the Creative Commons Attribution 4.0 License.
the Creative Commons Attribution 4.0 License.
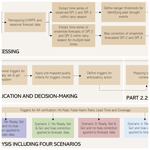
Ready, Set & Go! An anticipatory action system against droughts
Gabriela Guimarães Nobre
Jamie Towner
Bernardino Nhantumbo
Célio João da Conceição Marcos Matuele
Isaias Raiva
Massimiliano Pasqui
Sara Quaresima
Rogério Manuel Lemos Pereira Bonifácio
The World Food Programme, in collaboration with the Mozambique National Meteorology Institute, is partnering with several governmental and non-governmental organizations to establish an advanced early warning system for droughts in pilot districts across Mozambique. The “Ready, Set & Go!” system is operational in Mozambique for activating anticipatory action (AA) against droughts based on predefined thresholds, triggers and pre-allocated financing. The system uses bias-corrected and downscaled seasonal forecasts from the European Centre for Medium-Range Weather Forecasts (ECMWF) as core information to anticipate severe reductions in rainfall during the rainy season. This information guides the implementation of actions to reduce the impacts of rainfall deficits in the critical window between a forecast and the onset of the drought event. Within this window of opportunity, the system releases an alert for readiness (Ready) and activation (Set) preceding the mobilization of anticipatory action on the ground (Go). With the recent adoption of the Southern African Development Community “Maputo Declaration on Bridging the Gap between Early Warning and Early Action”, member states have committed to enhancing the reach of early warning system by leaving no one behind. Therefore, there is a need to assess the opportunities and limitations of the Ready, Set & Go! system to scale up drought AA information to all districts in Mozambique. This study describes the Ready, Set & Go! system, which uses ensemble forecasts of the Standardized Precipitation Index to trigger anticipatory action against droughts on a seasonal timescale. The Ready, Set & Go! optimizes the use of seasonal forecast information by choosing triggers for anticipatory action based on verification statistics and on a double-confirmation process, which combines longer lead times with shorter lead time forecasts for issuing drought alerts. In this study, we show the strengths of the system by benchmarking it against three simpler triggering approaches. Our findings indicate that the Ready, Set & Go! system has significant potential to scale up AA activities against severe droughts throughout the entire rainy season, covering on average 76 % of the Mozambican districts. This approach outperforms the three benchmarked methods, demonstrating higher hit rates, extended lead times and a lower false alarm. If efforts are concentrated on the first part of the rainy season, national coverage against severe droughts could be expanded to 87 % of all districts. By aligning with the objectives outlined in the “Maputo Declaration” and the “Early Warning for All” initiative, this research contributes to safeguarding communities against the adverse impacts of climate-related events, aligning with the ambitious goal of universal protection by 2027.
- Article
(5279 KB) - Full-text XML
-
Supplement
(5117 KB) - BibTeX
- EndNote
Mozambique experienced one of its worst drought events in decades in 2015/2016, which affected the food security of approximately 2.3 million people, leading to its government to declare a state of national emergency (OCHA, 2017). This El Niño-induced drought caused an exceptional lack of precipitation in two consecutive rainy seasons, which resulted in significant losses in rain-fed yields, below-average irrigated crops, poor pasture conditions and high cattle mortalities (WFP, 2016). The dryness propagated into water reservoirs in southern Mozambique, where the impact on water levels remained for 5 years (Echo Flash, 2023).
Mozambique is a country exposed and vulnerable to multiple hazards due to its geographical location and latitudinal extent. Its climate is affected by several modes of climate variability such as the El Niño–Southern Oscillation (ENSO; Rapolaki et al., 2019; Blamey et al., 2018), the Indian Ocean Dipole (IOD; Ashok et al., 2001; Manatsa et al., 2011; Saji et al., 1999) and the Subtropical Indian Ocean Dipole (SIOD; Behera and Yamagata, 2001). These climate modes of variability modulate the frequency and intensity of the various weather systems that are directly associated with multiple natural hazards happening as a single or consecutive risk (e.g. Hart et al., 2010; Manhique et al., 2015, 2021; Mawren et al., 2020; Rapolaki et al., 2019; Reason and Keibel, 2004). Impacts of single and consecutive hazards including flooding, cyclones and droughts are exacerbated by poverty and weak institutional development, where climate-related disasters are one of the main driving forces of inequality and food insecurity in the country (Baez et al., 2020; De Ruiter et al., 2020). In Mozambique, nearly 25 % of its population lives in areas with a high probability of experiencing a climate shock (World Bank, 2018). Therefore, the adoption of protective mechanisms and systems to anticipate and prepare the government and communities to climate shocks is crucial for building resilience and for sustainable development. Recently, the national government has made climate risk management a priority strategy following the adoption of the Maputo Declaration on Bridging the Gap between Early Warning and Early Action, in which member states of the Southern African Development Community (SADC) have committed to take an active people-centred role to ensure all citizens have access to effective early warning and early action systems (SADC, 2022).
Since 2019, a multi-sector government-led anticipatory action (AA) trigger system against drought (WFP, 2023) has been under development in Mozambique, coordinated by the Mozambique National Institute of Disaster Management (INGD) with the technical support of relevant actors, including the National Meteorological Institute (INAM) and the World Food Programme (WFP). Droughts are a slow, recurrent and predictable phenomenon (Guimarães Nobre et al., 2023), yet they cause an estimated yearly loss of USD 20 million (Baez et al., 2020) to Mozambique. A drought early warning system (EWS) has a great potential to reduce some of these losses when AA is implemented ahead of a shock based on forecast information. Previous studies have assessed the skill of seasonal forecasts to predict the onset of droughts (Gebrechorkos et al., 2022; Guimarães Nobre et al., 2023; Trambauer et al., 2015; Winsemius et al., 2014), whereas only a few have focused on an in-depth interpretability of the forecast quality through the lenses of decision-making and practical implications. For instance, a reflection on the adequateness of lead time of information for action and/or definition of probabilistic trigger values for releasing drought alerts and advisories for AA are aspects largely missing in the scientific literature.
AA approaches are gaining more traction with an increased number of institutions dedicating funding and pilot studies in Mozambique and elsewhere. There are currently anticipatory action initiatives and projects in 43 countries, supported by 179 organizations, including the Red Cross movement and UN entities such as the United Nations Office for the Coordination of Humanitarian Affairs and the WFP (Anticipatory action in the world, 2024). However, the evidence of the benefits of acting earlier is still fairly new and limited. Overall, existing evidence based on pilot experiences in other parts of the world has mainly suggested a positive impact of AA at household level, with beneficiaries reporting higher crop productivity and less food insecurity during prolonged periods of drought (Weingärtner et al., 2020). In Mozambique, AA drought pilots are limited – to date – to 11 districts, and further scale-up of activities to the national level is desired. However, an assessment of the opportunities and limitations of the current drought AA trigger system is currently missing, especially given the 2023 El Niño scenario, which negatively affected the 2023–2024 rainy season. In response to the need for assessing the potential of bringing AA to scale, this study describes the operational triggering system for drought AA being piloted in Mozambique during the southern Africa rainy season 2023–2024. This article presents the analytical routines involved in the definition and monitoring of triggers for AA, describing the technical methodologies of the system by outlining data processes, forecast application, decision-making and operational activities linked to the release of AA advisories to pilot areas.
2.1 Case study
We developed a methodology that is being piloted and is scalable for triggering AA against droughts for all districts in Zimbabwe and Mozambique, although this study has a special focus on the latter. Currently in Mozambique, a government-led AA plan is in place for 11 pilot districts (see Fig. 1). However, an anticipatory action system is desired for the whole country that requires the upscaling of the current set-up. Concerning climatology, the rainy season in Mozambique lasts from October to May, although the largest amounts of precipitation are experienced between November and April. The wettest months are December and January; however January alone is the wettest month across the country (WFP, 2018). Rainfall amounts increase from south to north. For instance, areas with low annual rainfall (less than 500 mm) include the southern provinces of Maputo, Gaza, Inhambane and the southern half of Tete, whereas areas with high total rainfall (over 2000 mm) include the provinces of Cabo Delgado, Niassa, Nampula and Zambezia. Rainfall interannual variability is stronger in areas of lower rainfall totals and is a major limiting factor in livelihoods and small-scale rain-fed agriculture (Guimarães Nobre et al., 2023). In addition, the province of Gaza has a remarkably variable and short growing season length (mostly below 3 months). Interannual climate variability in the southern Africa region is particularly linked to the El Niño–Southern Oscillation (ENSO) (Richard et al., 2001). During the months of October to December, the El Niño phase often drives rainfall increases in Cabo Delgado and Niassa in northern Mozambique (and decreases in the southern provinces of Maputo, Gaza and Inhambane). During these months, when a La Niña state is observed, rainfall increases are observed in parts of the central provinces of Manica, Sofala and northern Inhambane. In addition, during the months of January to March, El Niño leads to drier conditions across most of the country, whereas in the south and centre of the country a moderate increase in rainfall is observed during La Niña phases (WFP, 2018). Mozambique is a highly climate vulnerable country where livelihoods rely on local natural resources (e.g. agriculture and fisheries) as their primary economic activity. Drought events affect the ability of farmers and fishers to sustain crops and fish, often cascading into situations of food insecurity, malnutrition and unsustainable incomes.
2.2 Methodological framework
The operational triggering system for drought AA is developed and tested in three stages (Fig. 2): (1) data pre-processing, (2) forecast application and decision-making, and (3) sensitivity analysis. A detailed explanation of each stage is provided in Sect. 2.2.1 to 2.2.3.
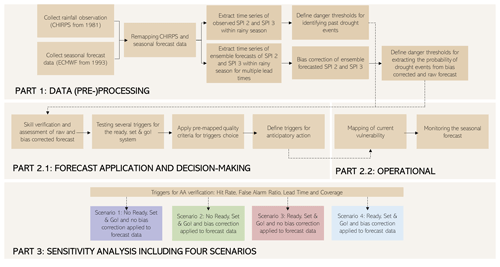
Figure 2Flowchart of the methodological framework applied in this study, handled in three stages: (1) data pre-processing, (2) forecast application and decision-making, and (3) sensitivity analysis.
2.2.1 Part 1: data pre-processing
Collecting rainfall observations (from 1981)
As source of rainfall estimates, we use daily blended precipitation records from the Climate Hazards Group InfraRed Precipitation with Station data (CHIRPS) version 2 for the period of January 1981 to near present. CHIRPS is a high-resolution (0.05°) precipitation dataset, which is used for drought early warning purposes by the Famine Early Warning Systems Network This dataset integrates data from real-time meteorological stations with infrared satellite data (therefore called a blended precipitation product), covering from 50° N to 50° S via a blending procedure further described in Funk et al. (2015).
Collecting seasonal forecast data (ECMWF from 1993)
As a source of forecast data, we use seasonal precipitation forecasts from the ECMWF's seasonal forecasting system (SEAS5) for the period 1993–2022. In its native resolution, the forecast is available at 1 arcdeg, and new forecasts are released monthly on the fifth day covering the coming 7 months. SEAS5 is composed of a set of 25 ensemble members until 2016 (hindcast period) and 51 ensemble members from 2017 onwards as part of the operational system (Ratri et al., 2019). It is important to highlight that ECMWF SEAS5 has had a new version (SEAS5.1) since November 2022 with an extended hindcast until 1981; the full time series of hindcast and operation forecast can be freely downloaded from the Copernicus Climate Data Store.
Remapping CHIRPS and seasonal forecast data
Since the datasets of rainfall estimates and forecasts are available in different spatial resolutions, we remapped them into an intermediate resolution of 0.25°. This moderate resolution was chosen taking into consideration the size of pilot districts in which the system will be implemented and the computational capacity, as well as to reduce the impact of rainfall small-scale variability. For this process, we used bilinear interpolation, one of the most commonly used methods of climate grid interpolation (The National Center for Atmospheric Research Staff, 2014). Bilinear interpolation resizes the data by estimating values at a point by averaging the values of the surrounding points.
Extracting time series of observed SPI 2 and SPI 3 within the rainy season
From the daily CHIRPS rainfall estimates, we extract the Standard Precipitation Index (SPI), a widely used indicator for measuring rainfall variability over a long-term climatological period (World Meteorological Organization, 2012). The SPI is centred around the mean rainfall for a given time and location, with values ranging from −4 to +4. Negative SPI values indicate various levels of rainfall deficits, which are particularly relevant to the designed trigger system. The SPI can also highlight drought situations when a “danger threshold” is identified, signalling rainfall deficits severe enough to prompt anticipatory action to mitigate the impacts on livelihoods.
In this study, SPI values are calculated using 2- and 3-month accumulation periods (SPI 2 and SPI 3, respectively). These accumulation windows are particularly suitable for detecting risks to agricultural systems during the crop development cycle. It is crucial to note that the AA framework aims to protect food security by reducing the risk of crop failures in rain-fed systems. Therefore, only SPI values extracted during the rainy season are relevant to the trigger system (see the section below for a detailed explanation of windows of opportunity for anticipatory action).
To derive the SPI estimates, the CHIRPS rainfall dataset, accumulated over 2 and 3 months, is fitted to a gamma distribution and subsequently transformed to a normal distribution with z values (Lloyd-Hughes and Saunders, 2002). The period from 1981 to 2018 serves as the reference climatology for calculating the gamma distribution parameters. This period was selected due to the availability of a complete series of rainfall observations at the start of the project in 2019. Periods with zero precipitation are handled by assigning SPI values based on the historical occurrence of such periods from 1981 to 2018. However, since we use precipitation data accumulated over 2 and 3 months, zero values are rare, especially as SPI is only extracted during the rainy season. For extracting SPI during the dry season or in arid regions, more-sophisticated techniques, such as those described by Stagge et al. (2015), are available and should be preferred.
Extracting time series of ensemble SPI 2 and SPI 3 within the rainy season for multiple lead times
For the forecasting series, the parameters of the gamma distribution are determined using data from all ensemble members for the years 1993 to 2018, as data prior to 1993 are not available in the Copernicus Climate Data Store (SEAS5). The routine adopted for handling zero values is similar to the one described for deriving SPI estimates (see above). In Fig. 3, we illustrate the extraction of SPIs for various lead times of the forecast system with a 7-month lead time. For example, the seasonal forecast released at the beginning of May covers the subsequent months (May to November). Therefore, the only indicator extracted from this forecast is SPI 2 ON, as October marks the first month of the rainy season in the country.
Defining danger threshold for identifying past drought events
Given that the Standardized Precipitation Index (SPI) is linked to the probability of certain rainfall amounts, we convert a specific z value into an expected frequency by calculating the area under the normal distribution curve up to that z value. This proportion, or probability (p), is then converted into a return period (T) by taking the inverse of the probability (). In the operational AA trigger system, three z-value thresholds are used, as highlighted by Guimarães Nobre et al. (2023), corresponding to different severity levels. This article focuses on the most severe category in the AA trigger system, which is SPI ≤ −1, as this negative anomaly is expected to cause the most significant damage among those adopted by the system.
However, it is important to highlight that the impact of a drought threshold should ideally be estimated using historical observations combined with information on who and what is exposed to a hazard (exposure and vulnerability). Due to the lack of extensive drought impact data at the district level, the choice of a threshold level is based on frequencies suitable for AA operations in the region. Typically, AA programmes target hazards that occur at least once every 3–6 years on average. Implementing AA pilots periodically is crucial for enhancing programme activities. Consequently, thresholds for AA operations should not be set too low, given that the occurrence of drought events of such intense magnitude is rare. A SPI ≤ −1 (named severe category in the AA trigger system) corresponds to an event occurring approximately once every 6–7 years (or p=15.87 %). By applying the SPI ≤ −1 threshold to the series estimated by SPI 2 and SPI 3, we obtain a time series since 1981 of past drought events for the respective 2- and 3-month periods in the pilot districts.
Bias correction of ensemble-forecasted SPI 2 and SPI 3
We employ a quantile–quantile mapping (QM) technique, conditioned on the state of ENSO, to adjust SPI forecast values. This is achieved by aligning the cumulative density function of SPI forecasts at each grid cell with the reference SPI data extracted from CHIRPS at the corresponding grid cell and its k nearest neighbours. The SPI forecast and reference distributions are matched by establishing an ENSO-informed, quantile-dependent correction function. This function adjusts the forecast quantiles based on their observed SPI counterparts, translating the SPI forecast time series into bias-adjusted values that accurately represent the observed SPI data distribution.
The transfer functions for bias correction are developed based on the SPI reference and SPI forecast time series, specifically targeting the AA drought indicator rather than daily or monthly rainfall. By incorporating ENSO information, we aim to ensure that rainfall variability is more accurately represented in the corrected forecast data, especially in regions and timescales where ENSO has a significant impact (Manzanas and Gutiérrez, 2019). This approach combines a statistical quantile mapping bias correction with ENSO state knowledge during rainy seasons. Furthermore, information from the nearest neighbours from the reference pixel is used to account for the spatial dependence inherent in climate data (k=9) (Cannon, 2018) and to extend the SPI time series used to create the transfer function. By targeting the SPI indicator directly with the transfer function, we aim to increase the accuracy of drought detection by bringing SPI forecasts closer to the observed SPI climatology, ensuring that the SPI derived from forecasts is more consistent with historical patterns and trends. This is critical for the Ready, Set & Go! system that releases alerts based on negative anomalies through the SPI indicator rather than on rainfall amounts.
In practical terms, incorporating ENSO information into quantile mapping involves the following: (i) categorizing data by ENSO phases, (ii) generating empirical cumulative distribution functions for each ENSO phase separately for both SPI observations and SPI forecasts, (iii) performing quantile mapping by applying the transfer function to the test year (year left out during cross-validation) of the analysis according to the ENSO phase of the year being bias corrected, and (iv) combining corrected forecast outputs if the bias correction is found to improve skill in detecting droughts.
In summary, the quantile mapping transfer function corrects the SPI forecast based on the SPI reference value of the pixel under investigation and its nine neighbouring pixels conditioned on the state of ENSO. To prevent inflating the skill of the bias correction, a leave-one-year-out cross-validation (LOCV) scheme is used. The bias correction transfer function is constructed by pooling all ensemble members of the forecast and then applied to all members of the left-out test year.
An overview of this scheme is available in Fig. 4. For a list of ENSO years, see Sect. S1 in the Supplement.
Defining danger threshold for extracting the probability of drought events from bias-corrected and raw forecasts
From both raw and bias-corrected forecasts, we apply the danger threshold (SPI ≤ −1, classified as severe in the AA trigger system) to determine the probability of a severe drought. This is done by calculating the proportion of ensemble members that meet or fall below the threshold. We repeat this process for each forecast issue month from 1993 to 2022, creating a time series of drought probabilities at different lead times for both the raw and bias-corrected forecasts.
In practice, the bias-corrected drought probabilities replace those from the raw forecast only when there is a demonstrable gain in skill for forecasting severe drought. This gain in skill is evaluated by comparing the area under the receiver operating characteristic (AUROC) curve scores of the raw and bias-corrected forecasts (further detailed in the section below). Consequently, the bias-corrected drought probability information is used only if it shows an improved ability to predict severe droughts in the pilot districts, considering specific cases (such as a particular forecast lead time and SPI 2 and SPI 3 aggregation).
2.2.2 Part 2.1: forecast application and decision-making
Skill verification and assessment of raw and bias-corrected data
As described in the previous section, we obtain drought probabilities from both the raw and bias-corrected forecasts. For each specific district, lead time and SPI indicator, we use the forecast with the higher skill in predicting severe drought to develop triggers for the AA. The forecast with lower skill is discarded from the AA system. Skill is assessed by extracting and comparing the AUROC scores of the forecasts.
The AUROC score (e.g. Fawcett, 2006) is a widely applied indicator that measures the ability of a probabilistic forecast to discriminate between binary outcomes (e.g. severe drought or no drought). The AUROC score calculation requires setting a range of trigger values to convert a probability forecast into a categorical one, and therefore it is related to decision-making in response to whether the forecast should release an alert. For the releasing of a “drought alert”, several triggers are tested, and a graph (known as a ROC curve) is produced to summarize the hit rate and false alarm rate that can be expected from different probability trigger values. The area under the ROC provides a summary statistic for the performance of probability forecasts, ranging from 0 to 1 (worst to best). Forecasts with little or no skill have a ROC score of approximately 0.5. Forecast is perfectly incorrect when the ROC is zero. In summary, for a specific district, lead time and SPI indicator, we choose which source of forecast to use for the Ready, Set & Go! triggers (raw or bias corrected) based on the forecast skill assessment informed by the AUROC score at the district level.
Testing several triggers for the Ready, Set & Go! system
Triggers for anticipatory action indicate the forecasted severity of drought that would prompt a response. If the forecast exceeds the trigger, funds are automatically allocated, and anticipatory actions are initiated. A trigger is essentially a value that converts a probability forecast into a decision on whether to take action, effectively determining whether a drought alert should be issued. Defining a trigger involves understanding when forecasting information can be trusted to successfully mobilize anticipatory actions, despite inherent uncertainties. Therefore, triggers are based on the skill levels of the forecasts, requiring an investigation of past forecast accuracy and an acknowledgement of forecast uncertainty.
Forecasts at any lead time can be tested to derive triggers for anticipatory action. It is common practice for organizations to define two types of triggers for anticipatory action: (i) a preparedness trigger with a longer lead time and (ii) a confirmatory trigger for the activation of activities with a shorter lead time before the drought onset. These triggers are defined based on the skill levels of the forecasts for each lead time. However, testing lead times independently may result in an unrealistic performance of the anticipatory action programme, as the system relies on both triggers being exceeded, even though they are set based on their individual performance. Additionally, organizations may assign preparedness and activation activities based on a single trigger from a specific lead time. This approach can vary depending on the organization's specific capacity to respond to the forecasted information.
The Ready, Set & Go! system employs a double-confirmation approach for drought alerts. This means that the trigger value, tailored for each forecast month, district and SPI indicator, must be exceeded for 2 consecutive months to prompt action. The performance of these triggers for anticipatory action is evaluated in combination rather than individually. For example, if the trigger based on the August forecast for Chibuto district, which predicts potential severe droughts in October–November, is exceeded, the “Ready” phase is activated. If the trigger based on the September forecast for the same district is also exceeded, the “Set” phase is activated, and activities are immediately mobilized on the ground, initiating the “Go!” phase. Testing triggers in combination with a double-confirmation process aims to create a more accurate trigger system and provide a longer window for readiness and preparedness activities before AA implementation. This approach is validated using a sensitivity analysis explained in Sect. 2.2.4.
For instance, readiness activities might involve preparing internal documents, which can then lead to initiating a procurement process if an AA advisory is issued. Practically, for each forecast month that can produce a “Ready” and “Set” trigger, we jointly test several candidate pairs of triggers. This testing is conducted in steps of 1 % ranging from 0 % to 100 %, resulting in 10 201 combinations of candidate triggers. This is done for each district, pair of forecast months and SPI 2 and SPI 3 indicator. For a complete overview of the triggers for SPI ON for a given district, we test all candidate pairs of triggers for the following forecast month combinations: May (Ready) and June (Set), June (Ready) and July (Set), July (Ready) and August (Set), August (Ready) and September (Set), and September (Ready) and October (Set). For each pair of triggers, we calculate key performance metrics (e.g. hit rate and false alarm ratio) to evaluate how the drought alerts would have performed in the past. The relevance of these metrics was identified during a workshop held in 2022 with governmental partners.
Applying pre-mapped quality criteria for the trigger choice
The definition of a trigger value for drought AA is intrinsically linked to the skill of the forecast and the identification of a certain degree of risk tolerance levels by users of the forecast (Lopez et al., 2018). In practice, when a low probability trigger value is chosen, one can expect to forecast droughts frequently, whereas if a very high value is chosen, the opposite is expected to happen. The optimum trigger value should reflect appropriateness through the lenses of the decision-maker and the relative importance given to drought false alarms versus missed drought events.
Users who are averse to missing a drought will choose a lower trigger value and deal with an increase in false alarms. For instance, a low trigger value can be a suitable option for actors that seek to assist very fragile populations and/or when the portfolio of AA is considered “non-regret” (Chaves-Gonzalez et al., 2022). Anticipatory actions are classified as “non-regret” when they are worth investing in even if a crisis does not materialize and would not be regretted with hindsight. Following this approach, we have created a menu of “emergency triggers”, to be used when pilot districts experience high levels of vulnerability. On the other hand, users who are averse to false alarms will choose a higher trigger and manage occasional missed events. For instance, a high trigger value can be a suitable option for actors that have limited funds and/or when the portfolio of AA contains actions that affect livelihoods, such as evacuations, which are considered highly regrettable if a false alarm occurs. This approach can be of high relevance for scaling up AA to all districts in Mozambique as the largest geographical coverage is desired and funding distribution/sharing across a wide area is expected. Following this approach, we have created a menu of “general triggers” to be used when pilot areas experience normal to low levels of vulnerability. As displayed in Table 1, the expected performance of both menus is different, especially concerning the tolerance to false alarms and the probability of drought detection. Operationally, the assessment of vulnerability information is done prior to the start of the AA season in Mozambique (see Sect. 2.2.3 for further explanation).
Define triggers for anticipatory action
After testing all combinations of trigger pairs for the “Ready” and “Set” phases and recording the statistics listed in Table 1, we began a selection process based on the quality criteria outlined in the same table. The suitable pairs were ranked according to their hit rate and false alarm ratio, considering both district-specific performance and the stage of the rainy season: (i) start to mid-season (referred to as Window 1) and (ii) middle to the end of the season (referred to as Window 2). Only the best-performing trigger pairs were selected for further analysis, which is presented in Sect. 3.4.
Table 2Description of anticipatory action windows per zone and province with an illustration of SPI indicators informing drought events.
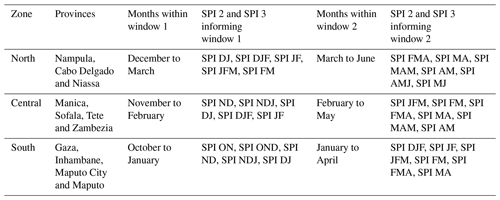
It is important to clarify that AA targets these two windows of the rainy season because the activities implemented before the onset of drought within these periods serve different purposes. The forecast of drought risks within these windows informs the refinement of the AA portfolio, as rainfall deficits during the start to mid-season and middle to the end of the season are expected to impact crops differently. For example, AA implemented before potential droughts in Window 1 aims to support planting and sowing activities, such as distributing drought-tolerant seeds, while AA implemented in Window 2 focuses on supporting livelihoods, such as providing cash transfers.
Furthermore, due to the variation in climatology across the country, the periods covered by Windows 1 and 2 differ by zone, shifting by approximately 1 month from south to north. Table 2 provides an overview of the timing of these windows, the indicators used to assess drought risks within them and the provinces associated with each zone. The division of the rainy season into these windows was defined by the technical working group (TWG) for drought early warning systems (EWS) and AA, which includes several governmental and non-governmental institutions (WFP, 2023). Further details can be found in the discussion section.
2.2.3 Operation
Once the repository of triggers for AA has been finalized, several operational activities follow. Although these activities do not impact the overall system performance (as presented in the results section), they provide valuable insight into the operationalization of the methodology showcased in this study. The first key activity following the initiation of forecast and trigger monitoring for AA is a vulnerability analysis. This analysis is conducted annually, typically around April and May as the rainy season concludes. Its purpose is to assess the levels of vulnerability in the AA pilot districts by examining recent climate shocks and projected food security outcomes. The results of this analysis inform decisions about which set of triggers – general or emergency – each pilot district should employ for the upcoming AA season. For example, if a district experienced drought during the most recent rainy season, with anticipated negative impacts on food security, the emergency triggers are selected for the next AA season due to the heightened vulnerability in that area. Once this decision is made, forecasts from May to February of the following year are processed, and the AA triggers are monitored on a monthly basis. The monitoring of the Ready, Set & Go! system triggers is conducted by INAM and WFP, with updates communicated to the technical working group (TWG) for drought early warning systems (EWSs) and AA through a dashboard and regular bulletins.
2.2.4 Sensitivity analysis including four scenarios
We evaluate the robustness of our methods through a sensitivity analysis, considering four distinct scenarios. For each scenario, we extract four key metrics:
-
Hit rate is the percentage of past severe droughts accurately captured by the AA trigger(s).
-
Tolerant false alarm ratio accounts for false alarms when the AA trigger is exceeded, but the drought threshold is narrowly missed. For example, a false alarm occurs if a severe drought trigger (SPI ≤ −1) is followed by an SPI value just below the threshold (e.g. −0.99). To better contextualize false alarms, we calculate the “tolerant” false alarm ratio, which considers the number of severe drought alarms followed by an SPI greater than −0.68 (see Table 1). This introduces extra tolerance when analysing forecasting errors, as severe drought alerts followed by SPI values between −0.68 and −0.99 are not counted as non-drought situations. This approach is based on the practical assumption that AA interventions will still benefit the population even if implemented during slightly less severe dryness.
-
Lead time of implementation is the time difference between the starting month of the SPI indicator and the month in which the forecast was issued. For instance, a forecast issued in May is considered to have a lead time of 4 months when providing outlooks of SPI ON.
-
AA percentage coverage is the percentage of Mozambican districts where an AA trigger was identified, meeting the criteria outlined in Table 1.
It is important to clarify that these metrics were derived from the skill assessment of the forecasts from 1993 to 2021. Specifically, the number of hits and false alarms during this period is used to calculate a key metric from the quality criteria list: the “return period (years) for the Implementation of AA against droughts”. This metric helps determine whether the empirical frequency of AA interventions aligns with the frequency of the threshold for severe droughts. Furthermore, the scenarios for the sensitivity analysis are defined as follows:
-
Scenario 1 is an AA advisory based solely on a single alert, using only one lead time from the raw SPI forecasts.
-
Scenario 2 is an AA advisory based solely on a single alert, using either raw or bias-corrected SPI forecasts, depending on which has the highest skill.
-
Scenario 3 is an AA advisory requiring double confirmation but using only raw SPI forecasts.
-
Scenario 4 is an AA advisory based on the Ready, Set & Go! system, requiring double confirmation and using a combination of bias-corrected and raw SPI forecasts.
3.1 Zonal-based overview of the years with severe drought conditions within the rainy season
In Fig. 5, we illustrate the frequency of severe drought occurrences during the rainy season from 1981 to the present. We began by extracting the mean SPI 2 and SPI 3 indicators for each district, focusing on the rainy windows relevant to each district and/or province (see Table 2 for SPI indicators and their associated windows). We then counted how often the severe drought threshold was met or exceeded. The top 5 years with the highest number of 2- and 3-month periods experiencing severe drought conditions are highlighted. Bars in the figure are coloured to indicate the ENSO phase during the respective rainy seasons in Mozambique (see Sect. S1 for classification). To simplify the data presentation, districts are grouped by zones (refer to Table 2 for zone-to-province list). A similar overview of severe drought years at the province and district levels is provided in Sect. S2.
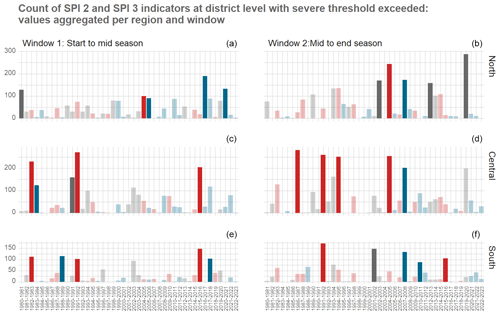
Figure 5The frequency with which the SPI 2 and SPI 3 indicators exceeded or equaled the severe drought threshold since 1981 is shown for each zone and window. The counts are first calculated at the district level and then aggregated by zone for window 1 (a, c, e) and window 2 (b, d, f). For details on which SPI 2 and SPI 3 indicators correspond to each window, refer to Table 2. The zones are defined as follows: (i) central zone includes districts from the provinces of Manica, Sofala, Tete and Zambezia; (ii) north zone includes districts from Nampula, Cabo Delgado and Niassa; and (iii) south zone includes districts from Gaza, Inhambane, the province of Maputo City and Maputo Province. Bars are colour-coded according to the dominant ENSO phase during the rainy season in Mozambique (red – El Niño, blue – La Niña and grey – neutral). The top 5 years for each window and zone are highlighted.
Overall, severe drought conditions can occur during any of the three ENSO phases across all zones. This underscores the need for an AA system that is effective regardless of the ENSO phase. However, we found that severe droughts are significantly more frequent during El Niño phases (mean frequency = 66) compared to neutral (mean frequency = 41) and La Niña phases (mean frequency = 31), as confirmed by a t test (p<0.01). Previous studies also support this finding (Araneda-Cabrera et al., 2021; Lyon and Mason, 2007). Additionally, the top 5 drought years for different windows vary considerably. In the north zone, only the rainy season of 2004–2005 appears in the top 5 for both windows. In the central zone, only the 1991–1992 rainy season ranks in the top 5 for both windows. In the south zone, the rainy seasons of 1991–1992 and 2015–2016 are among the top 5 for both windows. This variation highlights the importance of developing an early warning system that accounts for different intra-seasonal rainfall patterns and adjusts operations according to the stages of the rainy cycle.
3.2 Zonal-based overview of bias correction
Figure 6 presents the percentage of areas per zone, the SPI indicator and the forecast month that showed an improved AUROC score after applying bias correction. The primary focus of our evaluation is the AUROC score, as it offers a practical measure of whether bias correction enhances the accuracy of severe drought forecasts, which is crucial for users. The goal of this approach is to identify opportunities for improving forecast accuracy, thereby reducing the risk of misallocated anticipatory action resources due to inaccurate predictions. For a spatial representation, similar results are displayed in a series of maps in Sect. S3.
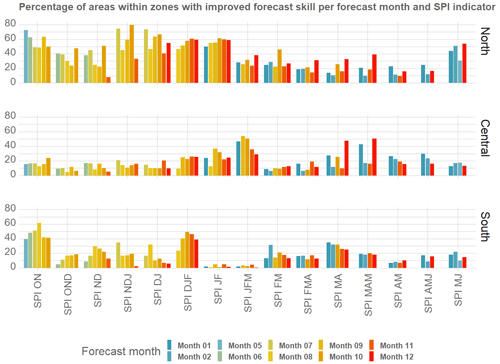
Figure 6Percentage of zonal areas in which skill was gained using bias correction for different lead times of the forecast used to extract the SPI 2 and SPI 3 indicators.
Overall, the north zone showed the highest mean percentage of improved forecast areas (38 %), followed by the central and south zones (both at 19 %). In the north zone, the forecast month with the highest mean improvement was July (56 %), while February had the lowest (20 %). For the central zone, January showed the greatest improvement (26 %), while August showed the least (10 %). In the south zone, July and August had the highest mean improvement (26 %), whereas December and January had the lowest (14 %). Across all forecast months, the SPI indicators that demonstrated the greatest skill improvement were SPI ON, SPI DJ and SPI NDJ for the north zone; SPI JFM for the central zone; and SPI ON for the south zone. Most of these indicators pertain to the first window of the rainy season in the country.
Additionally, for all districts and all SPI 2 and SPI 3 indicators across all lead times, 24 % demonstrated improved skill (measured by AUROC score) after bias correction compared to the raw forecast. A more detailed overview of the AUROC scores can be found in Sect. 3.3.
3.3 Overview of the maximum AUROC score
Figure 7 shows the mean AUROC index per district for predicting severe droughts, combining outcomes from both raw and bias-corrected forecasts across all extracted SPI 2 and SPI 3 periods and lead times. On average, the SPI DJ indicator had the highest AUROC score (0.79), while SPI AM had the lowest (0.63). Severe drought events are generally more predictable during the early to mid-rainy season (average AUROC score of 0.76 for window 1; see Table 2 for indicator details) compared to the middle to late rainy season (average AUROC score of 0.69 for window 2). In particular, the predictability of severe droughts in districts located in the south zone is notably high during window 1 (average AUROC = 0.77), primarily driven by high forecast accuracy in December and January (SPI 2 DJ). In the central and north zones, severe droughts are most predictable during December to February (average AUROC of 0.78) and November to January (average AUROC of 0.80), respectively.
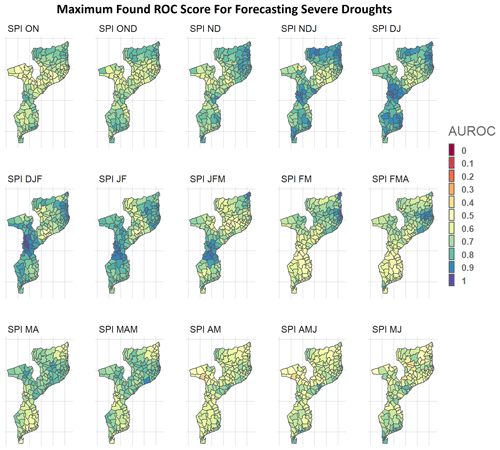
Figure 7Overview of the maximum AUROC score across lead times combining outcomes of both raw and bias-corrected forecasts.
In Sect. S4, we highlight the lead times that yield the highest forecast skill for severe drought prediction. In the south zone, about 44 % of districts achieve the highest AUROC score using the December forecast for SPI DJ. In the central zone, 55 % of districts achieve their best performance using the August forecast for SPI DJF. In the north zone, around 66 % of districts see their highest AUROC scores based on the November forecast for SPI NDJ.
However, it is crucial to note that the implementation of AA requires at least 1 full month for the “Go!” phase (see Table 1 for criteria). As a result, forecasts released in November, which predict severe droughts between November and January, are not used in operational mode. This means that the Ready, Set & Go! trigger system often cannot rely on the most accurate lead times, as they do not allow enough time for action mobilization.
After determining whether to use the raw or bias-corrected forecast for a specific lead time, SPI indicator and district, we move to the most computationally intensive phase of the Ready, Set & Go! trigger system. This phase involves testing pairs of triggers for AA, as described in the section “Testing several triggers for the Set & Go! system”. The testing is conducted in 1 % increments, ranging from 0 % to 100 %, resulting in 10 201 combinations of candidate triggers per district, forecast month pair and SPI 2 and SPI 3 indicator. After testing all combinations and recording their statistical performance, only the best-performing trigger pair for each window is selected for presentation in the next section. The statistical performance of triggers, for the different scenarios, is based on the overall performance using hindcasts from 1993 and 2021 compared to observed SPI 2 and SPI 3 values within this period.
All selected trigger pairs must meet the quality criteria outlined in Table 1. To evaluate the value of using mixed forecast information (raw and bias corrected) with a double-confirmation approach, we expanded the analysis to include additional testing. This extended analysis examines the performance of single versus double triggers and the impact of including or excluding bias correction in the methodology.
3.4 Sensitivity analysis
Table 3 presents the average performance of the best triggers for AA during both window 1 and window 2, comparing different activation mechanisms:
-
Scenario 1 issues an AA advisory based on a single alert using only the raw SPI forecasts from a specific lead time. If the forecast for a specific month, district and indicator exceeds the assigned probabilistic trigger, an AA advisory is issued and implemented.
-
Scenario 2 issues an AA advisory based on a single alert, using either raw or bias-corrected SPI forecasts, depending on which has higher predictive skill.
-
Scenario 3 requires double confirmation of drought conditions but uses only raw SPI forecasts.
-
Scenario 4 represents the operational Ready, Set & Go! system, which issues an AA advisory based on double confirmation, using a combination of both bias-corrected and raw SPI forecasts.
Overall, scenarios using a double-confirmation approach perform better than those relying on a single drought alert for AA activation.
Table 3Sensitivity analysis of different approaches for establishing an AA drought trigger system for the two menus of triggers. Statistics of the different scenarios are based on the average of the best-performing SPI 2 or SPI 3 indicator for AA within windows 1 and 2.
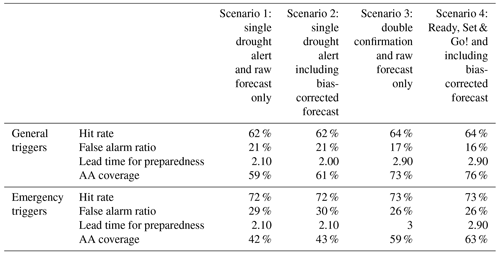
Specifically, in the simplest scenario (Scenario 1), 59 % of districts in Mozambique would be covered by a general AA trigger, while 42 % would be covered by an emergency trigger (see the section “Applying pre-mapped quality criteria for the trigger choice” for definitions of these trigger types). This indicates that raw forecasts alone provide reasonably accurate severe drought predictions for many districts. Incorporating bias correction (Scenario 2) only marginally increases coverage to 61 % (general trigger) and 43 % (emergency trigger).
However, applying a double-confirmation approach significantly increases the proportion of districts covered by an AA trigger. In Scenario 3, coverage increases to 73 % (general trigger) and 59 % (emergency trigger). Scenario 4, which is the operational system in Mozambique, achieves the highest national AA coverage across all approaches. Additionally, the Ready, Set & Go! system improves both the hit rate and reduces the false alarm ratio compared to single-alert systems (Scenarios 1 and 2). Furthermore, the Ready, Set & Go! approach extends the lead time for preparedness activities. While single-alert scenarios provide, on average, 2 months of lead time for AA implementation once the trigger is exceeded, the Ready, Set & Go! system increases this lead time to nearly 3 months.
3.5 Spatial overview of the Ready, Set & Go! system
Figure 8 provides a detailed spatial statistical overview of the performance of the Ready, Set & Go! triggers, complementing the results for Scenario 4 presented in Sect. 3.4. As noted earlier, severe droughts are predicted with greater skill in window 1 compared to window 2, allowing for AA triggers to be assigned to more districts in window 1. The percentage of districts with a valid AA trigger is as follows: (i) 66 % for the emergency trigger menu in window 1 and 59 % in window 2 and (ii) 87 % for the general trigger menu in window 1 and 64 % in window 2. Notably, every district with an emergency AA trigger also has a general AA trigger, indicating that for most districts, AA triggers can be adjusted annually based on current vulnerability levels. However, in some cases, the general trigger is the only applicable option.
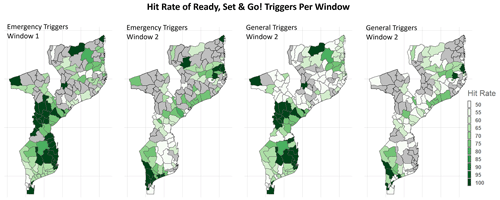
Figure 8Hit rate of the Ready, Set & Go! trigger system for severe droughts for two trigger menus (emergency and general) and two windows of intervention (window 1 and window 2). No triggers for the Ready, Set & Go! for severe droughts were found for the districts in grey.
In terms of trigger performance across windows, the central zone showed the highest and lowest mean hit rates, with window 1 achieving 74 % and window 2 achieving 61 %. Across all menus and windows, the emergency menu in window 1 had the highest mean hit rate (77 %), while the general menu in window 2 had the lowest (61 %). This result is expected, as the emergency menu is designed for higher hit rates, particularly given the greater predictability of severe droughts in window 1.
In addition to the highest drought predictability, the south zone of Mozambique also exhibited the highest total AA coverage, with an average of 86 % of districts having an AA trigger. The highest single window and trigger menu coverage was in the south zone under the general menu, with 97 % of districts having a trigger. Spatial differences in trigger performance were also observed between neighbouring provinces, such as Manica and Tete in window 1 under the general menu. These differences could be driven by varying forecast skill levels. For instance, the AUROC scores for the general trigger in window 1 are 0.82 for Manica and 0.68 for Tete. Factors contributing to these differences could include under- or over-estimation of rainfall events used to verify forecasts in Mozambique (as noted in a previous study by Toté et al., 2015), numerical effects from data rescaling, and the resolution of district-level assessments using CHIRPS and ECMWF forecasts.
Regarding the average false alarm ratio of the triggers across different windows (Fig. 9), the highest and lowest ratios are observed in the south zone for window 2 (20 %) and the central zone for window 1 (10 %), respectively. Across various menus and windows, the emergency menu and window 2 exhibit the highest false alarm ratio (16 %), while the general menu and window 1 have the lowest (10 %). This pattern is expected, as the emergency menu is designed to tolerate a higher false alarm ratio to ensure a higher hit rate, making it less prone to missing a drought forecast.
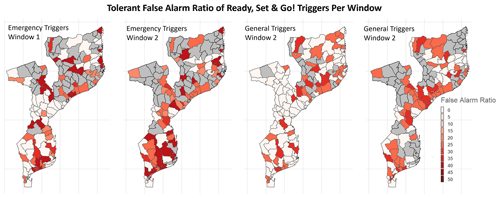
Figure 9False alarm ratio of the Ready, Set & Go! trigger system for severe droughts for two trigger menus (emergency and general) and two windows of intervention (window 1 and window 2). No triggers for the Ready, Set & Go! for severe droughts were found for the districts in grey.
Section S5 details the specific SPI indicators used for AA triggers. For window 1, SPI DJ is the most commonly selected indicator across all zones. In window 2, different SPIs are chosen per zone: (i) SPI FMA for the north zone, (ii) SPI JFM for the central zone and (iii) SPI DJF for the south zone.
Regarding lead times, the earliest “Ready” alert for preparedness in window 1 can be issued for a few districts in the south zone based on the May forecast. However, for most districts in the south zone, the July forecast is used for preparedness, whereas in the north and central zones, the September forecast is most commonly used for the “Ready” alert. In window 2, most districts in the south zone use the August forecast for preparedness, while the north and central zones typically use the October forecast.
It is important to note that regional rainfall climatology significantly influences the choice of intervention windows and indicators. As a result, districts in the south zone may receive readiness alerts earlier in the season compared to other areas. This factor is crucial for planning AA activities and allocating geographical funding.
In this study, we present the methodology behind the operational Ready, Set & Go! trigger system used by Mozambican governmental institutions and their partners to guide AA activities against droughts. The system optimizes the use of seasonal forecast information by identifying triggers for AA through a double-confirmation process. This approach combines longer and shorter lead time forecasts to issue more-reliable drought alerts. Our findings indicate that by utilizing both bias-corrected and raw ensemble rainfall forecasts, AA efforts could potentially be scaled up to cover the entire rainy season in 76 % of Mozambique's districts. If focused solely on the first part of the rainy season, where drought predictability is higher, AA activities could expand to 87 % of all districts. This demonstrates that seasonal forecasts can reliably inform AA months before the onset of severe droughts, meeting the quality criteria established by multiple institutions. Such scalability indicates strong potential for expanding current AA pilots nationwide, supporting the ambitious goals of the Maputo Declaration, in which southern Africa governments committed to extending early warning systems across the region (SADC, 2022). Globally, the Ready, Set & Go! system also aligns with the Early Warning for All initiative, which aims to ensure that every individual worldwide is protected from climate events through early warning systems by 2027 (WMO, 2022). This initiative underscores the need for expanding the climate information portfolio of national meteorological and hydrological services for direct application in disaster risk management. However, there are still limitations and opportunities for improvements, which we discuss in the following sections.
This study demonstrates that the Ready, Set & Go! trigger system can effectively issue severe drought alerts using SPI 2 and SPI 3 indicators, which the technical working group in Mozambique has deemed suitable for monitoring and anticipating drought risks in agricultural systems. However, these indicators and thresholds are not flawless in detecting drought damage, as the relationship between drought risk and impact is often location-specific, is non-linear and is influenced by non-climatic factors such as vulnerability (Brida et al., 2013; Silva and Matyas, 2014). The ideal method for establishing AA thresholds that reliably detect drought-related losses would involve a historical analysis examining the connection between drought events and socio-economic impacts, such as crop yields, income losses, health outcomes and food security. Past studies on index-based insurance for the agricultural sector have extensively explored the gap between rainfall measurements and actual agricultural losses, highlighting challenges in accurately capturing real-world farmer impacts (Clarke and Dercon, 2009; Clement et al., 2018; Greatrex et al., 2015). Unfortunately, comprehensive, downscaled impact data are largely unavailable, particularly across African countries, limiting further refinement of thresholds and indicators within the system and hindering the ability to solidify links between drought conditions and past impacts. Future efforts should focus on refining these thresholds to strengthen the relationship between physical drought hazards and expected impacts. This could be achieved by utilizing spatially explicit socio-economic datasets, such as the Integrated Food Security Phase Classification indicator from the Famine Early Warning Systems Network, along with data recovery exercises. This would allow users to better understand food security outcomes tied to drought events.
Additionally, the Ready, Set & Go! system issues drought alerts based on a multi-month SPI indicator, which can overlook the effects of short but impactful dry spells, poorly distributed rainfall, intense rainfall episodes or delayed/early cessation of rain. Incorporating additional drought indicators could help better capture these risks, ideally through an exploratory analysis that links specific drought indicators to negative impacts and evaluates their predictability.
Two technical aspects related to the extraction of the SPI indicator also require further improvement. First, more-sensitive statistical tests could be used to identify candidate probability distributions for normalizing drought indices. Although this study applies the two-parameter gamma distribution, as recommended by Stagge et al. (2015), a more rigorous assessment of the assumed SPI distributions could be beneficial. Second, the handling of zero precipitation poses challenges, particularly in regions with very low seasonal rainfall. In this system, zero-precipitation events are accounted for by assigning SPI values based on their historical occurrence. However, this approach can be problematic when many zero values are present, as SPI requires a mean value of 0 to reflect typical conditions, where half of the years are wetter, and half are drier. While the presence of zero precipitation was rare in this study, further refinement is needed to handle these cases more effectively. Using a method such as the centre of probability mass, as suggested by Stagge et al. (2015), could offer a more robust approach to calculating SPI in extremely dry regions.
The Ready, Set & Go! trigger system aims to extend AA and reliable early warning information to all districts in Mozambique. Although we have not yet fully achieved this goal using our current technique, we believe that refining the bias correction methodology will enhance the system's effectiveness. Bias correction is a critical element in precipitation forecasts, with QM being one of the most commonly applied techniques. In developing an AA trigger system, we designed and evaluated a bias correction methodology to improve the accuracy of seasonal forecast in predicting severe droughts. While our methodology has increased forecast for 24 % of the predicted SPI at the district level and expanded AA coverage by 4 % (as shown in Table 3, comparing Scenario 3 to Scenario 4), there is still potential to further enhance the bias correction approach. Below, we outline the improvements that can be made.
Firstly, our method uses an ENSO-informed quantile mapping transfer function to correct the SPI forecast based on the SPI reference value of the pixel under investigation and its nine neighbouring pixels conditioned on the state of ENSO. This process ensures that the bias correction accounts for variations in the SPI quantities according to the climatology of different ENSO phases, effectively capturing relevant global processes (Manzanas and Gutiérrez, 2019; Maraun et al., 2017). In practice, this involves splitting SPI time series, derived from both CHIRPS and ECMWF ensemble forecasts, into neutral, La Niña and El Niño years depending on the ENSO phase (detailed in Sect. S1). However, in some regions of Mozambique, such as part of Tete, the ENSO rainfall signal is weak, particularly during October to December (WFP, 2018). Therefore, relying solely on an ENSO-based approach may not be ideal in these areas. Other climate variability modes, such as the Indian Ocean Dipole, are also known to influence annual rainfall variability in Mozambique (Ficchì et al., 2021; Harp et al., 2021; Ogwang et al., 2021). This suggests a need to investigate the suitability of incorporating additional teleconnection modes into the bias correction process.
Second, since extreme droughts generally affect broad areas rather than single locations (Eskridge et al., 1997; Liu et al., 2021), our bias correction methodology accounts for the spatial dependence of SPI. To bias-correct a single grid point of the SPI ensemble forecast, we incorporate data from multiple grid points (the target grid point and its nine neighbours) from the reference SPI dataset to build the transfer function. Previous research has shown that addressing spatial dependence reduces bias in climate model outputs (Cannon, 2018; Nahar et al., 2018). To avoid overfitting, we use a leave-one-year-out cross-validation scheme, excluding the year being bias corrected from the transfer function. For the spatial dependence set-up, we tested two k values (4 and 9), ultimately selecting 9 based on improved spatial homogeneity of AUROC scores. However, this approach could benefit from further optimization by assessing the k value that yields the highest AUROC scores for specific locations.
Third, improvements in bias correction may be achieved by exploring emerging methodologies such as machine learning (ML). Recent studies indicate that ML has the potential to outperform traditional techniques like QM (e.g. Yoshikane and Yoshimura, 2023; Zarei et al., 2021). Lastly, our initial internal tests showed significant improvements in drought predictability by creating a transfer function that directly links SPI forecasts to SPI observations rather than taking the traditional approach of bias correcting daily or monthly raw rainfall forecasts before converting them into SPI values. This direct approach has led to both statistical and practical gains, as it allows the system to focus directly on drought detection. If the system evolves to include additional rainfall-based indicators, such as dry spells or the start/cessation of rain, a method that directly bias-corrects raw forecasts could offer operational advantages, as it can be widely applied to generate additional indicators.
We also highlight the potential to scale up AA by utilizing rainfall seasonal forecasts from the ECMWF. In our approach, the seasonal forecast is downscaled from 1 to 0.25° using bilinear interpolation, which allows us to assess forecasting skill at the district level. Extracting drought alerts at the district level is crucial to align with the geographical targeting of AA interventions. However, further investigation into other downscaling techniques, such as ML, could be beneficial, as ML has been shown to enhance forecast skill (Jin et al., 2023). ECMWF was initially selected as our primary source of forecasting information due to its superior skill in predicting precipitation over the African continent compared to other centres (Gebrechorkos et al., 2022). Nevertheless, future studies may benefit from shifting from a single-model approach to a multi-model ensemble (MME) strategy. MME integrates independent models from various forecasting centres of information, which helps mitigate model errors and can enhance the reliability of seasonal outlooks (Doblas-Reyes et al., 2010; Gebrechorkos et al., 2022; Rozante et al., 2014).
We demonstrate that the Ready, Set & Go! system improves the accuracy of AA advisories, resulting in a higher hit rate and a lower false alarm ratio compared to a system that relies on a single alert for AA advisories. Additionally, we observe that this system extends the lead time for preparedness activities, allowing for the scale-up of AA efforts against severe droughts during the first window of the rainy season, covering 87 % of districts in Mozambique. However, since AA triggers are identified and optimized at the district level, the system is prone to issuing advisories for individual districts, even though past severe droughts have often had broader impacts, including widespread socio-economic consequences (Baez et al., 2020). This discrepancy may occur because the system uses different lead times for forecasting information across districts within the same province or because triggers for different implementation windows within a province are based on varying SPI indicators. An example of this can be seen in southern Mozambique (refer to Sect. S5). Despite these statistical gains, optimizing AA triggers at the district level needs to be contextualized for practical decision-making, particularly for large-scale operations and the distribution and management of funding. Therefore, while district-level optimization may be effective statistically, it may not always be the most appropriate approach for AA planning, especially when scaling up AA across the entire country. One potential solution to avoid asynchrony in AA triggers is to refine the selection of indicators and lead times by evaluating their performance across the majority of districts within a province, ensuring more synchronized and coordinated AA efforts.
We also demonstrate that the triggers for the Ready, Set & Go! system can be adjusted based on vulnerability information, adding an important nuance to AA operations (Baez et al., 2020). However, measuring vulnerability is a complex task that often requires frequent updates, location-specific data, and further disaggregation by age and gender (Chaves-Gonzalez et al., 2022). In Mozambique, the Technical Secretariat for Food Security and Nutrition (SETSAN) is responsible for providing such information. AA operations would greatly benefit if these data were made available in a timely manner, ideally before the start of the AA season. Unfortunately, this is not always the case. More research is needed to understand vulnerability trends and their relationship to climate hazards (Baez et al., 2020; Hallegatte et al., 2016). As the system expands, collecting timely vulnerability data may become increasingly challenging. Therefore, a systematic, rapid, yet robust methodology for vulnerability analysis is essential. We have also observed a lower percentage of districts covered by AA when emergency triggers – modulated by vulnerability – are used. These emergency triggers inherently allow for a higher rate of false alarms and focus on “no-regret” actions (Chaves-Gonzalez et al., 2022) while increasing the probability of detection. This approach aims to maximize the number of extreme droughts anticipated by AA interventions and provide a safety net for areas with high vulnerability. However, the current criteria for identifying emergency triggers are not achieving higher coverage compared to general triggers. Revisiting these criteria (see Table 1) through a statistical optimization process may help enhance the system's effectiveness.
As previously mentioned, the Ready, Set & Go! system is currently being piloted in 11 districts across Mozambique, with plans to scale up AA operations in 2024. Due the 2023–2024 El Niño, several AA advisories have already been issued to districts in the Gaza, Sofala and Tete provinces, marking the system's first operational deployment during the 2023–2024 rainy season. While humanitarian and governmental organizations have substantial experience in responding to hazards after they occur, most monitoring and evaluation (M&E) efforts have focused on the effects of emergency responses post-crisis. There is limited evidence of the benefits of AA, particularly regarding drought interventions, partially given the small number of pilot interventions to date and the challenges faced by studies in benefit estimations and/or modelling. As the evidence base for the value for money begins to form, WFP's AA programmes are showing potential as a sustainable way to support climate-vulnerable governments with limited resources (Anticipatory Action for Climate Shocks, 2024). In Kenya, drought-related AA could save up to USD 20 billion over 20 years, even with false alarms costing significantly less than a late response. In Ethiopia, Kenya and Somalia, AA could save USD 1.6 billion over 15 years by mitigating drought impacts before price spikes and negative coping strategies. In Nepal, AA reduced damage to vulnerable populations by 75 % and cuts asset losses by 50 %, saving USD 34 for every dollar invested and reducing long-term recovery costs. In Zimbabwe, AA reached 32 500 people before drought impacts, with 97 % of farmers benefiting from climate information and 80 % adapting their practices, leading to higher resilience than a control group.
Given that AA represents an innovative approach and a relatively new concept in risk management, it is crucial to establish a robust M&E system to evaluate the effectiveness of AA interventions. This system will provide valuable insights into what has worked well in practice and highlight areas for improvement in future operations. Ultimately, a well-designed M&E process will help determine whether AA interventions effectively reduce or mitigate the impacts of droughts on affected populations (Gros and Heinrich, 2021).
In this article, we introduced and benchmarked the “Ready, Set & Go!” system, which is being piloted in Mozambique to trigger anticipatory action against severe droughts. This system is designed to implement measures that mitigate the impacts of rainfall deficits during the critical period between forecasting and the onset of drought. Following the recent adoption of the SADC Maputo Declaration by its member states, there is a need to evaluate the system's opportunities and limitations for expanding drought AA coverage to all districts in Mozambique. Our findings include the following:
-
Potential for expansion. The Ready, Set & Go! system could potentially scale AA activities to 76 % of Mozambican districts. Additionally, 63 % of these districts could adopt an alternative trigger system tailored to vulnerability levels. This feature allows the system to proactively address potential vulnerabilities for the upcoming season. If only the first window of the rainy season is targeted, coverage could increase to 87 %.
-
Impact of bias correction. The bias correction methodology used in the Ready, Set & Go! system enhances forecasting skill for 24 % of all forecasted SPI indicators at the district level. This improvement slightly raises AA coverage from 73 % to 76 % for the general menu and from 59 % to 63 % for the emergency menu. This means bias correction can extend operational AA coverage to about six additional districts, representing a slight improvement but also enhancing the potential for life-saving AA.
-
Increased hit rate and lead time. The Ready, Set & Go! system improves both the hit rate and lead time for AA compared to three alternative triggering approaches. The highest mean hit rate across different windows was observed in the central zone within window 1 (74 %). SPI DJ is the most commonly used indicator for AA in window 1. The earliest “Ready” alert for preparedness can be issued for a few districts in the south zone based on the May forecast.
-
Reduced false alarm ratio. The Ready, Set & Go! system achieves a lower false alarm ratio compared to the three alternative approaches. The mean lowest average false alarm ratio is found in the central zone for window 1 (10 %). Among different menus and windows, the mean highest false alarm ratio is 21 % for the emergency menu in window 2, while the mean lowest is 10 % for the general menu in window 1.
We observed that the piloted drought EWS has significant potential for scaling up AA across Mozambique, aligning with the goals of the Maputo Declaration and the Early Warning for All initiative to provide climate event coverage and protection to all citizens by 2027. However, several next steps could further enhance the effectiveness of the EWS.
Enhancing bias correction methodology to
-
explore additional climate indices to incorporate more indices related to climate variability to refine the transfer function.
-
optimize nearest neighbours to fine-tune the number of nearest neighbours used in bias correction.
-
investigate emerging techniques to explore advanced methods such as machine learning to improve accuracy.
Improving forecast resolution to
-
explore downscaling techniques to investigate alternative downscaling methods to enhance the resolution of seasonal forecasts.
-
consider multi-model ensemble approaches to evaluate whether combining multiple models could improve the reliability of seasonal outlooks.
Strengthening impact links to
-
connect thresholds to socio-economic impacts to enhance understanding of the socio-economic consequences of droughts to better plan and target AA activities.
-
incorporate additional indicators to include other relevant drought indicators, such as the onset of rain and rainfall cessation, to provide a more comprehensive assessment.
Contextualizing trigger optimization to
-
refine triggers for practical decision-making to consider the impact of optimizing triggers at the district level, which may lead to asynchrony in AA activations among neighbouring districts. Select SPI 2 or SPI 3 indicators and lead times based on their performance across most districts within a province.
Investing in monitoring and evaluation to
-
support ongoing pilots to invest in monitoring, evaluation and learning to inform future expansion of the anticipatory approach and maximize the impact of AA activities.
These steps may help to maximize the effectiveness and coverage of the EWS, ensuring that AA efforts are timely, more accurate and well targeted.
Data will be made available on request.
The supplement related to this article is available online at: https://doi.org/10.5194/nhess-24-4661-2024-supplement.
GGN: conceptualization, data curation, visualization, writing, reviewing and editing. JT: writing, reviewing and editing. BN: conceptualization, writing, reviewing and editing. CJdCMM: conceptualization, writing, reviewing and editing. IR: conceptualization, writing, reviewing and editing. MP: conceptualization, data curation, writing, reviewing and editing. SQ: data curation, writing, reviewing and editing. RMLPB: conceptualization, writing, reviewing and editing.
The contact author has declared that none of the authors has any competing interests.
Publisher's note: Copernicus Publications remains neutral with regard to jurisdictional claims made in the text, published maps, institutional affiliations, or any other geographical representation in this paper. While Copernicus Publications makes every effort to include appropriate place names, the final responsibility lies with the authors.
This article is part of the special issue “Drought, society, and ecosystems (NHESS/BG/GC/HESS inter-journal SI)”. It is not associated with a conference.
This research has been supported by the Direktoratet for Utviklingssamarbeid (grant no. 70001923).
This paper was edited by Floris van Ogtrop and reviewed by Daniel Osgood and one anonymous referee.
Anticipatory Action for Climate Shocks: Anticipatory Action for climate shocks, https://www.wfp.org/anticipatory-actions (last access: 21 August 2024), 2024.
Anticipatory action in the world: Anticipatory action at a glance, https://www.anticipation-hub.org/experience/global-map (last access: 21 August 2024), 2024.
Araneda-Cabrera, R. J., Bermudez, M., and Puertas, J.: Revealing the spatio-temporal characteristics of drought in Mozambique and their relationship with large-scale climate variability, J. Hydrol.: Reg. Stud., 38, 100938, https://doi.org/10.1016/j.ejrh.2021.100938, 2021.
Ashok, K., Guan, Z., and Yamagata, T.: Impact of the Indian Ocean dipole on the relationship between the Indian monsoon rainfall and ENSO, Geophys. Res. Lett., 28, 4499–4502, https://doi.org/10.1029/2001GL013294, 2001.
Baez, J. E., Caruso, G., and Niu, C.: Extreme Weather and Poverty Risk: Evidence from Multiple Shocks in Mozambique, Econ. Disast. Clim. Change, 4, 103–127, https://doi.org/10.1007/s41885-019-00049-9, 2020.
Behera, S. K. and Yamagata, T.: Subtropical SST dipole events in the southern Indian Ocean, Geophys. Res. Lett., 28, 327–330, https://doi.org/10.1029/2000GL011451, 2001.
Blamey, R. C., Kolusu, S. R., Mahlalela, P., Todd, M. C., and Reason, C. J. C.: The role of regional circulation features in regulating El Niño climate impacts over southern Africa: A comparison of the 2015/2016 drought with previous events, Int. J. Climatol., 38, 4276–4295, https://doi.org/10.1002/joc.5668, 2018.
Brida, A. B., Owiyo, T., and Sokona, Y.: Loss and damage from the double blow of flood and drought in Mozambique, Int. J. Glob. Warm., 5, 514–531, https://doi.org/10.1504/IJGW.2013.057291, 2013.
Cannon, A. J.: Multivariate quantile mapping bias correction: an N-dimensional probability density function transform for climate model simulations of multiple variables, Clim. Dynam., 50, 31–49, https://doi.org/10.1007/s00382-017-3580-6, 2018.
Chaves-Gonzalez, J., Milano, L., Omtzigt, D.-J., Pfister, D., Poirier, J., Pople, A., Wittig, J., and Zommers, Z.: Anticipatory action: Lessons for the future, Front. Clim., 4, 932336, https://doi.org/10.3389/fclim.2022.932336, 2022.
Clarke, D. and Dercon, S.: Insurance, credit and safety nets for the poor in a world of risk, DESA working paper no. 81, United Nations Department of Economic & Social Affairs, New York, USA, 2009.
Clement, K. Y., Wouter Botzen, W. J., Brouwer, R., and Aerts, J. C. J. H.: A global review of the impact of basis risk on the functioning of and demand for index insurance, Int. J. Disast. Risk Reduct., 28, 845–853, https://doi.org/10.1016/j.ijdrr.2018.01.001, 2018.
De Ruiter, M. C., Couasnon, A., van den Homberg, M. J. C., Daniell, J. E., Gill, J. C., and Ward, P. J.: Why we can no longer ignore consecutive disasters, Earth's Future, 8, e2019EF001425, https://doi.org/10.1029/2019ef001425, 2020.
Doblas-Reyes, F. J., Déqué, M., and Piedelievre, J.-P.: Multi-model spread and probabilistic seasonal forecasts in PROVOST, Q. J. Roy. Meteorol. Soc., 126, 2069–2087, https://doi.org/10.1002/qj.49712656705, 2010.
Echo Flash: https://erccportal.jrc.ec.europa.eu/ECHO-Products/Echo-Flash#/daily-flash-archive/4117 (last access: 27 October 2023), 2023.
Eskridge, R. E., Ku, J. Y., Rao, S. T., Porter, P. S., and Zurbenko, I. G.: Separating Different Scales of Motion in Time Series of Meteorological Variables, B. Am. Meteorol. Soc., 78, 1473–1483, https://doi.org/10.1175/1520-0477(1997)078<1473:SDSOMI>2.0.CO;2, 1997.
Fawcett, T.: An introduction to ROC analysis, Pattern Recognit. Lett., 27, 861–874, https://doi.org/10.1016/j.patrec.2005.10.010, 2006.
Ficchì, A., Cloke, H., Neves, C., Woolnough, S., Coughlan de Perez, E., Zsoter, E., Pinto, I., Meque, A., and Stephens, E.: Beyond El Niño: Unsung climate modes drive African floods, Weather Clim. Extrem., 33, 100345, https://doi.org/10.1016/j.wace.2021.100345, 2021.
Funk, C., Peterson, P., Landsfeld, M., Pedreros, D., Verdin, J., Shukla, S., Husak, G., Rowland, J., Harrison, L., Hoell, A., and Michaelsen, J.: The climate hazards infrared precipitation with stations – A new environmental record for monitoring extremes, Sci. Data, 8, 150066, https://doi.org/10.1038/sdata.2015.66, 2015.
Gebrechorkos, S. H., Pan, M., Beck, H. E., and Sheffield, J.: Performance of State-of-the-Art C3S European Seasonal Climate Forecast Models for Mean and Extreme Precipitation Over Africa, Water Resour. Res., 58, e2021WR031480, https://doi.org/10.1029/2021WR031480, 2022.
Greatrex, H., Hansen, J., Garvin, S., Diro, R., Le Guen, M., Blakeley, S., Rao, K., and Osgood, D.: Scaling up index insurance for smallholder farmers: Recent evidence and insights, CCAFS Rep., https://cgspace.cgiar.org/items/34d65f48-ad14-409b-9e4e-b6da7d28adda (last access: 12 December 2024), 2015.
Gros, C. and Heinrich, D.: Monitoring and evaluation of anticipatory actions for fast and slow-onset hazards: Guidance and tools for Forecast-based Financing, https://www.wfp.org/publications/monitoring-and-evaluation-anticipatory-actions-fast-and-slow (last access: 12 December 2024), 2021.
Guimarães Nobre, G., Pasqui, M., Quaresima, S., Pieretto, S., and Lemos Pereira Bonifácio, R. M.: Forecasting, thresholds, and triggers: Towards developing a Forecast-based Financing system for droughts in Mozambique, Clim. Serv., 30, 100344, https://doi.org/10.1016/j.cliser.2023.100344, 2023.
Hallegatte, S., Bangalore, M., Bonzanigo, L., Fay, M., Kane, T., Narloch, U., Rozenberg, J., Treguer, D., and Vogt-Schilb, A.: Shock Waves: Managing the Impacts of Climate Change on Poverty, World Bank, Washington, D.C., https://doi.org/10.1596/978-1-4648-0673-5, 2016.
Harp, R. D., Colborn, J. M., Candrinho, B., Colborn, K. L., Zhang, L., and Karnauskas, K. B.: Interannual Climate Variability and Malaria in Mozambique, GeoHealth, 5, e2020GH000322, https://doi.org/10.1029/2020GH000322, 2021.
Hart, N. C. G., Reason, C. J. C., and Fauchereau, N.: Tropical–Extratropical Interactions over Southern Africa: Three Cases of Heavy Summer Season Rainfall, Mon. Weather Rev., 138, 2608–2623, https://doi.org/10.1175/2010MWR3070.1, 2010.
Jin, H., Jiang, W., Chen, M., Li, M., Bakar, K. S., and Shao, Q.: Downscaling long lead time daily rainfall ensemble forecasts through deep learning, Stoch. Environ. Res. Risk A., 37, 3185–3203, https://doi.org/10.1007/s00477-023-02444-x, 2023.
Liu, Z., Xie, Y., Cheng, L., Lin, K., Tu, X., and Chen, X.: Stability of spatial dependence structure of extreme precipitation and the concurrent risk over a nested basin, J. Hydrol., 602, 126766, https://doi.org/10.1016/j.jhydrol.2021.126766, 2021.
Lloyd-Hughes, B. and Saunders, M. A.: A drought climatology for Europe, Int. J. Climatol., 22, 1571–1592, https://doi.org/10.1002/JOC.846, 2002.
Lopez, A., Coughlan de Perez, E., Bazo, J., Suarez, P., van den Hurk, B., and van Aalst, M.: Bridging forecast verification and humanitarian decisions: A valuation approach for setting up action-oriented early warnings, Weather Clim. Extrem., 27, 100167, https://doi.org/10.1016/j.wace.2018.03.006, 2018.
Lyon, B. and Mason, S. J.: The 1997–98 Summer Rainfall Season in Southern Africa. Part I: Observations, J. Climate, 20, 5134–5148, https://doi.org/10.1175/JCLI4225.1, 2007.
Manatsa, D., Matarira, C. H., and Mukwada, G.: Relative impacts of ENSO and Indian Ocean dipole/zonal mode on east SADC rainfall, Int. J. Climatol., 31, 558–577, https://doi.org/10.1002/joc.2086, 2011.
Manhique, A. J., Reason, C. J. C., Silinto, B., Zucula, J., Raiva, I., Congolo, F., and Mavume, A. F.: Extreme rainfall and floods in southern Africa in January 2013 and associated circulation patterns, Nat. Hazards, 77, 679–691, https://doi.org/10.1007/s11069-015-1616-y, 2015.
Manhique, A. J., Guirrugo, I. A., Nhantumbo, B. J., and Mavume, A. F.: Seasonal to Interannual Variability of Vertical Wind Shear and Its Relationship with Tropical Cyclogenesis in the Mozambique Channel, Atmosphere, 12, 739, https://doi.org/10.3390/atmos12060739, 2021.
Manzanas, R. and Gutiérrez, J. M.: Process-conditioned bias correction for seasonal forecasting: a case-study with ENSO in Peru, Clim. Dynam., 52, 1673–1683, https://doi.org/10.1007/s00382-018-4226-z, 2019.
Maraun, D., Shepherd, T. G., Widmann, M., Zappa, G., Walton, D., Gutiérrez, J. M., Hagemann, S., Richter, I., Soares, P. M. M., Hall, A., and Mearns, L. O.: Towards process-informed bias correction of climate change simulations, Nat. Clim. Change, 7, 764–773, https://doi.org/10.1038/nclimate3418, 2017.
Mawren, D., Hermes, J., and Reason, C. J. C.: Exceptional Tropical Cyclone Kenneth in the Far Northern Mozambique Channel and Ocean Eddy Influences, Geophys. Res. Lett., 47, e2020GL088715, https://doi.org/10.1029/2020GL088715, 2020.
Nahar, J., Johnson, F., and Sharma, A.: Addressing Spatial Dependence Bias in Climate Model Simulations – An Independent Component Analysis Approach, Water Resour. Res., 54, 827–841, https://doi.org/10.1002/2017WR021293, 2018.
National Center for Atmospheric Research Staff (Eds.): The Climate Data Guide: Regridding Overview, https://climatedataguide.ucar.edu/climate-tools/regridding-overview (last access: 13 December 2024), 2014.
OCHA: Report on the RIASCO Action Plan for the El Niño – Induced Drought in Southern Africa 2016/2017, https://www.unocha.org/publications/report/world/report-riasco-action-plan-el-ni-o-induced-drought-southern (last access: 12 December 2024), 2017.
Ogwang, B. A., Ongoma, V., Shilenje, Z. W., Ramotubei, T. S., Letuma, M. N. J.: Influence of Indian Ocean dipole on rainfall variability and extremes over southern Africa, MAUSAM, 71, 637–648, https://doi.org/10.54302/mausam.v71i4.50, 2021.
Rapolaki, R. S., Blamey, R. C., Hermes, J. C., and Reason, C. J. C.: A classification of synoptic weather patterns linked to extreme rainfall over the Limpopo River Basin in southern Africa, Clim. Dynam., 53, 2265–2279, https://doi.org/10.1007/s00382-019-04829-7, 2019.
Ratri, D. N., Whan, K., and Schmeits, M.: A Comparative Verification of Raw and Bias-Corrected ECMWF Seasonal Ensemble Precipitation Reforecasts in Java (Indonesia), J. Appl. Meteorol. Clim., 58, 1709–1723, https://doi.org/10.1175/JAMC-D-18-0210.1, 2019.
Reason, C. J. C. and Keibel, A.: Tropical cyclone Eline and its unusual penetration and impacts over the southern African mainland, Weather Forecast., 19, 789–805, 2004.
Richard, Y., Fauchereau, N., Poccard, I., Rouault, M., and Trzaska, S.: 20th century droughts in Southern Africa: Spatial and temporal variability, teleconnections with oceanic and atmospheric conditions, Int. J. Climatol., 21, 873–885, https://doi.org/10.1002/joc.656, 2001.
Rozante, J. R., Moreira, D. S., Godoy, R. C. M., and Fernandes, A. A.: Multi-model ensemble: technique and validation, Geosci. Model Dev., 7, 2333–2343, https://doi.org/10.5194/gmd-7-2333-2014, 2014.
SADC: Maputo Declaration on the Commitment by SADC to enhance Early Warning and Early Action in the Region, https://au.int/sites/default/files/pressreleases/42156-other-Maputo_Declaration_Final_AUC_11_Sept-2022.pdf (last access d: 12 December 2024), 2022.
Saji, N. H., Goswami, B. N., Vinayachandran, P. N., and Yamagata, T.: A dipole mode in the tropical Indian Ocean, Nature, 401, 360–363, https://doi.org/10.1038/43854, 1999.
Silva, J. A. and Matyas, C. J.: Relating Rainfall Patterns to Agricultural Income: Implications for Rural Development in Mozambique, Weather. Clim. Soc., 6, 218–237, https://doi.org/10.1175/WCAS-D-13-00012.1, 2014.
Stagge, J. H., Tallaksen, L. M., Gudmundsson, L., Van Loon, A. F., and Stahl, K.: Candidate Distributions for Climatological Drought Indices (SPI and SPEI), Int. J. Climatol., 35, 4027–4040, https://doi.org/10.1002/joc.4267, 2015.
Toté, C., Patricio, D., Boogaard, H., Van der Wijngaart, R., Tarnavsky, E., and Funk, C.: Evaluation of Satellite Rainfall Estimates for Drought and Flood Monitoring in Mozambique, Remote Sens., 7, 1758–1776, https://doi.org/10.3390/RS70201758, 2015.
Trambauer, P., Werner, M., Winsemius, H. C., Maskey, S., Dutra, E., and Uhlenbrook, S.: Hydrological drought forecasting and skill assessment for the Limpopo River basin, southern Africa, Hydrol. Earth Syst. Sci., 19, 1695–1711, https://doi.org/10.5194/hess-19-1695-2015, 2015.
Weingärtner, L., Pforr, T., and Wilkinson, E.: The evidence base on Anticipatory Action, https://www.wfp.org/publications/evidence-base-anticipatory-action (last access: 12 December 2024), 2020.
WFP: Regional Bureau for Southern Africa SPECIAL OPERATION 200993, https://documents.wfp.org/stellent/groups/internal/documents/projects/wfp285532.pdf 2016.
WFP: MOZAMBIQUE: A Climate Analysis, https://doi.org/10.54302/mausam.v71i4.50, 2018.
WFP: Building systems to anticipate drought in Mozambique, https://reliefweb.int/report/mozambique/anticipatory-action-building-systems-anticipate-drought (last accessed: 12 December 2024), 2023.
Winsemius, H. C., Dutra, E., Engelbrecht, F. A., Archer Van Garderen, E., Wetterhall, F., Pappenberger, F., and Werner, M. G. F.: The potential value of seasonal forecasts in a changing climate in southern Africa, Hydrol. Earth Syst. Sci., 18, 1525–1538, https://doi.org/10.5194/hess-18-1525-2014, 2014.
WMO: Early Warnings For All: The UN Global Early Warning Initiative for the Implementation of Climate Adaptation, https://wmo.int/activities/early-warnings-all/wmo-and-early-warnings-all-initiative (last access: 12 December 2024), 2022.
World Bank: Mozambique food market monitoring and resilient agriculture planning, Policy Report 645, Washington, D.C., 2018.
World Meteorological Organization: Standardized Precipitation Index User Guide (M. Svoboda, M. Hayes and D. Wood), WMO-No. 1090, Geneva, https://www.droughtmanagement.info/literature/WMO_standardized_precipitation_index_user_guide_en_2012.pdf (last access: 18 December 2024), 2012.
Yoshikane, T. and Yoshimura, K.: A downscaling and bias correction method for climate model ensemble simulations of local-scale hourly precipitation, Sci. Rep., 13, 9412, https://doi.org/10.1038/s41598-023-36489-3, 2023.
Zarei, M., Najarchi, M., and Mastouri, R.: Bias correction of global ensemble precipitation forecasts by Random Forest method, Earth Sci. Inform., 14, 677–689, https://doi.org/10.1007/s12145-021-00577-7, 2021.
Ready, Set & Go!system, developed by the World Food Programme and partners, employs seasonal forecasts to tackle droughts in Mozambique. With the Maputo Declaration, efforts to expand early warning systems are aligning with global initiatives for universal protection by 2027. Through advanced forecasting and anticipatory action, it could cover 76 % of districts against severe droughts, reaching 87 % national coverage for the first months of the rainy season.
Ready, Set & Go!system, developed by the World Food Programme and partners, employs...