the Creative Commons Attribution 4.0 License.
the Creative Commons Attribution 4.0 License.
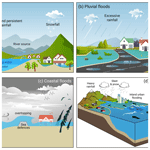
Review article: A comprehensive review of compound flooding literature with a focus on coastal and estuarine regions
Ivan D. Haigh
Niall Quinn
Jeff Neal
Thomas Wahl
Melissa Wood
Dirk Eilander
Marleen de Ruiter
Philip Ward
Paula Camus
Compound flooding, where the combination or successive occurrence of two or more flood drivers leads to a greater impact, can exacerbate the adverse consequences of flooding, particularly in coastal–estuarine regions. This paper reviews the practices and trends in coastal–estuarine compound flood research and synthesizes regional to global findings. A systematic review is employed to construct a literature database of 279 studies relevant to compound flooding in a coastal–estuarine context. This review explores the types of compound flood events and their mechanistic processes, and it synthesizes terminology throughout the literature. Considered in the review are six flood drivers (fluvial, pluvial, coastal, groundwater, damming/dam failure, and tsunami) and five precursor events and environmental conditions (soil moisture, snow, temp/heat, fire, and drought). Furthermore, this review summarizes research methodology and study application trends, as well as considers the influences of climate change and urban environments. Finally, this review highlights knowledge gaps in compound flood research and discusses the implications on future practices. Our five recommendations for compound flood research are (1) adopt consistent terminology and approaches, (2) expand the geographic coverage of research, (3) pursue more inter-comparison projects, (4) develop modelling frameworks that better couple dynamic Earth systems, and (5) design urban and coastal infrastructure with compounding in mind.
- Article
(4806 KB) - Full-text XML
- BibTeX
- EndNote
Flooding is the costliest and most common hazard worldwide (Bevere and Remondi, 2022; Mishra et al., 2022; Rentschler et al., 2022) and can lead to a wide range of environmental, economic, and social repercussions. Over 1.8 billion people, almost a quarter (23 %) of the world's population, are exposed to 1-in-100-year flooding (Rentschler et al., 2022). The vast majority (89 %) of these people live in low- and middle-income countries, and socially vulnerable communities are disproportionately at risk (Rentschler et al., 2022). Since 1980, global floods have caused over 250 000 fatalities and USD 1 trillion in losses (Munich Re, 2017; EM-DAT, 2022). In 2021 alone there were more than 50 severe flood disasters recorded worldwide, causing economic losses totalling USD 82 billion (2022 USD) (Bevere and Remondi, 2022).
A large proportion of deaths and the economic losses associated with flooding have historically occurred in densely populated coastal–estuarine regions. Today, near-coastal zones and low-elevation coastal zones, subject to flooding from a range of drivers, are respectively home to 2.15 billion and ∼ 900 million people globally (Reimann et al., 2023). In the past decade, floods associated with strong onshore wind and pressure fields (e.g. 2013/2014 UK winter floods, 2017 Atlantic hurricane season, 2019 Atlantic Hurricane Dorian, 2019 East Africa Tropical Cyclone Idai, 2019 Pacific typhoon season, and 2022 eastern Australia floods) have showcased the ever-present threat of extreme flood impacts in coastal settings. Even in regions where coastal defence standards are among the highest in the world (e.g. Europe, Japan, the Netherlands), potential defence failure during events that exceed the standard of protection (e.g. major overtopping or a breach) still poses considerable risk to populations and development in coastal floodplains. Moreover, flooding is a rapidly growing threat to most coastal regions and their communities due to (i) sea-level rise, changes in storminess, and increasingly variable rainfall patterns driven by climate change (Church et al., 2001; Wood et al., 2023); (ii) population growth, urbanization, and continued development in floodplains (Hallegatte et al., 2013); and (iii) the continued decline in the extent of shorelines and habitats which act as natural buffers to flooding (Woodruff et al., 2013; Oppenheimer et al., 2019). Average global flood losses in large coastal cities are estimated to increase approximately 10-fold by 2050 due to socio-economic change alone, reaching up to USD 1 trillion or more per year when considering sea-level rise and land subsidence (Hallegatte et al., 2013). There is clear importance in advancing our understanding of flooding in coastal–estuarine regions.
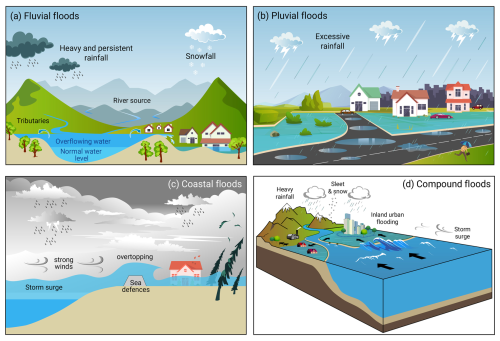
Figure 1Schematic diagram of flood drivers showing (a) fluvial (river discharge), (b) pluvial (rainfall–runoff), and (c) coastal (surge, tide, waves, and total sea level) components, as well as their (d) compound flood interactions.
This review focuses on compound flooding that takes place in coastal (ocean/lake) and estuarine regions, which primarily arises from three main drivers: (1a) river discharge (fluvial); (1b) precipitation surface runoff (pluvial); and (1c) coastal processes including storm surge, astronomical tides, wave action, and relative sea-level rise (SLR) (coastal) as shown in Fig. 1. Traditionally, most existing flood risk assessments consider these main drivers of flooding separately, and many oversimplify or ignore key interactions altogether. However, in many coastal–estuarine regions, floods are often caused by more than one driver as the processes are naturally correlated. For example, intense tropical/extratropical cyclones (TCs/ETCs) can generate heavy precipitation that enhances river discharges, while at the same time strong winds and low pressures cause large storm surges and waves. When fluvial, pluvial, and/or coastal drivers occur at the same time, or within a few hours or days, the adverse effects of flooding can be measurably exacerbated (Gori et al., 2020a; Khalil et al., 2022). The synergy of multiple hazard drivers can result in disproportionately extreme events, even if individual flood drivers are not extreme themselves. This is often referred to as “compound events” (Hewitt and Burton, 1971; Adhikari et al., 2010; Seneviratne et al., 2012; Leonard et al., 2014; Zscheischler et al., 2020). It is only in the last decade that we are beginning to recognize the necessity of compound-event-based approaches to flood risk assessment, as traditional univariate methods of analysis fail to capture the non-linear impacts of multiple flood drivers (Kappes et al., 2010; Leonard et al., 2014; Eshrati et al., 2015; Klerk et al., 2015; Ridder et al., 2018; Zscheischler et al., 2018; Hao and Singh, 2020; Ridder et al., 2020; Manoj et al., 2022).
In recent decades our knowledge of individual flood drivers has improved tremendously, as a result of better in situ and remote sensing datasets, as well as advances in statistical and numerical modelling techniques. However, our understanding of compound flood events is still limited – from the synergetic processes to the spatiotemporal trends and scales of interacting drivers. Compound-event-based research is relatively new (Wu et al., 2020; Bevacqua et al., 2021), having only gained notable attention in 2012 when it was formally defined in the Intergovernmental Panel on Climate Change's (IPCC) Special Report on Climate Extremes (SREX) (Seneviratne et al., 2012), and is a key guiding principle of the 2015 UN Sendai Framework for Disaster Risk Reduction (UNDRR, 2015). Additionally, there has been growing public awareness of extreme compound flooding following a decade of increasingly frequent extreme weather events, where catastrophic disasters arose from multiple interacting flood drivers. For example, in 2017 Hurricane Harvey resulted in record-breaking rainfall, river discharge, and runoff, which when combined with long-lasting storm surge resulted in catastrophic flooding in Houston, Texas (Valle-Levinson et al., 2020; Huang et al., 2021; Gutenson et al., 2023). This was the second costliest (USD 152.5 billion) natural hazard in US history (NCEI, 2023). As a result of this event, it has been recognized that by failing to consider compound flooding, the risk to Houston and elsewhere had been, and currently remains, greatly underestimated.
Compound flood research at local, regional, and recently global scales has experienced growing recognition and substantial advancements over the past decade, with rapid increases in the number of academic publications (particularly since 2020). However, to date, there have only been a handful of published reviews that have synthesized the current understanding of compound flooding. Moreover, the reviews that do exist have only focused on specific elements of the broader compound flood subject. Bensi et al. (2020) reviewed the drivers and mechanisms of compound flooding, the methods of joint distribution analysis regarding probability hazard assessment, and the key findings of various bivariate coastal–fluvial and coastal–pluvial flood studies. Recently, Guan et al. (2023) completed a brief review of 13 compound pluvial–fluvial flood papers, synthesizing case studies, approaches, and knowledge gaps, in addition to highlighting the value of including damage models in risk management. To the best of our knowledge, three publications have reviewed compound flood modelling approaches in coastal regions (Santiago-Collazo et al., 2019; Xu et al., 2022; Jafarzadegan et al., 2023). Santiago-Collazo et al. (2019) summarized practices of numerical compound flood modelling methodologies including different frameworks for linking (or coupling) multiple hydrologic, hydrodynamic, and ocean circulation models. Xu et al. (2022) examined the advancements, benefits, limitations, and uncertainties of varying numerical and statistical (joint probability and dependence) models and frameworks for compound flood inundation. Lastly, Jafarzadegan et al. (2023) provided a general review of advancements in both univariate riverine and coastal modelling, briefly touching on a hybrid compound modelling approach using linked statistical–hydrodynamic models and physics-informed machine learning (ML). More broadly, two additional papers by Hao et al. (2018) and Zhang et al. (2021a) reviewed the advancing work on compound flood extremes in the realm of hydrometeorology, evaluating the physical drivers and underlying mechanisms (Hao et al., 2018) plus analytical and modelling research methods (Zhang et al., 2021a). Hao et al. (2018) outlined the characteristics and key statistical tools for assessing compound flood and other compound hydroclimatic extremes (drought, heatwave, cold wave, extreme rainfall). Zhang et al. (2021a) discussed these same statistical approaches when reviewing drivers, mechanisms, and means of quantifying risk for compound flooding and four other compound extremes (drought, hot–wet, cold–wet, cold–dry). In addition, they reflected on methods of numerical modelling and collated findings on pluvial-surge, fluvial-surge, sea-level-tide, and fluvial-tide compound flood studies. Regarding compound events and driver dependence, Hao and Singh (2020) and Zscheischler and Seneviratne (2017) reviewed standard methods of measuring dependence (using copulas) as well as approaches for quantifying the likelihood of compound floods. Abbaszadeh et al. (2022) reviewed the sources and challenges of uncertainty in flood modelling and forecasting and offered guidance on reducing uncertainty in the context of compound floods. In addition to these aforementioned papers that reviewed specific aspects of compound flooding, there are several articles (e.g. Leonard et al., 2014; AghaKouchak et al., 2020; Ridder et al., 2020; Zscheischler et al., 2020; Bevacqua et al., 2021; Simmonds et al., 2022; Van Den Hurk et al., 2023) that have reviewed broader compound event research involving a range of hazards beyond just flooding. These papers have discussed compound flooding and provide a diversity of detailed case examples but largely focus on the frameworks, typologies, theories, and perspectives of compound-event-based research and disaster risk reduction as a whole (Leonard et al., 2014; AghaKouchak et al., 2020; Ridder et al., 2020; Zscheischler et al., 2020; Bevacqua et al., 2021; Simmonds et al., 2022). Overall, these previous reviews have provided an excellent synthesis of specific aspects of compound flooding; however, they have each only focused on a narrow area within the much broader compound flooding discipline. To date, a detailed state-of-the-art review of the entire body of compound flood literature has yet to be done.
Therefore, the overall aim of this paper is to carry out a comprehensive systematic review and synthesis of compound flood literature, with a focus on coastal–estuarine regions where compound flooding is most prevalent. We stress that this is not a review of coastal flooding but rather compound flooding occurring in coastal (ocean/lake) and estuarine settings.
To address this aim we have six objectives around which the paper is structured:
-
to survey the range of compound event definitions and terminologies and examine how they pertain to the scope of compound flooding (Sect. 2);
-
to briefly discuss the key physical processes contributing to flood events from individual drivers (Sect. 3);
-
to develop an extensive literature database on compound flood research in coastal–estuarine regions (Sect. 4);
-
to identify trends in the characteristics of compound flood research (Sect. 5);
-
to synthesize the key findings (dependence hotspots and driver dominance), considerations (coastal urban infrastructure and climate change), and standard practices (application cases and analytical methods) of compound flood research (Sect. 6); and
-
to reflect on the knowledge gaps in multivariate flood hazard research and suggest potential directions for research going forward (Sect. 7).
Finally, overall conclusions are given (Sect. 8). Compound flood research is a rapidly developing field of science. As well as providing a comprehensive review, identifying knowledge gaps, and suggesting potential areas for future research, one of our secondary goals of this paper is to provide an initial starting point to better inform researchers and decision-makers new to the emerging field.
Our first objective is to survey the range of compound event terminologies observed in literature and to establish the scope of compound flooding considered in this review. First, we do this broadly, reflecting on the definitions of compound events across different types of hazards (and risks) that have been defined in the literature, and then we examine how the various definitions pertain specifically to compound flood types and accompanying drivers. After this, we seek to champion a unifying definition framework (i.e. encompassing a diversity of perspectives and use cases around compound events) for this review.
Throughout the natural hazard literature, terminology around “compound event”, “compound hazard”, and “multi-hazard” is highly inconsistent. In the past, these terms have sometimes been applied interchangeably. Some refer to compound hazards as a type of multi-hazard event within the larger umbrella of the multi-hazard framework. We believe each of these terms are distinct from one another, and thus for the purposes of this review we use the phrase “compound event”. Examples of different compound event (and related) terminologies are listed in Table 1 (general disaster and hazard definitions are also provided for context). Several terms have been used to describe similar concepts that all broadly involve the consideration of multiple hazards, drivers, mechanisms, variables, and extremes in a multivariate and non-linear assessment of risk (i.e. hazard exposure × vulnerability × capacity) and impact as defined by the IPCC (IPCC, 2012, 2014).
Table 1Examples of different compound event (and related) terminologies, types, and definitions in scientific literature. Unique aspects of varying definitions are emphasized in bold. The “Multi-” category refers to any terminology involving the truncated word root “multi” and includes terms such as “multiple hazards”, “multi-hazards”, “multihazards”, and more.

Use of the term “compound event” (and similar phrases) has been observed in older academic publications (Hewitt and Burton, 1971); however, it was only formally defined in an official context in the 2012 IPCC SREX (Seneviratne et al., 2012). As of present, the most widely accepted definitions of compound events are those from the IPCC SREX (Seneviratne et al., 2012), Leonard et al. (2014), and Zscheischler et al. (2020), which we briefly discuss below.
The IPCC SREX (Seneviratne et al., 2012) defines compound events as a combination of multiple divers or hazards with adverse environmental or social risk/impact. A more detailed explanation is as follows: (1) two or more extreme events occurring simultaneously or successively, (2) combinations of extreme events with underlying conditions that amplify the impact of the events, or (3) combinations of events that are not themselves extremes but lead to an extreme event or impact when combined. The contributing events can be of similar (clustered multiple events) or different type(s).
According to this definition, compound flooding could, for instance, describe the occurrence of a moderate rainfall event that causes surface runoff and discharges at the coast, in addition to elevated coastal water levels from storm surge and wave action (whether simultaneous or a few days later). None, one, or both of the two events may be considered extreme according to threshold or probability-based approaches, but together they lead to extreme coastal water levels. This definition also emphasizes the potential for compounding from the temporal clustering of the same (or different) types of events (e.g. storm clustering involving quick succession of storm events and associated coastal hazards; Jenkins et al., 2023).
Leonard et al. (2014) argue that the IPCC SREX (Seneviratne et al., 2012) definition is unable to capture extreme event edge cases (i.e. unexpected or outlier situations) and is not founded on the physical systems at play. They instead propose a definition that focuses on the variable interactions and event impact, as follows. Our definition emphasizes three characteristics: (1) the extremeness of the impact rather than the climate or weather event, (2) the multivariate nature of the event, and (3) statistical dependence between variables or events that cause the impact.
Thus, according to this definition, the classification of compound flood events necessitates an extreme impact. In the context of flooding, the IPCC SREX may recognize, for example, the simultaneous overtopping of riverine channels and surfacing of groundwater as compounding. However, unless the impact is extreme, it would not pass as a compound flood according to Leonard et al. (2014). This interpretation also requires definitive dependence between the extremes in question. Therefore, a fluke spatiotemporal overlap of extreme rainfall due to an atmospheric river in a region with elevated river levels from recent snowmelt would not be considered a compound flood as the two events are fully independent. In contrast, an intense multivariate storm event involving dependent extreme storm surge and intense rainfall is deemed a compound event.
More recently, Zscheischler et al. (2018) proposed a broader definition that is specific to compound weather/climate events, as follows: the combination of multiple drivers and/or hazards that contributes to societal or environmental risk.
Under this definition, the extremeness of individual drivers and/or hazards is not considered; however, their combination must still exhibit some extent of impact to contribute to overall risk. Furthermore, compound events are strictly limited to the combination of natural (weather/climate) drivers and hazards. Thus, anthropogenic hazards (e.g. dam failure and deforestation) are not included within their scope of compound events. To date, the definition proposed by Zscheischler et al. (2018) offers strong potential for a unified discussion of compound climate events across scientific disciplines. In the past few years, numerous compound flood studies have accordingly adopted their definition framework (Hao and Singh, 2020; Ridder et al., 2020; Bevacqua et al., 2021; Zhang et al., 2021a; Xu et al., 2022).
Finally, for the scope of this review, we adopt the IPCC definitions of “hazard” and “compound event” (IPCC, 2012; Seneviratne et al., 2012) and thus consider compound events as a combination of two or more co-occurring or consecutive drivers (natural or anthropogenic), which together have a greater impact than either of the individual events. Neither the individual driver nor their combinations must explicitly be considered extreme. Potential driver interaction types within this compound event framework include the temporal and/or spatially overlapping combination of multiple hazards (often from shared modulators, e.g. storm event prompts simultaneously rainfall and storm surge), the direct triggering or cascading of one hazard by another (e.g. heavy rainfall on top of existing bankfull river discharge), and the random or by-chance spatial/temporal overlapping of independent hazards (e.g. atmospheric river rainfall during peak spring snowmelt).
Having considered the compound event definitions, our second objective is to briefly discuss the key physical processes contributing to flooding and the individual drivers/hazards recognized in this review. In this review we focus on coastal regions. Here, flooding mainly arises from three main flood drivers, namely (i) fluvial, (ii) pluvial, and (iii) coastal. In this section we start by discussing these three drivers and their mechanisms individually (Sect. 3.1). It is these three drivers, in different combinations, that most often result in compound flood events. Schematic diagrams illustrating the varying flood processes associated with these three main drivers are shown in Fig. 1. However, flooding can also arise from three less frequent auxiliary flood drivers, that is (iv) groundwater, (v) damming and dam failure, and (vi) tsunamis. These additional flood drivers are also briefly discussed (Sect. 3.2). Finally, we also highlight several precursor events and environmental conditions that can influence the magnitude and/or occurrence of flooding (Sect. 3.3).
3.1 Main drivers of flooding in coastal regions
Fluvial flooding (Fig. 1a), also known as river (or riverine) flooding, is induced by the accumulation of large volumes of excessive rainfall and snowmelt. Intense precipitation during extreme meteorological events (e.g. TCs/ETCs and atmospheric rivers) and weather seasons (e.g. monsoons) can inundate rivers quickly (Gori et al., 2020b). Elevated volumes of water cause the level in rivers, creeks, and streams to rise above their channel banks and spill out into the adjacent low-lying area known as the floodplain. Thus, fluvial flooding depends on topography, hydrometeorological conditions, and catchment characteristics (e.g. size, shape, slope, land cover, and soil properties) (Harrison et al., 2022). The peak of river flooding can have a time lag of hours to weeks between the rainfall over a catchment and the exceedance of downstream channels (Valle-Levinson et al., 2020). In the spring, fluvial flooding can also be driven by snowmelt (or glacial melt) as large reservoirs of melting freshwater flow into downstream river channels (Melone, 1985; Benestad and Haugen, 2007). Freshwater fluvial flooding occurs worldwide but is more frequent in high-latitude (e.g. Canada and northern Europe) and high-elevation (e.g. Hindu Kush and Andes Mountains) regions.
Pluvial flooding (Fig. 1b) is the result of intense rainfall (flash flooding) or long-sustained moderate rainfall. As the rain reaches the ground, flooding occurs when the soil becomes fully saturated and can no longer absorb water (saturation excess) and/or the infiltration capacity is overwhelmed (infiltration excess) (Bronstert et al., 2023), causing ponding and surface runoff (overland flooding) that flows down terrain and into rivers (in practice, the boundary between pluvial and fluvial flooding is not well defined and is usually based on catchment area rather than physical process). Urban flooding is closely linked with pluvial flooding where excessive runoff in areas of human development has insufficient drainage, often due to impervious surfaces such as concrete and asphalt (Gallien et al., 2018; Bronstert et al., 2023). Urban flooding also ties in with sewer and stormwater flooding in which pluvial surface runoff infiltrates waste management infrastructure and exceeds drainage system capacity (Mark et al., 2004; Archetti et al., 2011; Gallien et al., 2018; Meyers et al., 2021).
Coastal flooding (Fig. 1c) mainly occurs from one or more combinations of high astronomical tides, storm surge, and wave action (runup, setup, swell, seiche), superimposed on relative mean sea level (Pugh, 1987; Haigh and Nicholls, 2017). Each of these components of total sea level contributes differently to flooding, but we have chosen to group them together for simplicity. Coastal flooding primarily refers to flooding at the interface of land and ocean; however, it is sometimes also used when discussing instances of flooding by these mechanisms (e.g. seiche) along the shoreline of lakes (Stevens and Lawrence, 1997). Tides are the regular and predictable rise and fall of the sea level caused by the gravitational attraction and rotation of the Earth, Moon, and Sun. Tides exhibit diurnal, semi-diurnal, or mixed diurnal cycles and experience shifts in amplitude on fortnightly, bimonthly, and interannual timescales (Pugh, 1987; Haigh et al., 2020). Storm surges are driven by storm events with low atmospheric pressure that cause sea levels to rise and strong winds that force water towards the coastline. Storms also generate waves, locally or remotely (e.g. swell), via the interaction of wind on a water's surface due to boundary friction and energy transfer. Waves mostly contribute to enhanced coastal flooding via setup (the increase in mean water level due to the presence of breaking waves) and runup (the maximum vertical extent of wave uprush on a beach or structure) (Phillips, 1966). Mean sea level is the average height of the sea after filtering out the short-term variations associated with tides, storm surges, and waves. Increases in relative mean sea level arise as a result of vertical land movements (i.e. isostatic SLR) and changes in ocean volume (i.e. eustatic SLR) from thermal expansion of water, mass loss from glaciers and polar ice sheets, and changes in terrestrial water storage (Oppenheimer et al., 2019).
3.2 Other drivers of flooding
In Sect. 3.1 we considered the three main flood drivers, which most frequently contribute to compound flooding in coastal regions. However, other less frequent drivers can also play an important role in compound floods and are briefly summarized below. Groundwater flooding is the rise of the water table to the ground surface or an elevation above human development (Holt, 2019). This occurs during an increase in the volume of water entering an underlying aquifer. This can be the result of prolonged rainfall and snowmelt but in the case of unconfined coastal aquifers can also be driven by SLR and saltwater intrusion (Plane et al., 2019; Befus et al., 2020; Rahimi et al., 2020). Groundwater flooding is often observed along shorelines that are equal to or below sea level (Plane et al., 2019; Befus et al., 2020; Rahimi et al., 2020), in regions with high ground-surface connectivity (Jane et al., 2020), and in areas experiencing ground subsidence (downward vertical shift of Earth's surface from processes such as compaction and groundwater extraction) (Rozell, 2021). As coastal groundwater flooding is the result of long-term changes, it is slow to dissipate and usually persists longer than floods driven by fluvial and pluvial processes (Rozell, 2021).
Damming and dam failure (whether occurring naturally or from anthropogenic activities) can result in flooding from a rapid release or build-up of large volumes of water. Natural damming including beaver dams, ice jams, volcanic dams, morainal dams, and landslide dams can inhibit flow and cause backwater flooding (and even lake formation) (Costa, 1985). Anthropogenic damming is the intentional inundation (via impoundment) of a hydrological network for purposes of resource management (Baxter, 1977). Natural dam failures such as glacial outbursts and landslide dam overtopping can release vast quantities of water that overwhelm and inundate downstream landscapes (Costa, 1985). The failure of human-engineered water control infrastructure (e.g. dams, levees, dykes, water supply systems) can also cause substantial downstream flooding, often posing a greater threat due to the close proximity to human development (e.g. 2017 Oroville Dam crisis (Koskinas et al., 2019) and 2023 Derna dam collapses (ReliefWeb, 2023)).
Tsunamis are a series of impulsive waves generated by the sudden displacement of large volumes of water due to undersea earthquakes and landslides, shifts in the tectonic plates, and underwater volcanic eruptions (IOTIC, 2020). While large-magnitude tsunami events occur infrequently compared to other flood drivers, they still have the potential to cause catastrophic flooding in coastal regions. Tsunamis are also unique in their potential to drive coastal flooding at oceanic scales, sometimes spanning multiple countries and continents (e.g. 2004 Indian Ocean tsunami (Lavigne et al., 2009; Leone et al., 2011) and 2022 Hunga Tonga tsunami (Manneela and Kumar, 2022; Borrero et al., 2023)).
3.3 Precursor events and environmental conditions
In addition to the aforementioned six flood drivers, we also bring to attention five important precursor events and environmental conditions that can strongly influence flooding and whether or not it occurs. First, high anomalous and antecedent soil moisture conditions commonly exacerbate surface flooding due to reduced soil drainage capacity and infiltration (Ganguli et al., 2019; Stein et al., 2019). Elevated freshwater volumes from snowmelt may escalate fluvial and groundwater flooding (Melone, 1985; Benestad and Haugen, 2007; Vormoor et al., 2015). Extreme temp/heat increases precipitable atmospheric water content via elevated relative humidity, as well as amplifies the rate of snowmelt, thus intensifying both pluvial and fluvial flooding, respectively (Berghuijs et al., 2019; Bermúdez et al., 2021). Wildfires can worsen pluvial and fluvial flooding by modifying soil properties such that ash deposits and burnt hydrophobic soils cause rapid surface flows and channelization (Bayazýt and Koç, 2022; Jong-Levinger et al., 2022; Belongia et al., 2023; Xu et al., 2023). Finally, drought is known to potentially intensify pluvial flooding when long-term water deficiencies dry out and harden the soil, in turn reducing ground infiltration and amplifying surface flows (Katwala, 2022). Prolonged drought, wildfire, and extreme heat each lead to vegetation loss, resulting in reduced surface roughness and consequently more intense overland flow. We note that many of these precursors and conditions have partially overlapping influences on flooding as they are inherently interlinked by shared climatic and meteorological forcings.
Our third objective is to develop a database of the extensive English-written scientific literature on compound flood research. In this section we describe how the database was compiled, and then we review and discuss the database contents in objectives four (Sect. 5) and five (Sect. 6).
A combination of systematic review and content analysis was used to collect scientific literature and filter for publications relevant to the scope and themes of this paper. Published journal articles, academic theses, conference proceedings (but not conference abstracts), and government and scientific reports up to and including the year 2022 were sourced using the Web of Science, Semantic Scholar, Google Scholar, and Dimensions AI database search engines. Papers were filtered by topic, title, abstract, and full text (when possible), entering different combinations of key search terms as shown in Table 2. Potential valid articles were also identified from the bibliographies of compound flood papers using literature mapping tools, including Connected Papers, Citation Gecko, Local Citation Network, and Open Knowledge Maps. Research literature was then screened for relevance based on the set of criteria defined below. See Fig. A1 for a PRISMA flow diagram of literature curation.
Table 2Literature database keywords and Boolean search terms. Asterisks act as multi-character wildcards used to capture alternative phrasing of truncated root words (e.g. “flood*” returns “flood-s”, “flood-ed”, and “flood-ing”).
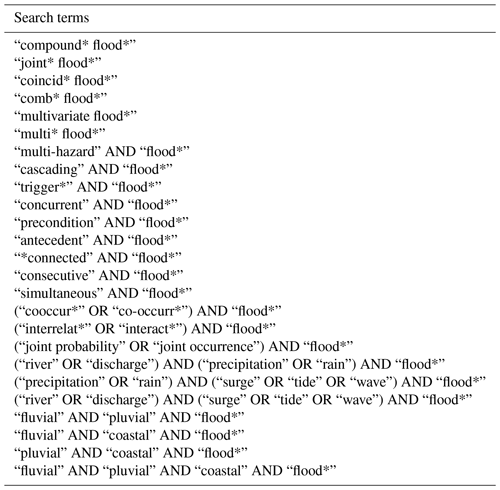
To be included in this review, applicable papers must
-
focus primarily on compound flooding and not simply mention it fleetingly in the abstract or conclusion when in fact addressing univariate flooding;
-
involve multivariate statistical analysis, numerical modelling (hydrological and/or hydrodynamic), and/or discussion of two or more flood drivers, precursors events, or environmental conditions, of which at least one being one of the main three flood drivers (fluvial, pluvial, coastal); and
-
take place in coastal regions (i.e. near an ocean, sea, inlet, estuary, or lake)
Papers deemed appropriate were added to the literature review database and categorized by
-
case study geographic scope;
-
case study scenario;
-
flood drivers, precursor events, and/or environmental conditions considered;
-
research approach (numerical modelling, statistical modelling/analysis, or both); and
-
study application (earth system processes, risk assessment, impact assessment, forecasting, planning and management, and methodological advancement).
To fully clarify the scope of this review, we again emphasize that this review is focused on compound flood literature in coastal (ocean/lake) and estuarine environments. Some may argue that all coastal flooding (or really flooding in general) involves a combination of multiple drivers. While this is not untrue, the majority of historical flood and coastal flood literature has not explicitly focussed on the compounding interactions between the different components of flooding and how those interactions influence flooding as a whole. For this reason, general coastal flood literature that does not explicitly examine the interactions of different flood mechanisms on total flooding is excluded. Additionally, while compound flood literature must examine flooding in coastal and estuarine regions, it does not necessarily require the consideration of coastal drivers to be included (e.g. compound fluvial–pluvial flooding at the coast). Finally, we highlight that historical literature that does not use the phrase “compound flood” may still be included as it would have satisfied the other keyword search terms listed in Table 2.
Keeping in line with the compound event definition framework outlined in Sect. 2 and the individual flood mechanisms detailed in Sect. 3, this review recognizes compound flooding as a combination of two or more of the six flood drivers (fluvial, pluvial, coastal, groundwater, damming/dam failure, and tsunami) and five precursor events and environmental conditions (soil moisture, snow, temp/heat, fire, and drought). In this paper, the coastal driver category will encapsulate processes at lake coasts in addition to oceanic coasts, as lakes exhibit wind-driven oscillating waves (seiche) that contribute to compound flooding similarly to oceanic tide and storm surge. Not considered in the review are studies that assess the co-occurrence or consecutive occurrence of flood characteristics that are not unique to a particular flood driver variable (e.g. flow velocity, flood volume, flood duration, flood intensity, flood depth/height). Additionally, this review does not recognize the confluence or convergence of river channels within the same river network as compound flooding. While there is considerable literature on this subject (e.g. Bender et al., 2016), fluvial–fluvial compounding predominantly occurs inland and therefore is not included within the scope of this paper, which we again emphasize focuses on coastal regions. This review does, however, recognize the compounding of like-type flood drivers in the case of pluvial–pluvial temporal clustering as well as coastal–coastal between different coastal components (e.g. tide–surge, surge–waves, or tide–waves).
While this review aims to provide an overview of existing research on compound flooding, it is necessary to recognize the limitations of the literature review database. Most notably, this review only considers English-written scientific literature and thus may not fully represent the perspectives and findings of all research communities. Throughout the literature database development process, a small number (< 5) of non-English compound flood studies were identified but omitted to preserve a consistent methodology. Similarly, ∼ 10 coastal compound flood papers were identified but inaccessible from the publisher. The final literature database used in this study is extensive but not exhaustive, as some compound flood literature may have been overlooked or excluded based on the drivers, precursor events, and environmental conditions in the review's scope.
From these literature search and database curation methodologies, we obtained a total of 279 compound flood publications. A detailed overview of the compound flood literature database (Green, 2024) is presented in the Appendix (Table A1) and available online at https://doi.org/10.5281/zenodo.14274658.
The fourth objective of the review is to identify and reflect on trends in the characteristics of compound flood research. We discuss general bibliometric characteristics of compound flood literature including publications over time (Sect. 5.1), the geographic scope of compound flood case studies (Sect. 5.2), and the key scientific journals and/or institutions (Sect. 5.3). We then review the flood drivers considered (Sect. 5.4), the analytical approaches applied in the studies (Sect. 5.4), and their various research applications (Sect. 5.5).
5.1 Publications by year
As mentioned previously, we identified 279 publications on compound flooding up to and including the year 2022. The number of publications per year identified in the review is shown in Fig. 2. Up until the year 2000 there were very few compound flood studies (17) (Rossiter, 1961; Myers, 1970; Ho and Myers, 1975; Prandle and Wolf, 1978; Mantz and Wakeling, 1979; Walden et al., 1982; Loganathan et al., 1987; Chou, 1989; Vongvisessomjai and Rojanakamthorn, 1989; Flick, 1991; Tawn, 1992; Acreman, 1994; Coles and Tawn, 1994; Dixon and Tawn, 1994; Jones, 1998; Coles et al., 1999; Rodríguez et al., 1999), with the earliest being Rossiter (1961). Since then, there has been a considerable increase in compound-flood-related papers. The past 3 years (2020–2022) in particular have spawned a considerable number of compound flood papers (133), nearly half (48 %).
5.2 Publications by geographic region
The number of compound-flood-related papers, organized by the geographical region on which the study focuses, are displayed in Fig. 3a and spatially mapped in Fig. 3b. Although there has been increasing focus on the compound nature of flooding, the spatial scope of compound flood research is largely limited to a few geographic regions. Nearly half the publications are directed at compound flooding along the US coastlines (114, 40 %). The spatial distribution of US-related studies is visualized in Fig. 3c. Following the US, some of the next most frequently studied regions are the UK (36, 13 %), China (20, 7.1 %), global (12, 4.3 %), Europe (12, 4.3 %), Australia (9, 3.2 %), the Netherlands (8, 2.8 %), Canada (8, 2.8 %), and Taiwan (7, 2.5 %). Additional geographic regions assessed in < 7 studies are presented in Fig. 3a.
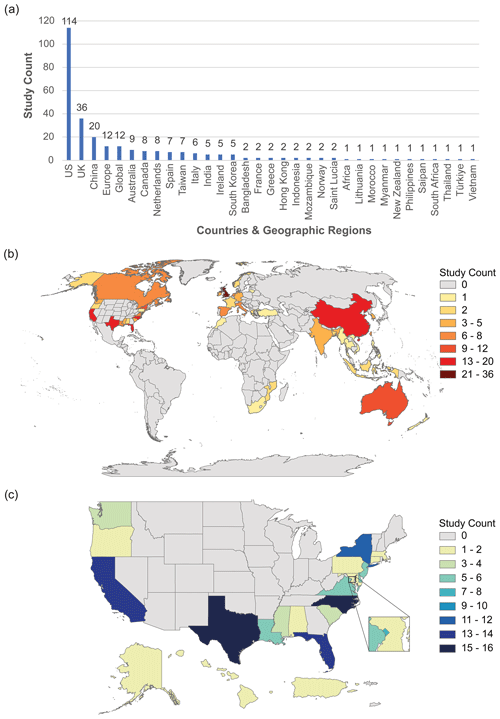
Figure 3(a) Histogram showing the geographic frequency of compound flood case study regions and geographic maps showing the frequency of compound flood case study sites (b) across the world (excluding global studies) and (c) throughout the United States (including Alaska; Hawaii; Puerto Rico; and Washington, DC).
5.3 Publications by journals and institutions
A total of 115 unique scientific journals and institutions (i.e. universities and government agencies) have published compound flood research (i.e. articles, reports, proceedings, and theses). More than half (141, 51 %) of the compound flood literature is published in 15 academic research journals (Fig. 4), with the top five most frequent journals being Natural Hazards and Earth System Sciences (26, 9.3 %), Journal of Hydrology (14, 5.0 %), Hydrology and Earth System Sciences (11, 3.9 %), Water Resources Research (10, 3.6 %), and Water (10, 3.6 %). Although a considerable volume of compound flood research is published by a select few journals and institutions, a total of 71 journals and institutions have only published a single compound flood study. We suspect that this will change in the years to come as the field of compound flood hazards gains further attention.
5.4 Review of flood drivers considered
Across the 279 studies in the review database, a total of 11 unique compound flood drivers, precursor events, and environmental conditions were identified. These are listed in Table 3 and visualized in Fig. 5. Due to the highly complex interactions between terrestrial, oceanic, and atmospheric systems, most studies choose to limit the scope of their research to a select few flood-driving mechanisms. For instance, some focus on TC/ETC and extreme precipitation events, while others address elevated river discharge in tandem with storm surge. Looking at the combination of drivers analysed, 44 (16 %) studies considered exactly the three main components of compound flooding (fluvial, pluvial, coastal); note that analysis of three drivers does not necessarily dictate trivariate analysis (e.g. fluvial–pluvial–coastal) but can also describe two separate bivariate analyses (e.g. fluvial–coastal and pluvial–fluvial) that together include three drivers. The remainder of the studies largely considered combinations of the main drivers (often as bivariate analyses), with the most prominent being fluvial–coastal (84, 30 %), pluvial–coastal (80, 29 %), and coastal–coastal (38, 14 %) (e.g. surge and tide) (Fig. 5). These results are to be expected as compounding is most prevalent at the coast. A select few examples of unique and less frequently studied compound flood driver combinations include pluvial–snow (Lawrence et al., 2014), pluvial–fire (Cannon et al., 2008; Bayazýt and Koç, 2022; Jong-Levinger et al., 2022), coastal–tsunami (Kowalik and Proshutinsky, 2010; Zhang et al., 2011), pluvial–temp/heat (Benestad and Haugen, 2007), pluvial–drought (Ridder et al., 2020), pluvial–coastal–damming/dam failure (Kim and Sanders, 2016), and coastal–groundwater (Habel et al., 2020).
Table 3List of unique flood drivers, precursor events, and environmental conditions (plus terms and variables) observed in compound flood research from the literature review database.
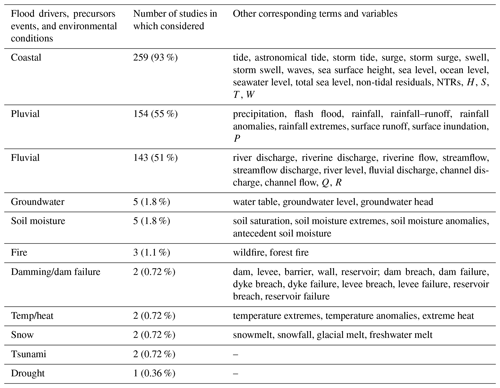
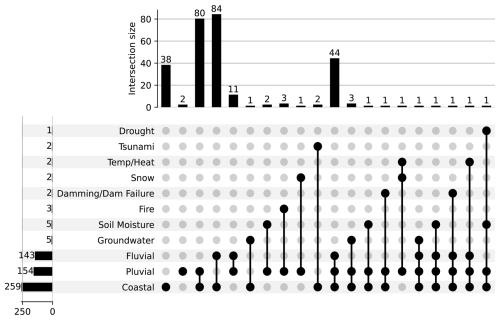
Figure 5UpSet plot (Lex et al., 2014) visualizing the combinations and frequency of driver multi-classifications assigned across the literature. The vertical histogram presents the total count of studies considering each of the 11 drivers (plus precursor events and environmental conditions) categorized non-exclusively, while the horizontal histogram presents the total count for each driver multi-classification combination exclusively. Flood driver classifications for like-type compounding (i.e. pluvial–pluvial and coastal–coastal) are indicated by a non-linked circle. Note that analysis of three drivers does not necessarily dictate trivariate analysis (e.g. fluvial–pluvial–coastal). It may instead describe two separate bivariate analyses (e.g. fluvial–coastal and pluvial–fluvial) as part of the same study that together consider three drivers.
5.5 Review of research approaches
Across the database, the compound flood studies have tended to apply approaches that generally fall into two categories: (1) physical (process-based) numerical modelling and/or (2) statistical modelling and analysis (similar findings to that of Tilloy et al., 2019). The number of studies applying each approach is illustrated in Fig. 6. In total, 102 (36 %) studies used only numerical modelling approaches, 95 (34 %) studies used only statistical approaches, and 80 (29 %) studies applied hybrid methods involving a combination of numerical and statistical approaches. Within the main two approach classes are many different methods for investigating compound floods, each of which exhibits its own benefits and limitations as discussed in Sect. 6. Lastly, 2 (< 1 %) studies used neither of these approaches, instead completing qualitative survey-based investigations related to the perception and understanding of compound flooding by disaster managers and the wider public (Curtis et al., 2022; Modrakowski et al., 2022).
5.6 Review of research applications
Across the database, the compound flood studies have tended to relate to six main application themes, as illustrated in Fig. 7. Assessing the individual research application categories non-exclusively, 130 (47 %) studies consider earth system processes, 129 (46 %) risk assessment, 11 (3.9 %) impact assessment, 21 (7.5 %) forecasting, 28 (10 %) planning and management, and 80 (29 %) methodological advancement (Fig. 7). These applications are discussed in more detail in Sect. 6.7. Reflecting on the exclusive multi-classification of applications, the three most common classifications are earth system processes (74, 27 %), risk assessment (50, 18 %), and methodological advancement (31, 11 %), which together account for over half of the literature database entries (Fig. 7). This is to be expected as they are the broadest of application categories but also the primary objective of most research. Other prominent research application classification categories include earth system processes and risk assessment (30, 11 %), methodological advancement and risk assessment (22, 7.9 %), earth system processes and methodological advancement (19, 6.8 %), and planning and management and risk assessment (12, 4.3 %) (Fig. 7).
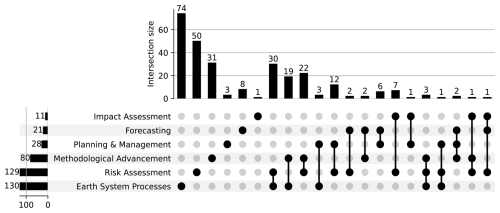
Figure 7UpSet plot (Lex et al., 2014) visualizing the combinations and frequency of application multi-classifications assigned across the literature. The vertical histogram presents the total count of studies considering each of the six application categories non-exclusively, while the horizontal histogram presents the total count of each application multi-classification combination exclusively. Instances of single application classification are indicated by a non-linked circle.
Our fifth objective is to synthesize the key findings (e.g. dependence hotspots and driver dominance), considerations (e.g. uncertainty and climate change), and standard practices (e.g. application cases and analytical methods) of the compound flood research from across the database. First, we examine the global and regional hotspots of compound flooding, outlining where and when different driver pairs exhibit significant dependence (Sect. 6.1). Next, we discuss the tendency for certain drivers to dominate the compound flooding process and examine how this changes spatially as influenced by landscape characteristics (Sect. 6.2). We then consider compound flooding in the context of urban and coastal infrastructure and how these environments are particularly susceptible to the compounding drivers as it is a common consideration throughout the literature (Sect. 6.3). Next, we assess how climate change is expected to affect the frequency, variability, and severity of compound flooding in the future (Sect. 6.4). Then, we reflect on the different approaches that have been used in the literature to analyse compound flooding (Sect. 6.5). Finally, we investigate the range of different applications considered across the literature (Sect. 6.6).
6.1 Compound flood hotspots and spatiotemporal dependence patterns
Our review highlights that knowledge of compound flooding hotspots, spatiotemporal patterns, and multivariate dependence characteristics has advanced considerably in recent years. However, the ways in which global meteorological and climate modulators affect the propensity of compound flooding in one region over another are not fully understood, and few studies consider the nonstationarity of multivariate flood variable dependence. Nonetheless, large-scale patterns in the seasonal and interannual occurrence of compound events have become apparent in several regions (Wu et al., 2018; Ganguli and Merz, 2019a, b; Ridder et al., 2020; Lai et al., 2021a; Lai et al., 2021b; Camus et al., 2022; Stephens and Wu, 2022).
Existing compound event literature has identified certain areas around the world that are especially prone to compound flooding, namely southern Asia, where monsoon floods and cyclones cause widespread damage; the Gulf Coast and East Coast of the United States, where hurricanes induce storm surge and intense rainfall, which exacerbate pluvial and/or fluvial flooding; global low-lying delta regions (e.g. Ganges, Irrawaddy, Mekong, Mississippi, Rhine, and Pearl), where riverine and coastal waters together induce severe flooding; northern and western Europe, which are prone to river flooding plus extreme precipitation and surge from storm events; and coastal areas of East Asia, Southeast Asia, and Oceania, where TCs/ETCs drive joint fluvial and coastal flooding (Apel et al., 2016; Ikeuchi et al., 2017; Bevacqua et al., 2020a; Couasnon et al., 2020; Eilander et al., 2020; Camus et al., 2021; Lai et al., 2021a). Below we further detail the spatiotemporal patterns in compound flooding and driver interdependence by region.
North America. The coasts of North America are the most studied in terms of compound flooding globally. Compound flooding predominantly occurs along the mid-eastern US coastline and the Gulf of Mexico due to TCs/ETCs that generate heavy rainfall and extreme sea levels (Ridder et al., 2020; Camus et al., 2021; Najafi et al., 2021; Camus et al., 2022). Joint pluvial–fluvial extremes account for the majority of compound flood events and occur frequently with low return periods (< 0.5 years) over the entire contiguous US, particularly along the coasts (Ridder et al., 2020). Coastal–fluvial drivers too exhibit positive dependence on both coasts (Ridder et al., 2020). Dependence is also measured between flood drivers along Canada's coasts, albeit less frequent relative to the US (Jalili Pirani and Najafi, 2020). Throughout the Great Lakes, consistent significant positive dependence is found between pluvial–coastal drivers. On the North American east coast, pluvial–fluvial extremes are frequent in late spring and early summer during the Atlantic hurricane season (Ridder et al., 2020; Nasr et al., 2021). This region exhibits strong correlations between pluvial–coastal (Wahl et al., 2015; Lai et al., 2021a) and fluvial–coastal (Moftakhari et al., 2017) drivers (Camus et al., 2021; Nasr et al., 2021). Lastly, the North American west coast features positive dependence for fluvial–coastal (Ward et al., 2018) and pluvial–coastal (Lai et al., 2021a) pairs during the winter ETC season (Nasr et al., 2021).
Central and South America. Current knowledge of compound flood events in Central and South America is lacking due to a void of localized research. Global studies on compound flooding indicate that fluvial–pluvial extremes are the most frequent cause of compound flooding in South America and largely occur in the eastern half of the continent (particularly Brazil) during austral summer/late autumn (Ridder et al., 2020). Similarly, there is a positive dependence between fluvial–coastal flood drivers on the southeast coast of Brazil, with large clustering in the highly populated states of São Paulo and Rio de Janeiro (Ward et al., 2018; Couasnon et al., 2020; Ridder et al., 2020). On the west coast, co-occurring fluvial–coastal extremes are located in the southern portion of Chile in austral summer (Couasnon et al., 2020; Ridder et al., 2020).
Europe. Across Europe, large-scale low-pressure systems are a prominent modulator of compound floods (Ridder et al., 2020), with most (∼ 90 %) events (Camus et al., 2021) occurring in the winter ETC season (Ridder et al., 2020; Lai et al., 2021a; Camus et al., 2022). The main hotspots of compound flooding are the west coast of the UK, the northwest coast of the Iberian Peninsula, around the Strait of Gibraltar, coasts along the North Sea, and the eastern portion of the Baltic Sea (Ward et al., 2018; Couasnon et al., 2020; Ridder et al., 2020; Camus et al., 2021). Concomitant pluvial–fluvial and pluvial–coastal extremes are most prominent in western Europe (Couasnon et al., 2020; Ridder et al., 2020; Camus et al., 2021; Lai et al., 2021a). In Ireland and the UK, joint occurrences of high skew surges and high river discharge are more common on the west and southwest coasts compared to the east coast (Svensson and Jones, 2002, 2004; Ward et al., 2018; Hendry et al., 2019; Camus et al., 2021). Pluvial–fluvial drivers also show strong positive correlations in southern Italy, the east coast of Türkiye, the eastern Mediterranean, the coasts along the North Sea, and parts of the Baltics. Compound rainfall and river discharge occur primarily in the early summer to late autumn. For fluvial–coastal and pluvial–coastal driver dependence, there are strong correlations along the Iberian coasts, the Strait of Gibraltar, and the UK west coast (Svensson and Jones, 2003; Svensson and Jones, 2004; Ward et al., 2018; Camus et al., 2021; Lai et al., 2021a). Lastly, positive pairwise dependence of temporally compounding pluvial–pluvial (“wet–wet”) conditions is prominent along the coastal Mediterranean (De Michele et al., 2020).
Africa. Research in Africa is sparse relative to the other continents; however, a few compound flood patterns have been ascertained along the northern, southern, and eastern coasts. Portions of northern Africa show significant positive pluvial–fluvial correlation along the southern Mediterranean and eastern Atlantic coasts including Libya, Tunisia, Algeria, and especially Morocco (Camus et al., 2021). Morocco has the greatest compound flood potential in northern Africa as it also demonstrates strong dependence for coastal–pluvial (Zellou and Rahali, 2019) and coastal–fluvial extremes (Camus et al., 2021). Analysis of rain gauges across northern Africa also reveals a select few sites in Algeria with pluvial–pluvial (wet–wet) pairwise dependence (De Michele et al., 2020). In southern and eastern Africa, both South Africa and Mozambique experience compound flooding from seasonal TCs during austral summer (Bischiniotis et al., 2018; Ward et al., 2018; Couasnon et al., 2020; Ridder et al., 2020; Claassen et al., 2023). As a result, this region has strong dependence relationships between the flood driver pairs coastal–fluvial, coastal–pluvial, and pluvial–fluvial (Van Berchum et al., 2020; Eilander et al., 2023; Kupfer et al., 2022). Lastly, Madagascar has significant positive coastal–fluvial dependence (Couasnon et al., 2020; Ridder et al., 2020) also due to its exposure to TCs (Claassen et al., 2023).
Asia. Compound flood spatiotemporal distributions are highly varied throughout Asia but tend to be most frequent in the south, southeast, and east. Strong correlations for fluvial–coastal extremes are seen at the coasts of India and Bangladesh (Bay of Bengal), Indonesia (North Natuna Sea), Vietnam (East Sea), Philippines (West/East Philippine seas), Malaysia, China, Taiwan, and Japan (Sea of Japan) (Ikeuchi et al., 2017; Ward et al., 2018; Couasnon et al., 2020; Ridder et al., 2020; Leijnse et al., 2021; Pandey et al., 2021; Sampurno et al., 2022b). Similarly, there is positive dependence for pluvial–fluvial drivers in India, Bangladesh, and Japan (Ganguli et al., 2019; Ridder et al., 2020; Khatun et al., 2022; Claassen et al., 2023). Co-occurring pluvial–coastal extremes are most prominent in the wet monsoon season in East Asia (particularly China, Taiwan, and Japan) (Lai et al., 2021a; Lai et al., 2021b), Southeast Asia (Lu et al., 2022) and South Asia (Vongvisessomjai and Rojanakamthorn, 1989; Shahapure et al., 2010; Mohanty et al., 2020). Most compound flood events within Asia occur from summer to late autumn, corresponding with the TC/ETC seasonality in the western Pacific.
Oceania. Within Oceania, compound flood events have been primarily observed in Australia and to a lesser degree New Zealand. In Australia, the highest frequency of compound flood events is along the northern coastlines (bearing the brunt of TCs; Claassen et al., 2023) followed by the east and west coasts, all of which predominantly occur during TC season in austral summer. Examining dependence, these patterns are consistent for nearly all flood driver pair combinations, with strong positive correlation in all areas except the southern coast (particularly Victoria) for pluvial–coastal, fluvial–coastal, and pluvial–fluvial (Zheng et al., 2013; Ward et al., 2018; Wu et al., 2018; Couasnon et al., 2020; Ridder et al., 2020; Lai et al., 2021a; Lai et al., 2021b). In New Zealand, compound flood events from pluvial–coastal and fluvial–coastal drivers have been observed as being substantial but are not strongly correlated (Stephens and Wu, 2022). Compound flooding likely affects small Pacific island nations; however, they have been scarcely studied. To date, there are only two localized studies (Chou, 1989; Habel et al., 2020) on co-occurring flood extremes for the entirety of Micronesia, Melanesia, and Polynesia. Habel et al. (2020) confirmed the occurrence of coastal–groundwater and pluvial–coastal flooding processes in Hawaii, and Chou (1989) quantified the frequency of compound flooding from tide and storm surge along Saipan in the Mariana Islands.
6.2 Dominant drivers of compound flooding
While compound flood events involve a combination of drivers, often one of the components contributes more than the other(s). Understanding how drivers dominate the flooding process and how these change with space and time is essential to improving compound flood forecasting and risk assessment. Most compound flood events highlighted in the literature contain regions that are pluvial-, fluvial-, coastal-, groundwater-, or compound-dominated in nature. Only a handful of studies examine driver dominance at a global scale (Eilander et al., 2020; Lai et al., 2021b), but those that do reveal general patterns that also tend to be supported by more localized research. First, estuaries tend to have a mixture of dominant drivers. In a global assessment of 3433 estuaries, Eilander et al. (2020) classified 19.7 % as compound-dominant, 69.2 % as fluvial-dominant, and 7.8 % as coastal-dominant. Next, coastal-only environments (i.e. coastal areas with little or no river interaction) have a much larger proportion of coastal-dominant compound floods due to the direct proximity of tide–surge processes and wave actions, as well as groundwater-dominated floods where sea level (and salinity differences) push the water table up. Excluding river processes, Lai et al. (2021b) deduced that coastal (storm surge) and pluvial flooding contributed 65 % and 35 % to the global change in annual compound floods, respectively. Finally, urban coastal regions are expected to have a greater number of pluvial-dominated compound floods.
Flood driver dominance can depend on topography and channel morphology (i.e. depth, width, size, shape, volume, slope, friction, and damping) (Eilander et al., 2020; Bermúdez et al., 2021; Tanim and Goharian, 2021; Familkhalili et al., 2022; Harrison et al., 2022), spatial extent (i.e. location within hydrological network and distance to the coast) (Moftakhari et al., 2019; Bermúdez et al., 2021; Del-Rosal-Salido et al., 2021; Huang et al., 2021; Ye et al., 2021; Gori and Lin, 2022; Juárez et al., 2022; Sampurno et al., 2022b; Sebastian, 2022; Zhang and Chen, 2022), elevation (Huang et al., 2021; Liang and Zhou, 2022), ground-surface connectivity (Jane et al., 2020), and meteorologic modulator characteristics (i.e. storm event timing and intensity) (Tanim and Goharian, 2021; Gori and Lin, 2022). Pluvial flooding is the least frequently reported dominating driver and primarily only occurs in areas disconnected from the river network with no fluvial inundation (Apel et al., 2016; Ye et al., 2021; Gori and Lin, 2022) or at higher elevations (Berghuijs et al., 2019; Huang et al., 2021). Pluvial-dominated flooding is also prevalent in urban zones when the capacity of drainage systems is exceeded (Shi et al., 2022), areas with high antecedent soil moisture (e.g. Europe as a whole) and/or snowmelt (e.g. Scandinavia and northeast Europe) (Berghuijs et al., 2019), and regions with strong connectivity of surface and groundwater networks (Jane et al., 2020). Fluvial processes dominate inland flooding in watershed catchments from channelized fresh water in dynamic hydrological networks. Flooding can also be fluvial-dominant in coastal regions fed by steep mountainous rivers that respond quickly to rainfall and snowmelt (e.g. Zhejiang China; Liang and Zhou, 2022). Within primarily coastally influenced regions, driver dominance can be further broken down into surge-, wave-, and tide-dominated. Which of the components of extreme sea level is the principal driver varies on continental to regional scales depending on meteorological modulators and characteristics of landmasses.
In the case of mixed fluvial and coastal flooding in estuaries and deltas, identifying the dominant driver is more challenging as it varies based on location and channel geomorphology. River–sea interactions are highly dynamic, and the sensitivities of flood components can fluctuate greatly within a single estuary or delta (Hoitink and Jay, 2016; Harrison et al., 2022). Common methods of classifying regions of driver dominance usually involve using flow interaction indices (Valle-Levinson et al., 2020; Juárez et al., 2022) and compound hazard ratio indices (Shen et al., 2019; Valle-Levinson et al., 2020; Jalili Pirani and Najafi, 2022b; Juárez et al., 2022). As expected, most researchers have found that the lower estuary is tide- or surge-dominated, the middle estuary transition zone may be considered compound-dominated, and the upper watershed region is discharge-dominated (Moftakhari et al., 2019; Bermúdez et al., 2021; Del-Rosal-Salido et al., 2021; Huang et al., 2021; Ye et al., 2021; Gori and Lin, 2022; Juárez et al., 2022; Qiu et al., 2022; Sampurno et al., 2022b; Sebastian, 2022; Zhang and Chen, 2022). General patterns of driver dominance are different across estuaries depending on the properties of watershed drainage basins (i.e. topography and morphology) and the behaviour of storm events (i.e. path, orientation, intensity, duration, and time lag between drivers). Numerous studies map out regions dominated by each of the different flood drivers (Chen et al., 2010; de Bruijn et al., 2014; Gori et al., 2020b; Bilskie et al., 2021; Del-Rosal-Salido et al., 2021; Maymandi et al., 2022), often zoned as coastal, hydrological (fluvial and/or pluvial), or transition/compound (combined drivers determine the max water levels) based on numerical model simulations using different scenarios. The exact scenario definitions, however, often vary between studies, making it difficult to compare results. Compound-dominant floods usually have greater surge extremes and quicker discharge due in part to flatter topography (Eilander et al., 2022). In estuaries, channel convergence has been shown to influence tidal propagation such that strongly dissipative and convergent estuaries tend to be flood-tide-dominant, while weakly dissipative estuaries are ebb-tide-dominated (Lanzoni and Seminara, 1998). Large rivers are usually fluvial-dominant, while smaller and less connected rivers are more likely to be influenced by precipitation at the coast (Bevacqua et al., 2020a). Similarly, increasing channel depth reduces the impact of fluvial processes while amplifying the effect of coastal drivers on total water level (Familkhalili et al., 2022). Therefore, channel deepening pushes the compound-dominated region further upstream and shortens the length of fluvial-dominated estuary. Flood dominance can also be significantly affected by the magnitude and severity of storm events such that a single location can be dominated by different drivers from different return period storms. Gori et al. (2022) observed surge-dominated flooding at the coast for low-return-period events but compound-dominated flooding for high (100-year) return periods.
Fewer studies have examined the role of timing on flood driver dominance. In the case of TC/ETC events, there is a time lag such that it can be hypothesized that coastal areas are first inundated by storm tide followed by river discharge from upstream rainfall. Thus, at the beginning of storm events flooding is likely coastal-dominated (and/or pluvial-dominated) and later switches to being compound-dominated and then finally fluvial-dominated (and/or pluvial-dominated). For instance, the 1991 cyclone that hit Chittagong, Bangladesh, had a 5 h difference between peak surge and peak rainfall (Tanim and Goharian, 2021). As a result, the flooding began as coastal-dominated and then shifted towards being pluvial-dominated. The importance of timing may also fluctuate depending on the size of the water bodies in question. Dykstra and Dzwonkowski (2021) found that slowing of river propagation in larger watersheds (> 5000 km2) led to a greater time lag between storm surge and river discharge, indicating a greater risk of fluvial–coastal compounding in smaller watersheds where discharge travels downstream faster. Likewise, differences observed in the UK's Humber and Dyfi estuaries explain why maximum flood depth from fluvial–coastal compounding is less sensitive to timing in the case of a larger estuary (Humber) subject to slow river discharge, compared with short intense discharge in a smaller estuary (Dyfi) (Harrison et al., 2022).
6.3 Urban and coastal infrastructure
Urban areas are identified in the literature database to be especially vulnerable to compound flooding, as the built environment can exacerbate the effects of flooding, and the concentration of people and infrastructure can lead to significant losses. In the coastal environment, hazard modelling and risk assessment practices regularly consider the influence of flood defence structure (i.e. barriers, sea walls, groynes, breakwaters); however, other aspects of human activity (e.g. coastal and floodplain development and modification, land use/land cover change) and urban infrastructure (e.g. sewer waste drainage systems, water management reservoirs) receive less attention. Furthermore, existing urban infrastructure planning and risk assessment practices generally do not consider the ramifications of compounding flood drivers and thus underperform or have a greater chance of failure from compound flooding (Archetti et al., 2011; Jasim et al., 2020; Najafi et al., 2021). For instance, in Jasim et al. (2020), coastal earthen levees were simulated to experience 8.7 % and 18.6 % reductions in the factor of safety for 2-year and 50-year recurrence intervals under compound pluvial–fluvial flood conditions compared to fluvial-only flooding. Similarly, Khanam et al. (2021) found that FEMA maps significantly underestimate risk at several power grid substations in coastal Connecticut by not accounting for compound flood interactions. This section will discuss how compound floods influence the performance of urban and coastal infrastructure and how infrastructure in these settings can either amplify or reduce the risks and impacts of compound floods.
It is well established that the risks and impacts of compound flooding can be elevated in coastal and urban settings. Private property and public utilities developed within floodplains and along shorelines are more likely to be exposed to multiple coinciding flood mechanisms. Over the past century, changes in land use and land cover have made the urban environment increasingly susceptible to flooding. Urban areas experience increased precipitation as unstable warm city air masses rise (i.e. urban heat island effect) and then cool, forming rain clouds. This rain falls onto impervious surfaces (i.e. asphalt and concrete) and compacted soils (from construction and agriculture), which prevent surface water from seeping into the ground and percolating down into underlying aquifers (Shahapure et al., 2010). Instead, water finds its way into river channels and urban drainage networks which act as highways and rapidly deliver vast volumes of water to the coast. During TC events, rainfall and river discharge are more likely to temporally overlap with coastal storm surge due to the heightened mobility of water within the urban environment. It is this combination of urban land cover and storm sewer drainage infrastructure that plays a substantial part in amplifying the impacts of urban coastal compound floods (Meyers et al., 2021). It has been well demonstrated that elevated water levels at the coast from storm surge can significantly reduce the rates of urban drainage resulting in more severe flooding (Bunya et al., 2010; Zellou and Rahali, 2019; Shi et al., 2022). Accumulated surface runoff in cities is meant to flow into rivers and ultimately the ocean, but high tides or waves can either block or force this water back inland. It has also been shown that poorly maintained and leaking stormwater drainage systems can cause compound pluvial–groundwater and fluvial–groundwater flooding where seawater travels inland via drainage systems (known as “drainage backflow” and “seawater intrusion”) and flood areas near (and sometimes far from) the coast (Habel et al., 2020; Qiang et al., 2021; Sangsefidi et al., 2022; Sebastian, 2022). Furthermore, human activity including coastal and riverine modifications (i.e. dredging and straightening) (Muñoz et al., 2022b) in favour of water utilities (e.g. hydroelectric) and transportation (e.g. marine shipping) also may increase the risks and impacts of compound flooding as decreased channel friction causes heightened propagation. Changing the morphology of coastal channels as often seen in urban ports can amplify fluvial–coastal and pluvial–coastal compound flooding due to reduced dissipation of energy and thus increased extreme peaks. Lastly, urban and coastal environments also pose the rare but catastrophic potential of damming/dam-failure-related compound flooding. For example, Typhoon Rusa led to compound coastal–pluvial–damming/dam failure flooding in the urban city of Gangneung (Kim and Sanders, 2016). The failure of two upstream dams in combination with heavy rainfall and storm surge caused extensive damage to major infrastructure and affected hundreds of households. A very similar scenario occurred in Qianbujing Creek, Shanghai, during Typhoon Fitow, involving compound heavy rainfall, river discharge, and levee-break flooding (Yang et al., 2021).
Urban infrastructure can also reduce the risks and impacts of compound flooding if designed to be resilient and forward-looking. Management and policy decisions regarding urban infrastructure investment, maintenance, and outreach can play a large role in shaping compound event risk through the lens of population exposure and vulnerability (Raymond et al., 2020). Well-maintained and operated coastal urban infrastructure from flood defence (e.g. storm surge barriers, sea walls, levees, breakwaters, and groynes) to flow management systems (e.g. dams, stormwater sewers, sump pumps, dry wells) can act to minimize compound flood risk when the dependence of multiple drivers is adequately considered. Furthermore, sustainable urban drainage systems (e.g. swales, infiltration trenches, retention basins, green roofs, and permeable paving) (EAA, 2017) can reduce the likelihood of compound flooding as they can create a time lag between peak pluvial, groundwater, and coastal processes. Lastly, natural flood management practices (e.g. wetland/floodplain/lake restoration, riverbed material re-naturalization, river re-meandering) (EAA, 2017) can also serve to spread out the duration and reduce the acute impact of compounding involving fluvial and coastal drivers, advancing the resiliency of urban and coastal environments.
6.4 Compound flooding and changing climate
Many studies in the database stress that future compound flood risk is likely to increase from changes in the variability, intensity, frequency, phasing, and seasonality of sea level, precipitation, river discharge, and temperature driven by climate change (Zscheischler et al., 2020; Harrison et al., 2022). Under a changing climate the interrelationships and dependence between variables contributing to compound events are likely to change, giving rise to greater uncertainty. A projected warmer atmosphere will bring more frequent and intense storms and rainfall in many parts of the world (Bevacqua et al., 2019; Bevacqua et al., 2020b; Wasko et al., 2021; Zhang et al., 2022), with some estimating a 25 % global increase in compound floods by 2100 (RCP8.5) (Bevacqua et al., 2020b). For example, the UK is expected to see increased clustering and intensity of storms (particularly in the winter) such as those seen in 2013/14 (Harrison et al., 2022; Jenkins et al., 2023). The relative influence of rainfall on total flooding is increasing due to the warming climate (Swain et al., 2020; Burn and Whitfield, 2023), and long-term increases in the frequency of compound coastal river flooding from intensifying precipitation has already been observed throughout the past century (Dykstra and Dzwonkowski, 2021). This is particularly the case for the high latitudes (Bevacqua et al., 2020b), including the US East Coast and Gulf Coast as well as northern Europe, which face increasing risk from the joint occurrence of rainfall and storm surge (Wahl et al., 2015; Bevacqua et al., 2019; Ghanbari et al., 2021; Gori et al., 2022), having already seen a rise in the number of annual compound events by one to four per decade (Lai et al., 2021b). Trends of raising frequency of concurrent precipitation and storm tide have additionally been observed at other coasts globally, including Russia, Japan, Korea, China, Bangladesh, northwest South America, southern Chile, northern Australia, and New Zealand (Bevacqua et al., 2020b; Lai et al., 2021b). SLR is additionally anticipated to substantially amplify the likelihood of compound flooding at the coast (Wahl et al., 2015; Ganguli et al., 2020; Bermúdez et al., 2021; Ghanbari et al., 2021; Harrison et al., 2022), with global mean sea level projected to increase 0.61–1.10 m (RCP8.5) by 2100 (Church et al., 2013). This is already drastically affecting island nations in Southeast Asia and the Pacific that are vulnerable to compound coastal flooding involving storm events (Kuleshov et al., 2014; Hsiao et al., 2021; Leijnse et al., 2021). Global coastal regions have become increasingly sensitive to inundation from combined influences of SLR, surge, tide, and waves (Dahl et al., 2017; Idier et al., 2019; Oppenheimer et al., 2019; Sheng et al., 2022). This is evident in coastal South and Southeast Asia where climate-induced storminess and high-tide extremes increasingly drive more extreme sea levels, in addition to sea-level rise (Xu et al., 2014; Wood et al., 2024). Tidal amplitude is also changing globally (Pickering et al., 2017), and in some regions it is driving a greater proportion of both extreme and nuisance flooding (Pickering et al., 2017; Sweet et al., 2018; Haigh et al., 2020; Shen et al., 2022). Total coastal flooding globally is estimated to be 68 % caused by storm and tide with 32 % attributed to relative SLR (RCP8.5) (Kirezci et al., 2020). Furthermore, nonstationarity in compound flooding has been well documented, with climate-induced shifts in the seasonal timing of peak flood driver occurrence. Analysis of historical long-term flood driver trends throughout Europe has revealed single-week to full-month shifts in mean flood occurrence date. Rainfall and river floods have shifted earlier along the Atlantic, in the Baltics, and in western Italy and later in eastern Europe, in southern France and Spain, and along the North Sea (Blöschl et al., 2017; Tramblay et al., 2023). In the case of mixed rainfall–snowmelt coastal catchments in the Nordic countries, Vormoor et al. (2015) observed a shift forward in the flood regime from spring–summer to fall–winter as rainfall replaces snowmelt as the dominant driver due to raising temperatures.
While compound flood frequency is generally thought to increase globally, it is critical to understand that compound flooding may also decrease in some select regions due to changing local hydrometeorological and climatic forcings. In the case of the upper Mahanadi River basin, Khatun et al. (2022) projected lower compound flood hazards under future climate scenarios involving preconditioned rainfall and river discharge. Bevacqua et al. (2020b) project that the joint probability of rainfall and storm surge will decrease in portions of the subtropics, noting the most significant shift in the Mediterranean and the Strait of Gibraltar (Bevacqua et al., 2019) and potentially attributing changes to reduced regional extreme sea levels. Lai et al. (2021b) have similar findings, observing a decrease in annual compound flood events in the southern Mediterranean and Japan. In contrast to that of Bevacqua et al. (2020b), Ganguli et al. (2020) project a lower joint probability of storm surge and river discharge extremes in northwest Europe, attributing changes to weakening driver dependence. The conflicting findings of these two studies highlight the limitations (e.g. sensitivity, internal variability, and uncertainty) of using a small ensemble of climate models for projecting future compound flood joint probability. Lastly, many of these trends towards decreased compounding are the result of changes in sea-level pressure, coastal wind, precipitable water content, and convection patterns that either reduce the magnitude of individual flood drivers (often precipitation in tropics) or the dependence between drivers.
In summary, across the studies reviewed, climate change is shown to have a profound impact on the frequency, severity, and timing of compound coastal flooding events (Sebastian, 2022). Furthermore, extreme total sea levels from the combination of SLR, surge, waves, tidal cycles, and changes in the frequency and intensity of storms are very likely to increase over the next century in many regions of the world (Idier et al., 2019; Oppenheimer et al., 2019).
6.5 Research approaches
As highlighted in Sect. 5.4, we identified two main categories of approaches that have been used to assess compound flooding, namely (1) physical (process-based) numerical modelling and/or (2) statistical modelling/analysis. In both approach classes we observed a diversity of methods, similar to the findings of Tilloy et al. (2019). Below, we discuss the use of computational numerical methods for compound flood modelling (Sect. 6.5.1), then provide an overview of the statistical and data-science-based techniques for analysing compound flooding (Sect. 6.5.2), and finally reflect on the benefits of hybrid (combined numerical–statistical) modelling approaches (Sect. 6.5.3).
6.5.1 Numerical modelling
Compound flood events are often examined by numerically modelling the physics-based interactions of their processes and mechanisms. Through the simulation of historical and synthetic compound flood events, researchers can develop a better understanding of present and future inundation magnitude and extent. Given the highly complex nature of compound flooding, numerical modelling often requires a combination of hydrological, hydrodynamic, and atmospheric/climate models to represent all Earth system components contributing to compound flooding. A range of different numerical models are used in the literature, as we briefly discuss here. Further information on the hydrological, hydrodynamic, and atmospheric models; frameworks; systems; and toolsets used in the reviewed studies is provided in Table A2.
Hydrological models are used to simulate the movement, storage, and transformation of water within the hydrological cycle. These include land–atmosphere water exchange (precipitation and evapotranspiration), the flow of water through the landscape (streamflow and rainfall–runoff), and the infiltration of water into the ground (groundwater recharge). Hydrodynamic models use a series of governing equations (e.g. shallow-water equations) to simulate the flow of water in rivers, oceans, estuaries, and coastal areas. Coastal hydrodynamic models replicate the propagation and advection of water based on a combination of tide, surge, and waves. In the realm of compound flooding, hydrodynamic models are vital for simulating the effects of complex river–ocean interactions, storm surge, lake seiche, and flood infrastructure. Atmospheric models simulate various atmospheric processes based on primitive dynamic equations explaining radiation, convection, heat flux, gas exchange, kinematics of air masses, the behaviour of water vapour (precipitation and clouds), and land/ocean–atmosphere interactions. In compound flood research, numerical atmospheric modelling is generally used to simulate synthetic or historical storm events (TCs/ETCs) and to generate meteorological inputs (e.g. precipitation, atmospheric pressure, and wind velocity) that force hydrological and hydrodynamic models.
Compound flood modelling often involves the use of coupled or linked models. Individually, hydrological and hydrodynamic models are unable to capture the full dynamic interactions between inland and coastal processes (Ye et al., 2020). However, integrating the capabilities of both types of models can serve to better simulate the movement and transformation of water within a particular system as shortcomings of one model can be complemented by the strengths of another. Santiago-Collazo et al. (2019) define four techniques for linking different types of models: one-way coupled, two-way (or loosely) coupled, tightly coupled, and fully coupled. One-way coupling involves using the output of one model as the direct input for another model, such that data only transfer in one direction. Alternatively, two-way coupling describes a relationship in which the outputs of both models transfer information to each other iteratively, creating a two-way loop that influences the behaviour of both. Tight coupling refers to the integration of two independent models into a single model framework at the source code level. A common example of tight coupling is the ADCIRC–SWAN model. SWAN sends simulated waves to ADCIRC, and ADCIRC sends water levels and wind velocities back to SWAN. Lastly, full coupling is the complete integration of all model components such that physical processes are calculated simultaneously under the same framework using the same governing equations. We observed that most of the existing compound flood indentation modelling implements simple one-way or two-way coupling approaches (Santiago-Collazo et al., 2019; Xu et al., 2022). Fully coupled numerical models are rare in compound flood research, as most models only specialize in one or two earth systems (i.e. meteorology, climatology, hydrology, and oceanography).
6.5.2 Statistical approaches and dependence analysis
Across the studies we have reviewed, a wide variety of statistical-based approaches have been employed to understand trends, patterns, and relationships using observed data, sometimes complemented by physically simulated data. This predominantly involves the use of statistical models as an indirect measure of compound flooding potential to better understand the dependence between different flood drivers and the likelihood of their joint occurrence.
Several broad statistical techniques are frequently used for compound flood research. Some of the most prominent methods include varying forms of spatial and temporal analysis, regression analysis, extreme value analysis, Bayesian probability, principal component analysis, index analysis, Markov chains, and machine learning (ML). Spatial and temporal analysis investigate correlations, covariance, trends, and patterns in where and when compound flood events occur. This can include identifying compound flood hotspots (Ganguli and Merz, 2019a; Ridder et al., 2020; Camus et al., 2021; Lai et al., 2021b; Camus et al., 2022) and temporal clustering (Haigh et al., 2016; Santos et al., 2017; Camus et al., 2021; Banfi and De Michele, 2022; Manoj et al., 2022) or examining the underlying spatiotemporal preconditions and interactions of flood components (Camus et al., 2022; Manoj et al., 2022). Regression analysis involves using statistical functions to identify relationships between independent and dependent flood variables by fitting data to linear and higher-order non-linear functions (Zhong et al., 2013; Orton et al., 2015; Van Den Hurk et al., 2015; Serafin et al., 2019; Bermúdez et al., 2021; Ghanbari et al., 2021; Lai et al., 2021b; Meyers et al., 2021; Mohammadi et al., 2021; Robins et al., 2021; Santos et al., 2021b; Zhang et al., 2021b; Jang and Chang, 2022; Sampurno et al., 2022a). Extreme value analysis examines the tail distribution or threshold exceedances of extreme flood variables to better understand joint probability, uncertainty, and severity (Dixon and Tawn, 1994; Kew et al., 2013; Orton et al., 2016; Vitousek et al., 2017; Pasquier et al., 2019). Bayesian statistical approaches can iteratively recalculate the likelihood of an event based on new evidence. Bayesian frameworks are often used to update predictions about compound flood hazards based on new data and to understand the uncertainties associated with these hazards (Orton et al., 2015; Bass and Bedient, 2018; Couasnon et al., 2018; Bermúdez et al., 2021; Mohammadi et al., 2021; Steinschneider, 2021; Gori and Lin, 2022; Naseri and Hummel, 2022). Principal component analysis is a method of reducing the dimensionality of data by selecting the most important variables and combining them into a smaller volume of composite variables. In compound flood research this approach can be used to reduce the complexity of compound flood data to identify the key factors contributing to compound flood hazards (Camus et al., 2022). Index analysis is a method of data interpretation in which statistical indices simplify our understanding of the behaviour of multiple variables, a practice commonly used for flood risk and impact analysis (Rueda et al., 2016; Valle-Levinson et al., 2020; Tanir et al., 2021; Huang, 2022; Jalili Pirani and Najafi, 2022b; Juárez et al., 2022; Khatun et al., 2022; Preisser et al., 2022; Tao et al., 2022). Compound flood research takes this further using various indices that also consider the synergy of multiple flood drivers (Tanir et al., 2021; Jalili Pirani and Najafi, 2022a, b; Juárez et al., 2022; Khatun et al., 2022; Preisser et al., 2022; Tao et al., 2022). Markov chains use records of past variable states to describe the probability of future states. With this approach, flood variable data such as rainfall and river levels can be fit to stochastic models to simulate the probability of joint extreme states. Additionally, Markov chain Monte Carlo (MCMC) approaches involving stochastic sampling of variables are sometimes also applied in compound flood research (De Michele et al., 2020; Ganguli et al., 2020; Jalili Pirani and Najafi, 2022a; Jong-Levinger et al., 2022). Lastly, in recent years ML models involving varying neural network structures have been trained using compound flood datasets to predict flood extremes or map inundation extents (Karamouz et al., 2014; Bass and Bedient, 2018; Serafin et al., 2019; Muñoz et al., 2021; Santos et al., 2021b; Huang, 2022; Sampurno et al., 2022a).
Understanding the dependence of compound flood variables is crucial as it tells us about their joint exceedance probability (Ward et al., 2018; Xu et al., 2022). Failure to investigate driver dependence will lead to an underestimation of flood probabilities. Varying forms of the joint probability method (JPM) (Myers, 1970; Ho and Myers, 1975; Pugh and Vassie, 1980), involving aspects of extreme value analysis, are commonly used to measure potential co-occurrence and dependence between compound flood drivers. Over time the analytical approaches have evolved but generally involve three main steps for investigating dependence and frequency of co-occurring events. First, the flood variable event sets are sampled. The second step involves a simple calculation of varying correlation coefficients from the driver data. The third step consists of fitting a multivariate distribution function.
In preparation for the following steps, flood variable datasets are created by sampling events (according to varying compound scenarios, i.e. AND, OR, Kendall) via block-maxima or threshold-excess (peak-over-threshold, POT) methods. Block-maxima sampling selects the maximum events within a given temporal block (annual, seasonal, daily), while the threshold-excess method selects events above a defined extreme threshold value. Lucey and Gallien (2022) suggest that block-maxima sampling has the potential to underestimate water levels for extreme events (in semi-arid climates); however, both block-maxima and threshold-excess approaches likely have limitations depending on their implementation. Next, the correlation coefficient step typically implements different types of rank correlation coefficients and tail coefficients. Correlation coefficients such as Kendall's tau τ and Spearman's ρ can reveal non-linear relationships between random variables based on their ordinal associations. Alternatively, the lower (λL) and upper (λU) tail coefficients help examine dependence between random variables at the extremes of their distributions. While random variables may appear to show no correlation at a standard significance level, the co-movement of their tails may reveal dependence relationships that only occur at the extremes. The joint probability distribution is then constructed from the sampled variable event datasets as the probability of all possible pairs across each input variable. The joint probability distribution thus defines the probability of two or more simultaneous events, where the variables are at least partially dependent and thus influence each other's occurrence (Hawkes, 2008). Similarly, event coincidence analysis can be used to examine the joint occurrence of variables. This approach relies on variable time series (observed or modelled) and counts instances of coincidence, where two or more variables or events co-occur within a defined time window (Donges et al., 2016). Coincidence rate can then by calculated to assess the frequency of event coincidence over time.
In recent years, copulas have also been used to measure dependence, gaining considerable attention for their ability to simplify the analysis of highly stochastic multivariate processes. A total of 64 (23 %) studies were observed using copula-based methods to assess dependence. Defined in Sklar's theorem (Sklar, 1959), a copula is a multivariate cumulative distribution made by joining or “coupling” the univariate marginal probability distributions of two or more individual variables. This can be done using several dependence structures, with common copula families being elliptical and Archimedean. In addition to measuring dependence, copulas are used in compound flood research to assess the non-linear relationships and uncertainties between extreme flood variables (Salvadori and De Michele, 2004, 2007). By fitting copula functions to multivariate flood data, it is possible to understand the strength and nature of the dependence between these variables and to predict the likelihood of compound flood events. To date, the majority of compound flood research involves bivariate case studies. Nonetheless, several studies have implemented trivariate approaches to simultaneously analyse three partially dependent variables (Hawkes et al., 2002; Yang and Qian, 2019; Jalili Pirani and Najafi, 2020; Jane et al., 2020; Santos et al., 2021a; Jalili Pirani and Najafi, 2022b; Latif and Simonovic, 2022b, a; Ming et al., 2022; Zhang and Chen, 2022; Latif and Simonovic, 2023), and others have taken more complex procedures integrating copulas with MCMC (Sadegh et al., 2018; Moftakhari et al., 2019; De Michele et al., 2020; Ganguli et al., 2020) and Bayesian network (Couasnon et al., 2018; Moftakhari et al., 2019; Jalili Pirani and Najafi, 2022a; Naseri and Hummel, 2022) approaches. For further detail on copula-based multivariate flood analysis see Latif and Mustafa (2020).
6.5.3 Hybrid modelling and analysis approaches
Research methodologies involving a combined numerical and statistical approach were observed in around one-third of the compound flood studies (Fig. 6). In this review we use “hybrid modelling” to refer to this combined numerical (process-based) and statistical (data-driven) approach. We note that there is currently no standard meaning around the term “hybrid”, and thus our interpretation may conflict with the perspectives of others. Some use “hybrid” when considering the linking of multiple numerical modelling components or in the case of various ML statistical models. Others use this term in reference to model frameworks involving a combination of parametric and nonparametric components. Nonetheless, these hybrid modelling approaches can complement each other or focus on multiple aspects of modelling in a way that would not be possible when using numerical or statistical approaches in isolation. For example, process-based numerical modelling of compound flood hazards may be ideal for physics-based inundation mapping and floodplain delineation but can be very computationally expensive (this has pushed the development of more computationally efficient models such as SFINCS; Leijnse et al., 2021). Conversely, simplified statistical models are less computationally expensive but typically make general assumptions about input data that do not fully consider the physical processes at play. In contrast, hybrid numerical–statistical modelling approaches offer the benefit of computationally efficient surrogate statistical modelling while still maintaining a realistic representation of the physical processes (Serafin et al., 2019). Additionally, numerical modelling can also be severely inhibited by historical data availability. Hydrodynamic modelling of astronomical tide and storm surge require atmospheric pressure and wind velocity forcing data, while past river level and rainfall data are dependent on the presence of in situ tide and rain gauge monitors. In the event of absent of poor spatiotemporal coverage, numerical hydrodynamic models must rely on reanalysis datasets (i.e. assimilation of observations and numerical weather prediction models). Statistical approaches to compound flood analysis, however, can sometimes make do with limited data by interpolating or extrapolating extreme hazard probabilities and distributions. In the absence of historical data, one solution is to numerically simulate synthetic events that are physically capable of occurring, albeit not present in short-term observations (Serafin et al., 2019). For instance, Bloemendaal et al. (2020) demonstrate the synthetic resampling algorithm STORM's ability to generate 10 000 years of TC activity based on 38 years of historical data from IBTrACS. Many hybrid modelling approach compound flood studies statistically simulate storm events that drive physical hydrodynamic and hydrological models (Moftakhari et al., 2019; Serafin et al., 2019). Limitations of this approach centre on the fact that statistically generated event sets and reanalysis data may under-represent extremes, exhibit inherent systematic modelling, and/or inadequately account for climate nonstationarity (Bengtsson et al., 2004; Easterling et al., 2016; Brönnimann et al., 2019).
6.6 Research applications
As highlighted in Sect. 5.5, we identified that six main applications have been the focus of most compound flood studies in the database. Discussed in the following order, prominent case study applications include earth system processes (Sect. 6.6.1), risk assessment (Sect. 6.6.2), impact assessment (Sect. 6.6.3), forecasting (Sect. 6.6.4), planning and management (Sect. 6.6.5), and methodological advancement (Sect. 6.6.6). Note that many of the compound flood studies fall into multiple application categories.
6.6.1 Earth system processes
From the 279 literature database entries, 130 (47 %) seek to better understand the processes, interactions, and behaviour of earth systems associated with compound flooding. Research papers within the earth system processes application theme examine a variety of topics including the role of various dynamic Earth systems on compound flooding, the environmental and landscape characteristics influencing flood drivers, the relationships between and relative significance of flood drivers, and the spatiotemporal distributions and frequency of compound flood events. Many of the papers discussed in Sect. 6.1, 6.2, and 6.5 fall within this application category.
Focusing on flood drivers relationships, there is a plethora of research examining aspects of spatiotemporal distribution, correlation, covariance, dominance, and dependence structures as demonstrated in the US (Serafin and Ruggiero, 2014; Nasr et al., 2021; Juárez et al., 2022; Maymandi et al., 2022), UK (Svensson and Jones, 2002, 2004; Haigh et al., 2016; Santos et al., 2017; Hendry et al., 2019), Europe (Klerk et al., 2015; Petroliagkis, 2018; Ganguli and Merz, 2019b; Camus et al., 2021), Australia (Zheng et al., 2013; Zheng et al., 2014; Wu et al., 2018; Wu and Leonard, 2019), Canada (Jalili Pirani and Najafi, 2020, 2022b), China (Qiu et al., 2022; Tao et al., 2022; Zhang and Chen, 2022), South Africa (Kupfer et al., 2022), India (Manoj et al., 2022), Indonesia (Sampurno et al., 2022b), New Zealand (Stephens and Wu, 2022), and globally (Ward et al., 2018; Couasnon et al., 2020; Ridder et al., 2020; Lai et al., 2021a). Many have simulated or projected how climate change (e.g. SLR and storm intensification) is expected to affect the future compounding interactions of flood drivers (Wahl et al., 2015; Bevacqua et al., 2019; Pasquier et al., 2019; Ganguli et al., 2020; Bermúdez et al., 2021; Ghanbari et al., 2021).
There is also notable insight into the large-scale meteorological and climatological modulators and underlying earth systems influencing the nature of compound flooding and the behaviour of flood drivers. For instance, Camus et al. (2022), Hendry et al. (2019), and Rueda et al. (2016) identify the meteorological conditions associated with the compound occurrence of extreme flood drivers in the North Atlantic, the UK, and Spain, respectively. Gori et al. (2020a) and Gori et al. (2020b) determine the type of TC events likely to cause compound pluvial–coastal flooding in North Carolina. Stephens and Wu (2022) identify the weather types corresponding with both univariate and coincident pluvial, fluvial, and coastal extremes in New Zealand. Furthermore, Wu and Leonard (2019) demonstrate how ENSO climate forcings impact the dependence between rainfall and storm surge extremes.
Other common focuses of earth-system-processes-themed literature include characterizing the physical mechanics and environmental properties that shape how flood drivers interact. Several papers including Vongvisessomjai and Rojanakamthorn (1989), Poulos et al. (2022), and Pietrafesa et al. (2019) evaluate the timing and mechanisms behind downstream blocking and dampening that often explain fluvial–coastal flooding. Similarly, Maymandi et al. (2022) measure the timing, extent, and intensity of storm surge, river discharge, and rainfall components to understand their relative importance. Likewise, Tanim and Goharian (2021) observe how changes in tidal phase alter the depth and duration of urban compound pluvial–coastal flooding. Harrison et al. (2022) and Helaire et al. (2020) measure how estuary characteristics (e.g. shape, size, width) influence fluvial–coastal dynamics. Wolf (2009) considers how wind stress, bottom friction, depth, bathymetry, and ocean current refraction change co-occurring surge and wave extremes (coastal–coastal). Torres et al. (2015) and Gori et al. (2020b) examine the influence of hurricane landfall location, angle of approach, and forward speed on compound rainfall–runoff and storm surge flooding (pluvial–coastal). Tao et al. (2022) explore compound fluvial–pluvial flood scenarios involving upstream and downstream water levels, as well as how intensity, timing, duration, and dependence change based on synoptic and topographic conditions.
Lastly, while the occurrence of compound flooding is well recognized in coastal, estuarine, and delta environments, we note that emerging research has enhanced the understanding of compound flood processes in the context of coastal lake environments (Saharia et al., 2021; Steinschneider, 2021; Banfi and De Michele, 2022; Jalili Pirani and Najafi, 2022b). For example, Banfi and De Michele (2022) determine that flooding of Italy's Lake Como is primarily (70 %) from temporal compounding of rainfall (pluvial–pluvial). In Lake Erie, Saharia et al. (2021) analyses compound flooding involving river flow and lake seiche (fluvial–coastal), showing for the first time how seiches can combine with hydrological processes to exacerbate flooding. Finally, along Lake Ontario, Steinschneider (2021) quantified the compounding nature and variability of storm surge and total water level (coastal–coastal).
6.6.2 Risk assessment
The overarching goal of most compound flood research is to better understand risk, hence why 129 (46 %) studies involve aspects of risk assessment. As defined by the UNDRR (2016), risk assessment is an approach for determining the state of risk posed by a potential hazard taking into account conditions of exposure and vulnerability. Risk assessment inherently plays a key role in several of the reviews' other research application categories including hazard planning and management as well as impact assessment.
As the field of compound event sciences advances, it has become increasingly clear that conventional univariate analysis cannot accurately capture the synergistic and non-linear risk of compound processes (Kappes et al., 2010; Leonard et al., 2014; Eshrati et al., 2015; Zscheischler and Seneviratne, 2017; Sadegh et al., 2018; Zscheischler et al., 2018; Ridder et al., 2020). A plethora of studies have concluded that traditional hazard analysis, in which flood variable dependence and synergy are not considered, underestimates the risk of compound extremes (Bevacqua et al., 2017; Bilskie and Hagen, 2018; Kumbier et al., 2018; Hendry et al., 2019; Huang et al., 2021; Eilander et al., 2022). Jang and Chang (2022) determine that by not considering the multivariate nature of pluvial–coastal flooding, Taiwan's flood risk would be severely misestimated, causing incorrect warning alarms and inadequate protection. Khalil et al. (2022) assert that failing to consider the interactions of multiple flood drivers would reduce flood levels by 0.62 and 0.12 m in Jindalee and Brisbane. Similarly, Santos et al. (2021a) measured 15–35 cm higher water levels for 1 % annual exceedance probability events when considering dependence for trivariate fluvial–pluvial–coastal flooding in Sabine Lake, Texas.
There is a diversity of topics within the risk-themed compound flood literature, but many papers involve simple regional case studies or framework proposals (Najafi et al., 2021; Ming et al., 2022; Naseri and Hummel, 2022; Peña et al., 2022). Čepienė et al. (2022) examine the risk associated with combined fluvial–coastal flooding and how it will change with SLR at the port city of Klaipėda. Bischiniotis et al. (2018) assess the influence of antecedent soil moisture on flood risk in sub-Saharan Africa, showing that precipitation alone cannot explain flood occurrence. Along the coasts of Mozambique, Eilander et al. (2023) demonstrate a globally applicable compound flood risk framework, and Van Berchum et al. (2020) present the novel Flood Risk Reduction Evaluation and Screening (FLORES) model. Bass and Bedient (2018) create joint pluvial–coastal flooding probabilistic risk models built upon TC risk products in Texas. Lastly, a few studies examine the risk of potential loss of life (PLL), such as de Bruijn et al. (2014), who present a Monte Carlo-based analysis framework for fluvial–coastal interactions in the Rhine–Meuse delta.
6.6.3 Impact assessment
Impact assessment is the least common compound flood application with only 11 (4 %) relevant studies. This may be because flood impact assessments have historically only been designed to address a single type of flooding at a time (Láng-Ritter et al., 2022). Additionally, flood loss modelling has largely targeted riverine floods, with less attention given to pluvial, coastal, or groundwater drivers. This is slowly changing, and in recent years a small portion of research has been dedicated to analysing the impacts of compound flood events (Habel et al., 2020; Tanir et al., 2021; Láng-Ritter et al., 2022; Preisser et al., 2022). Impact assessment differs from risk assessment in that it looks at the realized or impending outcomes of flood events rather than simply the event likelihood as a product of exposure and vulnerability. This involves identifying and analysing the physical (e.g. building and infrastructure damage), social (e.g. loss of essential services, household displacement, and community cohesion), and economic (e.g. loss of income, damage to business and industry, and disruption of transportation and supply chain) impacts of flooding.
Physical parameters for quantifying the empirical impact of flooding in an affected area can include water depth, flow velocity, inundation duration, water quality (contamination), land use/land cover change, and infrastructure damage. For example, Habel et al. (2020) look at the influence of compound floods and SLR on urban infrastructure and identify the roadways, drainage inlets, and cesspools that would fail under compound extreme conditions.
Social and economic flood impacts are routinely measured using multifaceted indices and damage models. Preisser et al. (2022) and Tanir et al. (2021) assess the impacts of compound flooding with SVI (social vulnerability index; 42 variables) and SOVI (socio-economic vulnerability index; 41 variables), respectively. Karamouz et al. (2017) apply a flood damage estimator (FDE) model to quantify pluvial–coastal flood damages to buildings structures in New York City. Similarly, Ming et al. (2022) calculate the average annual loss in value of residential buildings in the Thames River catchment from compound high river flow, heavy rainfall, and extreme surge.
6.6.4 Forecasting
A total of compound flood studies in the database focus on flood forecasting. Flood forecasts are valuable emergency management tools that provide information on the location, timing, magnitude, and potential impact of impending flood scenarios (Merz et al., 2020). Together with monitoring and prediction, forecasts guide time-sensitive early warning systems and disaster reduction strategies to help communities prepare for and respond to flooding. As compound-event-based perspectives gain traction, there has been emerging development of flood forecast models that consider the compound interaction of multiple drivers.
Several studies demonstrate the capabilities of integrated near-real-time observation-based hydrological river and hydrodynamic coastal flood models forced by already established meteorological forecasting systems (Stamey et al., 2007; Mashriqui et al., 2010; Park et al., 2011; Blanton et al., 2012; Dresback et al., 2013; Mashriqui et al., 2014; Blanton et al., 2018; Tehranirad et al., 2020; Cifelli et al., 2021). For instance, the fluvial–coastal flood forecasting system Hydro-CoSMoS detailed in Tehranirad et al. (2020) can predict tidal river interactions in San Francisco Bay. Over the Korean Peninsula, Park et al. (2011) design a model for real-time water level forecasting of pluvial–coastal inundation such as seen during Typhoon Maemi.
Much of the existing compound flood forecasting research has focused on advances in the development of monitoring and early warning systems for the US East Coast and Gulf of Mexico. Blanton et al. (2012) feature development of the North Carolina Forecasting System (NCFS), which predicts fluvial–pluvial–coastal flood variables. Van Cooten et al. (2011) showcase the Coastal and Inland Flooding Observation and Warning Project's (CI-FLOW) 7 d total water level forecasts and potential for near-real-time fluvial–pluvial–coastal flood prediction. Dresback et al. (2013) develop the coupled hydrological–hydrodynamic model ASGS-STORM for forecasting joint fluvial–coastal inundation. Multiple studies also concentrate on flood forecasting in the Chesapeake Bay and the tidally influenced Potomac River. Stamey et al. (2007) introduce the Chesapeake Bay Inundation Prediction System (CIPS), a prototype operational flood forecasting system for TC/ETC storm-induced fluvial–coastal flooding. This is followed by Mashriqui et al. (2010) and Mashriqui et al. (2014), who build a river–estuary–ocean (REO) forecast system to fill gaps in existing operational models.
Accurate forecast products are crucial to effective emergency management practices and reliable early warning systems. Ensemble modelling has been implemented in two compound forecasting studies as a means of minimizing uncertainty. Blanton et al. (2018) develop a hurricane ensemble hazard prediction framework and demonstrate the ability to forecast pluvial–coastal flooding with a 7 d lead simulation of Hurricane Isabel. Similarly, Saleh et al. (2017) showcase a 4 d advance operational ensemble forecasting framework for fluvial–coastal flooding in Newark Bay during Hurricane Irene and Hurricane Sandy.
A small number of studies have also investigated the use case of ML for forecasting compound flooding (Bass and Bedient, 2018; Huang, 2022; Sampurno et al., 2022a). For instance, Sampurno et al. (2022a) use a combined hydrodynamic and ML approach to forecast fluvial–pluvial–coastal flooding in Indonesia's Kapuas River delta. Bass and Bedient (2018) take peak inundation levels from a coupled hydrological–hydrodynamic model's results to train an artificial neural network (ANN) and Kriging ML model for rapid forecasting of TC-driven pluvial–coastal extremes in Houston, Texas, as a result of Hurricane Allison and Hurricane Ike. Finally, Huang (2022) constructs a recurrent neural network (RNN) model that considers downstream geomorphological and hydrological characteristics to predict joint pluvial–coastal flooding in Taiwan.
6.6.5 Planning and management
Within the literature database there are 28 (10 %) papers that focus on different aspects of flood management and planning from emergency response to risk mitigation strategies. The UNDRR (2016) defines disaster management as the organization, planning, and application of measures for disaster response and recovery. Subsequently, disaster risk management is described as the use of disaster risk reduction strategies and policies to prevent, reduce, and manage risk (UNDRR, 2016). Flood management strategies might involve identifying areas for prioritized flood protection and building risk reduction structures such as building levees, dykes, barriers, and sea walls or enacting changes in land use planning and zoning policy to minimize habitation and activity in floodplains.
Flood defence and water management structures have long been in use; however, these features have predominantly been designed for responding to a single flood driver (e.g. storm surge) (Sebastian, 2022). Several studies examine the effectiveness of flood defence structures protecting against compound events. Christian et al. (2015) investigate the feasibility of a proposed storm surge barrier for mitigating pluvial–coastal flooding in the Houston Ship Channel. Findings on the magnitude of reductions in surface height and floodplain area help guide project development decision-making by coastal and port authorities. Del-Rosal-Salido et al. (2021) develop management maps to support decision-making and long-term climate and SLR adaptation planning in Spain's Guadalete Estuary, identifying sites for potential flood barriers.
During extreme flood events, unpredictable impacts on utility and transportation infrastructure can exacerbate loss. Thus, another key component of flood management is flexible emergency response planning. Several articles address these elements of response planning and identify evacuation areas, routes, and emergency shelters in the event of compound flooding. In their analysis of urban infrastructure failure from compound flooding in Hawaii, Habel et al. (2020) locate road networks and urban spaces that are likely to be impassable and estimate the effects of traffic on resident evacuation. In the event of a typhoon landfall in the Korean Peninsula, Park et al. (2011) design an early warning system for pluvial–coastal flooding that supports decision-making and response from local officials by identifying areas to evacuate. Blanton et al. (2018) also address emergency planning, developing a hurricane-driven inundation evacuation model that dynamically accounts for interactions of compound drivers.
Effective communication and outreach are additional critical components of flood hazard planning and mitigation. This includes educating the public about the types and considerations of flooding; collaborating with hazard managers and policy makers to address challenges in flood management; and timely dissemination of information on flood risk, evacuation routes, and emergency shelters. In a unique narrative paper, Curtis et al. (2022) interview emergency managers and planners on compound flood risk perceptions and challenges in North Carolina, revealing inadequacies in communication mediums and the ability to convey compound flood severity to the public. Similarly, hazard expert interviews in Modrakowski et al. (2022) centre on the use of precautionary risk management strategies in the Netherlands and examine how the perception of compound flood events in part shapes the flood management practices of local authorities. Interestingly, the two studies produce different findings for individual flood drivers, highlighting the regional differences in flood mechanisms. Curtis et al. (2022) recorded a greater perception of risk from fluvial- and coastal-dominant flooding as opposed to pluvial inundation. Conversely, Modrakowski et al. (2022) found that pluvial flooding (specifically heavy rainfall from cloudbursts) had a larger perceived risk, being equal if not greater than fluvial and coastal. Societal intuitions, beliefs, and attitudes surrounding hazards are not often considered, yet they can provide substantial value for shaping the strategies and practices for more effective emergency response and risk reduction.
6.6.6 Methodological advancement
The third most common application category is methodological advancement with 80 (29 %) of the 279 studies aimed at testing and developing methodologies for research on compound floods. Methodological advancement is a broad application category but most often describes research studies that investigate either (i) new setups and frameworks for running numerical model simulations or (ii) novel statistical modelling and analysis techniques for quantifying the likelihood of compounding extremes or behaviour of interacting drivers. Papers classified as methodological advancement seek to better understand and showcase the feasibility, development, and/or performance of compound flood research methods. See Table A2 for full model names and descriptions.
In relation to advancements in numerical-based methodologies, many papers explicitly state their primary research objective is the development of a compound flood modelling system itself, such as Chen and Liu (2014) and Lee et al. (2019), who test whether their respective SELFE and HEC-HMS + Delft3D-FLOW model frameworks can sufficiently replicate the fluvial–coastal flood conditions observed during historical storm events. Bates et al. (2021) showcase a sophisticated 30 m resolution large-scale LISFLOOD-FP model of the contiguous US that incorporates pluvial, fluvial, and coastal processes under the same methodological framework. Numerous papers focus on assessing the performance of specific computational software applications for simulating compound flooding. These primarily seek to provide insight for future development and use case applications. For instance, Bush et al. (2022) examine the benefits and drawbacks between ADCIRC and linked ADCIRC + HEC-RAS simulations of fluvial–coastal flooding. Bilskie et al. (2021) demonstrate a new approach for delineating coastal floodplains and simulating water levels using ADCIRC's rain-on-mesh modules forced by antecedent rainfall, TC-driven rainfall, and storm surge. Ye et al. (2020) use SCHISM to develop a 3D model that incorporates the baroclinic effects of storm surge and compare its performance against 3D barotropic and 2D model alternatives. Numerous studies incorporate sensitivity assessments, experimenting with model parameters and settings, as well as examining how they influence performance and uncertainty (McInnes et al., 2002; Brown et al., 2007; Orton et al., 2012; Olbert et al., 2017; Silva-Araya et al., 2018; Leijnse et al., 2021; Khalil et al., 2022; Lyddon et al., 2022). For example, Khalil et al. (2022) investigate how model mesh resolution affects flood discharge rates, revealing that finer meshes best replicate peak flows. Some studies introduce newly developed numerical models, such as Olbert et al. (2017), who present the first instance of a dynamically linked and nested POM + MSN_Flood framework for fluvial–pluvial–coastal flooding. Others focus on the computational efficiency of compound flood frameworks, such as Leijnse et al. (2021), who assess the reduced physical solver SFINCS's ability to accurately simulate fluvial–pluvial–coastal interactions with less computational resources.
Many of the literature database studies showcase innovations in statistical approaches to compound flood research. Sampurno et al. (2022a) assess the operational viability and performance of three ML algorithms for a compound flood forecasting system. Similarly, Muñoz et al. (2021) examine the capability of ML and data fusion-based approaches for post-event mapping of compound floods from satellite imagery. Muñoz et al. (2022a) demonstrate techniques for employing data assimilation to reduce uncertainty in compound flood modelling. Wu et al. (2021) experiment with three methods of compound flood frequency analysis and discuss the advantages and disadvantages of each approach. Phillips et al. (2022) examine combinations of varying copula structures and statistical fitting frameworks to further approaches for measuring driver dependence. Thompson and Frazier (2014) test out different means of deterministic and probabilistic modelling for quantifying compound flood risk. Lastly, some studies expand on existing methodologies to overcome known limitations, such as Gouldby et al. (2017), who develop a method of full multivariate probability analysis that overcomes drawbacks of the prevalent joint probability contour (JPC) method by directly quantifying response variable extremes.
Our final objective is to reflect on the knowledge gaps in compound flood research and suggest potential directions for research going forward. Based on our detailed review we have five main recommendations moving forward, as follows.
Recommendation 1 – adopt consistent definitions, terminology, and approaches. Definitions and use cases of compound event, compound hazard, multi-hazard, and associated terminology (Table 1) are highly inconsistent throughout the literature (Kappes et al., 2012; Gallina et al., 2016; Tilloy et al., 2019). This is well recognized by Tilloy et al. (2019), who refer to the variety of terms as a “fragmentation of [the] literature”. Similarly, Pescaroli and Alexander (2018) draw attention to trends in the “superficial” and “ambiguous” use of hazard terms by academics and practitioners. This tendency to use differing concepts synonymously is blurring the state of compound flood research (something we continuously observed while completing this review). They warn of potential confusion and duplication of research as a result of overlapping definitions. In summary, compound event and related terms have a wide range of overlapping and interlinked definitions, and there is a considerable need for clarity. Recent preliminary efforts by the collaborative MYRIAD-EU project to develop a multi-hazard and multi-risk definitions handbook appear promising for fostering a common understanding of hazard concepts across disciplines (Gill et al., 2020). Similarly, there is early collaborative work on the development of a compound flood practices manual and primer document as part of the ASCE-MOP project (Shields et al., 2023).
Recommendation 2 – expand the geographic coverage of research. Geographically, much of the existing compound flood research is too narrowly focused on a select few regions (i.e. North America, Europe, Southeast Asia, the UK, China, the Netherlands, Australia) (Fig. 3b). To date, there are no localized English-language studies, to our knowledge, on compound flooding in any parts of South America, Central America, or the Middle East. South America regularly experiences catastrophic flooding from both long-term heavy rainfall and extreme river discharge (e.g. 2015/16 (ReliefWeb, 2016) and 2016/17 (ReliefWeb, 2017) South American floods); however, existing research in these regions has not considered their combined interactions. Furthermore, there are very few compound flood papers within the African subcontinent (Bischiniotis et al., 2018; De Michele et al., 2020; Van Berchum et al., 2020; Kupfer et al., 2022) (a region deserving of greater attention given the projected extreme coastal hazard exposure as a result of SLR, population growth, and coastal urbanization; Neumann et al., 2015) due to a lack of data. While there are a handful of global studies, localized research on the interactions and dependence of flood variables is missing for many parts of the world. Future compound flood research must be dedicated to improving our understanding of these neglected regions, including strategic data collection and developing methodologies for assessing compound flooding in data-sparse areas.
Recommendation 3 – pursue more inter-comparison and collaborative compound flood projects. Current methodologies for analysing compound flooding are highly diverse, inhibiting quantitative comparisons between studies. Considerable subjectivity is observed in compound event mechanism and variable selection, temporal and spatial bounds, hazard scenario design, conditional and joint probability, and dependence measurement (Zscheischler et al., 2020). Standard approaches for compound flood risk analysis have yet to be established (Kappes et al., 2012; Sebastian, 2022). Furthermore, methods for analysing compound events vary across scientific communities (Pietrafesa et al., 2019; Tilloy et al., 2019). Discussions involving emergency managers and stakeholders have revealed the leading barrier to the use of multi-hazard and multi-risk approaches was a lack of common methodologies and data (Komendantova et al., 2014). Further highlighting this point, Tilloy et al. (2019) identified a staggering 79 unique uses of 19 different methods for analysing compound events. There is a substantial need for a standardized framework that addresses assorted analytical methods and considerations (Sebastian, 2022), including flood variable choice and pairing, flood threshold definition, case study hazard design, spatiotemporal scales and resolutions, statistical model assumptions, numerical parameter choice, and interpretation of results. Future water management practices and coastal hazard mitigation strategies must better reflect the perspectives of compound events. To aid this we would recommend that the community develop educational training resources to guide the next generation of compound flood researchers. Furthermore, we suggest creating a compound flood inter-comparison project and associated working group, similar to that set up for the wave and coastal modelling communities (e.g. COWCLIP, Hemer et al., 2010, and CoastMIP, Hinkel et al., 2014) and hydrological forecasting communities (e.g. HEPEX, Schaake et al., 2007).
Recommendation 4 – develop modelling frameworks that holistically represent dynamic earth systems. While there have been substantial advancements in compound flood research over the past decade, the overall ability to identify, model, quantify, and forecast compound flood events remains a substantial challenge. These difficulties stem from the highly complex and chaotic nature of hydrological, meteorological, and oceanographic systems (Sebastian, 2022). Connections between flood modulators and drivers are spatiotemporally dynamic, and how those relationships are affected by the changing climate is uncertain and ever-changing. Stand-alone numerical models generally lack the ability to holistically simulate the dynamic interconnected systems necessary to explain compound flooding (especially in the coastal setting). The skill of compound flood forecasting systems and numerical models has improved but still largely remains inadequate (Mashriqui et al., 2014; Pietrafesa et al., 2019). Going forward, we recommend the adoption of standardized modelling interfaces (e.g. Basic Model Interface; Hutton et al., 2020) to facilitate coupling between numerical models to develop holistic modelling frameworks that better disentangle the complex earth system processes driving compound floods. We additionally suggest the development of ensemble forecast systems with ocean–land–atmosphere coupling and compound flood modelling in mind (e.g. Saleh et al., 2017; Blanton et al., 2018). Compound flood research also serves to greatly benefit from the use of hybrid modelling frameworks that couple numerical and statistical models. While this review discovered many studies that employed hybrid numerical–statistical modelling methods, few explicitly outlined a standardized frameworks for linking the models. Thus, we additionally recommend further evaluation of hybrid frameworks as the linking of statistical and numerical models has considerable room for improvement.
Recommendation 5 – plan, design, and manage urban and coastal infrastructure with compound flooding in mind. We advise reshaping the planning, design, and operation of urban and coastal infrastructure to fully recognize the dependence and synergetic extremes of interacting flood drivers. As we look to a future of increasing flood frequency, proactive flood management is vital to lowering the vulnerability and exposure of urban and coastal communities. This can include investing in long-term resilient infrastructure (i.e. > 100-year extremes), developing flood hazard maps that consider compound flood return periods to aid planning (e.g. update FEMA hazard maps), supporting development blue-green and natural flood management (e.g. wetland protection, riverbank restoration, and leaky dams), enacting operational early warning systems (e.g. coupled ensemble forecast systems, Saleh et al., 2017) and emergency response measures, and educating the public about the risks of inhabiting coastal floodplains.
We have long known that high-impact hazard events involve a combination of drivers; however, existing research has largely been limited to single-factor or univariate analysis of climate extremes due to technical or methodological constraints. Such is the case with flooding, as standard flood hazard assessment practices have traditionally accounted for the effects of the different drivers of flooding independently. Only in recent years has flood research more closely examined the non-linear combination of these variables through the lens of compound events.
This paper has presented a systematic review of the existing literature on compound flooding in coastal regions. Analysis of 279 studies up to and including the year 2022 has revealed significantly increasing attention to compound flood research in recent years. This review identified different definitions and terminologies of compound flood events, categories of compound flood drivers, numerical modelling frameworks, and statistical analysis techniques. Furthermore, several compound flood hotspots have been identified throughout the world, including the US East Coast and Gulf of Mexico, northern Europe, East Asia, southern Asia, Southeast Asia, northern Australia, and global low-lying deltas and estuaries. Research has shown that compound floods are likely to have increasing frequency and severity in the future as a result of climate change and that societal risks of extreme climate hazards are underestimated when the compound effects of climatic processes are not considered in combination. Compound flood research thus requires a more holistic and integrated approach to risk analysis that reflects on the complex interactions and nonstationarity of Earth systems. We must recognize the threats posed by the interactions between hazard drivers for accurate risk assessment. Further research must also focus on identifying the dominant drivers of flooding, the precursors that make certain regions particularly susceptible to compound flooding, and the dependence relationships between flood drivers, as well as investigate how all these aspects change spatiotemporally. Going forward, an improved understanding of compound flooding processes and precursors is vital to coastal management, hazard risk reduction, and community resilience in the face of changing climates.
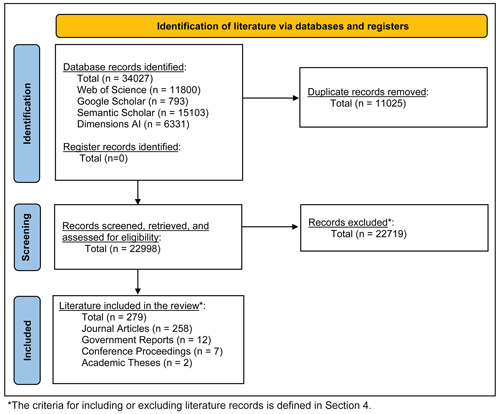
Figure A1PRISMA 2020 flow diagram (Page et al., 2021) visualizing the review's literature database curation process. This includes the number of papers identified in literature databases using the search terms in Table 2, removed due to being a duplicate, screened against scoping criteria as outlined in Sect. 4, excluded from consideration, and included in the review analysis.
Table A1Overview of the literature database containing 279 compound flood research publications. Note that numerical models without defined names are given simple descriptions. Statistical methods are defined as explicitly stated in the literature and then simplified for brevity.

The full contents of the compound flood literature database developed in this review can be accessed at https://doi.org/10.5281/zenodo.14274658 (Green, 2024).
JG: conceptualization, writing (original draft preparation), writing (review and editing), data curation, methodology, and formal analysis. IDH: conceptualization and writing (review and editing). NQ: writing (review and editing). JN: writing (review and editing). TW: writing (review and editing). MW: writing (review and editing). DE: writing (review and editing). MdR: writing (review and editing). PW: writing (review and editing). PC: writing (review and editing).
At least one of the (co-)authors is a member of the editorial board of Natural Hazards and Earth System Sciences. The peer-review process was guided by an independent editor, and the authors also have no other competing interests to declare.
Publisher’s note: Copernicus Publications remains neutral with regard to jurisdictional claims made in the text, published maps, institutional affiliations, or any other geographical representation in this paper. While Copernicus Publications makes every effort to include appropriate place names, the final responsibility lies with the authors.
We thank Kate Davis for the creation of the schematic diagram (Fig. 1).
Joshua Green time was supported by the UKRI Natural Environment Research Council (NERC) (grant no. NE/S007210/1). Ivan D. Haigh time was supported by NERC (grant no. NE/S010262/1). Jeff Neal time was supported by NERC (grant no. NE/S015639/1). Thomas Wahl was supported by the National Science Foundation (grant nos. 1929382 and 2103754) and the USACE Climate Preparedness and Resilience Community of Practice and Programs. Philip Ward and Marleen de Ruiter received support from the MYRIAD-EU project, which received funding from the European Union's Horizon 2020 research and innovation programme (grant agreement no. 101003276). Marleen de Ruiter also received support from the Netherlands Organisation for Scientific Research (NWO) (VENI; grant no. VI.Veni.222.169). Paula Camus recognizes funding from the MyFlood project (PLEC2022-009362 – MCIN/AEI/10.13039/501100011033 and European Union Next GenerationEU/PRTR).
This paper was edited by Kai Schröter and reviewed by Hamed Moftakhari and one anonymous referee.
Abbaszadeh, P., Muñoz, D. F., Moftakhari, H., Jafarzadegan, K., and Moradkhani, H.: Perspective on uncertainty quantification and reduction in compound flood modeling and forecasting, iScience, 25, 105201, https://doi.org/10.1016/j.isci.2022.105201, 2022.
Acreman, M. C.: Assessing the Joint Probability of Fluvial and Tidal Floods in the River Roding, Water Environ. J., 8, 490–496, https://doi.org/10.1111/j.1747-6593.1994.tb01140.x, 1994.
Adhikari, P., Hong, Y., Douglas, K. R., Kirschbaum, D. B., Gourley, J., Adler, R., and Brakenridge, G. R.: A digitized global flood inventory (1998–2008): Compilation and preliminary results, Natural Hazards, 55, 405-422, https://doi.org/10.1007/S11069-010-9537-2, 2010.
AghaKouchak, A., Chiang, F., Huning, L. S., Love, C. A., Mallakpour, I., Mazdiyasni, O., Moftakhari, H., Papalexiou, S. M., Ragno, E., and Sadegh, M.: Climate Extremes and Compound Hazards in a Warming World, Annu. Rev. Earth Pl. Sc., 48, 519–548, https://doi.org/10.1146/annurev-earth-071719-055228, 2020.
Ai, P., Yuan, D., and Xiong, C.: Copula-based joint probability analysis of compound floods from rainstorm and typhoon surge: A case study of Jiangsu Coastal Areas, China, Sustainability, 10, 2232, https://doi.org/10.3390/SU10072232, 2018.
Apel, H., Martínez Trepat, O., Hung, N. N., Chinh, D. T., Merz, B., and Dung, N. V.: Combined fluvial and pluvial urban flood hazard analysis: concept development and application to Can Tho city, Mekong Delta, Vietnam, Nat. Hazards Earth Syst. Sci., 16, 941–961, https://doi.org/10.5194/nhess-16-941-2016, 2016.
Archetti, R., Bolognesi, A., Casadio, A., and Maglionico, M.: Development of flood probability charts for urban drainage network in coastal areas through a simplified joint assessment approach, Hydrol. Earth Syst. Sci., 15, 3115–3122, https://doi.org/10.5194/hess-15-3115-2011, 2011.
Bacopoulos, P., Tang, Y., Asce, S. M., Wang, D., Asce, A. M., Hagen, S. C., and Asce, F.: Integrated Hydrologic-Hydrodynamic Modeling of Estuarine-Riverine Flooding: 2008 Tropical Storm Fay, J. Hydrol. Eng., 22, 04017022, https://doi.org/10.1061/(ASCE)HE.1943-5584.0001539, 2017.
Bakhtyar, R., Maitaria, K., Velissariou, P., Trimble, B., Mashriqui, H., Moghimi, S., Abdolali, A., Van der Westhuysen, A. J., Ma, Z., Clark, E. P., and Flowers, T.: A New 1D/2D Coupled Modeling Approach for a Riverine-Estuarine System Under Storm Events: Application to Delaware River Basin, J. Geophys. Res.-Oceans, 125, e2019JC015822, https://doi.org/10.1029/2019JC015822, 2020.
Ballesteros, C., Jiménez, J. A., and Viavattene, C.: A multi-component flood risk assessment in the Maresme coast (NW Mediterranean), Nat. Hazards, 90, 265–292, https://doi.org/10.1007/s11069-017-3042-9, 2018.
Banfi, F. and De Michele, C.: Compound flood hazard at Lake Como, Italy, is driven by temporal clustering of rainfall events, Commun. Earth Environ., 3, 234, https://doi.org/10.1038/s43247-022-00557-9, 2022.
Bao, D., Xue, Z. G., Warner, J. C., Moulton, M., Yin, D., Hegermiller, C. A., Zambon, J. B., and He, R.: A Numerical Investigation of Hurricane Florence-Induced Compound Flooding in the Cape Fear Estuary Using a Dynamically Coupled Hydrological-Ocean Model, J. Adv. Model. Earth Sy., 14, e2022MS003131, https://doi.org/10.1029/2022MS003131, 2022.
Bass, B. and Bedient, P.: Surrogate modeling of joint flood risk across coastal watersheds, J. Hydrol., 558, 159–173, https://doi.org/10.1016/j.jhydrol.2018.01.014, 2018.
Bates, P. D., Quinn, N., Sampson, C., Smith, A., Wing, O., Sosa, J., Savage, J., Olcese, G., Neal, J., Schumann, G., Giustarini, L., Coxon, G., Porter, J. R., Amodeo, M. F., Chu, Z., Lewis-Gruss, S., Freeman, N. B., Houser, T., Delgado, M., Hamidi, A., Bolliger, I., E. McCusker, K., Emanuel, K., Ferreira, C. M., Khalid, A., Haigh, I. D., Couasnon, A., E. Kopp, R., Hsiang, S., and Krajewski, W. F.: Combined Modeling of US Fluvial, Pluvial, and Coastal Flood Hazard Under Current and Future Climates, Water Resour. Res., 57, https://doi.org/10.1029/2020WR028673, 2021.
Baxter, R. M.: Environmental Effects of Dams and Impoundments, Annu. Rev. Ecol. Syst., 8, 255–283, 1977.
Bayazýt, Y. and Koç, C.: The impact of forest fires on floods and erosion: Marmaris, Turkey, Environ. Dev. Sustain., 24, 13426–13445, https://doi.org/10.1007/s10668-022-02624-9, 2022.
Beardsley, R. C., Chen, C., and Xu, Q.: Coastal flooding in Scituate (MA): A FVCOM study of the 27 December 2010 nor'easter, J. Geophys. Res.-Oceans, 118, 6030–6045, https://doi.org/10.1002/2013JC008862, 2013.
Befus, K. M., Barnard, P. L., Hoover, D. J., Finzi Hart, J. A., and Voss, C. I.: Increasing threat of coastal groundwater hazards from sea-level rise in California, Nat. Clim. Change, 10, 946–952, https://doi.org/10.1038/s41558-020-0874-1, 2020.
Belongia, M. F., Hammond Wagner, C., Seipp, K. Q., and Ajami, N. K.: Building water resilience in the face of cascading wildfire risks, Science Advances, 9, eadf9534, https://doi.org/10.1126/sciadv.adf9534, 2023.
Bender, J., Wahl, T., Müller, A., and Jensen, J.: A multivariate design framework for river confluences, Hydrolog. Sci. J., 61, 471–482, https://doi.org/10.1080/02626667.2015.1052816, 2016.
Benestad, R. E. and Haugen, J. E.: On complex extremes: Flood hazards and combined high spring-time precipitation and temperature in Norway, Climatic Change, 85, 381–406, https://doi.org/10.1007/S10584-007-9263-2, 2007.
Bengtsson, L., Hagemann, S., and Hodges, K. I.: Can climate trends be calculated from reanalysis data?, J. Geophys. Res.-Atmos., 109, D11111, https://doi.org/10.1029/2004JD004536, 2004.
Bensi, M., Mohammadi, S., Kao, S.-C., and DeNeale, S. T.: Multi-Mechanism Flood Hazard Assessment: Critical Review of Current Practice and Approaches, Oak Ridge National Lab, United States, https://doi.org/10.2172/1637939, 2020.
Berghuijs, W. R., Harrigan, S., Molnar, P., Slater, L. J., and Kirchner, J. W.: The Relative Importance of Different Flood-Generating Mechanisms Across Europe, Water Resour. Res., 55, 4582–4593, https://doi.org/10.1029/2019WR024841, 2019.
Bermúdez, M., Cea, L., and Sopelana, J.: Quantifying the role of individual flood drivers and their correlations in flooding of coastal river reaches, Stoch. Environ. Res. Risk A., 33, 1851–1861, https://doi.org/10.1007/S00477-019-01733-8, 2019.
Bermúdez, M., Farfán, J. F., Willems, P., and Cea, L.: Assessing the Effects of Climate Change on Compound Flooding in Coastal River Areas, Water Resour. Res., 57, e2020WR029321, https://doi.org/10.1029/2020WR029321, 2021.
Bevacqua, E., Maraun, D., Hobæk Haff, I., Widmann, M., and Vrac, M.: Multivariate statistical modelling of compound events via pair-copula constructions: analysis of floods in Ravenna (Italy), Hydrol. Earth Syst. Sci., 21, 2701–2723, https://doi.org/10.5194/hess-21-2701-2017, 2017.
Bevacqua, E., Maraun, D., Vousdoukas, M. I., Voukouvalas, E., Vrac, M., Mentaschi, L., and Widmann, M.: Higher probability of compound flooding from precipitation and storm surge in Europe under anthropogenic climate change, Science Advances, 5, eaaw5531, https://doi.org/10.1126/SCIADV.AAW5531, 2019.
Bevacqua, E., Vousdoukas, M. I., Shepherd, T. G., and Vrac, M.: Brief communication: The role of using precipitation or river discharge data when assessing global coastal compound flooding, Nat. Hazards Earth Syst. Sci., 20, 1765–1782, https://doi.org/10.5194/nhess-20-1765-2020, 2020a.
Bevacqua, E., Vousdoukas, M. I., Zappa, G., Hodges, K., Shepherd, T. G., Maraun, D., Mentaschi, L., and Feyen, L.: More meteorological events that drive compound coastal flooding are projected under climate change, Communications Earth and Environment, 1, 47, https://doi.org/10.1038/S43247-020-00044-Z, 2020b.
Bevacqua, E., De Michele, C., Manning, C., Couasnon, A., Ribeiro, A. F. S., Ramos, A. M., Vignotto, E., Bastos, A., Blesic, S., Durante, F., Hillier, J., Oliveira, S. C., Pinto, J. G., Ragno, E., Rivoire, P., Saunders, K., Van Der Wiel, K., Wu, W., Zhang, T., and Zscheischler, J.: Bottom-up identification of key elements of compound events, ESS Open Archive, https://doi.org/10.1002/ESSOAR.10507809.1, 2022.
Bevacqua, E., De Michele, C., Manning, C., Couasnon, A., Ribeiro, A. F. S., Ramos, A. M., Vignotto, E., Bastos, A., Blesiæ, S., Durante, F., Hillier, J., Oliveira, S. C., Pinto, J. G., Ragno, E., Rivoire, P., Saunders, K., van der Wiel, K., Wu, W., Zhang, T., and Zscheischler, J.: Guidelines for Studying Diverse Types of Compound Weather and Climate Events, Earth's Future, 9, e2021EF002340, https://doi.org/10.1029/2021EF002340, 2021.
Bevere, L. and Remondi, F.: Natural catastrophes in 2021: the floodgates are open, Swiss Re Institute, Zurich, Switzerland, https://www.swissre.com/dam/jcr:326182d5-d433-46b1-af36-06f2aedd9d9a/swiss-re-institute-sigma-natcat-2022-en.pdf (last access: 17 July 2024), 2022.
Bilskie, M. V. and Hagen, S. C.: Defining Flood Zone Transitions in Low-Gradient Coastal Regions, Geophys. Res. Lett., 45, 2761–2770, https://doi.org/10.1002/2018GL077524, 2018.
Bilskie, M. V., Zhao, H., Resio, D., Atkinson, J., Cobell, Z., and Hagen, S. C.: Enhancing Flood Hazard Assessments in Coastal Louisiana Through Coupled Hydrologic and Surge Processes, Frontiers in Water, 3, 609231, https://doi.org/10.3389/FRWA.2021.609231, 2021.
Bischiniotis, K., van den Hurk, B., Jongman, B., Coughlan de Perez, E., Veldkamp, T., de Moel, H., and Aerts, J.: The influence of antecedent conditions on flood risk in sub-Saharan Africa, Nat. Hazards Earth Syst. Sci., 18, 271–285, https://doi.org/10.5194/nhess-18-271-2018, 2018.
Blanton, B., McGee, J., Fleming, J., Kaiser, C., Kaiser, H., Lander, H., Luettich, R., Dresback, K., and Kolar, R.: Urgent Computing of Storm Surge for North Carolina's Coast, Procedia Comput. Sci., 9, 1677–1686, https://doi.org/10.1016/J.PROCS.2012.04.185, 2012.
Blanton, B., Dresback, K., Colle, B., Kolar, R., Vergara, H., Hong, Y., Leonardo, N., Davidson, R., Nozick, L., and Wachtendorf, T.: An Integrated Scenario Ensemble-Based Framework for Hurricane Evacuation Modeling: Part 2 – Hazard Modeling, Risk Anal., 40, 117–133, https://doi.org/10.1111/RISA.13004, 2018.
Bloemendaal, N., Haigh, I. D., de Moel, H., Muis, S., Haarsma, R. J., and Aerts, J. C. J. H.: Generation of a global synthetic tropical cyclone hazard dataset using STORM, Scientific Data, 7, 40, https://doi.org/10.1038/s41597-020-0381-2, 2020.
Blöschl, G., Hall, J., Parajka, J., Perdigão, R. A. P., Merz, B., Arheimer, B., Aronica, G. T., Bilibashi, A., Bonacci, O., Borga, M., Èanjevac, I., Castellarin, A., Chirico, G. B., Claps, P., Fiala, K., Frolova, N., Gorbachova, L., Gül, A., Hannaford, J., Harrigan, S., Kireeva, M., Kiss, A., Kjeldsen, T. R., Kohnová, S., Koskela, J. J., Ledvinka, O., Macdonald, N., Mavrova-Guirguinova, M., Mediero, L., Merz, R., Molnar, P., Montanari, A., Murphy, C., Osuch, M., Ovcharuk, V., Radevski, I., Rogger, M., Salinas, J. L., Sauquet, E., Šraj, M., Szolgay, J., Viglione, A., Volpi, E., Wilson, D., Zaimi, K., and Živkoviæ, N.: Changing climate shifts timing of European floods, Science, 357, 588–590, https://doi.org/10.1126/science.aan2506, 2017.
Borrero, J. C., Cronin, S. J., Latu'ila, F. H., Tukuafu, P., Heni, N., Tupou, A. M., Kula, T., Fa'anunu, O., Bosserelle, C., Lane, E., Lynett, P., and Kong, L.: Tsunami Runup and Inundation in Tonga from the January 2022 Eruption of Hunga Volcano, Pure Appl. Geophys., 180, 1–22, https://doi.org/10.1007/s00024-022-03215-5, 2023.
Brönnimann, S., Martius, O., Rohr, C., Bresch, D. N., and Lin, K.-H. E.: Historical weather data for climate risk assessment, Ann. NY Acad. Sci., 1436, 121–137, https://doi.org/10.1111/nyas.13966, 2019.
Bronstert, A., Niehoff, D., and Schiffler, G. R.: Modelling infiltration and infiltration excess: The importance of fast and local processes, Hydrol. Process., 37, e14875, https://doi.org/10.1002/hyp.14875, 2023.
Brown, J. D., Spencer, T., and Moeller, I.: Modeling storm surge flooding of an urban area with particular reference to modeling uncertainties: A case study of Canvey Island, United Kingdom, Water Resour. Res., 43, W06402, https://doi.org/10.1029/2005WR004597, 2007.
Bunya, S., Dietrich, J. C., Westerink, J. J., Ebersole, B. A., Smith, J. M., Atkinson, J. H., Jensen, R., Resio, D. T., Luettich, R. A., Dawson, C., Cardone, V. J., Cox, A. T., Powell, M. D., Westerink, H. J., and Roberts, H. J.: A High-Resolution Coupled Riverine Flow, Tide, Wind, Wind Wave, and Storm Surge Model for Southern Louisiana and Mississippi. Part I: Model Development and Validation, Mon. Weather Rev., 138, 345–377, https://doi.org/10.1175/2009MWR2906.1, 2010.
Burn, D. H. and Whitfield, P. H.: Climate related changes to flood regimes show an increasing rainfall influence, J. Hydrol., 617, 129075, https://doi.org/10.1016/j.jhydrol.2023.129075, 2023.
Bush, S. T., Dresback, K. M., Szpilka, C. M., and Kolar, R. L.: Use of 1D Unsteady HEC-RAS in a Coupled System for Compound Flood Modeling: North Carolina Case Study, Journal of Marine Science and Engineering, 10, 306, https://doi.org/10.3390/JMSE10030306, 2022.
Camus, P., Haigh, I. D., Nasr, A. A., Wahl, T., Darby, S. E., and Nicholls, R. J.: Regional analysis of multivariate compound coastal flooding potential around Europe and environs: sensitivity analysis and spatial patterns, Nat. Hazards Earth Syst. Sci., 21, 2021–2040, https://doi.org/10.5194/nhess-21-2021-2021, 2021.
Camus, P., Haigh, I. D., Wahl, T., Nasr, A. A., Méndez, F. J., Darby, S. E., and Nicholls, R. J.: Daily synoptic conditions associated with occurrences of compound events in estuaries along North Atlantic coastlines, Int. J. Climatol., 42, 5694–5713, https://doi.org/10.1002/JOC.7556, 2022.
Cannon, S. H., Gartner, J. E., Wilson, R. C., Bowers, J. C., and Laber, J. L.: Storm rainfall conditions for floods and debris flows from recently burned areas in southwestern Colorado and southern California, Geomorphology, 96, 250–269, https://doi.org/10.1016/j.geomorph.2007.03.019, 2008.
Čepienė, E., Dailidytė, L., Stonevičius, E., and Dailidienė, I.: Sea Level Rise Impact on Compound Coastal River Flood Risk in Klaipėda City (Baltic Coast, Lithuania), Water, 14, 414, https://doi.org/10.3390/W14030414, 2022.
Chen, A. S., Djordjeviæ, S., Leandro, J., and Saviæ, D. A.: An analysis of the combined consequences of pluvial and fluvial flooding, Water Sci. Technol., 62, 1491–1498, https://doi.org/10.2166/wst.2010.486, 2010.
Chen, C. N., Tsai, C. H., Wu, M. H., and Tsai, C. T.: Numerical simulation of potential inundation in a coastal zone, J. Flood Risk Manag., 8, 208–223, https://doi.org/10.1111/JFR3.12088, 2015.
Chen, W. B. and Liu, W. C.: Modeling flood inundation induced by river flow and storm surges over a river basin, Water, 6, 3182–3199, https://doi.org/10.3390/W6103182, 2014.
Chen, W. B. and Liu, W. C.: Assessment of storm surge inundation and potential hazard maps for the southern coast of Taiwan, Nat. Hazards, 82, 591–616, https://doi.org/10.1007/S11069-016-2199-Y, 2016.
Chou, L. W.: Typhoon water surface analysis for west coast of Saipan: Mariana Islands, Coastal Engineering Research Center, https://hdl.handle.net/11681/12955 (last access: 17 July 2024), 1989.
Christian, J., Fang, Z., Torres, J., Deitz, R., and Bedient, P.: Modeling the Hydraulic Effectiveness of a Proposed Storm Surge Barrier System for the Houston Ship Channel during Hurricane Events, Nat. Hazards Rev., 16, 04014015, https://doi.org/10.1061/(ASCE)NH.1527-6996.0000150, 2015.
Church, J., Clark, P., Cazenave, A., Gregory, J., Jevrejeva, S., Levermann, A., Merrifield, M., Milne, G., Nerem, R., and Nunn, P.: Climate Change 2013: The Physical Science Basis: Contribution of Working Group I to the Fifth Assessment Report of the Intergovernmental Panel on Climate Change, Cambridge University Press, Cambridge, United Kingdom, New York, USA, 1137–1216, https://doi.org/10.1017/CBO9781107415324.026, 2013.
Church, J. A., Gregory, J. M., Huybrechts, P., Kuhn, M., Lambeck, K., Nhuan, M. T., Qin, D., and Woodworth, P. L.: Changes in Sea Level, in: Climate Change 2001: The Scientific Basis: Contribution of Working Group I to the Third Assessment Report of the Intergovernmental Panel, 639–694, 10013/epic.15081.d001, 2001.
Cifelli, R., Johnson, L. E., Kim, J., Coleman, T., Pratt, G., Herdman, L., Martyr-Koller, R., Finzihart, J. A., Erikson, L., Barnard, P., and Anderson, M.: Assessment of flood forecast products for a coupled tributary-coastal model, Water, 13, 1–24, https://doi.org/10.3390/W13030312, 2021.
Claassen, J., Ward, P., Daniell, J., Koks, E., Tiggeloven, T., and de Ruiter, M.: MYRIAD-HESA: A New Method to Generate Global Multi-Hazard Event Sets, Vrije Universiteit Amsterdam, https://doi.org/10.21203/rs.3.rs-2635188/v1, 2023.
Coles, S., Heffernan, J., and Tawn, J.: Dependence Measures for Extreme Value Analyses, Extremes, 2, 339–365, https://doi.org/10.1023/A:1009963131610, 1999.
Coles, S. G. and Tawn, J. A.: Statistical Methods for Multivariate Extremes: An Application to Structural Design, J. R. Stat. Soc. C-Appl., 43, 1–48, https://doi.org/10.2307/2986112, 1994.
Comer, J., Olbert, A. I., Nash, S., and Hartnett, M.: Development of high-resolution multi-scale modelling system for simulation of coastal-fluvial urban flooding, Nat. Hazards Earth Syst. Sci., 17, 205–224, https://doi.org/10.5194/nhess-17-205-2017, 2017.
Costa, J. E.: Floods from dam failures, Report 85-560, https://doi.org/10.3133/ofr85560, 1985.
Couasnon, A., Sebastian, A., and Morales-Nápoles, O.: A Copula-based bayesian network for modeling compound flood hazard from riverine and coastal interactions at the catchment scale: An application to the houston ship channel, Texas, Water, 10, 1190, https://doi.org/10.3390/W10091190, 2018.
Couasnon, A., Eilander, D., Muis, S., Veldkamp, T. I. E., Haigh, I. D., Wahl, T., Winsemius, H. C., and Ward, P. J.: Measuring compound flood potential from river discharge and storm surge extremes at the global scale, Nat. Hazards Earth Syst. Sci., 20, 489–504, https://doi.org/10.5194/nhess-20-489-2020, 2020.
Curtis, S., Mukherji, A., Kruse, J., Helgeson, J., Ghosh, A., and Adeniji, N.: Perceptions of risk to compound coastal water events: A case study in eastern North Carolina, USA, Progress in Disaster Science, 16, 100266–100266, https://doi.org/10.1016/J.PDISAS.2022.100266, 2022.
Cutter, S. L.: Compound, Cascading, or Complex Disasters: What's in a Name?, Environment: Science and Policy for Sustainable Development, 60, 16–25, https://doi.org/10.1080/00139157.2018.1517518, 2018.
Dahl, K. A., Fitzpatrick, M. F., and Spanger-Siegfried, E.: Sea level rise drives increased tidal flooding frequency at tide gauges along the U.S. East and Gulf Coasts: Projections for 2030 and 2045, PLOS ONE, 12, e0170949, https://doi.org/10.1371/journal.pone.0170949, 2017.
Daniell, J. E., Schaefer, A. M., and Wenzel, F.: Losses Associated with Secondary Effects in Earthquakes, Front. Built Environ., 3, 30, https://doi.org/10.3389/fbuil.2017.00030, 2017.
Daoued, A. B., Mouhous-Voyneau, N., Hamdi, Y., and Sergent, P.: Development of a Probabilistic Multi-flood Hazard Approach Considering Uncertainties and Climate Change–Application to the Coastal Flooding of the Havre (France), Recent Advances in Environmental Science from the Euro-Mediterranean and Surrounding Regions (2nd Edition), Cham, 2011–2016, https://doi.org/10.1007/978-3-030-51210-1_315, 2021.
de Bruijn, K. M., Diermanse, F. L. M., and Beckers, J. V. L.: An advanced method for flood risk analysis in river deltas, applied to societal flood fatality risk in the Netherlands, Nat. Hazards Earth Syst. Sci., 14, 2767–2781, https://doi.org/10.5194/nhess-14-2767-2014, 2014.
Deidda, C., Rahimi, L., and de Michele, C.: Causes of dependence between extreme floods, Environ. Res. Lett., 16, 084002, https://doi.org/10.1088/1748-9326/AC07D5, 2021.
De Michele, C., Meroni, V., Rahimi, L., Deidda, C., and Ghezzi, A.: Dependence Types in a Binarized Precipitation Network, Geophys. Res. Lett., 47, e2020GL090196, https://doi.org/10.1029/2020GL090196, 2020.
De Ruiter, M. C., Couasnon, A., van den Homberg, M. J. C., Daniell, J. E., Gill, J. C., and Ward, P. J.: Why We Can No Longer Ignore Consecutive Disasters, Earth's Future, 8, e2019EF001425, https://doi.org/10.1029/2019EF001425, 2020.
Del-Rosal-Salido, J., Folgueras, P., Bermúdez, M., Ortega-Sánchez, M., and Losada, M.: Flood management challenges in transitional environments: Assessing the effects of sea-level rise on compound flooding in the 21st century, Coast. Eng., 167, 103872, https://doi.org/10.1016/J.COASTALENG.2021.103872, 2021.
Dietrich, J. C., Bunya, S., Westerink, J. J., Ebersole, B. A., Smith, J. M., Atkinson, J. H., Jensen, R., Resio, D. T., Luettich, R. A., Dawson, C., Cardone, V. J., Cox, A. T., Powell, M. D., Westerink, H. J., and Roberts, H. J.: A High-Resolution Coupled Riverine Flow, Tide, Wind, Wind Wave, and Storm Surge Model for Southern Louisiana and Mississippi. Part II: Synoptic Description and Analysis of Hurricanes Katrina and Rita, Mon. Weather Rev., 138, 378–404, https://doi.org/10.1175/2009mwr2907.1, 2010.
Dixon, M. J. and Tawn, J. A.: Extreme sea-levels at the UK A-class sites: site-by-site analyses, Proudman Oceanographic Laboratory, https://ntslf.org/sites/ntslf/files/pdf/other_reports/id65.pdf (last access: 17 July 2024), 1994.
Donges, J. F., Schleussner, C. F., Siegmund, J. F., and Donner, R. V.: Event coincidence analysis for quantifying statistical interrelationships between event time series, The European Physical Journal Special Topics, 225, 471–487, https://doi.org/10.1140/epjst/e2015-50233-y, 2016.
Dresback, K. M., Fleming, J. G., Blanton, B. O., Kaiser, C., Gourley, J. J., Tromble, E. M., Luettich, R. A., Kolar, R. L., Hong, Y., Van Cooten, S., Vergara, H. J., Flamig, Z. L., Lander, H. M., Kelleher, K. E., and Nemunaitis-Monroe, K. L.: Skill assessment of a real-time forecast system utilizing a coupled hydrologic and coastal hydrodynamic model during Hurricane Irene (2011), Cont. Shelf Res., 71, 78–94, https://doi.org/10.1016/J.CSR.2013.10.007, 2013.
Dykstra, S. L. and Dzwonkowski, B.: The Role of Intensifying Precipitation on Coastal River Flooding and Compound River-Storm Surge Events, Northeast Gulf of Mexico, Water Resour. Res., 57, e2020WR029363, https://doi.org/10.1029/2020WR029363, 2021.
EAA: Green Infrastructure and Flood Management, European Environment Agency (EEA), Copenhagen, Denmark, https://doi.org/10.2800/324289, 2017.
Easterling, D. R., Kunkel, K. E., Wehner, M. F., and Sun, L.: Detection and attribution of climate extremes in the observed record, Weather and Climate Extremes, 11, 17–27, https://doi.org/10.1016/j.wace.2016.01.001, 2016.
Eilander, D., Couasnon, A., Ikeuchi, H., Muis, S., Yamazaki, D., Winsemius, H. C., and Ward, P. J.: The effect of surge on riverine flood hazard and impact in deltas globally, Environ. Res. Lett., 15, 104007–104007, https://doi.org/10.1088/1748-9326/AB8CA6, 2020.
Eilander, D., Couasnon, A., Leijnse, T., Ikeuchi, H., Yamazaki, D., Muis, S., Dullaart, J., Winsemius, H. C., and Ward, P. J.: A globally-applicable framework for compound flood hazard modeling, EGUsphere, 1-40, https://doi.org/10.5194/egusphere-2022-149, 2022.
Eilander, D., Couasnon, A., Sperna Weiland, F. C., Ligtvoet, W., Bouwman, A., Winsemius, H. C., and Ward, P. J.: Modeling compound flood risk and risk reduction using a globally applicable framework: a pilot in the Sofala province of Mozambique, Nat. Hazards Earth Syst. Sci., 23, 2251–2272, https://doi.org/10.5194/nhess-23-2251-2023, 2023.
EM-DAT: International Disaster Database, https://www.emdat.be (last access: 17 July 2024), 2022.
Erikson, L. H., O'Neill, A. C., and Barnard, P. L.: Estimating fluvial discharges coincident with 21st century coastal storms modeled with CoSMoS, J. Coastal Res., 85, 791–795, https://doi.org/10.2112/SI85-159.1, 2018.
Eshrati, L., Mahmoudzadeh, A., and Taghvaei, M.: Multi hazards risk assessment, a new methodology, International Journal of Health System and Disaster Management, 3, 79, https://www.researchgate.net/publication/331687882 (last access: 17 July 2024), 2015.
Familkhalili, R., Talke, S. A., and Jay, D. A.: Compound flooding in convergent estuaries: insights from an analytical model, Ocean Sci., 18, 1203–1220, https://doi.org/10.5194/os-18-1203-2022, 2022.
Fang, J., Wahl, T., Fang, J., Sun, X., Kong, F., and Liu, M.: Compound flood potential from storm surge and heavy precipitation in coastal China: dependence, drivers, and impacts, Hydrol. Earth Syst. Sci., 25, 4403–4416, https://doi.org/10.5194/hess-25-4403-2021, 2021.
Feng, Y. and Brubaker, K. L.: Change and Sea-Level Rise Using a One-Dimensional Hydraulic Model, J. Hydrol. Eng., 21, 05016015, https://doi.org/10.1061/(ASCE)HE.1943-5584.0001378, 2016.
Ferrarin, C., Lionello, P., Orlić, M., Raicich, F., and Salvadori, G.: Venice as a paradigm of coastal flooding under multiple compound drivers, Sci. Rep., 12, 5754, https://doi.org/10.1038/s41598-022-09652-5, 2022.
Flick, R. E.: Joint Occurrence of High Tide and Storm Surge in California, World Marina'91, 52–60, https://escholarship.org/uc/item/0h62w9w3 (last access: 17 July 2024), 1991.
Galiatsatou, P. and Prinos, P.: Joint probability analysis of extreme wave heights and storm surges in the Aegean Sea in a changing climate, FLOODrisk 2016 – 3rd European Conference on Flood Risk Management, 7, 12, https://doi.org/10.1051/e3sconf/20160702002, 2016.
Gallien, T. W., Kalligeris, N., Delisle, M. P. C., Tang, B. X., Lucey, J. T. D., and Winters, M. A.: Coastal flood modeling challenges in defended urban backshores, Geosciences, 8, 450, https://doi.org/10.3390/geosciences8120450, 2018.
Gallina, V., Torresan, S., Critto, A., Sperotto, A., Glade, T., and Marcomini, A.: A review of multi-risk methodologies for natural hazards: Consequences and challenges for a climate change impact assessment, J. Environ. Manage., 168, 123–132, https://doi.org/10.1016/J.JENVMAN.2015.11.011, 2016.
Ganguli, P. and Merz, B.: Extreme Coastal Water Levels Exacerbate Fluvial Flood Hazards in Northwestern Europe, Sci. Rep., 9, 13165, https://doi.org/10.1038/s41598-019-49822-6, 2019a.
Ganguli, P. and Merz, B.: Trends in Compound Flooding in Northwestern Europe During 1901–2014, Geophys. Res. Lett., 46, 10810–10820, https://doi.org/10.1029/2019GL084220, 2019b.
Ganguli, P., Nandamuri, Y. R., and Chatterjee, C.: Analysis of persistence in the flood timing and the role of catchment wetness on flood generation in a large river basin in India, Theor. Appl. Climatol., 139, 373–388, https://doi.org/10.1007/s00704-019-02964-z, 2019.
Ganguli, P., Paprotny, D., Hasan, M., Güntner, A., and Merz, B.: Projected Changes in Compound Flood Hazard From Riverine and Coastal Floods in Northwestern Europe, Earth's Future, 8, e2020EF001752, https://doi.org/10.1029/2020EF001752, 2020.
Georgas, N., Blumberg, A., Herrington, T., Wakeman, T., Saleh, F., Runnels, D., Jordi, A., Ying, K., Yin, L., Ramaswamy, V., Yakubovskiy, A., Lopez, O., McNally, J., Schulte, J., and Wang, Y.: The stevens flood advisory system: operational H3Eflood forecasts for the greater New York/New Jersey metropolitan region, International Journal of Safety and Security Engineering, 6, 648–662, https://doi.org/10.2495/SAFE-V6-N3-648-662, 2016.
Ghanbari, M., Arabi, M., Kao, S. C., Obeysekera, J., and Sweet, W.: Climate Change and Changes in Compound Coastal-Riverine Flooding Hazard Along the U.S. Coasts, Earth's Future, 9, e2021EF002055, https://doi.org/10.1029/2021EF002055, 2021.
Gill, J. C. and Malamud, B. D.: Reviewing and visualizing the interactions of natural hazards, Rev. Geophys., 52, 680–722, https://doi.org/10.1002/2013RG000445, 2014.
Gill, J. C., Duncan, M., Ciurean, R., Smale, L., Stuparu, D., Schlumberger, J., de Ruiter, M., Tiggeloven, T., Torresan, S., Gottardo, S., Mysiak, J., Harris, R., Petrescu, E.-C., Girard, T., Khazai, B., Claassen, J., Dai, R., Champion, A., Daloz, A. S., Blanco Cipollone, F., Campillo Torres, C., Palomino Antolin, I., Ferrario, D., Tatman, S., Tijessen, A., Vaidya, S., Adesiyun, A., Goger, T., Angiuli, A., Audren, M., Machado, M., Hochrainer-Stigler, S., Šakiæ Trogrliæ, R., Daniell, J., Bulder, B., Krishna Swamy, S., Wiggelinkhuizen, E.-J., Díaz Pacheco, J., López Díez, A., Mendoza Jiménez, J., Padrón-Fumero, N., Appulo, L., Orth, R., Sillmann, J., and Ward, P.: MYRIAD-EU Project D1.2 Handbook of multi-hazard, multi-risk definitions and concepts, 75, http://nora.nerc.ac.uk/id/eprint/533237/ (last access: 17 July 2024), 2020.
Gori, A. and Lin, N.: Projecting compound flood hazard under climate change with physical models and joint probability methods, Earth's Future, 10, e2022EF003097, https://doi.org/10.1029/2022EF003097, 2022.
Gori, A., Lin, N., and Smith, J.: Assessing Compound Flooding From Landfalling Tropical Cyclones on the North Carolina Coast, Water Resour. Res., 56, e2019WR026788, https://doi.org/10.1029/2019WR026788, 2020a.
Gori, A., Lin, N., and Xi, D.: Tropical Cyclone Compound Flood Hazard Assessment: From Investigating Drivers to Quantifying Extreme Water Levels, Earth's Future, 8, e2020EF001660, https://doi.org/10.1029/2020EF001660, 2020b.
Gori, A., Lin, N., Xi, D., and Emanuel, K.: Tropical cyclone climatology change greatly exacerbates US extreme rainfall–surge hazard, Nat. Clim. Change, 12, 171–178, https://doi.org/10.1038/s41558-021-01272-7, 2022.
Gouldby, B., Wyncoll, D., Panzeri, M., Franklin, M., Hunt, T., Hames, D., Tozer, N., Hawkes, P., Dornbusch, U., and Pullen, T.: Multivariate extreme value modelling of sea conditions around the coast of England, P. I. Civil Eng.-Mar. Eng., 170, 3–20, https://doi.org/10.1680/jmaen.2016.16, 2017.
Green, J.: Green et al. 2024 – Compound Flood Literature Review Database, Zenodo [data set], https://doi.org/10.5281/zenodo.14274658, 2024.
Guan, X., Vorogushyn, S., Apel, H., and Merz, B.: Assessing compound pluvial-fluvial flooding: Research status and ways forward, Water Security, 19, 100136, https://doi.org/10.1016/j.wasec.2023.100136, 2023.
Gutenson, J. L., Tavakoly, A. A., Islam, M. S., Wing, O. E. J., Lehman, W. P., Hamilton, C. O., Wahl, M. D., and Massey, T. C.: Comparison of estimated flood exposure and consequences generated by different event-based inland flood inundation maps, Nat. Hazards Earth Syst. Sci., 23, 261–277, https://doi.org/10.5194/nhess-23-261-2023, 2023.
Habel, S., Fletcher, C. H., Anderson, T. R., and Thompson, P. R.: Sea-Level Rise Induced Multi-Mechanism Flooding and Contribution to Urban Infrastructure Failure, Sci. Rep., 10, 1–12, https://doi.org/10.1038/s41598-020-60762-4, 2020.
Haigh, I. and Nicholls, R. J.: Coastal Flooding, Marine Climate Change Impacts Partnership, 98-104, https://doi.org/10.14465/2017.arc10.009-cof, 2017.
Haigh, I. D., Wadey, M. P., Wahl, T., Ozsoy, O., Nicholls, R. J., Brown, J. M., Horsburgh, K., and Gouldby, B.: Spatial and temporal analysis of extreme sea level and storm surge events around the coastline of the UK, Scientific Data, 3, 160107, https://doi.org/10.1038/SDATA.2016.107, 2016.
Haigh, I. D., Pickering, M. D., Green, J. A. M., Arbic, B. K., Arns, A., Dangendorf, S., Hill, D. F., Horsburgh, K., Howard, T., Idier, D., Jay, D. A., Jänicke, L., Lee, S. B., Müller, M., Schindelegger, M., Talke, S. A., Wilmes, S. B., and Woodworth, P. L.: The Tides They Are A-Changin': A Comprehensive Review of Past and Future Nonastronomical Changes in Tides, Their Driving Mechanisms, and Future Implications, Rev. Geophys., 58, e2018RG000636, https://doi.org/10.1029/2018RG000636, 2020.
Hall, T. M. and Jewson, S.: Statistical modelling of North Atlantic tropical cyclone tracks, Tellus A, 59, 486–498, https://doi.org/10.1111/j.1600-0870.2007.00240.x, 2007.
Hall, T. and Yonekura, E.: North American Tropical Cyclone Landfall and SST: A Statistical Model Study, J. Climate, 26, 8422–8439, https://doi.org/10.1175/JCLI-D-12-00756.1, 2013.
Hallegatte, S., Green, C., Nicholls, R. J., and Corfee-Morlot, J.: Future flood losses in major coastal cities, Nat. Clim. Change, 3, 802–806, https://doi.org/10.1038/NCLIMATE1979, 2013.
Hao, Z. and Singh, V. P.: Compound Events under Global Warming: A Dependence Perspective, J. Hydrol. Eng., 25, 03120001, https://doi.org/10.1061/(ASCE)HE.1943-5584.0001991, 2020.
Hao, Z., Singh, V. P., and Hao, F.: Compound extremes in hydroclimatology: A review, Water, 10, 718, https://doi.org/10.3390/W10060718, 2018.
Harrison, L. M., Coulthard, T. J., Robins, P. E., and Lewis, M. J.: Sensitivity of Estuaries to Compound Flooding, Estuar. Coast., 45, 1250–1269, https://doi.org/10.1007/S12237-021-00996-1, 2022.
Hawkes, P.: Extreme water levels in estuaries and rivers: the combined influence of tides, river flows and waves, Defra/Environment Agency R&D Technical Report FD0206/TR1, HR Wallingford, https://assets.publishing.service.gov.uk/media/60254e8de90e070561b313da/Joint_probability_of_extreme_estuarine_water_levels__technical_report.pdf (last access: 17 July 2024), 2003.
Hawkes, P. J.: Joint probability analysis for estimation of extremes, J. Hydraul. Res., 46, 246–256, https://doi.org/10.1080/00221686.2008.9521958, 2008.
Hawkes, P. and Svensson, C.: Joint Probability: Dependence Mapping and Best Practice, Defra/Environment Agency R&D Interim Technical Report FD2308/TR1, HR Wallingford, https://assets.publishing.service.gov.uk/media/602bae1fe90e07055e422831/Technical_Report_-_Interim_Product__Joint_probability_dependence_mapping_and_best_practice__.pdf (last access: 17 July 2024), 2003.
Hawkes, P. and Svensson, C.: Use of Joint Probability Methods in Flood Management: A guide to best practice, Defra/Environment Agency R&D Technical Report FD2308/TR2, HR Wallingford, https://assets.publishing.service.gov.uk/media/602bae7ae90e070559970fbc/Use_of_joint_probability_methods_in_flood_management_A_guide_to_best_practice_technical_report.pdf (last access: 17 July 2024), 2006.
Hawkes, P. J., Gouldby, B. P., Tawn, J. A., and Owen, M. W.: The joint probability of waves and water levels in coastal engineering, J. Hydraul. Res., 40, 241–251, https://doi.org/10.1080/00221680209499940, 2002.
Helaire, L. T., Talke, S. A., Jay, D. A., and Chang, H.: Present and Future Flood Hazard in the Lower Columbia River Estuary: Changing Flood Hazards in the Portland-Vancouver Metropolitan Area, J. Geophys. Res.-Oceans, 125, e2019JC015928, https://doi.org/10.1029/2019JC015928, 2020.
Hemer, M. A., Wang, X. L., Church, J. A., and Swail, V. R.: Coordinating Global Ocean Wave Climate Projections, B. Am. Meteorol. Soc., 91, 451–454, https://doi.org/10.1175/2009BAMS2951.1, 2010.
Hendry, A., Haigh, I. D., Nicholls, R. J., Winter, H., Neal, R., Wahl, T., Joly-Laugel, A., and Darby, S. E.: Assessing the characteristics and drivers of compound flooding events around the UK coast, Hydrol. Earth Syst. Sci., 23, 3117–3139, https://doi.org/10.5194/hess-23-3117-2019, 2019.
Herdman, L., Erikson, L., and Barnard, P.: Storm Surge Propagation and Flooding in Small Tidal Rivers during Events of Mixed Coastal and Fluvial Influence, Journal of Marine Science and Engineering, 6, 158–158, https://doi.org/10.3390/JMSE6040158, 2018.
Herring, D.: What is an “extreme event”? Is there evidence that global warming has caused or contributed to any particular extreme event?, National Oceanic and Atmospheric Administration (NOAA), https://www.climate.gov/news-features/climate-qa/what-extreme-event-there-evidence-global-warming-has-caused-or-contributed (last access: 1 January 2024), 2020.
Hewitt, K. and Burton, I.: Hazardousness of a place: A regional ecology of damaging events, University of Toronto Press, https://www.researchgate.net/publication/275689020 (last access: 17 July 2024), 1971.
Hinkel, J., Lincke, D., Vafeidis, A. T., Perrette, M., Nicholls, R. J., Tol, R. S. J., Marzeion, B., Fettweis, X., Ionescu, C., and Levermann, A.: Coastal flood damage and adaptation costs under 21st century sea-level rise, P. Natl. Acad. Sci. USA, 111, 3292–3297, https://doi.org/10.1073/PNAS.1222469111, 2014.
Ho, F. P. and Myers, V. A.: Joint probability method of tide frequency analysis applied to Apalachicola Bay and St. George Sound, Florida, NOAA, https://repository.library.noaa.gov/view/noaa/33908/noaa_33908_DS1.pdf (last access: 17 July 2024), 1975.
Hoitink, A. J. F. and Jay, D. A.: Tidal river dynamics: Implications for deltas, Rev. Geophys., 54, 240–272, https://doi.org/10.1002/2015RG000507, 2016.
Holt, C.: What is groundwater flooding?, Environment Agency, UK, https://environmentagency.blog.gov.uk/2019/12/23/what-is-groundwater-flooding/ (last access: 1 January 2024), 2019.
Hsiao, S. C., Chiang, W. S., Jang, J. H., Wu, H. L., Lu, W. S., Chen, W. B., and Wu, Y. T.: Flood risk influenced by the compound effect of storm surge and rainfall under climate change for low-lying coastal areas, Sci. Total Environ., 764, 144439, https://doi.org/10.1016/j.scitotenv.2020.144439, 2021.
Huang, P. C.: An effective alternative for predicting coastal floodplain inundation by considering rainfall, storm surge, and downstream topographic characteristics, J. Hydrol., 607, 127544–127544, https://doi.org/10.1016/J.JHYDROL.2022.127544, 2022.
Huang, W., Ye, F., Zhang, Y. J., Park, K., Du, J., Moghimi, S., Myers, E., Pe'eri, S., Calzada, J. R., Yu, H. C., Nunez, K., and Liu, Z.: Compounding factors for extreme flooding around Galveston Bay during Hurricane Harvey, Ocean Model., 158, 101735, https://doi.org/10.1016/j.ocemod.2020.101735, 2021.
Hutton, E. W. H., Piper, M. D., and Tucker, G. E.: The Basic Model Interface 2.0: A standard interface for coupling numerical models in the geosciences, Journal of Open Source Software, 51, 5, https://doi.org/10.21105/joss.02317, 2020.
Idier, D., Bertin, X., Thompson, P., and Pickering, M.: Interactions Between Mean Sea Level, Tide, Surge, Waves and Flooding: Mechanisms and Contributions to Sea Level Variations at the Coast, Surv. Geophys., 40, 1603–1630, https://doi.org/10.1007/s10712-019-09549-5, 2019.
Ikeuchi, H., Hirabayashi, Y., Yamazaki, D., Muis, S., Ward, P. J., Winsemius, H. C., Verlaan, M., and Kanae, S.: Compound simulation of fluvial floods and storm surges in a global coupled river-coast flood model: Model development and its application to 2007 Cyclone Sidr in Bangladesh, J. Adv. Model. Earth Sy., 9, 1847–1862, https://doi.org/10.1002/2017MS000943, 2017.
IOTIC: What Causes Tsunami, Indian Ocean Tsunami InformationCentre (IOTIC), https://iotic.ioc-unesco.org/what-causes-tsunami/, (last access: 17 July 2024), 2020.
IPCC: Glossary of Terms, Cambridge University Press, Cambridge, United Kingdom, New York, USA, 555–564, https://doi.org/10.1017/CBO9781139177245.014, 2012.
IPCC: Annex II: Glossary, IPCC, Geneva, Switzerland, 117-130, ISBN 978-92-9169-143-2, https://archive.ipcc.ch/pdf/assessment-report/ar5/syr/SYR_AR5_FINAL_full_wcover.pdf (last access: 17 July 2024), 2014.
Jafarzadegan, K., Moradkhani, H., Pappenberger, F., Moftakhari, H., Bates, P., Abbaszadeh, P., Marsooli, R., Ferreira, C., Cloke, H. L., Ogden, F., and Duan, Q.: Recent Advances and New Frontiers in Riverine and Coastal Flood Modeling, Rev. Geophys., 61, e2022RG000788, https://doi.org/10.1029/2022RG000788, 2023.
Jalili Pirani, F. and Najafi, M. R.: Recent Trends in Individual and Multivariate Compound Flood Drivers in Canada's Coasts, Water Resour. Res., 56, e2020WR027785, https://doi.org/10.1029/2020WR027785, 2020.
Jalili Pirani, F. and Najafi, M. R.: Characterizing compound flooding potential and the corresponding driving mechanisms across coastal environments, Stoch. Environ. Res. Risk A., 37, 1943–1961, https://doi.org/10.1007/s00477-022-02374-0, 2022a.
Jalili Pirani, F. and Najafi, M. R.: Multivariate Analysis of Compound Flood Hazard Across Canada's Atlantic, Pacific and Great Lakes Coastal Areas, Earth's Future, 10, e2022EF002655, https://doi.org/10.1029/2022EF002655, 2022b.
Jane, R., Cadavid, L., Obeysekera, J., and Wahl, T.: Multivariate statistical modelling of the drivers of compound flood events in south Florida, Nat. Hazards Earth Syst. Sci., 20, 2681–2699, https://doi.org/10.5194/nhess-20-2681-2020, 2020.
Jane, R., Wahl, T., Santos Victor, M., Misra Shubhra, K., and White Kathleen, D.: Assessing the Potential for Compound Storm Surge and Extreme River Discharge Events at the Catchment Scale with Statistical Models: Sensitivity Analysis and Recommendations for Best Practice, J. Hydrol. Eng., 27, 16, https://doi.org/10.1061/(ASCE)HE.1943-5584.0002154, 2022.
Jang, J. H. and Chang, T. H.: Flood risk estimation under the compound influence of rainfall and tide, J. Hydrol., 606, 127446, https://doi.org/10.1016/j.jhydrol.2022.127446, 2022.
Jasim, F. H., Vahedifard, F., Alborzi, A., Moftakhari, H., and AghaKouchak, A.: Effect of Compound Flooding on Performance of Earthen Levees, Geo-Congress 2020, 707–716, https://doi.org/10.1061/9780784482797.069, 2020.
Jenkins, L. J., Haigh, I. D., Camus, P., Pender, D., Sansom, J., Lamb, R., and Kassem, H.: The temporal clustering of storm surge, wave height, and high sea level exceedances around the UK coastline, Nat. Hazards, 115, 1761–1797, https://doi.org/10.1007/s11069-022-05617-z, 2023.
Jones, D.: Joint Probability Fluvial-Tidal Analyses: Structure Functions and Historical Emulation, Institute of Hydrology, https://nora.nerc.ac.uk/id/eprint/6919/ (last access: 17 July 2024), 1998.
Jong-Levinger, A., Banerjee, T., Houston, D., and Sanders, B. F.: Compound Post-Fire Flood Hazards Considering Infrastructure Sedimentation, Earth's Future, 10, e2022EF002670, https://doi.org/10.1029/2022EF002670, 2022.
Joyce, J., Chang, N. B., Harji, R., Ruppert, T., and Singhofen, P.: Cascade impact of hurricane movement, storm tidal surge, sea level rise and precipitation variability on flood assessment in a coastal urban watershed, Clim. Dynam., 51, 383–409, https://doi.org/10.1007/S00382-017-3930-4, 2018.
Juárez, B., Stockton, S. A., Serafin, K. A., and Valle-Levinson, A.: Compound Flooding in a Subtropical Estuary Caused by Hurricane Irma 2017, Geophys. Res. Lett., 49, e2022GL099360, https://doi.org/10.1029/2022GL099360, 2022.
Kappes, M., Keiler, M., and Glade, T.: From Single- to Multi-Hazard Risk analyses: a concept addressing emerging challenges, Mountain Risks International Conference, Firenze, Italy, 24–26 November 2010, 351–356, ISBN 2-95183317-1-5, 2010.
Kappes, M. S., Keiler, M., von Elverfeldt, K., and Glade, T.: Challenges of analyzing multi-hazard risk: A review, Nat. Hazards, 64, 1925–1958, https://doi.org/10.1007/S11069-012-0294-2, 2012.
Karamouz, M., Ahmadvand, F., and Zahmatkesh, Z.: Distributed Hydrologic Modeling of Coastal Flood Inundation and Damage: Nonstationary Approach, J. Irrig. Drain. Eng., 143, 04017019, https://doi.org/10.1061/(ASCE)IR.1943-4774.0001173, 2017.
Karamouz, M., Zahmatkesh, Z., Goharian, E., and Nazif, S.: Combined Impact of Inland and Coastal Floods: Mapping Knowledge Base for Development of Planning Strategies, J. Water Res. Plan. Man., 141, 04014098, https://doi.org/10.1061/(ASCE)WR.1943-5452.0000497, 2014.
Katwala, A.: How Long Droughts Make Flooding Worse, WIRED, https://www.wired.co.uk/article/drought-causing-floods (last access: 17 July 2024), 2022.
Kerr, P. C., Westerink, J. J., Dietrich, J. C., Martyr, R. C., Tanaka, S., Resio, D. T., Smith, J. M., Westerink, H. J., Westerink, L. G., Wamsley, T., Ledden, M. v., and Jong, W. d.: Surge Generation Mechanisms in the Lower Mississippi River and Discharge Dependency, J. Waterw. Port C., 139, 326-335, https://doi.org/10.1061/(ASCE)WW.1943-5460.0000185, 2013.
Kew, S. F., Selten, F. M., Lenderink, G., and Hazeleger, W.: The simultaneous occurrence of surge and discharge extremes for the Rhine delta, Nat. Hazards Earth Syst. Sci., 13, 2017–2029, https://doi.org/10.5194/nhess-13-2017-2013, 2013.
Khalil, U., Yang, S., Sivakumar, M., Enever, K., Bin Riaz, M. Z., and Sajid, M.: Modelling the compound flood hydrodynamics under mesh convergence and future storm surge events in Brisbane River Estuary, Australia, Nat. Hazards Earth Syst. Sci. Discuss. [preprint], https://doi.org/10.5194/nhess-2021-284, 2022.
Khanal, S., Ridder, N., de Vries, H., Terink, W., and van den Hurk, B.: Storm Surge and Extreme River Discharge: A Compound Event Analysis Using Ensemble Impact Modeling, Front. Earth Sci., 7, 224, https://doi.org/10.3389/feart.2019.00224, 2019.
Khanam, M., Sofia, G., Koukoula, M., Lazin, R., Nikolopoulos, E. I., Shen, X., and Anagnostou, E. N.: Impact of compound flood event on coastal critical infrastructures considering current and future climate, Nat. Hazards Earth Syst. Sci., 21, 587–605, https://doi.org/10.5194/nhess-21-587-2021, 2021.
Khatun, A., Ganguli, P., Bisht, D. S., Chatterjee, C., and Sahoo, B.: Understanding the impacts of predecessor rain events on flood hazard in a changing climate, Hydrol. Process., 36, e14500, https://doi.org/10.1002/HYP.14500, 2022.
Kim, H., Villarini, G., Jane, R., Wahl, T., Misra, S., and Michalek, A.: On the generation of high-resolution probabilistic design events capturing the joint occurrence of rainfall and storm surge in coastal basins, Int. J. Climatol., 43, 761–771, https://doi.org/10.1002/JOC.7825, 2022.
Kim, B. and Sanders, B.: Dam-Break Flood Model Uncertainty Assessment: Case Study of Extreme Flooding with Multiple Dam Failures in Gangneung, South Korea, J. Hydraul. Eng., 142, 05016002, https://doi.org/10.1061/(ASCE)HY.1943-7900.0001097, 2016.
Kirezci, E., Young, I. R., Ranasinghe, R., Muis, S., Nicholls, R. J., Lincke, D., and Hinkel, J.: Projections of global-scale extreme sea levels and resulting episodic coastal flooding over the 21st Century, Sci. Rep., 10, 11629, https://doi.org/10.1038/s41598-020-67736-6, 2020.
Kirkpatrick, J. I. M. and Olbert, A. I.: Modelling the effects of climate change on urban coastal-fluvial flooding, J. Water Clim. Change, 11, 270-288, https://doi.org/10.2166/wcc.2020.166, 2020.
Klerk, W. J., Winsemius, H. C., Van Verseveld, W. J., Bakker, A. M. R., and Diermanse, F. L. M.: The co-incidence of storm surges and extreme discharges within the Rhine-Meuse Delta, Environ. Res. Lett., 10, 035005, https://doi.org/10.1088/1748-9326/10/3/035005, 2015.
Komendantova, N., Mrzyglocki, R., Mignan, A., Khazai, B., Wenzel, F., Patt, A., and Fleming, K.: Multi-hazard and multi-risk decision-support tools as a part of participatory risk governance: Feedback from civil protection stakeholders, Int. J. Disast. Risk Re., 8, 50–67, https://doi.org/10.1016/J.IJDRR.2013.12.006, 2014.
Koskinas, A., Tegos, A., Tsira, P., Dimitriadis, P., Iliopoulou, T., Papanicolaou, P., Koutsoyiannis, D., and Williamson, T.: Insights into the Oroville Dam 2017 Spillway Incident, https://doi.org/10.3390/geosciences9010037, 2019.
Kowalik, Z. and Proshutinsky, A.: Tsunami-tide interactions: A Cook Inlet case study, Cont. Shelf Res., 30, 633–642, https://doi.org/10.1016/j.csr.2009.10.004, 2010.
Kuleshov, Y., McGree, S., Jones, D., Charles, A., Cottrill, D., Prakash, B., Atalifo, T., Nihmei, S., Lagomauitumua, F., and Seuseu, S.: Extreme Weather and Climate Events and Their Impacts on Island Countries in the Western Pacific: Cyclones, Floods and Droughts, Atmos. Clim. Sci., 4, 803–818, https://doi.org/10.4236/acs.2014.45071, 2014.
Kumbier, K., Carvalho, R. C., Vafeidis, A. T., and Woodroffe, C. D.: Investigating compound flooding in an estuary using hydrodynamic modelling: a case study from the Shoalhaven River, Australia, Nat. Hazards Earth Syst. Sci., 18, 463–477, https://doi.org/10.5194/nhess-18-463-2018, 2018.
Kupfer, S., Santamaria-Aguilar, S., van Niekerk, L., Lück-Vogel, M., and Vafeidis, A. T.: Investigating the interaction of waves and river discharge during compound flooding at Breede Estuary, South Africa, Nat. Hazards Earth Syst. Sci., 22, 187–205, https://doi.org/10.5194/nhess-22-187-2022, 2022.
Kudryavtseva, N., Räämet, A., and Soomere, T.: Coastal Flooding: Joint Probability of Extreme Water Levels and Waves along the Baltic Sea Coast, J. Coastal Res., 95, 1146–1151, https://doi.org/10.2112/SI95-222.1, 2020.
Lai, Y., Li, J., Gu, X., Liu, C., and Chen, Y. D.: Global compound floods from precipitation and storm surge: Hazards and the roles of cyclones, J. Climate, 34, 8319–8339, https://doi.org/10.1175/JCLI-D-21-0050.1, 2021a.
Lai, Y., Li, Q., Li, J., Zhou, Q., Zhang, X., and Wu, G.: Evolution of Frequency and Intensity of Concurrent Heavy Precipitation and Storm Surge at the Global Scale: Implications for Compound Floods, Frontiers in Earth Science, 9, 660359, https://doi.org/10.3389/feart.2021.660359, 2021b.
Láng-Ritter, J., Berenguer, M., Dottori, F., Kalas, M., and Sempere-Torres, D.: Compound flood impact forecasting: integrating fluvial and flash flood impact assessments into a unified system, Hydrol. Earth Syst. Sci., 26, 689–709, https://doi.org/10.5194/hess-26-689-2022, 2022.
Lanzoni, S. and Seminara, G.: On tide propagation in convergent estuaries, J. Geophys. Res.-Oceans, 103, 30793–30812, https://doi.org/10.1029/1998JC900015, 1998.
Latif, S. and Mustafa, F.: Copula-based multivariate flood probability construction: a review, Arab. J. Geosci., 13, 132, https://doi.org/10.1007/s12517-020-5077-6, 2020.
Latif, S. and Simonovic, S. P.: Trivariate Joint Distribution Modelling of Compound Events Using the Nonparametric D-Vine Copula Developed Based on a Bernstein and Beta Kernel Copula Density Framework, Hydrology, 9, 221, https://doi.org/10.3390/hydrology9120221, 2022a.
Latif, S. and Simonovic, S. P.: Parametric Vine Copula Framework in the Trivariate Probability Analysis of Compound Flooding Events, Water, 14, 2214, https://doi.org/10.3390/W14142214, 2022b.
Latif, S. and Simonovic, S. P.: Compounding joint impact of rainfall, storm surge and river discharge on coastal flood risk: an approach based on 3D fully nested Archimedean copulas, Environ. Earth Sci., 82, 63, https://doi.org/10.1007/s12665-022-10719-9, 2023.
Lavigne, F., Paris, R., Grancher, D., Wassmer, P., Brunstein, D., Vautier, F., Leone, F., Flohic, F., De Coster, B., Gunawan, T., Gomez, C., Setiawan, A., Cahyadi, R., and Fachrizal: Reconstruction of Tsunami Inland Propagation on December 26, 2004 in Banda Aceh, Indonesia, through Field Investigations, Pure Appl. Geophys., 166, 259–281, https://doi.org/10.1007/s00024-008-0431-8, 2009.
Lawrence, D., Paquet, E., Gailhard, J., and Fleig, A. K.: Stochastic semi-continuous simulation for extreme flood estimation in catchments with combined rainfall–snowmelt flood regimes, Nat. Hazards Earth Syst. Sci., 14, 1283–1298, https://doi.org/10.5194/nhess-14-1283-2014, 2014.
Lee, C., Hwang, S., Do, K., and Son, S.: Increasing flood risk due to river runoff in the estuarine area during a storm landfall, Estuar. Coast. Shelf S., 221, 104–118, https://doi.org/10.1016/J.ECSS.2019.03.021, 2019.
Lee, S., Kang, T., Sun, D., and Park, J. J.: Enhancing an analysis method of compound flooding in coastal areas by linking flow simulation models of coasts and watershed, Sustainability, 12, 6572, https://doi.org/10.3390/SU12166572, 2020.
Leijnse, T., van Ormondt, M., Nederhoff, K., and van Dongeren, A.: Modeling compound flooding in coastal systems using a computationally efficient reduced-physics solver: Including fluvial, pluvial, tidal, wind- and wave-driven processes, Coast. Eng., 163, 103796, https://doi.org/10.1016/j.coastaleng.2020.103796, 2021.
Leonard, M., Westra, S., Phatak, A., Lambert, M., van den Hurk, B., McInnes, K., Risbey, J., Schuster, S., Jakob, D., and Stafford-Smith, M.: A compound event framework for understanding extreme impacts, Wires Clim. Change, 5, 113–128, https://doi.org/10.1002/WCC.252, 2014.
Leone, F., Lavigne, F., Paris, R., Denain, J.-C., and Vinet, F.: A spatial analysis of the December 26th, 2004 tsunami-induced damages: Lessons learned for a better risk assessment integrating buildings vulnerability, Appl. Geogr., 31, 363–375, https://doi.org/10.1016/j.apgeog.2010.07.009, 2011.
Lex, A., Gehlenborg, N., Strobelt, H., Vuillemot, R., and Pfister, H.: UpSet: Visualization of Intersecting Sets, IEEE T. Vis. Comput. Gr., 20, 1983–1992, https://doi.org/10.1109/TVCG.2014.2346248, 2014.
Li, L. and Jun, K. S.: The Joint Effect of River Flow and Tides on the Determination of Design Flood Levels in the Han River, J. Coastal Res., 95, 257–261, https://doi.org/10.2112/SI95-050.1, 2020.
Li, Y., Shen, P., Yan, Y., and Zhou, W.-H.: Flood risk assessment of artificial islands under compound rain-tide-wind effects during tropical cyclones, J. Hydrol., 615, 128736, https://doi.org/10.1016/J.JHYDROL.2022.128736, 2022.
Lian, J. J., Xu, K., and Ma, C.: Joint impact of rainfall and tidal level on flood risk in a coastal city with a complex river network: a case study of Fuzhou City, China, Hydrol. Earth Syst. Sci., 17, 679–689, https://doi.org/10.5194/hess-17-679-2013, 2013.
Lian, J., Xu, H., Xu, K., and Ma, C.: Optimal management of the flooding risk caused by the joint occurrence of extreme rainfall and high tide level in a coastal city, Nat. Hazards, 89, 183–200, https://doi.org/10.1007/s11069-017-2958-4, 2017.
Liang, H. and Zhou, X.: Impact of Tides and Surges on Fluvial Floods in Coastal Regions, Remote Sensing, 14, 5779–5779, https://doi.org/10.3390/RS14225779, 2022.
Lin, N., Smith, J. A., Villarini, G., Marchok, T. P., and Baeck, M. L.: Modeling extreme rainfall, winds, and surge from Hurricane Isabel (2003), Weather Forecast., 25, 1342–1361, https://doi.org/10.1175/2010WAF2222349.1, 2010.
Liu, Q., Xu, H., and Wang, J.: Assessing tropical cyclone compound flood risk using hydrodynamic modelling: a case study in Haikou City, China, Nat. Hazards Earth Syst. Sci., 22, 665–675, https://doi.org/10.5194/nhess-22-665-2022, 2022.
Loganathan, G. V., Kuo, C. Y., and Yannaccone, J.: Joint Probability Distribution of Streamflows and Tides in Estuaries, Hydrol. Res., 18, 237–246, https://doi.org/10.2166/NH.1987.0017, 1987.
Loveland, M., Kiaghadi, A., Dawson, C. N., Rifai, H. S., Misra, S., Mosser, H., and Parola, A.: Developing a Modeling Framework to Simulate Compound Flooding: When Storm Surge Interacts With Riverine Flow, Frontiers in Climate, 2, 609610, https://doi.org/10.3389/fclim.2020.609610, 2021.
Lu, W., Tang, L., Yang, D., Wu, H., and Liu, Z.: Compounding Effects of Fluvial Flooding and Storm Tides on Coastal Flooding Risk in the Coastal-Estuarine Region of Southeastern China, Atmosphere, 13, 238, https://doi.org/10.3390/ATMOS13020238, 2022.
Lucey, J. T. D. and Gallien, T. W.: Characterizing multivariate coastal flooding events in a semi-arid region: the implications of copula choice, sampling, and infrastructure, Nat. Hazards Earth Syst. Sci., 22, 2145–2167, https://doi.org/10.5194/nhess-22-2145-2022, 2022.
Lyddon, C., Robins, P., Lewis, M., Barkwith, A., Vasilopoulos, G., Haigh, I., and Coulthard, T.: Historic Spatial Patterns of Storm-Driven Compound Events in UK Estuaries, Estuar. Coast., 30, 30–56, https://doi.org/10.1007/S12237-022-01115-4, 2022.
Manneela, S. and Kumar, S.: Overview of the Hunga Tonga-Hunga Ha'apai Volcanic Eruption and Tsunami, J. Geol. Soc. India, 98, 299–304, https://doi.org/10.1007/s12594-022-1980-7, 2022.
Manoj J, A., Guntu, R. K., and Agarwal, A.: Spatiotemporal dependence of soil moisture and precipitation over India, J. Hydrol., 610, 127898–127898, https://doi.org/10.1016/J.JHYDROL.2022.127898, 2022.
Mantz, P. A. and Wakeling, H. L.: Forecasting Flood Levels for Joint Events of Rainfall and Tidal Surge Flooding using Extreme Values Statistics, P. Civil Eng., 67, 31–50, https://doi.org/10.1680/iicep.1979.2315, 1979.
Mark, O., Weesakul, S., Apirumanekul, C., Aroonnet, S. B., and Djordjeviæ, S.: Potential and limitations of 1D modelling of urban flooding, J. Hydrol., 299, 284–299, https://doi.org/10.1016/j.jhydrol.2004.08.014, 2004.
Martyr, R. C., Dietrich, J. C., Westerink, J. J., Kerr, P. C., Dawson, C., Smith, J. M., Pourtaheri, H., Powell, N., Ledden, M. V., Tanaka, S., Roberts, H. J., Westerink, H. J., and Westerink, L. G.: Simulating Hurricane Storm Surge in the Lower Mississippi River under Varying Flow Conditions, J. Hydraul. Eng., 139, 492–501, https://doi.org/10.1061/(ASCE)HY.1943-7900.0000699, 2013.
Mashriqui, H. S., Halgren, J. S., and Reed, S. M.: 1D River Hydraulic Model for Operational Flood Forecasting in the Tidal Potomac: Evaluation for Freshwater, Tidal, and Wind-Driven Events, J. Hydraul. Eng., 140, 04014005, https://doi.org/10.1061/(ASCE)HY.1943-7900.0000862, 2014.
Mashriqui, H. S., Reed, S., and Aschwanden, C.: Toward Modeling of River-Estuary-Ocean Interactions to Enhance Operational River Forecasting in the NOAA National Weather Service, 2nd Joint Federal Interagency Conference, Las Vegas, NV, https://hdsc.nws.noaa.gov/pub/hdsc/data/papers/articles/HRL_Pubs_PDF_May12_2009/New_Scans_December_2010/11F_Mashriqui_12_31_09.pdf (last access: 17 July 2024), 2010.
Masina, M., Lamberti, A., and Archetti, R.: Coastal flooding: A copula based approach for estimating the joint probability of water levels and waves, Coast. Eng., 97, 37–52, https://doi.org/10.1016/J.COASTALENG.2014.12.010, 2015.
Maskell, J., Horsburgh, K., Lewis, M., and Bates, P.: Investigating River–Surge Interaction in Idealised Estuaries, J. Coastal Res., 30, 248–259, https://doi.org/10.2112/JCOASTRES-D-12-00221.1, 2014.
Maymandi, N., Hummel, M. A., and Zhang, Y.: Compound Coastal, Fluvial, and Pluvial Flooding During Historical Hurricane Events in the Sabine–Neches Estuary, Texas, Water Resour. Res., 58, e2022WR033144, https://doi.org/10.1029/2022WR033144, 2022.
Mazas, F., Kergadallan, X., Garat, P., and Hamm, L.: Applying POT methods to the Revised Joint Probability Method for determining extreme sea levels, Coast. Eng., 91, 140–150, https://doi.org/10.1016/j.coastaleng.2014.05.006, 2014.
McGuigan, K., Webster, T., and Collins, K.: A Flood Risk Assessment of the LaHave River Watershed, Canada Using GIS Techniques and an Unstructured Grid Combined River-Coastal Hydrodynamic Model, Journal of Marine Science and Engineering, 3, 1093–1116, https://doi.org/10.3390/jmse3031093, 2015.
McInnes, K. L., Hubbert, G. D., Abbs, D. J., and Oliver, S. E.: A numerical modelling study of coastal flooding, Meteorol. Atmos. Phys., 80, 217–233, https://doi.org/10.1007/S007030200027, 2002.
Melone, A. M.: Flood Producing Mechanisms in Coastal British Columbia, Can. Water Resour. J., 10, 46–64, https://doi.org/10.4296/cwrj1003046, 1985.
Merz, B., Kuhlicke, C., Kunz, M., Pittore, M., Babeyko, A., Bresch, D. N., Domeisen, D. I. V., Feser, F., Koszalka, I., Kreibich, H., Pantillon, F., Parolai, S., Pinto, J. G., Punge, H. J., Rivalta, E., Schröter, K., Strehlow, K., Weisse, R., and Wurpts, A.: Impact Forecasting to Support Emergency Management of Natural Hazards, Rev. Geophys., 58, e2020RG000704, https://doi.org/10.1029/2020RG000704, 2020.
Meyers, S. D., Landry, S., Beck, M. W., and Luther, M. E.: Using logistic regression to model the risk of sewer overflows triggered by compound flooding with application to sea level rise, Urban Clim., 35, 100752, https://doi.org/10.1016/j.uclim.2020.100752, 2021.
Ming, X., Liang, Q., Dawson, R., Xia, X., and Hou, J.: A quantitative multi-hazard risk assessment framework for compound flooding considering hazard inter-dependencies and interactions, J. Hydrol., 607, 127477, https://doi.org/10.1016/j.jhydrol.2022.127477, 2022.
Mishra, A., Alnahit, A., and Campbell, B.: Impact of land uses, drought, flood, wildfire, and cascading events on water quality and microbial communities: A review and analysis, J. Hydrol., 596, 125707, https://doi.org/10.1016/j.jhydrol.2020.125707, 2021.
Mishra, A., Mukherjee, S., Merz, B., Singh, V. P., Wright, D. B., Villarini, G., Paul, S., Kumar, D. N., Khedun, C. P., Niyogi, D., Schumann, G., and Stedinger, J. R.: An Overview of Flood Concepts, Challenges, and Future Directions, J. Hydrol. Eng., 27, 03122001, https://doi.org/10.1061/(ASCE)HE.1943-5584.0002164, 2022.
Modrakowski, L.-C., Su, J., and Nielsen, A. B.: The Precautionary Principles of the Potential Risks of Compound Events in Danish Municipalities, Frontiers in Climate, 3, 772629, https://doi.org/10.3389/fclim.2021.772629, 2022.
Moftakhari, H., Schubert, J. E., AghaKouchak, A., Matthew, R. A., and Sanders, B. F.: Linking statistical and hydrodynamic modeling for compound flood hazard assessment in tidal channels and estuaries, Adv. Water Resour., 128, 28–38, https://doi.org/10.1016/j.advwatres.2019.04.009, 2019.
Moftakhari, H. R., Salvadori, G., AghaKouchak, A., Sanders, B. F., and Matthew, R. A.: Compounding effects of sea level rise and fluvial flooding, P. Natl. Acad. Sci. USA, 114, 9785–9790, https://doi.org/10.1073/PNAS.1620325114, 2017.
Mohammadi, S., Bensi, M., Kao, S.-C., and Deneale, S. T.: Multi-Mechanism Flood Hazard Assessment: Example Use Case Studies, https://doi.org/10.2172/1637939, 2021.
Mohanty, M. P., Sherly, M. A., Ghosh, S., and Karmakar, S.: Tide-rainfall flood quotient: an incisive measure of comprehending a region's response to storm-tide and pluvial flooding, Environ. Res. Lett., 15, 064029, https://doi.org/10.1088/1748-9326/ab8092, 2020.
Munich Re: Topics Geo natural catastrophes 2016: analyses, assessments, positions, Munich Reinsurance, https://www.preventionweb.net/publication/topics-geo-natural-catastrophes-2016-analyses-assessments-positions (last access: 17 July 2024), 2017.
Muñoz, D. F., Moftakhari, H., and Moradkhani, H.: Compound Effects of Flood Drivers and Wetland Elevation Correction on Coastal Flood Hazard Assessment, Water Resour. Res., 56, e2020WR027544, https://doi.org/10.1029/2020WR027544, 2020.
Muñoz, D. F., Muñoz, P., Moftakhari, H., and Moradkhani, H.: From local to regional compound flood mapping with deep learning and data fusion techniques, Sci. Total Environ., 782, 146927, https://doi.org/10.1016/j.scitotenv.2021.146927, 2021.
Muñoz, D. F., Abbaszadeh, P., Moftakhari, H., and Moradkhani, H.: Accounting for uncertainties in compound flood hazard assessment: The value of data assimilation, Coast. Eng., 171, 104057, https://doi.org/10.1016/j.coastaleng.2021.104057, 2022a.
Muñoz, D. F., Moftakhari, H., Kumar, M., and Moradkhani, H.: Compound Effects of Flood Drivers, Sea Level Rise, and Dredging Protocols on Vessel Navigability and Wetland Inundation Dynamics, Frontiers in Marine Science, 9, 906376, https://doi.org/10.3389/fmars.2022.906376, 2022b.
Myers, V. A.: Joint probability method of tide frequency analysis applied to Atlantic City and Long Beach Island, N.J, Environmental Sciences Services Administration (ESSA), https://doi.org/10.7282/T3ZK5DVQ, 1970.
Najafi, M. R., Zhang, Y., and Martyn, N.: A flood risk assessment framework for interdependent infrastructure systems in coastal environments, Sustain. Cities Soc., 64, 102516, https://doi.org/10.1016/j.scs.2020.102516, 2021.
Naseri, K. and Hummel, M. A.: A Bayesian copula-based nonstationary framework for compound flood risk assessment along US coastlines, J. Hydrol., 610, 128005, https://doi.org/10.1016/j.jhydrol.2022.128005, 2022.
Nash, S., Comer, J., Olbert, A., and Hartnett, M.: High Resolution Urban Flood Modelling: A Case Study of Cork City, Ireland, EPiC Series in Engineering, 3, 1495–1504, https://doi.org/10.29007/RZWJ, 2018.
Nasr, A. A., Wahl, T., Rashid, M. M., Camus, P., and Haigh, I. D.: Assessing the dependence structure between oceanographic, fluvial, and pluvial flooding drivers along the United States coastline, Hydrol. Earth Syst. Sci., 25, 6203–6222, https://doi.org/10.5194/hess-25-6203-2021, 2021.
NCEI: U.S. Billion-Dollar Weather and Climate Disasters, National Centers for Environmental Information (NCEI) [data set], https://doi.org/10.25921/stkw-7w73, 2023.
Neumann, B., Vafeidis, A. T., Zimmermann, J., and Nicholls, R. J.: Future Coastal Population Growth and Exposure to Sea-Level Rise and Coastal Flooding – A Global Assessment, PLOS ONE, 10, e0118571, https://doi.org/10.1371/journal.pone.0118571, 2015.
Olabarrieta, M., Warner, J. C., Armstrong, B., Zambon, J. B., and He, R.: Ocean–atmosphere dynamics during Hurricane Ida and Nor’Ida: An application of the coupled ocean–atmosphere–wave–sediment transport (COAWST) modeling system, Ocean Model., 43–44, 112–137, https://doi.org/10.1016/j.ocemod.2011.12.008, 2012.
Olbert, A. I., Nash, S., Cunnane, C., and Hartnett, M.: Tide–surge interactions and their effects on total sea levels in Irish coastal waters, Ocean Dynam., 63, 599–614, https://doi.org/10.1007/s10236-013-0618-0, 2013.
Olbert, A. I., Comer, J., Nash, S., and Hartnett, M.: High-resolution multi-scale modelling of coastal flooding due to tides, storm surges and rivers inflows. A Cork City example, Coast. Eng., 121, 278–296, https://doi.org/10.1016/J.COASTALENG.2016.12.006, 2017.
Oppenheimer, M., Glavovic, B., Hinkel, J., van de Wal, R., Magnan, A., Abd-EIgawad, A., Cai, R., Cifuentes-Jara, M., DeConto, R., Ghosh, T., Hay, J., Isla, F., Marzeion, B., Meyssignac, B., and Sebesvari, Z.: Sea Level Rise and Implications for Low-Lying Islands, Coasts and Communities, Intergovernmental Panel on Climate Change, Geneva, 321–446, https://doi.org/10.1017/9781009157964.006, 2019.
Orton, P., Georgas, N., Blumberg, A., and Pullen, J.: Detailed modeling of recent severe storm tides in estuaries of the New York City region, J. Geophys. Res.-Oceans, 117, C09030, https://doi.org/10.1029/2012JC008220, 2012.
Orton, P., Conticello, F., Cioffi, F., Hall, T., Georgas, N., Lall, U., Blumberg, A., Conticello, F., Cioffi, F., Hall, T., Georgas, N., Lall, U., and Blumberg, A.: Hazard Assessment from Storm Tides and Rainfall on a Tidal River Estuary, 36th IAHR World Congress, The Hague, Netherlands, 1–10, https://ntrs.nasa.gov/api/citations/20150021054/downloads/20150021054.pdf (last access: 17 July 2024), 2015.
Orton, P. M., Hall, T. M., Talke, S. A., Blumberg, A. F., Georgas, N., and Vinogradov, S.: A validated tropical-extratropical flood hazard assessment for New York Harbor, J. Geophys. Res.-Oceans, 121, 8904–8929, https://doi.org/10.1002/2016JC011679, 2016.
Orton, P. M., Conticello, F. R., Cioffi, F., Hall, T. M., Georgas, N., Lall, U., Blumberg, A. F., and MacManus, K.: Flood hazard assessment from storm tides, rain and sea level rise for a tidal river estuary, Nat. Hazards, 102, 729–757, https://doi.org/10.1007/S11069-018-3251-X, 2018.
Page, M. J., McKenzie, J. E., Bossuyt, P. M., Boutron, I., Hoffmann, T. C., Mulrow, C. D., Shamseer, L., Tetzlaff, J. M., Akl, E. A., Brennan, S. E., Chou, R., Glanville, J., Grimshaw, J. M., Hróbjartsson, A., Lalu, M. M., Li, T., Loder, E. W., Mayo-Wilson, E., McDonald, S., McGuinness, L. A., Stewart, L. A., Thomas, J., Tricco, A. C., Welch, V. A., Whiting, P., and Moher, D.: The PRISMA 2020 statement: an updated guideline for reporting systematic reviews, BMJ, 372, n71, https://doi.org/10.1136/bmj.n71, 2021.
Pandey, S., Rao, A. D., and Haldar, R.: Modeling of Coastal Inundation in Response to a Tropical Cyclone Using a Coupled Hydraulic HEC-RAS and ADCIRC Model, J. Geophys. Res.-Oceans, 126, e2020JC016810, https://doi.org/10.1029/2020JC016810, 2021.
Paprotny, D., Vousdoukas, M. I., Morales-Nápoles, O., Jonkman, S. N., and Feyen, L.: Pan-European hydrodynamic models and their ability to identify compound floods, Nat. Hazards, 101, 933–957, https://doi.org/10.1007/S11069-020-03902-3, 2020.
Park, G. H., Kim, I. C., Suh, K. S., and Lee, J. L.: Prediction of Storm Surge and Runoff Combined Inundation, J. Coast. Res., Proceedings of the 11th International Coastal Symposium, Szczecin, Poland, 1150–1154, https://www.jstor.org/stable/pdf/26482354.pdf (last access: 17 July 2024), 2011.
Pasquier, U., He, Y., Hooton, S., Goulden, M., and Hiscock, K. M.: An integrated 1D–2D hydraulic modelling approach to assess the sensitivity of a coastal region to compound flooding hazard under climate change, Nat. Hazards, 98, 915–937, https://doi.org/10.1007/S11069-018-3462-1, 2019.
Peña, F., Nardi, F., Melesse, A., Obeysekera, J., Castelli, F., Price, R. M., Crowl, T., and Gonzalez-Ramirez, N.: Compound flood modeling framework for surface–subsurface water interactions, Nat. Hazards Earth Syst. Sci., 22, 775–793, https://doi.org/10.5194/nhess-22-775-2022, 2022.
Pescaroli, G. and Alexander, D.: A definition of cascading disasters and cascading effects: Going beyond the “toppling dominos” metaphor, Global Risk Forum GRF Davos, Planet@Risk, 2, 58–67, https://discovery.ucl.ac.uk/id/eprint/1465510/ (last access: 17 July 2024), 2015.
Pescaroli, G. and Alexander, D.: Understanding Compound, Interconnected, Interacting, and Cascading Risks: A Holistic Framework, Risk Anal., 38, 2245–2257, https://doi.org/10.1111/RISA.13128, 2018.
Petroliagkis, T. I.: Estimations of statistical dependence as joint return period modulator of compound events – Part 1: Storm surge and wave height, Nat. Hazards Earth Syst. Sci., 18, 1937–1955, https://doi.org/10.5194/nhess-18-1937-2018, 2018.
Petroliagkis, T. I., Voukouvalas, E., Disperati, J., Bidlot, J., and European Commission. Joint Research Center: Joint Probabilities of Storm Surge, Significant Wave Height and River Discharge Components of Coastal Flooding Events. Utilising statistical dependence methodologies & techniques, Publications Office of the European Union, ISBN 978-92-79-57666-9, https://doi.org/10.2788/951583, 2016.
Phillips, M.: The Dynamics of the Upper Ocean, J. Fluid Mech., 29, 261, https://doi.org/10.1017/S0022112067211193, 1966.
Phillips, R. C., Samadi, S., Hitchcock, D. B., Meadows, M. E., and Wilson, C. A. M. E.: The Devil Is in the Tail Dependence: An Assessment of Multivariate Copula-Based Frameworks and Dependence Concepts for Coastal Compound Flood Dynamics, Earth's Future, 10, e2022EF002705, https://doi.org/10.1029/2022EF002705, 2022.
Pickering, M. D., Horsburgh, K. J., Blundell, J. R., Hirschi, J. J. M., Nicholls, R. J., Verlaan, M., and Wells, N. C.: The impact of future sea-level rise on the global tides, Cont. Shelf Res., 142, 50–68, https://doi.org/10.1016/j.csr.2017.02.004, 2017.
Piecuch, C. G., Coats, S., Dangendorf, S., Landerer, F. W., Reager, J. T., Thompson, P. R., and Wahl, T.: High-Tide Floods and Storm Surges During Atmospheric Rivers on the US West Coast, Geophys. Res. Lett., 49, e2021GL096820, https://doi.org/10.1029/2021GL096820, 2022.
Pietrafesa, L. J., Zhang, H., Bao, S., Gayes, P. T., and Hallstrom, J. O.: Coastal flooding and inundation and inland flooding due to downstream blocking, Journal of Marine Science and Engineering, 7, 336, https://doi.org/10.3390/JMSE7100336, 2019.
Plane, E., Hill, K., and May, C.: A Rapid Assessment Method to Identify Potential Groundwater Flooding Hotspots as Sea Levels Rise in Coastal Cities, https://doi.org/10.3390/w11112228, 2019.
Poulos, S., Karditsa, A., Hatzaki, M., Tsapanou, A., Papapostolou, C., and Chouvardas, K.: An Insight into the Factors Controlling Delta Flood Events: The Case of the Evros River Deltaic Plain (NE Aegean Sea), Water, 14, 497, https://doi.org/10.3390/W14030497, 2022.
Prandle, D.: Storm surges in the southern North Sea and River Thames, P. R. Soc. Lond. A., 344, 509–539, https://doi.org/10.1098/rspa.1975.0117, 1975.
Prandle, D. and Wolf, J.: The interaction of surge and tide in the North Sea and River Thames, Geophys. J. Int., 55, 203–216, https://doi.org/10.1111/j.1365-246X.1978.tb04758.x, 1978.
Preisser, M., Passalacqua, P., Bixler, R. P., and Hofmann, J.: Intersecting near-real time fluvial and pluvial inundation estimates with sociodemographic vulnerability to quantify a household flood impact index, Hydrology and Earth System Sciences, 26, 3941-3964, https://doi.org/10.5194/hess-26-3941-2022, 2022.
Pugh, D. T.: Tides, surges and mean sea-level, John Wiley & Sons Ltd, Chichester, 472 pp.1987.
Pugh, D. T. and Vassie, J. M.: Applications of the Joint Probability Method for Extreme Sea Level Computations, Proceedings of the Institution of Civil Engineers, 69, 959-975, https://doi.org/10.1680/iicep.1980.2179, 1980.
Qiang, Y., He, J., Xiao, T., Lu, W., Li, J., and Zhang, L.: Coastal town flooding upon compound rainfall-wave overtopping-storm surge during extreme tropical cyclones in Hong Kong, Journal of Hydrology: Regional Studies, 37, https://doi.org/10.1016/j.ejrh.2021.100890, 2021.
Qiu, J., Liu, B., Yang, F., Wang, X., and He, X.: Quantitative Stress Test of Compound Coastal-Fluvial Floods in China's Pearl River Delta, Earth's Future, 10, e2021EF002638, https://doi.org/10.1029/2021EF002638, 2022.
Quagliolo, C., Comino, E., and Pezzoli, A.: Experimental Flash Floods Assessment Through Urban Flood Risk Mitigation (UFRM) Model: The Case Study of Ligurian Coastal Cities, Front. Water, 3, 663378, https://doi.org/10.3389/FRWA.2021.663378, 2021.
Rahimi, R., Tavakol-Davani, H., Graves, C., Gomez, A., and Valipour, M. F.: Compound inundation impacts of coastal climate change: Sea-level rise, groundwater rise, and coastal precipitation, Water, 12, https://doi.org/10.3390/W12102776, 2020.
Ray, T., Asce, M., Stepinski, E., Sebastian, A., Bedient, P. B., and Asce, F.: Dynamic Modeling of Storm Surge and Inland Flooding in a Texas Coastal Floodplain, J. Hydraul. Eng., 137, 1103–1110, https://doi.org/10.1061/(ASCE)HY.1943-7900.0000398, 2011.
Raymond, C., Horton, R. M., Zscheischler, J., Martius, O., AghaKouchak, A., Balch, J., Bowen, S. G., Camargo, S. J., Hess, J., Kornhuber, K., Oppenheimer, M., Ruane, A. C., Wahl, T., and White, K.: Understanding and managing connected extreme events, Nature Climate Change, 10, 611-621, https://doi.org/10.1038/s41558-020-0790-4, 2020.
Razmi, A., Mardani-Fard, H. A., Golian, S., and Zahmatkesh, Z.: Time-Varying Univariate and Bivariate Frequency Analysis of Nonstationary Extreme Sea Level for New York City, Environ. Process., 9, 8, https://doi.org/10.1007/S40710-021-00553-9, 2022.
Reimann, L., Vafeidis, A. T., and Honsel, L. E.: Population development as a driver of coastal risk: Current trends and future pathways, Cambridge Prisms: Coastal Futures, 1, e14, https://doi.org/10.1017/cft.2023.3, 2023.
ReliefWeb: South America: Floods and Landslides – Nov 2015–Dec 2016, UN Office for the Coordination of Humanitarian Affairs, https://reliefweb.int/disaster/fl-2015-000171-pry (last access: 17 July 2024), 2016.
ReliefWeb: South America: Floods and Landslides – Dec 2016, UN Office for the Coordination of Humanitarian Affairs, https://reliefweb.int/disaster/fl-2016-000137-arg (last access: 17 July 2024), 2017.
ReliefWeb: Libya: Flood update Flash Update No. 3 September 16 2023, UN Office for the Coordination of Humanitarian Affairs, https://reliefweb.int/report/libya/libya-flood-update-flash-update-no3-16-september-2023-5pm-local-time (last access: 17 July 2024), 2023.
Rentschler, J., Salhab, M., and Jafino, B. A.: Flood exposure and poverty in 188 countries, Nat. Commun., 13, 3527, https://doi.org/10.1038/s41467-022-30727-4, 2022.
Ridder, N., De Vries, H., and Drijfhout, S.: The role of atmospheric rivers in compound events consisting of heavy precipitation and high storm surges along the Dutch coast, Natural Hazards and Earth System Sciences, 18, 3311-3326, https://doi.org/10.5194/NHESS-18-3311-2018, 2018.
Ridder, N. N., Pitman, A. J., Westra, S., Ukkola, A., Hong, X. D., Bador, M., Hirsch, A. L., Evans, J. P., Di Luca, A., and Zscheischler, J.: Global hotspots for the occurrence of compound events, Nature Communications, 11, 1–10, https://doi.org/10.1038/s41467-020-19639-3, 2020.
Robins, P. E., Davies, A. G., and Jones, R.: Application of a coastal model to simulate present and future inundation and aid coastal management, J. Coast. Conserv., 15, 1–14, https://doi.org/10.1007/S11852-010-0113-4, 2011.
Robins, P. E., Lewis, M. J., Elnahrawi, M., Lyddon, C., Dickson, N., and Coulthard, T. J.: Compound Flooding: Dependence at Sub-daily Scales Between Extreme Storm Surge and Fluvial Flow, Frontiers in Built Environment, 7, https://doi.org/10.3389/fbuil.2021.727294, 2021.
Rodríguez, G., Nistal, A., and Pérez, B.: Joint occurrence of high tide, surge and storm-waves on the northwest Spanish coast, Boletín-Instituto Español de Oceanografía, 1999.
Rossiter, J. R.: Interaction Between Tide and Surge in the Thames, Geophysical Journal International, 6, 29-53, https://doi.org/10.1111/j.1365-246X.1961.tb02960.x, 1961.
Rozell, D. J.: Overestimating coastal urban resilience: The groundwater problem, Cities, 118, 103369, https://doi.org/10.1016/j.cities.2021.103369, 2021.
Rueda, A., Camus, P., Tomás, A., Vitousek, S., and Méndez, F. J.: A multivariate extreme wave and storm surge climate emulator based on weather patterns, Ocean Modelling, 104, 242-251, https://doi.org/10.1016/J.OCEMOD.2016.06.008, 2016.
Ruggiero, P., Serafin, K., Parker, K., and Hill, D.: Assessing the Impacts of Coastal Flooding on Treaty of Olympia Infrastructure: A report to the Quinault Indian Nation, Hoh Tribe, and Quileute Tribe, Corvallis, Oregon, https://www.cakex.org/sites/default/files/documents/QTAII_Report_Final_withAppendices.pdf (last access: 17 July 2024), 2019.
Sadegh, M., Moftakhari, H., Gupta, H. V., Ragno, E., Mazdiyasni, O., Sanders, B., Matthew, R., and AghaKouchak, A.: Multihazard Scenarios for Analysis of Compound Extreme Events, Geophys. Res. Lett., 45, 5470-5480, https://doi.org/10.1029/2018GL077317, 2018.
Saharia, A. M., Zhu, Z., and Atkinson, J. F.: Compound flooding from lake seiche and river flow in a freshwater coastal river, J. Hydrol., 603, https://doi.org/10.1016/j.jhydrol.2021.126969, 2021.
Saleh, F., Ramaswamy, V., Wang, Y., Georgas, N., Blumberg, A., and Pullen, J.: A multi-scale ensemble-based framework for forecasting compound coastal-riverine flooding: The Hackensack-Passaic watershed and Newark Bay, Advances in Water Resources, 110, 371-386, https://doi.org/10.1016/j.advwatres.2017.10.026, 2017.
Salvadori, G. and De Michele, C.: Frequency analysis via copulas: Theoretical aspects and applications to hydrological events, Water Resour. Res., 40, https://doi.org/10.1029/2004WR003133, 2004.
Salvadori, G. and De Michele, C.: On the Use of Copulas in Hydrology: Theory and Practice, Journal of Hydrologic Engineering, 12, 369-380, https://doi.org/10.1061/(ASCE)1084-0699(2007)12:4(369), 2007.
Sampurno, J., Vallaeys, V., Ardianto, R., and Hanert, E.: Integrated hydrodynamic and machine learning models for compound flooding prediction in a data-scarce estuarine delta, Nonlinear Processes in Geophysics, 29, 301-315, https://doi.org/10.5194/npg-29-301-2022, 2022a.
Sampurno, J., Vallaeys, V., Ardianto, R., and Hanert, E.: Modeling interactions between tides, storm surges, and river discharges in the Kapuas River delta, Biogeosciences, 19, 2741-2757, https://doi.org/10.5194/bg-19-2741-2022, 2022b.
Sangsefidi, Y., Bagheri, K., Davani, H., and Merrifield, M.: Vulnerability of coastal drainage infrastructure to compound flooding under climate change, J. Hydrol., 128823-128823, https://doi.org/10.1016/J.JHYDROL.2022.128823, 2022.
Santiago-Collazo, F. L., Bilskie, M. V., and Hagen, S. C.: A comprehensive review of compound inundation models in low-gradient coastal watersheds, Environmental Modelling and Software, 119, 166-181, https://doi.org/10.1016/j.envsoft.2019.06.002, 2019.
Santiago-Collazo, F. L., Bilskie, M. V., Bacopoulos, P., and Hagen, S. C.: An Examination of Compound Flood Hazard Zones for Past, Present, and Future Low-Gradient Coastal Land-Margins, Frontiers in Climate, 3, 684035, https://doi.org/10.3389/FCLIM.2021.684035, 2021.
Santos, V. M., Haigh, I. D., and Wahl, T.: Spatial and temporal clustering analysis of extreme wave events around the UK coastline, Journal of Marine Science and Engineering, 5, https://doi.org/10.3390/JMSE5030028, 2017.
Santos, V. M., Wahl, T., Jane, R., Misra, S. K., and White, K. D.: Assessing compound flooding potential with multivariate statistical models in a complex estuarine system under data constraints, Journal of Flood Risk Management, 14, https://doi.org/10.1111/JFR3.12749, 2021a.
Santos, V. M., Casas-Prat, M., Poschlod, B., Ragno, E., Van Den Hurk, B., Hao, Z., Kalmár, T., Zhu, L., and Najafi, H.: Statistical modelling and climate variability of compound surge and precipitation events in a managed water system: A case study in the Netherlands, Hydrology and Earth System Sciences, 25, 3595-3615, https://doi.org/10.5194/HESS-25-3595-2021, 2021b.
Sarewitz, D. and Pielke, R.: Extreme Events: A Research and Policy Framework for Disasters in Context, International Geology Review, 43, 406-418, https://doi.org/10.1080/00206810109465022, 2001.
Schaake, J. C., Hamill, T. M., Buizza, R., and Clark, M.: HEPEX: The Hydrological Ensemble Prediction Experiment, Bulletin of the American Meteorological Society, 88, 1541-1548, https://doi.org/10.1175/BAMS-88-10-1541, 2007.
Sebastian, A.: Chapter 7 – Compound Flooding, in: Coastal Flood Risk Reduction: The Netherlands and the U.S. Upper Texas Coast, Elsevier, 77-88, https://doi.org/10.1016/B978-0-323-85251-7.00007-X, 2022.
Seneviratne, S. I., Nicholls, N., Easterling, D., Goodess, C. M., Kanae, S., Kossin, J., Luo, Y., Marengo, J., Mc Innes, K., Rahimi, M., Reichstein, M., Sorteberg, A., Vera, C., Zhang, X., Rusticucci, M., Semenov, V., Alexander, L. V., Allen, S., Benito, G., Cavazos, T., Clague, J., Conway, D., Della-Marta, P. M., Gerber, M., Gong, S., Goswami, B. N., Hemer, M., Huggel, C., Van den Hurk, B., Kharin, V. V., Kitoh, A., Klein Tank, A. M. G., Li, G., Mason, S., Mc Guire, W., Van Oldenborgh, G. J., Orlowsky, B., Smith, S., Thiaw, W., Velegrakis, A., Yiou, P., Zhang, T., Zhou, T., and Zwiers, F. W.: Changes in Climate Extremes and their Impacts on the Natural Physical Environment, Cambridge University Press, Cambridge, United Kingdom, New York, USA9781139177245, 109-230, https://doi.org/10.1017/CBO9781139177245.006, 2012.
Serafin, K. A. and Ruggiero, P.: Simulating extreme total water levels using a time-dependent, extreme value approach, Journal of Geophysical Research: Oceans, 119, 6305-6329, https://doi.org/10.1002/2014JC010093, 2014.
Serafin, K. A., Ruggiero, P., Parker, K., and Hill, D. F.: What's streamflow got to do with it? A probabilistic simulation of the competing oceanographic and fluvial processes driving extreme along-river water levels, Natural Hazards and Earth System Sciences, 19, 1415-1431, https://doi.org/10.5194/NHESS-19-1415-2019, 2019.
Shahapure, S. S., Eldho, T. I., and Rao, E. P.: Coastal Urban Flood Simulation Using FEM, GIS and Remote Sensing, Water Resources Management, 24, 3615-3640, https://doi.org/10.1007/s11269-010-9623-y, 2010.
Shen, Y., Morsy, M. M., Huxley, C., Tahvildari, N., and Goodall, J. L.: Flood risk assessment and increased resilience for coastal urban watersheds under the combined impact of storm tide and heavy rainfall, J. Hydrol., 579, 124159-124159, https://doi.org/10.1016/J.JHYDROL.2019.124159, 2019.
Shen, Y., Tahvildari, N., Morsy, M. M., Huxley, C., Chen, T. D., and Goodall, J. L.: Dynamic Modeling of Inland Flooding and Storm Surge on Coastal Cities under Climate Change Scenarios: Transportation Infrastructure Impacts in Norfolk, Virginia USA as a Case Study, https://doi.org/10.3390/geosciences12060224, 2022.
Sheng, Y. P., Paramygin, V. A., Yang, K., and Rivera-Nieves, A. A.: A sensitivity study of rising compound coastal inundation over large flood plains in a changing climate, Scientific Reports, 12, https://doi.org/10.1038/s41598-022-07010-z, 2022.
Shi, S., Yang, B., and Jiang, W.: Numerical simulations of compound flooding caused by storm surge and heavy rain with the presence of urban drainage system, coastal dam and tide gates: A case study of Xiangshan, China, Coastal Engineering, 172, https://doi.org/10.1016/j.coastaleng.2021.104064, 2022.
Shields, G., Olsen, R., Medina, M., Obeysekera, J., Ganguli, P., DeMichele, C., Salvadori, G., Najafi Mohammad, R., Moftakhari, H., Diermanse, F., and AghaKouchak, A.: Compound Flooding in a Non-Stationary World: A Primer for Practice, in: ASCE Inspire 2023, Proceedings, 18–27, https://doi.org/10.1061/9780784485163.003, 2023.
Silva-Araya, W. F., Santiago-Collazo, F. L., Gonzalez-Lopez, J., and Maldonado-Maldonado, J.: Dynamic Modeling of Surface Runoff and Storm Surge during Hurricane and Tropical Storm Events, Hydrology, 5, 13–13, https://doi.org/10.3390/HYDROLOGY5010013, 2018.
Simmonds, R., White, C. J., Douglas, J., Sauter, C., and Brett, L.: A review of interacting natural hazards and cascading impacts in Scotland Research funded by the National Centre for Resilience, https://eprints.gla.ac.uk/267515/ (last access: 17 July 2024), 2022.
Skinner, C. J., Coulthard, T. J., Parsons, D. R., Ramirez, J. A., Mullen, L., and Manson, S.: Simulating tidal and storm surge hydraulics with a simple 2D inertia based model, in the Humber Estuary, U.K, Estuarine, Coastal and Shelf Science, 155, 126–136, https://doi.org/10.1016/J.ECSS.2015.01.019, 2015.
Sklar, M.: Fonctions de répartition à n dimensions et leurs marges, Annales de l'ISUP, 229–231, https://hal.science/hal-04094463 (last access: 17 July 2024), 1959.
Sopelana, J., Cea, L., and Ruano, S.: A continuous simulation approach for the estimation of extreme flood inundation in coastal river reaches affected by meso- and macrotides, Nat. Hazards, 93, 1337–1358, https://doi.org/10.1007/S11069-018-3360-6, 2018.
Stamey, B., Smith, W., Carey, K., Garbin, D., Klein, F., Wang, H., Shen, J., Gong, W., Cho, J., Forrest, D., Friedrichs, C., Boicourt, W., Li, M., Koterba, M., King, D., Titlow, J., Smith, E., Siebers, A., Billet, J., Lee, J., Manning, D., Szatkowski, G., Wilson, D., Ahnert, P., and Ostrowski, J.: Chesapeake Inundation Prediction System (CIPS): A regional prototype for a national problem, Oceans 2007, Vancouver, https://doi.org/10.1109/OCEANS.2007.4449222, 2007.
Stein, L., Pianosi, F., and Woods, R.: Event-based classification for global study of river flood generating processes, Hydrol. Process., 34, 1514–1529, https://doi.org/10.1002/hyp.13678, 2019.
Steinschneider, S.: A hierarchical Bayesian model of storm surge and total water levels across the Great Lakes shoreline – Lake Ontario, J. Great Lakes Res., 47, 829–843, https://doi.org/10.1016/J.JGLR.2021.03.007, 2021.
Stephens, S. A. and Wu, W.: Mapping Dependence between Extreme Skew-Surge, Rainfall, and River-Flow, Journal of Marine Science and Engineering, 10, 1818, https://doi.org/10.3390/JMSE10121818, 2022.
Stephens, T. A., Savant, G., Sanborn, S. C., Wallen, C. M., and Roy, S.: Monolithic Multiphysics Simulation of Compound Flooding, J. Hydraul. Eng., 148, 05022003, https://doi.org/10.1061/(ASCE)HY.1943-7900.0002000, 2022.
Stevens, C. L. and Lawrence, G. A.: Estimation of wind-forced internal seiche amplitudes in lakes and reservoirs, with data from British Columbia, Canada, Aquat. Sci., 59, 115–134, https://doi.org/10.1007/BF02523176, 1997.
Svensson, C. and Jones, D. A.: Dependence between extreme sea surge, river flow and precipitation in eastern Britain, Int. J. Climatol., 22, 1149–1168, https://doi.org/10.1002/JOC.794, 2002.
Svensson, C. and Jones, D. A.: Dependence between extreme sea surge, river flow and precipitation: a study in south and west Britain, CEH Wallingford, R&D Interim Technical Report FD2308/TR1, https://assets.publishing.service.gov.uk/media/602bae1fe90e07055e422831/Technical_Report_-_Interim_Product__Joint_probability_dependence_mapping_and_best_practice__.pdf (last access: 17 July 2024), 2003.
Svensson, C. and Jones, D. A.: Dependence between sea surge, river flow and precipitation in south and west Britain, Hydrol. Earth Syst. Sci., 8, 973–992, https://doi.org/10.5194/hess-8-973-2004, 2004.
Swain, D. L., Wing, O. E. J., Bates, P. D., Done, J. M., Johnson, K. A., and Cameron, D. R.: Increased Flood Exposure Due to Climate Change and Population Growth in the United States, Earth's Future, 8, e2020EF001778, https://doi.org/10.1029/2020EF001778, 2020.
Sweet, W., Dusek, G., Obeysekera, J. T. B., and Marra, J. J.: Patterns and projections of high tide flooding along the U.S. coastline using a common impact threshold, NOAA, Technical Report NOS CO-OPs 086, https://doi.org/10.7289/V5/TR-NOS-COOPS-086, 2018.
Tahvildari, N., Abi Aad, M., Sahu, A., Shen, Y., Morsy, M., Murray-Tuite, P., Goodall, J. L., Heaslip, K., and Cetin, M.: Quantification of Compound Flooding over Roadway Network during Extreme Events for Planning Emergency Operations, Nat. Hazards Rev., 23, 04021067, https://doi.org/10.1061/(ASCE)NH.1527-6996.0000524, 2022.
Tanim, A. H. and Goharian, E.: Developing a hybrid modeling and multivariate analysis framework for storm surge and runoff interactions in urban coastal flooding, J. Hydrol., 595, 125670, https://doi.org/10.1016/j.jhydrol.2020.125670, 2021.
Tanir, T., Sumi, S. J., de Lima, A. d. S., de A. Coelho, G., Uzun, S., Cassalho, F., and Ferreira, C. M.: Multi-scale comparison of urban socio-economic vulnerability in the Washington, DC metropolitan region resulting from compound flooding, Int. J. Disast. Risk Re., 61, 102362, https://doi.org/10.1016/j.ijdrr.2021.102362, 2021.
Tao, K., Fang, J., Yang, W., Fang, J., and Liu, B.: Characterizing compound floods from heavy rainfall and upstream–downstream extreme flow in middle Yangtze River from 1980 to 2020, Nat. Hazards, 115, 1097–1114, https://doi.org/10.1007/S11069-022-05585-4, 2022.
Tawn, J. A.: Estimating Probabilities of Extreme Sea-Levels, J. Roy. Stat. Soc.-C App., 41, 77–93, https://doi.org/10.2307/2347619, 1992.
Tehranirad, B., Herdman, L., Nederhoff, K., Erikson, L., Cifelli, R., Pratt, G., Leon, M., and Barnard, P.: Effect of fluvial discharges and remote non-tidal residuals on compound flood forecasting in San Francisco Bay, Water, 12, 2481, https://doi.org/10.3390/W12092481, 2020.
Thompson, C. M. and Frazier, T. G.: Deterministic and probabilistic flood modeling for contemporary and future coastal and inland precipitation inundation, Appl. Geogr., 50, 1–14, https://doi.org/10.1016/J.APGEOG.2014.01.013, 2014.
Tilloy, A., Malamud, B. D., Winter, H., and Joly-Laugel, A.: A review of quantification methodologies for multi-hazard interrelationships, Earth-Sci. Rev., 196, 102881–102881, https://doi.org/10.1016/J.EARSCIREV.2019.102881, 2019.
Torres, J. M., Bass, B., Irza, N., Fang, Z., Proft, J., Dawson, C., Kiani, M., and Bedient, P.: Characterizing the hydraulic interactions of hurricane storm surge and rainfall-runoff for the Houston-Galveston region, Coast. Eng., 106, 7–19, https://doi.org/10.1016/j.coastaleng.2015.09.004, 2015.
Tramblay, Y., Arnaud, P., Artigue, G., Lang, M., Paquet, E., Neppel, L., and Sauquet, E.: Changes in Mediterranean flood processes and seasonality, Hydrol. Earth Syst. Sci., 27, 2973–2987, https://doi.org/10.5194/hess-27-2973-2023, 2023.
Tromble, E., Kolar, R., Dresback, K., Hong, Y., Vieux, B., Luettich, R., Gourley, J., Kelleher, K., and Van Cooten, S.: Aspects of Coupled Hydrologic-Hydrodynamic Modeling for Coastal Flood Inundation, Proceedings of the International Conference on Estuarine and Coastal Modeling, 388, 724–743, https://doi.org/10.1061/41121(388)42, 2010.
Tu, X., Du, Y., Singh, V. P., and Chen, X.: Joint distribution of design precipitation and tide and impact of sampling in a coastal area, Int. J. Climatol., 38, e290–e302, https://doi.org/10.1002/JOC.5368, 2018.
UNDRR: Sendai Framework for Disaster Risk Reduction 2015–2030, Sendai, Japan, https://www.undrr.org/media/16176 (last access: 17 July 2024), 2015.
UNDRR: Report of the open ended intergovernmental expert working group on indicators and terminology relating to disaster risk reduction, United Nations Office for Disaster Risk Reduction (UNDRR), 41, https://www.undrr.org/publication/report-open-ended-intergovernmental-expert-working-group-indicators-and-terminology (last access: 17 July 2024), 2016.
UNDRR: Global Assessment Report on Disaster Risk Reduction, United Nations Office for Disaster Risk Reduction (UNDRR), Geneva, Switzerland, 425, ISBN 978-92-1-004180-5, 2019.
Valle-Levinson, A., Olabarrieta, M., and Heilman, L.: Compound flooding in Houston-Galveston Bay during Hurricane Harvey, Sci. Total Environ., 747, 141272, https://doi.org/10.1016/j.scitotenv.2020.141272, 2020.
Van Berchum, E. C., Van Ledden, M., Timmermans, J. S., Kwakkel, J. H., and Jonkman, S. N.: Rapid flood risk screening model for compound flood events in Beira, Mozambique, Natural Hazards and Earth System Sciences, 20, 2633-2646, https://doi.org/10.5194/NHESS-20-2633-2020, 2020.
Van Cooten, S., Kelleher, K. E., Howard, K., Zhang, J., Gourley, J. J., Kain, J. S., Nemunaitis-Monroe, K., Flamig, Z., Moser, H., Arthur, A., Langston, C., Kolar, R., Hong, Y., Dresback, K., Tromble, E., Vergara, H., Luettich, R. A., Blanton, B., Lander, H., Galluppi, K., Losego, J. P., Blain, C. A., Thigpen, J., Mosher, K., Figurskey, D., Moneypenny, M., Blaes, J., Orrock, J., Bandy, R., Goodall, C., Kelley, J. G. W., Greenlaw, J., Wengren, M., Eslinger, D., Payne, J., Olmi, G., Feldt, J., Schmidt, J., Hamill, T., Bacon, R., Stickney, R., and Spence, L.: The CI-FLOW Project: A System for Total Water Level Prediction from the Summit to the Sea, B. Am. Meteorol. Soc., 92, 1427–1442, https://doi.org/10.1175/2011BAMS3150.1, 2011.
van den Brink, H. W., Können, G. P., Opsteegh, J. D., van Oldenborgh, G. J., and Burgers, G.: Estimating return periods of extreme events from ECMWF seasonal forecast ensembles, Int. J. Climatol., 25, 1345–1354, https://doi.org/10.1002/joc.1155, 2005.
Van Den Hurk, B., Van Meijgaard, E., De Valk, P., Van Heeringen, K. J., and Gooijer, J.: Analysis of a compounding surge and precipitation event in the Netherlands, Environ. Res. Lett., 10, 035001, https://doi.org/10.1088/1748-9326/10/3/035001, 2015.
Van den Hurk, B. J. J. M., White, C. J., Ramos, A. M., Ward, P. J., Martius, O., Olbert, I., Roscoe, K., Goulart, H. M. D., and Zscheischler, J.: Consideration of compound drivers and impacts in the disaster risk reduction cycle, iScience, 26, 106030, https://doi.org/10.1016/j.isci.2023.106030, 2023.
Vitousek, S., Barnard, P. L., Fletcher, C. H., Frazer, N., Erikson, L., and Storlazzi, C. D.: Doubling of coastal flooding frequency within decades due to sea-level rise, Sci. Rep., 7, 1–9, https://doi.org/10.1038/s41598-017-01362-7, 2017.
Vongvisessomjai, S. and Rojanakamthorn, S.: Interaction of Tide and River Flow, Journal of Waterway, Port, Coastal, and Ocean Engineering, 115, 86–104, https://doi.org/10.1061/(ASCE)0733-950X(1989)115:1(86), 1989.
Vormoor, K., Lawrence, D., Heistermann, M., and Bronstert, A.: Climate change impacts on the seasonality and generation processes of floods – projections and uncertainties for catchments with mixed snowmelt/rainfall regimes, Hydrol. Earth Syst. Sci., 19, 913–931, https://doi.org/10.5194/hess-19-913-2015, 2015.
Wadey, M. P., Brown, J. M., Haigh, I. D., Dolphin, T., and Wisse, P.: Assessment and comparison of extreme sea levels and waves during the 2013/14 storm season in two UK coastal regions, Nat. Hazards Earth Syst. Sci., 15, 2209–2225, https://doi.org/10.5194/nhess-15-2209-2015, 2015.
Wahl, T., Jain, S., Bender, J., Meyers, S. D., and Luther, M. E.: Increasing risk of compound flooding from storm surge and rainfall for major US cities, Nat. Clim. Change, 5, 1093–1097, https://doi.org/10.1038/NCLIMATE2736, 2015.
Walden, A. T., Prescott, P., and Webber, N. B.: The examination of surge-tide interaction at two ports on the central south coast of England, Coast. Eng., 6, 59–70, https://doi.org/10.1016/0378-3839(82)90015-1, 1982.
Wang, H. V., Loftis, J. D., Liu, Z., Forrest, D., and Zhang, J.: The Storm Surge and Sub-Grid Inundation Modeling in New York City during Hurricane Sandy, Journal of Marine Science and Engineering, 2, 226–246, https://doi.org/10.3390/JMSE2010226, 2014.
Wang, H. V., Loftis, J. D., Forrest, D., Smith, W., and Stamey, B.: Modeling Storm Surge and Inundation in Washington, DC, during Hurricane Isabel and the 1936 Potomac River Great Flood, Journal of Marine Science and Engineering, 3, 607–629, https://doi.org/10.3390/jmse3030607, 2015.
Wang, S., Najafi, M. R., Cannon, A. J., and Khan, A. A.: Uncertainties in Riverine and Coastal Flood Impacts under Climate Change, Water, 13, 1774–1774, https://doi.org/10.3390/W13131774, 2021.
Ward, P. J., Couasnon, A., Eilander, D., Haigh, I. D., Hendry, A., Muis, S., Veldkamp, T. I. E., Winsemius, H. C., and Wahl, T.: Dependence between high sea-level and high river discharge increases flood hazard in global deltas and estuaries, Environ. Res. Lett., 13, 084012, https://doi.org/10.1088/1748-9326/AAD400, 2018.
Wasko, C., Nathan, R., Stein, L., and O'Shea, D.: Evidence of shorter more extreme rainfalls and increased flood variability under climate change, J. Hydrol., 603, 126994, https://doi.org/10.1016/j.jhydrol.2021.126994, 2021.
Webster, T., McGuigan, K., Collins, K., and MacDonald, C.: Integrated River and Coastal Hydrodynamic Flood Risk Mapping of the LaHave River Estuary and Town of Bridgewater, Nova Scotia, Canada, Water, 6, 517–546, https://doi.org/10.3390/w6030517, 2014.
White, C.: The use of joint probability analysis to predict flood frequency in estuaries and tidal rivers, School of Civil Engineering and the Environment, University of Southampton, 357 pp., https://eprints.soton.ac.uk/63847/ (last access: 17 July 2024), 2007.
Williams, J., Horsburgh, K. J., Williams, J. A., and Proctor, R. N. F.: Tide and skew surge independence: New insights for flood risk, Geophys. Res. Lett., 43, 6410–6417, https://doi.org/10.1002/2016GL069522, 2016.
Wolf, J.: Coastal flooding: Impacts of coupled wave-surge-tide models, Nat. Hazards, 49, 241–260, https://doi.org/10.1007/S11069-008-9316-5, 2009.
Wood, M., Haigh, I. D., Le, Q. Q., Nguyen, H. N., Ba, H. T., Darby, S. E., Marsh, R., Skliris, N., and Hirschi, J. J.-M.: Risk of compound flooding substantially increases in the future Mekong River delta, EGUsphere [preprint], https://doi.org/10.5194/egusphere-2024-949, 2024.
Wood, M., Haigh, I. D., Le, Q. Q., Nguyen, H. N., Tran, H. B., Darby, S. E., Marsh, R., Skliris, N., Hirschi, J. J.-M., Nicholls, R. J., and Bloemendaal, N.: Climate-induced storminess forces major increases in future storm surge hazard in the South China Sea region, Nat. Hazards Earth Syst. Sci., 23, 2475–2504, https://doi.org/10.5194/nhess-23-2475-2023, 2023.
Woodruff, J. D., Irish, J. L., and Camargo, S. J.: Coastal flooding by tropical cyclones and sea-level rise, Nature, 504, 44–52, https://doi.org/10.1038/nature12855, 2013.
Wu, W. and Leonard, M.: Impact of ENSO on dependence between extreme rainfall and storm surge, Environ. Res. Lett., 14, 124043, https://doi.org/10.1088/1748-9326/AB59C2, 2019.
Wu, W., Westra, S., and Leonard, M.: Estimating the probability of compound floods in estuarine regions, Hydrol. Earth Syst. Sci., 25, 2821–2841, https://doi.org/10.5194/hess-25-2821-2021, 2021.
Wu, W., Emerton, R., Duan, Q., Wood, A. W., Wetterhall, F., and Robertson, D. E.: Ensemble flood forecasting: Current status and future opportunities, Wiley Interdisciplinary Reviews: Water, 7, e1432, https://doi.org/10.1002/WAT2.1432, 2020.
Wu, W., McInnes, K., O'Grady, J., Hoeke, R., Leonard, M., and Westra, S.: Mapping Dependence Between Extreme Rainfall and Storm Surge, J. Geophys. Res.-Oceans, 123, 2461–2474, https://doi.org/10.1002/2017JC013472, 2018.
Xiao, Z., Yang, Z., Wang, T., Sun, N., Wigmosta, M., and Judi, D.: Characterizing the Non-linear Interactions Between Tide, Storm Surge, and River Flow in the Delaware Bay Estuary, United States, Frontiers in Marine Science, 8, 715557, https://doi.org/10.3389/fmars.2021.715557, 2021.
Xu, H., Xu, K., Lian, J., and Ma, C.: Compound effects of rainfall and storm tides on coastal flooding risk, Stoch. Environ. Res. Risk A., 33, 1249–1261, https://doi.org/10.1007/S00477-019-01695-X, 2019.
Xu, K., Wang, C., and Bin, L.: Compound flood models in coastal areas: a review of methods and uncertainty analysis, Nat. Hazards, 116, 469–496, https://doi.org/10.1007/s11069-022-05683-3, 2022.
Xu, K., Ma, C., Lian, J., and Bin, L.: Joint probability analysis of extreme precipitation and storm tide in a coastal city under changing environment, PLoS ONE, 9, e109341, https://doi.org/10.1371/journal.pone.0109341, 2014.
Xu, Z., Zhang, Y., Blöschl, G., and Piao, S.: Mega Forest Fires Intensify Flood Magnitudes in Southeast Australia, Geophys. Res. Lett., 50, e2023GL103812, https://doi.org/10.1029/2023GL103812, 2023.
Yang, X. and Qian, J.: Joint occurrence probability analysis of typhoon-induced storm surges and rainstorms using trivariate Archimedean copulas, Ocean Eng., 171, 533–539, https://doi.org/10.1016/j.oceaneng.2018.11.039, 2019.
Yang, X., Wang, J., and Weng, S.: Joint Probability Study of Destructive Factors Related to the “Triad” Phenomenon during Typhoon Events in the Coastal Regions: Taking Jiangsu Province as an Example, J. Hydrol. Eng., 25, 05020038, https://doi.org/10.1061/(ASCE)HE.1943-5584.0002007, 2020.
Yang, Y., Yin, J., Zhang, W., Zhang, Y., Lu, Y., Liu, Y., Xiao, A., Wang, Y., and Song, W.: Modeling of a compound flood induced by the levee breach at Qianbujing Creek, Shanghai, during Typhoon Fitow, Nat. Hazards Earth Syst. Sci., 21, 3563–3572, https://doi.org/10.5194/nhess-21-3563-2021, 2021.
Ye, F., Huang, W., Zhang, Y. J., Moghimi, S., Myers, E., Pe'eri, S., and Yu, H.-C.: A cross-scale study for compound flooding processes during Hurricane Florence, Nat. Hazards Earth Syst. Sci., 21, 1703–1719, https://doi.org/10.5194/nhess-21-1703-2021, 2021.
Ye, F., Zhang, Y. J., Yu, H., Sun, W., Moghimi, S., Myers, E., Nunez, K., Zhang, R., Wang, H. V., Roland, A., Martins, K., Bertin, X., Du, J., and Liu, Z.: Simulating storm surge and compound flooding events with a creek-to-ocean model: Importance of baroclinic effects, Ocean Model., 145, 101526, https://doi.org/10.1016/j.ocemod.2019.101526, 2020.
Yeh, S.-P., Ou, S.-H., Doong, D.-J., Kao, C., and Hsieh, D.: Joint Probability Analysis of Waves and Water Level during Typhoons, Proceedings of the 3rd Chinese-German joint symposium on coastal and ocean engineering, https://citeseerx.ist.psu.edu/document?repid=rep1&type=pdf&doi=a7707b7ede5eb8792559dcbea297c5ab2bd2349b (last access: 17 July 2024), 2006.
Zahura, F. T. and Goodall, J. L.: Predicting combined tidal and pluvial flood inundation using a machine learning surrogate model, J. Hydrol., 41, 101087, https://doi.org/10.1016/j.ejrh.2022.101087, 2022.
Zellou, B. and Rahali, H.: Assessment of the joint impact of extreme rainfall and storm surge on the risk of flooding in a coastal area, J. Hydrol., 569, 647–665, https://doi.org/10.1016/J.JHYDROL.2018.12.028, 2019.
Zhang, L. and Chen, X.: Temporal and spatial distribution of compound flood potential in China's coastal areas, J. Hydrol., 615, 128719, https://doi.org/10.1016/J.JHYDROL.2022.128719, 2022.
Zhang, W., Liu, Y., Tang, W., Wang, W., and Liu, Z.: Assessment of the effects of natural and anthropogenic drivers on extreme flood events in coastal regions, Stoch. Environ. Res. Risk A., 37, 697–715, https://doi.org/10.1007/S00477-022-02306-Y, 2022.
Zhang, W., Luo, M., Gao, S., Chen, W., Hari, V., and Khouakhi, A.: Compound Hydrometeorological Extremes: Drivers, Mechanisms and Methods, Frontiers in Earth Science, 9, 673495, https://doi.org/10.3389/FEART.2021.673495, 2021a.
Zhang, Y., Sun, X., and Chen, C.: Characteristics of concurrent precipitation and wind speed extremes in China, Weather and Climate Extremes, 32, 100322, https://doi.org/10.1016/j.wace.2021.100322, 2021b.
Zhang, Y. J., Witter, R. C., and Priest, G. R.: Tsunami–tide interaction in 1964 Prince William Sound tsunami, Ocean Model., 40, 246–259, https://doi.org/10.1016/J.OCEMOD.2011.09.005, 2011.
Zhang, Y. J., Ye, F., Yu, H., Sun, W., Moghimi, S., Myers, E., Nunez, K., Zhang, R., Wang, H., Roland, A., Du, J., and Liu, Z.: Simulating compound flooding events in a hurricane, Ocean Dynam., 70, 621–640, https://doi.org/10.1007/S10236-020-01351-X, 2020.
Zheng, F., Westra, S., and Sisson, S. A.: Quantifying the dependence between extreme rainfall and storm surge in the coastal zone, J. Hydrol., 505, 172–187, https://doi.org/10.1016/j.jhydrol.2013.09.054, 2013.
Zheng, F., Westra, S., Leonard, M., and Sisson, S. A.: Modeling dependence between extreme rainfall and storm surge to estimate coastal flooding risk, Water Resour. Res., 50, 2050–2071, https://doi.org/10.1002/2013wr014616, 2014.
Zhong, H., van Overloop, P.-J., and van Gelder, P. H. A. J. M.: A joint probability approach using a 1-D hydrodynamic model for estimating high water level frequencies in the Lower Rhine Delta, Nat. Hazards Earth Syst. Sci., 13, 1841–1852, https://doi.org/10.5194/nhess-13-1841-2013, 2013.
Zschau, J.: Where are we with multihazards, multirisks assessment capacities?, European Union Joint Research Council, Luxembourg, https://doi.org/10.2788/688605, 2017.
Zscheischler, J. and Seneviratne, S. I.: Dependence of drivers affects risks associated with compound events, Science Advances, 3, e1700263, https://doi.org/10.1126/SCIADV.1700263, 2017.
Zscheischler, J., Westra, S., Van Den Hurk, B. J. J. M., Seneviratne, S. I., Ward, P. J., Pitman, A., Aghakouchak, A., Bresch, D. N., Leonard, M., Wahl, T., and Zhang, X.: Future climate risk from compound events, Nat. Clim. Change, 8, 469–477, https://doi.org/10.1038/S41558-018-0156-3, 2018.
Zscheischler, J., Martius, O., Westra, S., Bevacqua, E., Raymond, C., Horton, R. M., van den Hurk, B., AghaKouchak, A., Jézéquel, A., Mahecha, M. D., Maraun, D., Ramos, A. M., Ridder, N. N., Thiery, W., and Vignotto, E.: A typology of compound weather and climate events, Nat. Rev. Earth Environ., 1, 333–347, https://doi.org/10.1038/s43017-020-0060-z, 2020.
- Abstract
- Introduction
- Definitions and types of compound events and multi-hazard events
- Flood processes and mechanisms
- Literature database methodology
- Review of literature database
- Discussion
- Knowledge gaps and improvements for future research
- Conclusions
- Appendix A
- Data availability
- Author contributions
- Competing interests
- Disclaimer
- Acknowledgements
- Financial support
- Review statement
- References
- Abstract
- Introduction
- Definitions and types of compound events and multi-hazard events
- Flood processes and mechanisms
- Literature database methodology
- Review of literature database
- Discussion
- Knowledge gaps and improvements for future research
- Conclusions
- Appendix A
- Data availability
- Author contributions
- Competing interests
- Disclaimer
- Acknowledgements
- Financial support
- Review statement
- References