOP7a case since results indicate an improvement in the predicted wind at short-term ranges (2 and 3 h) and in the 11 h precipitation forecasts. However, in the 45-day cycled period, the comparison against other assimilated observations shows an overall neutral impact. Results are still encouraging since a slight positive improvement in the 5, 8 and 11 h precipitation forecasts was demonstrated.
- Article
(5891 KB) - Full-text XML
- BibTeX
- EndNote
The Mediterranean area is frequently subject to heavy precipitation events, causing heavy damage and significant human loss (Ducrocq et al., 2014). Over the last years, numerical weather prediction (NWP) models have been operationally implemented to improve the accuracy and timely prediction of such severe weather. The quality of the predictions depends, among others, on the initial atmospheric state. Several studies suggested that the impact of the assimilation of wind observations was beneficial for analyses and forecasts (Horányi et al., 2015).
Over land, ground-based Doppler precipitation radar data are now operationally assimilated in kilometre-scale NWP systems, since their potential to improve the short-term forecasts has been demonstrated (Montmerle and Faccani, 2009; Simonin et al., 2014). In clear-air conditions, wind observations can be provided by insect-derived Doppler radar measurements (Kawabata et al., 2007; Rennie et al., 2011) or by Doppler lidars (Weissmann et al., 2012; Kawabata et al., 2014). To fill the gap in clear-air conditions, radar wind profilers provide vertical profiles of the horizontal wind at a high vertical resolution. Several studies highlighted the benefit of the assimilation of these data into NWP models to improve short-term forecasts (Benjamin et al., 2004; Illingworth et al., 2015b). However, the main drawback of ground-based radars and radar profilers is that they are only distributed over land.
Because wind observations are too sparse over ocean, atmospheric motion vectors (AMVs) are now operationally derived using the movement of cloud and water vapour tracers from consecutive satellite images. They provide tropospheric wind data measurements on a global scale with a high temporal resolution. Recent studies indicate an overall positive impact of the assimilation of AMV data in NWP models on the subsequent forecasts (Deb et al., 2016; Kumar et al., 2017). Nevertheless, contrary to most active sensors, AMV measurements do not provide wind vertical profile information but only cloud-top information. Besides, there is an uncertainty in the knowledge of the observed cloud-top level (Salonen et al., 2015).
To fill the gap within the existing observing system, Baker et al. (2014) highlighted the need for extra wind vertical profile measurements over ocean to improve the initial conditions for NWP forecasts. This need for new wind measurements particularly applies in the Mediterranean region since offshore convective systems, which are responsible for heavy precipitation events, are not well predicted by kilometre-scale NWP models (Duffourg et al., 2016; Martinet et al., 2017). In the near future, the Doppler W-band radar on board the EarthCare satellite mission (scheduled to be launched in middle 2021, Illingworth et al., 2015a) will provide vertical profiles of wind data from Doppler radar at a high vertical resolution over land and over sea for the first time. In the meantime, the WIVERN satellite concept mission carrying a conically scanning Doppler W-band radar is also being conceived (Illingworth et al., 2018). So far, the impact of the assimilation of wind vertical profiles from W-band radar has never been investigated.
Airborne Doppler radars have the advantage of collecting a large data set of measurements over land and sea on very fine scales. Pu et al. (2009) showed that the 3DVAR assimilation of wind data from airborne Doppler radar results in significant improvement in the intensity and precipitation forecasts of Hurricane Dennis. Following on, Li et al. (2014) demonstrated the benefits of the 4DVAR assimilation of the ELDORA X-band radar velocity data in the simulation of Hurricane Nuri's genesis. The positive impact due to airborne Doppler velocity data assimilation for hurricane forecasts has also been investigated with an ensemble Kalman filter by Weng and Zhang (2012). So far, this kind of study has never been done in the Mediterranean area. In addition, the measurements used in the hurricane studies listed above were collected with side-looking radar (elevation angle ≤70∘) at lower frequencies (X or C bands).
The primary objective of this article is to evaluate for the first time the impact of assimilating wind profiles retrieved by airborne W-band radar in a kilometre-scale NWP model. The current study covers a 2-month period with the airborne Doppler W-band radar RASTA (Radar Airborne System Tool for Atmosphere) during the HyMeX (HYdrological cycle in the Mediterranean eXperiment, Drobinski et al., 2014) first Special Observation Period (HyMeX-SOP1, Ducrocq et al., 2014) over a region of the Mediterranean area prone to heavy rainfall. The main goal of the HyMeX-SOP1 was to document the heavy precipitation events that regularly affect north-western Mediterranean coastal areas. RASTA is a multibeam antenna system (six beams in total) that allows the documentation of the three components of the wind field in the vertical at a high resolution of 60 m and quasi-continuously in time during the flights. The current assimilation study is performed in a quasi-operational framework, using a version of the Météo-France operational kilometre-scale model AROME (named AROME-WMED) specifically designed for the HyMeX-SOP1, with its 3DVAR assimilation system associated with a 3 h assimilation cycle.
To assess the potential of RASTA wind data to improve short-term forecasts, a series of experiments are first conducted for a heavy rainfall event, which occurred during the Intensive Observation Period 7a (IOP7a) over south-eastern France on 26 September 2012. Next, a cycling data assimilation run is conducted over a 45-day period from 24 September to 5 November 2012 in order to study the impact of the assimilation of RASTA wind data in various conditions during the whole HyMeX-SOP1. This article investigates the impact of the choice of the assimilation window in a 3DVAR system. Indeed, data from moving platforms, such as RASTA, have the disadvantage of not being measured simultaneously at the assimilation time but over the flight leg. A small assimilation window constrains the number of assimilated data to those that are nearly valid at the assimilation time. By contrast, a larger assimilation window leads to a larger coverage but with observations which might be no longer valid. Therefore, a sensitivity study to the assimilation window is performed in this study.
This article is organized as follows. In Sect. 2, the airborne Doppler W-band radar RASTA and the period of study are described. The kilometre-scale NWP model AROME-WMED with its 3DVAR assimilation system are then presented in Sect. 3. Following on, the different model simulations are detailed in Sect. 4. Finally, the evaluation of the different experiments is first focused on IOP7a in Sect. 5, followed by a statistical evaluation over the whole HyMeX SOP1 in Sect. 6.
The Doppler W-band radar RASTA is first described in Sect. 2.1, and details about the data collected by RASTA during the HyMeX first Special Observation Period (SOP1) field campaign are then briefly given in Sect. 2.2.
2.1 The Doppler W-band radar RASTA
The airborne cloud radar RASTA is a monostatic Doppler multibeam antenna system operating at 95 GHz (Bouniol et al., 2008; Protat et al., 2009; Delanoë et al., 2013). The aircraft platform used is the French Falcon 20 research aircraft from the SAFIRE unit (Service des Avions Français Instrumentés pour la Recherche en Environnement). This unique instrument allows the documentation of the microphysical properties and the three components of the wind field in the vertical at a high resolution of 60 m and quasi-continuously in time during the flights.
The radar RASTA is equipped with six Cassegrain antennas pointing either upward (antennas 1–3) or downward (antennas 4–6). Therefore, RASTA measures the reflectivity and the radial velocity in three non-collinear directions above and below the aircraft in the clouds. A schematic figure of the RASTA configuration during the HyMeX-SOP1 is given by Bousquet et al. (2016), their Fig. 1. The radial velocity measurements are collected at a vertical resolution of 60 m and a time resolution of 250 ms (i.e. 1.5 s between two measurements of the same antenna). The maximum range is 15 km with a Nyquist velocity of 7.8 m s−1 (the pulse repetition frequency equals 10 kHz).
The data processing described by Bousquet et al. (2016) is applied to RASTA wind observations. First, the exact speed of the aircraft and the pointing angles are used to rigorously determine the component related to the aircraft's movement. Doppler measurements are then processed by removing the projection of aircraft ground speed along the six antenna beams. Next, Doppler velocities are unfolded using an in situ wind sensor for the first gate and by applying a gate-to-gate correction for the other gates. In addition to that, the combination of the three non-collinear beams is used to verify potential unfolding issues as the retrieval would be locally inconsistent. For ground-pointing antennas, a check-up is conducted in order to ensure that ground return velocities are close to 0 m s−1. Upward-looking antennas errors are estimated and corrected by ensuring continuity between the data collected above and below the aircraft. After processing, the Doppler velocity of the three downward-looking and upward-looking antennas are combined to retrieve the horizontal and vertical wind components above and below the aircraft. More details on the RASTA configuration during HyMeX can be found by Bousquet et al. (2016). The retrieved horizontal wind components will be assimilated in the 3DVAR assimilation system of AROME-WMED.
2.2 RASTA data during the HyMeX first Special Observation Period (SOP1)
This study takes advantage of the data collected by RASTA during the HyMeX SOP1, which took place from 5 September to 5 November 2012 over the western Mediterranean (Ducrocq et al., 2014). The main goal of the SOP1 was to document the heavy rainfall events that regularly affect north-western Mediterranean coastal areas. During the 2-month campaign, approximately 20 rainfall events were documented in France, Italy and Spain (Ducrocq et al., 2014). Specifically, the RASTA radar aboard the Falcon 20 collected data during 18 flights in and around mesoscale convective systems in diverse conditions.
The data collected by RASTA during the SOP1 offer a wide variety of conditions over land, sea and complex terrains. Among all the observed vertical columns over the SOP1, 72.6 % were collected in stratiform areas, 13.1 % in clear sky and 14.3 % in convective areas (Borderies et al., 2018). RASTA flight paths during the HyMeX SOP1 are represented by the black lines in Fig. 1.
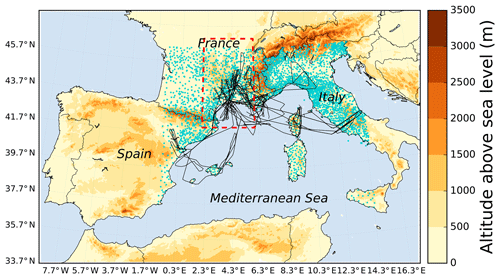
Figure 1The Falcon 20 flight paths (black lines) during the HyMeX first Special Observation Period over the AROME-WMED domain. The altitude of ground above sea level (in metres) is represented by the coloured gradient. Rain gauges are represented by the blue markers. The area surrounding the IOP7a case study is indicated by the red box.
3.1 The AROME-WMED NWP model
This study is conducted with AROME-WMED (Fourrié et al., 2015), the HyMeX-dedicated version of the Météo-France operational kilometre-scale NWP model AROME (Seity et al., 2011). AROME-WMED, which covers the entire north-western Mediterranean Basin, was specially designed for the HyMeX-SOP1 and ran in real time to plan the airborne operations in advance, especially in the mesoscale convective systems. AROME-WMED is based on the AROME-France version operationally employed in 2012: the deep convection is explicitly resolved and the microphysical processes are governed by the ICE3 one-moment bulk microphysical scheme (Pinty and Jabouille, 1998). AROME-WMED runs at a horizontal resolution of 2.5×2.5 km with 60 vertical levels, ranging from approximately 10 m above ground level to 1 hPa. Compared to AROME, AROME-WMED covers an extended domain centred on the north-western Mediterranean area. The AROME-WMED domain is displayed in Fig. 1. It has 948×628 horizontal grid points, which is equivalent to a horizontal size of 2370×1570 km2. In addition, to increase the observation coverage in the southern part of the domain, more satellite (AMSU) and ground-based Spanish weather station observations are assimilated in AROME-WMED.
3.2 3DVAR assimilation system
AROME-WMED has a three-dimensional variational (3DVAR) data assimilation system (Brousseau et al., 2011) associated with a 3 h assimilation cycle. It is based on an incremental formulation (Fischer et al., 2005) and the control variables are temperature, specific humidity, surface pressure, vorticity and divergence. AROME-WMED background error covariances were computed using a period in October 2010 characterized by convective systems over the north-western Mediterranean region (Fourrié et al., 2015).
Every 3 h an analysis is computed by using all observations available within a ±1 h 30 min assimilation window and a 3 h forecast to produce a first guess for the next cycle. The assimilation system ingests a wide variety of observations from satellite, ground-based Global Navigation Satellite System (GNSS), aircraft, radiosondes, drifting buoys, balloons and wind profilers, automatic land and ship weather stations, and ground-based radars of the French network ARAMIS (reflectivity and radial velocity).
To assess the potential of RASTA wind data to improve short-term forecasts of heavy precipitation events, a total of four experiments is conducted over a 45-day cycled period during the HyMeX-SOP1. Focus is also made on one of the most significant episodes which occurred within France during the HyMeX SOP1 campaign on 26 September 2012.
4.1 RASTA wind data pre-processing
First, “super-observations” are created to reduce observation and representativeness errors. They are calculated by interpolating RASTA wind data in the model vertical and horizontal resolutions. This interpolation is done by taking the median value of all data available along the aircraft track within a box of 2.5 km length between the two half model levels surrounding each model level. Applying a median filter instead of averaging allows the influence of outliers to be reduced due to the difficulty of having high-quality measurements for airborne Doppler radar (Bosart et al., 2002). Indeed, after the data processing described in Sect. 2.1, some spurious data were still occasionally present. Using a median filter instead of a mean filter helps to reduce the weight that these spurious observations can have in the calculation of RASTA wind super-observations.
When the aircraft roll and/or pitch angles are too high (i.e. if in Fig. 2, with R being the range from the radar), some data might not be in the same box at a given range from the aircraft (for instance in the box number 3 in Fig. 2). Therefore, these data are not taken into account.
After this pre-processing, to satisfy assumptions about observation error covariances, which are supposed to be 0 m2 s−2, a thinning is applied to RASTA wind super-observations. One super-observation out of three is then assimilated, which is equivalent to approximately one observation every 5 to 9 km depending on the aircraft speed.
4.2 Experimental set-up
RASTA wind data are not measured simultaneously but over the flight leg. Therefore, at each assimilation time T from 00:00 to 21:00 UTC, the 3DVAR assimilation system of AROME-WMED ingests all RASTA wind data available during an assimilation window Δt centred on the assimilation time T, as if they were valid at the time T. Too large an assimilation window Δt would result in assimilating data that are no longer valid at the current assimilation time T, especially for convective systems which can evolve quickly in time. On the other hand, it is likely that the impact will be neutral if the assimilation window is too short, because less data are assimilated. Therefore, the impact of the assimilation of RASTA wind data is tested with three different assimilation windows Δt: 3 h (RASTA3 h), 2 h (RASTA2 h) and 1 h (RASTA1 h) centred on the assimilation time T.
Finally, four different experimental designs are defined. The analyses of the global operational NWP model ARPEGE are used to initialize the experiments and to provide boundary conditions. In the control (CTRL) experimental design, only the observations that are operationally assimilated are taken into account (see Sect. 3.2). The three additional RASTA experimental designs (RASTA3 h, RASTA2 h and RASTA1 h) share the same configuration as CTRL but include the assimilation of RASTA wind data every 3 h from 00:00 to 21:00 UTC.
Because the Doppler multibeam antenna system of RASTA can retrieve the horizontal wind components (u, v), which are linked to two control variables of AROME-WMED (vorticity and divergence), the assimilation of RASTA wind data is straightforward and does not require the use of a radial wind observation operator. Bousquet et al. (2016) demonstrated that the root-mean-square error of RASTA wind data vs. ground-based centimetre-wavelength radars is of the order of 4 m s−1. They argued that this error mainly originated from the sampling volume of ground-based radars being much larger than that of RASTA. In this study, it has been decided to use the same observation error as the one used for radiosondes, which increases with altitude (from ≈1.8 m s−1 at 900 hPa to ≈2.52 m s−1 at 200 hPa). Finally, in addition to the pre-processing described in Sect. 4.1, a quality control is also performed prior to the assimilation: observations with innovation (observations − background) greater than a threshold are rejected. This threshold depends on both the observation and background errors.
First, the four different experimental designs are run during a 45-day cycled period from 00:00 UTC 24 September 2012, which is the day when the Falcon 20 first flew during HyMeX-SOP1, to 5 November 2012, after the last flight. During this period, the different assimilation experiments are named CTRLSOP1, RASTA, RASTA and RASTA. The number of assimilated data over the covered period are represented as a function of the pressure level in Fig. 3 for the three RASTA experiments. Table 1 summarizes the different assimilation experiments. The fourth column shows the percentage of analyses in which RASTA wind data were assimilated over the total number of analyses (360) during the 45-day cycled period for the different RASTA experiments. A larger assimilation window results in assimilating data more frequently, but the time lag between the observation time and the analysis time is greater than 1 h. On the other hand, a smaller assimilation window constrains the number of analyses to those for which the observations are valid near the analysis time. Therefore, the percentage of analyses in which RASTA wind data were assimilated decreases with the length of the assimilation window from 9.5 % in the RASTA experiment to 7.2 % in the RASTA experiment. Finally, the last column of Table 1 represents the percentage of RASTA wind data which were assimilated among the total number of assimilated data (conventional, GNSS, radar, satellite, RASTA, etc.) over the entire AROME-WMED domain (represented in Fig. 1). This percentage is quite small because of the already dense observing network used in AROME-WMED.
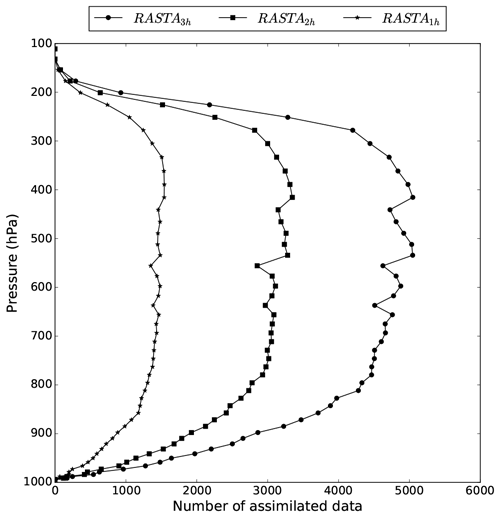
Figure 3Number of RASTA horizontal wind data that are assimilated as a function of pressure for the RASTA, RASTA and RASTA1 h.
Finally, the four different experimental designs are also run on a heavy precipitation event which occurred during the Intensive Observation Period 7a (IOP7a) on 26 September 2012 during the morning. The CTRLIOP7, RASTA, RASTA and RASTA experiments start at 00:00 UTC on 26 September 2012 and end at 12:00 UTC on 26 September 2012.
The impact of RASTA wind data is first illustrated on a heavy precipitation event which occurred during the Intensive Observation Period 7a (IOP7a) on 26 September 2012.
5.1 Case description: IOP7a
The IOP7a precipitation event is one of the most significant episodes that occurred within France during the HyMeX SOP1 campaign (Hally et al., 2014). This case study was located over south-eastern France in the area delimited by the red box in Fig. 1, which has been enlarged in Fig. 4. The precipitation event consisted of a convective line over the mountainous region and a band of stratiform rainfall over the Gard and the Ardèche departments. More than 100 mm of rain was observed between 00:00 UTC on 26 September and 00:00 UTC on 27 September. A first peak of rainfall accumulation is observed in the morning at 08:00 UTC and a second one in the late afternoon at 17:00 UTC. This event is further described by Hally et al. (2014).
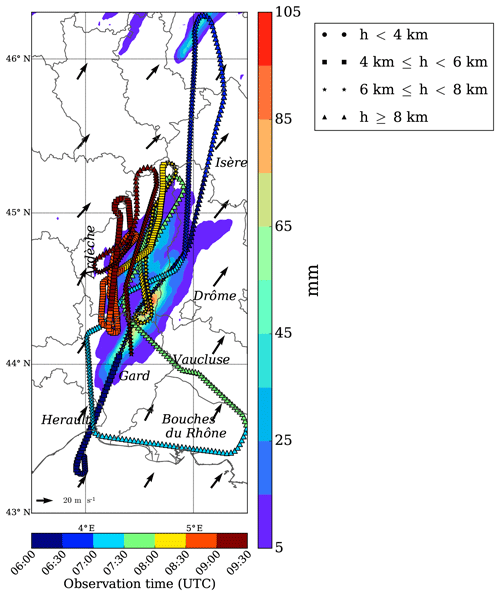
Figure 4Area under the red box in Fig. 1: 24 h accumulated rainfall observed by weather radar between 00:00 UTC on 26 September and 00:00 UTC on 27 September is represented by the shadings (scale on the right). The observation time along the Falcon 20 flight path is indicated by the coloured data points (scale on the bottom). The circle, square, star and triangle markers indicate the aircraft's altitude (see legend). Arrows represent the wind direction from the CTRLIOP7 analysis at 06:00 UTC at approximately 4 km of altitude (model level 30).
During the IOP7a, RASTA data were collected during Flight 15 between 06:10 and 09:45 UTC. Therefore, RASTA wind data are assimilated for the first time at 06:00 UTC. Since the Falcon 20 took off at 06:10 UTC, the RASTA experiment assimilates all the RASTA wind data that are available between 06:10 and 06:30 UTC, as if they were valid at 06:00 UTC. Similarly, the RASTA (RASTA) experiment assimilates RASTA wind data until 07:00 UTC (07:30 UTC) as if they were valid at 06:00 UTC.
The observation time along the aircraft flight path is represented by the coloured data points in Fig. 4. Figure 4 shows that data were mainly collected in the area where the band of rainfall was located, over the Ardèche and the Gard departments. In particular, most of the data that are assimilated at the 06:00 UTC analysis (before an observation time of 07:30 UTC) are located upwind of where the rainfall event occurred over the Ardèche department. Therefore, the assimilation of RASTA wind data at 06:00 UTC is expected to have an impact on the forecasts, especially for the first peak of rainfall accumulation, which occurred in the morning.
5.2 Impact on analyses
Figure 5 shows (from the top to the bottom) the wind speed (left panels, a to e) and the wind direction (right panels, f to j) for the observations, the CTRLIOP7, the RASTA, the RASTA and the RASTA analyses. The different analyses were computed using the same background state. The three different assimilation windows Δt are delimited by the vertical lines.
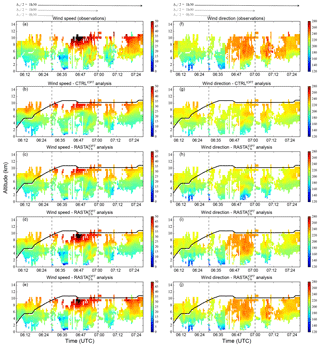
Figure 5Wind speed (a–e) and wind direction (f–j) for (a, f) the observations, (b, g) the CTRLIOP7, (c, h) the RASTA, (d, i) the RASTA and (e, j) the RASTA 06:00 UTC analyses on 26 September 2012 (IOP7a). The three different assimilation windows Δt are delimited by the vertical lines. The aircraft's altitude above sea level is represented by the black line.
As expected, Fig. 5 indicate a better agreement with the observations if RASTA wind data are assimilated in terms of both direction and speed. The RASTA, RASTA and RASTA experiments assimilate all the observations until 06:30, 07:00 and 07:30 UTC, respectively. These different time limitations explain the differences in wind and direction between the different RASTA experiments.
Even though the three RASTA analyses are very similar to each other within their respective assimilation windows Δt, at 06:30 UTC the RASTA (panel e) and the RASTA (panel d) experiments exhibit larger velocities at 10 km of altitude than the RASTA (panel c) experiment. This discrepancy is explained by the fact that the aircraft does not have a rectilinear trajectory and passes over the same location several times. In particular, Fig. 4 shows that RASTA collected data at the same location at 06:30 and at 07:00 UTC. In such a case, all data are assimilated as if they were equally valid at the assimilation time T (06:00 UTC here). This overpass explains why the RASTA and the RASTA are slightly different from the RASTA experiment at 06:30 UTC, in terms of both direction and speed. Similarly, there is an overpass of the aircraft at 06:15 and at 07:20 UTC. At this location, while the RASTA and the RASTA experiments only assimilate the data available at 06:15 UTC, the RASTA experiment also assimilates the data collected at 07:20 UTC. However, the wind has increased between 06:00 and 07:30 UTC. Hence, the RASTA experiment exhibits higher velocity at 06:15 UTC and a different direction (panels e and j) at approximately 10 km of altitude, compared to the RASTA (panels c and h) and the RASTA (panels d and i) experiments.
Figure 6a represents the wind speed increments at approximately 4 km of altitude (model level 30) between the RASTA and the CTRLIOP7 analysis. Wind directions are also indicated by the green (or black) arrows for the CTRLIOP7 (or RASTA) analysis. The data points assimilated in the RASTA experiment until 07:30 UTC are also represented by the black data points. As expected, the analysis increments are well localized around the aircraft flight path. The assimilation of RASTA wind data has a large impact on the analysis since the increments can reach a value of approximately 12 m s−1. The same behaviour is also seen when RASTA wind data are assimilated with smaller assimilation windows (Δt=2 h and Δt=1 h, not shown).
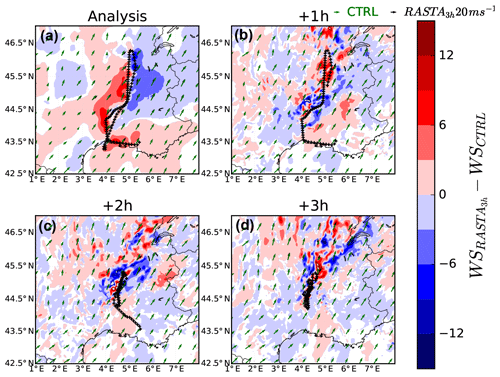
Figure 6Wind increments between the RASTA and the CTRLIOP7 experiments for the analysis at 06:00 UTC and for the 1, 2 and 3 h forecasts at 07:00, 08:00 and 09:00 UTC on 26 September 2012 (IOP7a) at approximately 4 km of altitude (model level 30). The black data points represent the location of RASTA data within a 1 h time window centred on the forecast term range.
5.3 Verification against RASTA observations
Figure 6 (panels b to d) represents the wind speed differences of the RASTA 1, 2 and 3 h forecasts and the CTRLIOP7 ones. At each forecast term, the black data points indicate the different RASTA locations which are available during a 1 h time window centred on the forecast time (forecast term ±30 min). Figure 6 shows that, even though the increments are less organized as the forecast term increases, there is a noticeable impact of the assimilation of RASTA wind data on the subsequent forecasts at 07:00, 08:00 and 09:00 UTC. Besides, some of the most substantial differences are co-located with RASTA locations (black data points in Fig. 6) and downstream of these locations.
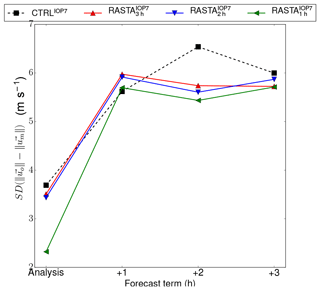
Figure 7Standard deviation of the wind differences between RASTA observations and each experiment (see legend) as a function of the forecast term from the 06:00 UTC analysis on 26 September 2012 (IOP7a).
Figure 7 represents the standard deviation of the wind speed differences between RASTA observations and each experiment as a function of the forecast term. The standard deviations were calculated using all the data available within a 1 h time window centred on the forecast time (black data points in Fig. 6). For instance, at 07:00 UTC, the 1 h forecast of each experiment is compared with the observations available between 06:30 and 07:30 UTC. Similarly, at 08:00 UTC (09:00 UTC), the 2 h (3 h) forecast of each experiment are compared with the observations available between 07:30 and 08:30 UTC (08:30 and 09:30 UTC).
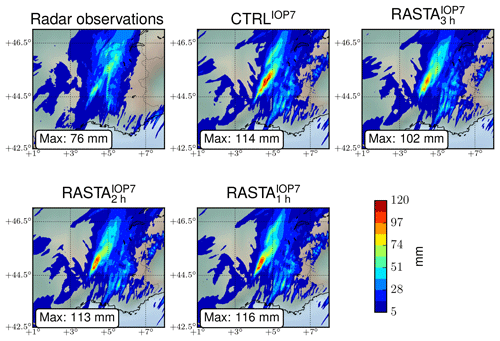
Figure 8Maps of 11 h accumulated rainfall between 07:00 and 18:00 UTC on 26 September 2012 (IOP7a) for radar observations, the CTRLIOP7, RASTA, RASTA, RASTA experiments.
As expected, the major differences between the different experiments appear on the analyses. The smallest standard deviation value is reached with the RASTA experiment. Indeed, compared to the CTRL, the standard deviation of the wind speed has been reduced by a value close to 1.5 m s−1. At the analysis time, the standard deviation values were calculated using the observations that were assimilated at the 06:00 UTC analysis in the RASTA experiment (06:00 UTC + 30 min). As explained in the previous section, because of the non-rectilinear trajectory of the aircraft, the different RASTA analyses are slightly different. These differences explain why, when the comparison is performed against the observations which are available until 06:30 UTC, the standard deviation increases with the increasing length of the assimilation window. Nevertheless, in all three RASTA experiments, the standard deviation is always reduced in the analyses when RASTA observations are assimilated.
At 2 and 3 h term ranges, compared to the CTRLIOP7, the assimilation of RASTA wind data leads to a systematic improvement in the standard deviation in the three RASTA experiments. By contrast, at 1 h term range, results indicate a negative impact of the assimilation of RASTA wind data since the three RASTA experiments exhibit larger standard deviation values. However, this negative impact should be taken cautiously since there are numerical noises and imbalances in the first 2 h of integration due to spin-up in the AROME-WMED system (Seity et al., 2011).
Finally, Fig. 7 demonstrates the benefit brought by the assimilation of RASTA wind data. Except at a 1 h term range, probably because of spin-up, there is an improvement in the predicted wind speed at all forecast term ranges. Nonetheless, it is hard to rank the different RASTA experiments. Similar results were also obtained in another case, which occurred over sea on 11 October 2012 (not shown).
5.4 Impact on rainfall forecasts
The impact of the assimilation of RASTA wind data at 06:00 UTC is now illustrated on rainfall accumulation forecasts. To avoid the spin-up problem, the first hour of rainfall accumulation has been removed from the calculations. Figure 8 shows the 11 h accumulated rainfall between 07:00 and 18:00 UTC on 26 September 2012 (IOP7a) for the radar observations, CTRLIOP7, the RASTA, the RASTA and the RASTA experiments.
In all experiments, the predicted rainfall accumulation patterns match the observations well. However, the maximum rainfall accumulation is much larger in the CTRLIOP7 experiment (114 mm) than the observed one (76 mm). The RASTA experiment is in much better agreement with the observations since the maximum rainfall accumulation has been reduced to a value close to 102 mm. The assimilation of RASTA wind data with smaller assimilation windows (2 and 3 h) does not significantly impact the rainfall forecasts. Indeed, the maximum rainfall accumulation is of same order of magnitude in the RASTA (113 mm) and RASTA experiments (116 mm), compared to the CTRLIOP7 one.
The results in Fig. 8 indicate a sensitivity to the choice of the assimilation windows. In particular, the best experiment is the one for which RASTA observations are assimilated with the larger assimilation window (RASTA). Therefore, in this specific case study, the rainfall accumulation forecasts are closer to the observations when more data are assimilated, even though some of them might no longer be valid at the assimilation time. This result can also be explained by the fact that horizontal wind components in moderately convective clouds are more representative of synoptic scales and less likely to change as quickly as other meteorological variables, such as humidity. However, this result may be only representative of this specific case study and should be taken cautiously.
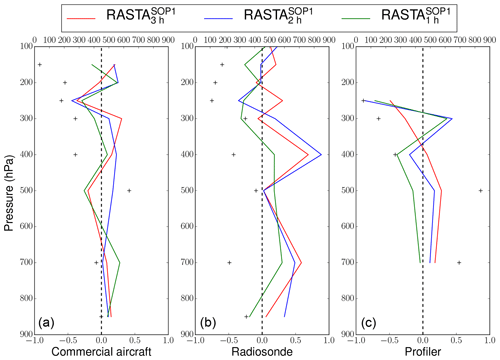
Figure 9Differences of standard deviation error for 3 h wind forecasts between the CTRLCOP1 experiment and the RASTA (red), the RASTA (blue) and the RASTA (green) experiments. Negative differences indicate a positive impact of the assimilation of RASTA wind data. The standard deviation errors are computed for commercial aircraft (a), radiosonde (b) and profiler (c) observations. All the scores are computed over the 35 runs in which RASTA wind data were assimilated with the largest assimilation window over the RASTA-limited area. In each panel, the number of observations used for the calculation is represented by the black data plus signs in the top x axis.
The impact of RASTA wind data assimilation is now assessed over the 45-day cycled period during the HyMeX SOP1. Verification is first carried out against other assimilated observations types in Sect. 6.1. Verification is then performed against rain gauges observations in Sect. 6.2.
6.1 Comparison against conventional observations
Averaged over the 45-day experiment, the assimilation of RASTA wind data does not substantially impact the specific humidity and the temperature on both the analyses and the forecasts. Therefore, because the most significant differences only appear on the zonal (u) and meridional (v) wind components, results are only shown for the wind. Calculations are not shown for the analyses but only for the 3 h forecasts. Indeed, since the observations used for the comparisons are all assimilated, the fit to observations is better in CTRLSOP1 than in the RASTA experimental runs.
Because RASTA wind data are limited in space around the Mediterranean area (see black lines in Fig. 1) and depend on the presence of cloud or precipitation along the aircraft flight path, their assimilation impact is also limited in space. Hence, at each assimilation time, a RASTA-limited validation area is employed. It contains the aircraft flight path ±0.5∘ both in latitude and longitude. Only the conventional observations (commercial aircraft data, radiosonde and profiler) which are available in the RASTA-limited area are used for the calculations. Since the assimilation impact of RASTA wind data is also limited in time, calculations are only performed over the 35 runs in which RASTA wind data were assimilated with the largest assimilation window. Figure 9 shows the differences in standard deviation error for 3 h wind forecasts between the CTRLSOP1 experiment and the RASTA (red), the RASTA (blue) and the RASTA (green) experiments. Negative (positive) differences indicate a positive (negative) impact of the assimilation of RASTA wind data. The total number of observations used for the calculation is represented by the black plus signs in the top x axis.
In general, Fig. 9 indicates that the impact of the assimilation of RASTA wind data on the 3 h forecasts is hard to assess. Indeed, compared to commercial aircraft wind observations (left panel), the vertical profiles of the standard deviation demonstrate a neutral impact. However, compared to radiosonde (middle panel) and profiler (right panel) observations, there is a slight positive to negative impact depending on the assimilation window, which is probably a deluding effect due to the small number of conventional observations available in the area of interest. The comparison with ground-based radar data gives similar results (not shown).
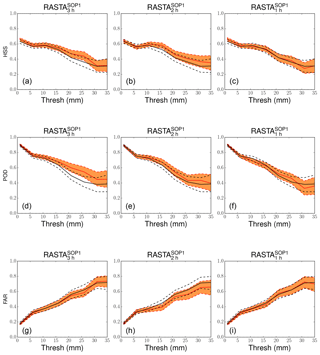
Figure 10HSS (a, b, c), POD (d, e, f) and FAR (g, h, i) of the 8 h cumulated precipitation forecasts vs. rain gauge measurements for the three RASTA experiments (in red) and for the CTRLSOP1 experiment (in black). Calculations were performed over the 35 runs in which RASTA wind data were assimilated with the largest assimilation window. The error bars (dashed lines) represent the 90 % bias-corrected and accelerated (BCa) bootstrap confidence intervals (see Efron and Tibshirani, 1993).
6.2 Impact on rainfall forecasts
Forecast scores against rainfall measurements are now calculated over the 35 runs (out of 360) in which RASTA data were assimilated with the largest assimilation window. The verification is conducted using the rain gauge network available from the HyMeX database (https://doi.org/10.6096/MISTRALS-HyMeX.904), the locations of which are indicated by the blue markers in Fig. 1. For the comparisons, model outputs are interpolated to the rain gauge station locations using a linear interpolation. Model outputs and rain gauge measurements are then averaged in boxes of 0.25∘ × 0.25∘ within each RASTA-limited validation area.
Categorical scores have been calculated: Heidke skill score (HSS), probability of detection (POD) and false alarm ratio (FAR). To avoid the spin-up problem, the first hour of rainfall accumulation has been removed from the calculations. The HSS, POD and FAR of the 8 h accumulated rainfall forecasts for the three RASTA experiments are displayed in red in Fig. 10 as a function of the rainfall accumulation threshold (mm). The scores of the CTRLSOP1 experiment are also shown in black. The bootstrap confidence intervals are displayed for each threshold by the dashed lines. The impact of the assimilation of RASTA wind data is positive if the red lines are above (below) the black ones for the HSS and POD (FAR).
Figure 10 shows that the general pattern is similar for the three RASTA experiments, which indicates that the choice of the assimilation window does not significantly impact the subsequent forecasts. Even though the bootstrap confidence intervals increase with the threshold, differences with the CTRLSOP1 experiment are more pronounced at larger thresholds in any of the three RASTA experiments. The most significant differences appear for the RASTA and RASTA experiments, which is consistent with the results found for the IOP7a case study in Sect. 5.4. In addition, except for the RASTA experiment, the assimilation of RASTA wind data tends to slightly improve the scores above approximately 10 mm.
It should be noted that this slight positive improvement of the heavier rainfall can also be seen for the 5 and 11 h forecasts (not shown). Finally, the benefit brought by the assimilation of RASTA wind data decreases with the forecast term range (≥11 h forecasts), which is partly explained by the lateral boundary conditions. Indeed, after a few hours, the increments are replaced by inputs from the same coupling model.
This article reports on the first study in which vertical profiles of wind measured by vertically pointing airborne Doppler W-band radar are assimilated in a kilometre-scale NWP model. The study was performed in a quasi-operational framework with a special version of the Météo-France operational kilometre-scale model AROME with its 3DVAR assimilation system. The data were provided by the airborne Doppler W-band radar RASTA during a 45-day period over a region of the Mediterranean area that is very prone to heavy rainfall. RASTA is a multibeam antenna system that can retrieve the three components of the wind fields, which allows the direct assimilation of the retrieved horizontal wind components.
A sensitivity study for the choice of the assimilation window was performed. Indeed, RASTA wind data are not measured simultaneously at the assimilation time but over the flight leg. Consequently, at the assimilation time T, the 3DVAR assimilation system of AROME-WMED ingests all data available along the aircraft path during the assimilation window Δt, as if they were valid at time T. Therefore, the ability of RASTA wind data to improve short-term forecasts of heavy precipitation events was tested with three different assimilation windows Δt: 3 h (RASTA3 h), 2 h (RASTA2 h) and 1 h (RASTA1 h).
The positive impact of the assimilation of RASTA wind data was first evidenced in a case of heavy rainfall, which occurred during the Intensive Observation Period 7a (IOP7a) on 26 September 2012. This case study was selected because the data that are assimilated at the 06:00 UTC analysis are located upwind from where the heavy rainfall took place. Such a configuration is required to study a potential impact of the assimilation of RASTA wind data on the subsequent forecasts. Except at very short-term range (1 h) because of spin-up, the assimilation of RASTA wind data led to a systematic improvement of the predicted wind at all short-term ranges (2 and 3 h) in any of the three RASTA experiments. It could be interesting to repeat the same study with the more recent operational AROME system because the model spin-up has been reduced to less than 1 h (Brousseau et al., 2016). Besides, the 11 h accumulated rainfall forecasts are also in much better agreement with the observations. Therefore, this case study demonstrates a positive impact of the assimilation of RASTA wind data to better predict this rainfall event. Similar results were also obtained for another case which occurred over sea on 11 November 2012 (not shown in this article).
A cycling data assimilation experiment has also been conducted over a 45-day period from 24 October to 5 November 2012, for the CTRL experiment and for the three RASTA data assimilation experiments. The comparisons against other assimilated observations and rain gauges measurements indicate an overall neutral impact, which is probably due to the small percentage of RASTA wind data which were assimilated among the total number of observations. Nevertheless, results of this statistical study are encouraging since no major detrimental effect was found and a slight positive improvement in the 5, 8 and 11 h precipitation forecasts of heavier rainfall was evidenced.
The sensitivity study to the assimilation window on the IOP7 case study and on the statistical study suggested that the quality of the rainfall accumulation forecasts increases with the length of the assimilation window. Hence, it seems preferable to assimilate more data to have larger coverage by increasing the length of the assimilation window. However, results should be taken cautiously since the sensitivity study was only conducted over 35 analysis cases. More cases should be explored over other field campaigns to corroborate the results of this sensitivity study. Besides, the issue of the length of the assimilation window becomes less important if the assimilation frequency increases and/or a shorter assimilation cycle is used, such as in the new AROME system (Brousseau et al., 2016).
It is probable that low-quality data did pass the quality control and were thus assimilated. Zhang et al. (2012) show the importance of specifying a strong data quality control. Hence, a more efficient data quality control should improve our results. Finally, another perspective is to assimilate the W-band radar reflectivity jointly with RASTA wind data to find out whether modifying the thermodynamic and the dynamic state of the model in a consistent way in the initial state would lead to more significant improvements. Indeed, Janisková (2015) demonstrated a slight positive impact of the assimilation of W-band space-borne radar using a 1D+4DVAR technique. The 1D+3DVAR assimilation method that is operationally used to assimilate the radar reflectivity in AROME (Caumont et al., 2010; Wattrelot et al., 2014) will be employed to assimilate the W-band reflectivity.
RASTA data and rain gauge data are all available from the HyMeX database (https://doi.org/10.17616/R3M34X; re3data.org, 2018). The simulation data that support the findings of this study are available from the corresponding author on reasonable request.
This work was carried out by MB as part of her PhD thesis under the supervision of VD and OC. JD processed and provided RASTA data. NF provided the files and set up that are necessary to run the different assimilation experiments with AROME-WMED. All co-authors collaborated, interpreted the results, wrote the paper and replied to the comments from the reviewers.
The authors declare that they have no conflict of interest.
This article is part of the special issue “Hydrological cycle in the Mediterranean (ACP/AMT/GMD/HESS/NHESS/OS inter-journal SI)”. It is not associated with a conference.
This work is a contribution to the HyMeX programme supported by MISTRALS, ANR IODA-MED grant ANR-11-BS56-0005 and ANR MUSIC grant ANR-14-CE01-0014. This work was supported by the French national programme LEFE/INSU. The authors acknowledge the DGA (Direction Générale de l'Armement), a part of the French Ministry of Defense, for its contribution to Mary Borderies's PhD. The authors thank SAFIRE for operating the French Falcon 20 research aircraft during HyMeX-SOP1. The authors are grateful to Pierre Brousseau for his technical help. Two anonymous reviewers are also gratefully acknowledged for their comments, which helped to significantly improve the quality of the article.
This paper was edited by Eric Martin and reviewed by two anonymous referees.
Baker, W. E., Atlas, R., Cardinali, C., Clement, A., Emmitt, G. D., Gentry, B. M., Hardesty, R. M., Källén, E., Kavaya, M. J., Langland, R., Ma, Z., Masutani, M., McCarty, W., Pierce, R. B., Pu, Z., Riishojgaard, L. P., Ryan, J., Tucker, S., Weissmann, M., and Yoe, J. G.: Lidar-Measured Wind Profiles: The Missing Link in the Global Observing System, B. Am. Meteorol. Soc., 95, 543–564, https://doi.org/10.1175/BAMS-D-12-00164.1, 2014. a
Benjamin, S. G., Schwartz, B. E., Szoke, E. J., and Koch, S. E.: The Value of Wind Profiler Data in U.S. Weather Forecasting, B. Am. Meteorol. Soc., 85, 1871–1886, https://doi.org/10.1175/BAMS-85-12-1871, 2004. a
Borderies, M., Caumont, O., Augros, C., Bresson, É., Delanoë, J., Ducrocq, V., Fourrié, N., Le Bastard, T., and Nuret, M.: Simulation of W-band radar reflectivity for model validation and data assimilation, Q. J. Roy. Meteor. Soc., 144, 391–403, https://doi.org/10.1002/qj.3210, 2018. a
Bosart, B. L., Lee, W.-C., and Wakimoto, R. M.: Procedures to improve the accuracy of airborne Doppler radar data, J. Atmos. Ocean. Tech., 19, 322–339, https://doi.org/10.1175/1520-0426-19.3.322, 2002. a
Bouniol, D., Protat, A., Plana-Fattori, A., Giraud, M., Vinson, J.-P., and Grand, N.: Comparison of Airborne and Spaceborne 95-GHz Radar Reflectivities and Evaluation of Multiple Scattering Effects in Spaceborne Measurements, J. Atmos. Ocean. Tech., 25, 1983–1995, https://doi.org/10.1175/2008jtecha1011.1, 2008. a
Bousquet, O., Delanoë, J., and Bielli, S.: Evaluation of 3D wind observations inferred from the analysis of airborne and ground-based radars during HyMeX SOP-1, Q. J. Roy. Meteor. Soc., 142, 86–94, https://doi.org/10.1002/qj.2710, 2016. a, b, c, d
Brousseau, P., Berre, L., Bouttier, F., and Desroziers, G.: Background-error covariances for a convective-scale data-assimilation system: AROME-France 3D-Var, Q. J. Roy. Meteor. Soc., 137, 409–422, https://doi.org/10.1002/qj.750, 2011. a
Brousseau, P., Seity, Y., Ricard, D., and Léger, J.: Improvement of the forecast of convective activity from the AROME-France system, Q. J. Roy. Meteor. Soc., 142, 2231–2243, https://doi.org/10.1002/qj.2822, 2016. a, b
Caumont, O., Ducrocq, V., Wattrelot, É., Jaubert, G., and Pradier-Vabre, S.: 1D+3DVar assimilation of radar reflectivity data: a proof of concept, Tellus A, 62, 173–187, https://doi.org/10.1111/j.1600-0870.2009.00430.x, 2010. a
Deb, S., Kishtawal, C., Kumar, P., Kumar, A. K., Pal, P., Kaushik, N., and Sangar, G.: Atmospheric Motion Vectors from INSAT-3D: Initial quality assessment and its impact on track forecast of cyclonic storm NANAUK, Atmos. Res., 169, 1–16, https://doi.org/10.1016/j.atmosres.2015.09.010, 2016. a
Delanoë, J., Protat, A., Jourdan, O., Pelon, J., Papazzoni, M., Dupuy, R., Gayet, J.-F., and Jouan, C.: Comparison of Airborne In Situ, Airborne Radar–Lidar, and Spaceborne Radar–Lidar Retrievals of Polar Ice Cloud Properties Sampled during the POLARCAT Campaign, J. Atmos. Ocean. Tech., 30, 57–73, https://doi.org/10.1175/JTECH-D-11-00200.1, 2013. a
Drobinski, P., Ducrocq, V., Alpert, P., Anagnostou, E., Béranger, K., Borga, M., Braud, I., Chanzy, A., Davolio, S., Delrieu, G., Estournel, C., Boubrahmi, N. F., Font, J., Grubišic, V., Gualdi, S., Homar, V., Ivancan-Picek, B., Kottmeier, C., Kotroni, V., Lagouvardos, K., Lionello, P., Llasat, M. C., Ludwig, W., Lutoff, C., Mariotti, A., Richard, E., Romero, R., Rotunno, R., Roussot, O., Ruin, I., Somot, S., Taupier-Letage, I., Tintore, J., Uijlenhoet, R., and Wernli, H.: HyMeX: A 10-year multidisciplinary program on the Mediterranean water cycle, B. Am. Meteorol. Soc., 95, 1063–1082, https://doi.org/10.1175/BAMS-D-12-00242.1, 2014. a
Ducrocq, V., Braud, I., Davolio, S., Ferretti, R., Flamant, C., Jansa, A., Kalthoff, N., Richard, E., Taupier-Letage, I., Ayral, P., Belamari, S., Berne, A., Borga, M., Boudevillain, B., Bock, O., Boichard, J., Bouin, M., Bousquet, O., Bouvier, C., Chiggiato, J., Cimini, D., Corsmeier, U., Coppola, L., Cocquerez, P., Defer, E., Delanoë, J., Di Girolamo, P., Doerenbecher, A., Drobinski, P., Dufournet, Y., Fourrié, N., Gourley, J. J., Labatut, L., Lambert, D., Le Coz, J., Marzano, F. S., Molinié, G., Montani, A., Nord, G., Nuret, M., Ramage, K., Rison, W., Roussot, O., Said, F., Schwarzenboeck, A., Testor, P., Van Baelen, J., Vincendon, B., Aran, M., and Tamayo, J.: HyMeX-SOP1: The field campaign dedicated to heavy precipitation and flash flooding in the northwestern Mediterranean, B. Am. Meteorol. Soc., 95, 1083–1100, https://doi.org/10.1175/BAMS-D-12-00244.1, 2014. a, b, c, d
Duffourg, F., Nuissier, O., Ducrocq, V., Flamant, C., Chazette, P., Delanoë, J., Doerenbecher, A., Fourrié, N., Di Girolamo, P., Lac, C., Legain, D., Martinet, M., Saïd, F., and Bock, O.: Offshore deep convection initiation and maintenance during the HyMeX IOP 16a heavy precipitation event, Q. J. Roy. Meteor. Soc., 142, 259–274, https://doi.org/10.1002/qj.2725, 2016. a
Efron, B. and Tibshirani, R. J.: An Introduction to the Bootstrap, Chapman & Hall/CRC Press, Boca Raton, FL, ISBN 9780412042317, 456 pp., 1994. a
Fischer, C., Montmerle, T., Berre, L., Auger, L., and Ştefănescu, S. E.: An overview of the variational assimilation in the ALADIN/France numerical weather-prediction system, Q. J. Roy. Meteor. Soc., 131, 3477–3492, https://doi.org/10.1256/qj.05.115, 2005. a
Fourrié, N., Bresson, é., Nuret, M., Jany, C., Brousseau, P., Doerenbecher, A., Kreitz, M., Nuissier, O., Sevault, E., Bénichou, H., Amodei, M., and Pouponneau, F.: AROME-WMED, a real-time mesoscale model designed for the HyMeX special observation periods, Geosci. Model Dev., 8, 1919–1941, https://doi.org/10.5194/gmd-8-1919-2015, 2015. a, b
Hally, A., Richard, E., and Ducrocq, V.: An ensemble study of HyMeX IOP6 and IOP7a: sensitivity to physical and initial and boundary condition uncertainties, Nat. Hazards Earth Syst. Sci., 14, 1071–1084, https://doi.org/10.5194/nhess-14-1071-2014, 2014. a, b
Horányi, A., Cardinali, C., Rennie, M., and Isaksen, L.: The assimilation of horizontal line-of-sight wind information into the ECMWF data assimilation and forecasting system. Part I: The assessment of wind impact, Q. J. Roy. Meteor. Soc., 141, 1223–1232, https://doi.org/10.1002/qj.2430, 2015. a
Illingworth, A., Battaglia, A., Bradford, J., Forsythe, M., Joe, P., Kollias, P., Lean, K., Lori, M., Mahfouf, J.-F., Mello, S., Midthassel, R., Munro, Y., Nicol, J., Potthast, R., Rennie, M., Stein, T., Tanelli, S., Tridon, F., Walden, C., and Wolde, M.: WIVERN: A new satellite concept to provide global in-cloud winds, precipitation and cloud properties, B. Am. Meteorol. Soc., 99, 1669–1687, https://doi.org/10.1175/BAMS-D-16-0047.1, 2018. a
Illingworth, A. J., Barker, H. W., Beljaars, A., Ceccaldi, M., Chepfer, H., Clerbaux, N., Cole, J., Delanoë, J., Domenech, C., Donovan, D. P., Fukuda, S., Hirakata, M., Hogan, R. J., Huenerbein, A., Kollias, P., Kubota, T., Nakajima, T., Nakajima, T. Y., Nishizawa, T., Ohno, Y., Okamoto, H., Oki, R., Sato, K., Satoh, M., Shephard, M. W., Velázquez-Blázquez, A., Wandinger, U., Wehr, T., and van Zadelhoff, G.: The EarthCARE Satellite: The Next Step Forward in Global Measurements of Clouds, Aerosols, Precipitation, and Radiation, B. Am. Meteorol. Soc., 96, 1311–1332, https://doi.org/10.1175/bams-d-12-00227.1, 2015a. a
Illingworth, A. J., Cimini, D., Gaffard, C., Haeffelin, M., Lehmann, V., Löhnert, U., O'Connor, E. J., and Ruffieux, D.: Exploiting existing ground-based remote sensing networks to improve high-resolution weather forecasts, B. Am. Meteorol. Soc., 96, 2107–2125, https://doi.org/10.1175/bams-d-13-00283.1, 2015b. a
Janisková, M.: Assimilation of cloud information from space-borne radar and lidar: experimental study using a 1D+4D-Var technique, Q. J. Roy. Meteor. Soc., 141, 2708–2725, https://doi.org/10.1002/qj.2558, 2015. a
Kawabata, T., Seko, H., Saito, K., Kuroda, T., Tamiya, K., Tsuyuki, T., Honda, Y., and Wakazuki, Y.: An Assimilation and Forecasting Experiment of the Nerima Heavy Rainfa11 with a Cloud-Resolving Nonhydrostatic 4-Dimensional Variational Data Assimilation System, J. Meteorol. Soc. Jpn., 85, 255–276, https://doi.org/10.2151/jmsj.85.255, 2007. a
Kawabata, T., Iwai, H., Seko, H., Shoji, Y., Saito, K., Ishii, S., and Mizutani, K.: Cloud-Resolving 4D-Var Assimilation of Doppler Wind Lidar Data on a Meso-Gamma-Scale Convective System, Mon. Weather Rev., 142, 4484–4498, https://doi.org/10.1175/MWR-D-13-00362.1, 2014. a
Kumar, P., Deb, S. K., Kishtawal, C., and Pal, P.: Impact of assimilation of INSAT-3D retrieved atmospheric motion vectors on short-range forecast of summer monsoon 2014 over the south Asian region, Theor. Appl. Climatol., 128, 575–586, https://doi.org/10.1007/s00704-015-1722-5, 2017. a
Li, Z., Pu, Z., Sun, J., and Lee, W.-C.: Impacts of 4DVAR assimilation of airborne Doppler radar observations on numerical simulations of the genesis of Typhoon Nuri (2008), J. Appl. Meteorol. Clim., 53, 2325–2343, https://doi.org/10.1175/JAMC-D-14-0046.1, 2014. a
Martinet, M., Nuissier, O., Duffourg, F., Ducrocq, V., and Ricard, D.: Fine-scale numerical analysis of the sensitivity of the HyMeX IOP16a heavy precipitating event to the turbulent mixing-length parametrization, Q. J. Roy. Meteor. Soc., 143, 3122–3135, https://doi.org/10.1002/qj.3167, 2017. a
Montmerle, T. and Faccani, C.: Mesoscale assimilation of radial velocities from Doppler radars in a preoperational framework, Mon. Weather Rev., 137, 1939–1953, https://doi.org/10.1175/2008MWR2725.1, 2009. a
Pinty, J. and Jabouille, P.: A mixed-phase cloud parameterization for use in mesoscale non-hydrostatic model: simulations of a squall line and of orographic precipitations, in: Conf. on Cloud Physics, 217–220, Amer. Meteor. Soc Everett, WA, 1998. a
Protat, A., Bouniol, D., Delanoë, J., O'Connor, E., May, P., Plana-Fattori, A., Hasson, A., Görsdorf, U., and Heymsfield, A.: Assessment of CloudSat reflectivity measurements and ice cloud properties using ground-based and airborne cloud radar observations, J. Atmos. Ocean. Tech., 26, 1717–1741, https://doi.org/10.1175/2009JTECHA1246.1, 2009. a
Pu, Z., Li, X., and Sun, J.: Impact of airborne Doppler radar data assimilation on the numerical simulation of intensity changes of Hurricane Dennis near a landfall, J. Atmos. Sci., 66, 3351–3365, https://doi.org/10.1175/2009JAS3121.1, 2009. a
re3data.org: HyMeX, re3data.org – Registry of Research Data Repositories, https://doi.org/10.17616/R3M34X, 2018. a
Rennie, S. J., Dance, S. L., Illingworth, A. J., Ballard, S. P., and Simonin, D.: 3D-Var Assimilation of Insect-Derived Doppler Radar Radial Winds in Convective Cases Using a High-Resolution Model, Mon. Weather Rev., 139, 1148–1163, https://doi.org/10.1175/2010MWR3482.1, 2011. a
Salonen, K., Cotton, J., Bormann, N., and Forsythe, M.: Characterizing AMV height-assignment error by comparing best-fit pressure statistics from the Met Office and ECMWF data assimilation systems, J. Appl. Meteorol. Clim., 54, 225–242, https://doi.org/10.1175/JAMC-D-14-0025.1, 2015. a
Seity, Y., Brousseau, P., Malardel, S., Hello, G., Bénard, P., Bouttier, F., Lac, C., and Masson, V.: The AROME-France Convective-Scale Operational Model, Mon. Weather Rev., 139, 976–991, https://doi.org/10.1175/2010mwr3425.1, 2011. a, b
Simonin, D., Ballard, S., and Li, Z.: Doppler radar radial wind assimilation using an hourly cycling 3D-Var with a 1.5 km resolution version of the Met Office Unified Model for nowcasting, Q. J. Roy. Meteor. Soc., 140, 2298–2314, https://doi.org/10.1002/qj.2298, 2014. a
Wattrelot, E., Caumont, O., and Mahfouf, J.-F.: Operational Implementation of the 1D+3D-Var Assimilation Method of Radar Reflectivity Data in the AROME Model, Mon. Weather Rev., 142, 1852–1873, https://doi.org/10.1175/mwr-d-13-00230.1, 2014. a
Weissmann, M., Langland, R. H., Cardinali, C., Pauley, P. M., and Rahm, S.: Influence of airborne Doppler wind lidar profiles near Typhoon Sinlaku on ECMWF and NOGAPS forecasts, Q. J. Roy. Meteor. Soc., 138, 118–130, https://doi.org/10.1002/qj.896, 2012. a
Weng, Y. and Zhang, F.: Assimilating airborne Doppler radar observations with an ensemble Kalman filter for convection-permitting hurricane initialization and prediction: Katrina (2005), Mon. Weather Rev., 140, 841–859, https://doi.org/10.1175/2011MWR3602.1, 2012. a
Zhang, L., Pu, Z., Lee, W.-C., and Zhao, Q.: The influence of airborne Doppler radar data quality on numerical simulations of a tropical cyclone, Weather Forecast., 27, 231–239, https://doi.org/10.1175/waf-d-11-00028.1, 2012. a
- Abstract
- Introduction
- Radar data and period of study
- Model and data assimilation system
- Data assimilation experiments
- Results on the case study
- Statistical study
- Discussions and conclusions
- Data availability
- Author contributions
- Competing interests
- Special issue statement
- Acknowledgements
- Review statement
- References
- Abstract
- Introduction
- Radar data and period of study
- Model and data assimilation system
- Data assimilation experiments
- Results on the case study
- Statistical study
- Discussions and conclusions
- Data availability
- Author contributions
- Competing interests
- Special issue statement
- Acknowledgements
- Review statement
- References