the Creative Commons Attribution 4.0 License.
the Creative Commons Attribution 4.0 License.
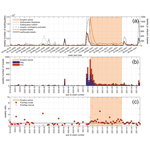
Brief communication: Small-scale geohazards cause significant and highly variable impacts on emotions
Evgenia Ilyinskaya
Vésteinn Snæbjarnarson
Hanne Krage Carlsen
Björn Oddsson
The impact of geohazards on the mental health of local populations is well recognised but understudied. We used natural language processing (NLP) of Twitter (now known as X) posts (n=10 341) to analyse the sentiments expressed in relation to a pre-eruptive seismic unrest and a subsequent volcanic eruption in Iceland in 2019–2021. We show that although these geohazards were of a small size and caused negligible material damage, they were associated with a measurable change in expressed emotions in the local population. The seismic unrest was associated with predominantly negative sentiments (positive-to-negative sentiment ratio of 1:1.3), but the eruption was associated with predominantly positive sentiments (positive-to-negative sentiment ratio of 1.4:1). We demonstrate a cost-effective tool for gauging public discourse that could be used in risk management.
- Article
(3027 KB) - Full-text XML
- BibTeX
- EndNote
Social media posts have been successfully used for assessing geophysical aspects of volcanic and seismic events, for example, locating earthquakes using crowd-sourced information (Earle, 2010; Saraò et al., 2023; Steed et al., 2019; Wang et al., 2023) and science communication between researchers and between researchers and citizens (Hicks, 2019; Lacassin et al., 2020; Watson et al., 2023). They have also been utilised for crisis and risk communication, rapid assessment of material damage, and aiding recovery efforts after several disasters, including the great Tohoku earthquake and tsunami, hurricanes (e.g. Harvey, Sandy, Eta, and Iota), and major floods (e.g. Bryan-Smith et al., 2023; Chatfield and Brajawidagda, 2012; Earle, 2010; Guan and Chen, 2014; Moghadas et al., 2023; Riddell and Fenner, 2021; Wang and Ye, 2018). Several recent studies have used social media posts for identifying challenges and inequalities in response, recovery, and aid delivery following geodisasters (Kam et al., 2021; Nielsen et al., 2024; Olynk Widmar et al., 2022). The non-material impact of geohazards, including on the mental health of local populations, is well recognised (Vo and Collier, 2013; Hlodversdottir et al., 2018; Becker et al., 2019; Gissurardóttir et al., 2019) but understudied. Studies are mostly done through interviews or clinical assessments, where the results typically become available long after the event. Social media language and expressed sentiments can be used as indicators for public discourse and have been shown to be predictive of individuals' mental health state and its deterioration (Cha et al., 2022; Eichstaedt et al., 2018; Kelley and Gillan, 2022; Lan et al., 2024; Malko et al., 2023; Oltmanns et al., 2021; Recharla et al., 2024). Using artificial intelligence, such as natural language processing (NLP), it is possible to quickly process very large volumes of data for content and sentiment analysis (Bryan-Smith et al., 2023; Cha et al., 2022; Eichstaedt et al., 2018; He et al., 2022; Kam et al., 2021; Lan et al., 2024; Oltmanns et al., 2021; Park et al., 2015; Venkit et al., 2023).
Here we use NLP on a dataset collected on the social media platform Twitter (now known as X) to analyse the public views and expressed sentiments related to two types of common global geohazards: a period of moderate seismic unrest and a small basaltic fissure eruption, using Iceland as the case study. The impacts of relatively small events are not well covered in the literature, although they are much more common than large ones. Small eruptions (volcanic explosivity index ≤ 2) account for ∼80 % of eruptions worldwide (Siebert et al., 2015). Pre-eruptive unrest is currently not considered in volcanic hazard and risk assessments beyond material damage to structures and has not been researched. There is a pressing need to understand the impacts of events of all sizes on local populations, given that ∼500 million people are living within a 50 km distance from a volcano and rising numbers within 10 km (Freire et al., 2019).
The Reykjanes Peninsula in Iceland provided a highly suitable natural laboratory. Between 2019 and 2021 this densely populated area (∼260 000 people within a 40 km radius) experienced two distinct and prolonged periods of geohazards: 15 months of elevated seismicity (Sigmundsson et al., 2022) (from here on termed “seismic unrest period”) that subsided abruptly and was followed by a basaltic fissure eruption that lasted 6 months (Halldórsson et al., 2022) (termed “eruption period”). The seismic unrest period took place between December 2019 and March 2021 and included several intense earthquake swarms, with the largest event of magnitude 5.6 (Sigmundsson et al., 2022). The eruption took place between 19 March and 19 September 2021 at Mt Fagradalsfjall and effused relatively small lava flows within uninhabited valleys. The material damage caused by the seismicity and the eruption was negligible, and no physical harm was reported. We were able to focus our study on local residents rather than tourists by analysing social media posts written in Icelandic, as the language is spoken predominantly by people living in Iceland. In addition, due to covid-19 restrictions during most of our study period, the number of people travelling internationally was at a record low in modern times.
Twitter was estimated to be used by 24 % of Iceland's population at the time of study (Gallup, 2021). The main potential limitation of our approach is that views expressed on Twitter may not fully represent the views of people who chose to use different social media platforms or none at all; this can be explored in future research by including more than one social media platform. This would demonstrate the applicability of the method to other countries, where the popularity of different social media platforms may differ.
Natural language processing
We performed sentiment analysis on tweets posted between 9 December 2019 and 31 December 2021 (n=10 341) containing a fixed set of earthquake- and eruption-related keywords in Icelandic. Appendix A contains further details about the methods, including the full list of keywords used. A subset of 636 tweets was manually labelled as having a “negative sentiment”, “positive sentiment”, or “neutral statement” (see Table A2 for examples of labelled tweets). The rest of the dataset was labelled automatically as the same three categories by a pre-trained language model that was fine-tuned for classification using the manually labelled data. The model, bilingual in English and Icelandic (Snæbjarnarson and Einarsson, 2022), was first adapted for sentiment analysis using the English Stanford Sentiment Treebank (SST) dataset (Socher et al., 2013), as no explicit Icelandic sentiment analysis dataset exists, and this was the first time NLP sentiment analysis in Icelandic was attempted. The approach of first adapting the model using English-only data has been shown to enable cross-lingual transfer (Conneau et al., 2020; Pires et al., 2019). Finally, we trained on the Icelandic-only sentiment data. We acknowledge other ways of mixing data but emphasise that our goal in this work was not to exhaustively compare these methods but simply evaluate one such methodology for the events we cover. This is a standard approach in NLP where data are used for transferring learning between a high-resource language and a low-resource language (Pfeiffer et al., 2020; Snæbjarnarson et al., 2023). Initial results showed that the model labelled earthquakes negatively and eruptions positively in sentences that should have been labelled as neutral. To mitigate this, we masked out all of the earthquake- and eruption-related keywords, both during model training and full dataset analysis. We hypothesise that this makes the model more robust to out-of-distribution settings, even though performance drops slightly on the evaluation split. Using a subset of tweets we manually verified that the model labelled sentiments correctly as neutral when the keywords were masked out. The drop in model performance when masking was introduced was minor: there was a drop in accuracy from 71 % to 69 %, and F1 (the harmonic mean of the precision and recall) also dropped from 0.71 to 0.69. Full evaluation results are given in Appendix A. The model performance reached the “benchmark” for good performance in Twitter sentiment analysis proposed by Zimbra et al. (2018). This demonstrates the potential of our method for broader use, as sentiment analysis can be successfully achieved by NLP models bilingual in English and a local language without the need for a sentiment dataset in the local language.
The two geohazards in our case study evoked a measurable emotional response as indicated by the content of Twitter posts. Figure 1 shows a time series spanning our study period and demonstrates changes in the number of tweets with earthquake-related and eruption-related keywords (from here on termed “earthquake tweets” and “eruption tweets”, respectively) and their sentiment labelling. Figure 1a shows the time-dependent changes in the number of tweets and the number of earthquakes in a calendar week, Fig. 1b shows the changes in the number of tweets labelled as negative and positive in a week, and Fig. 1c shows the changes in the positive-to-negative sentiment ratio in a week. The majority of the tweets containing earthquake- and/or eruption-related keywords (62 %) were evaluated as containing a sentiment (30 % negative and 32 % positive). The remaining 38 % were neutral statements. Examples of tweets labelled neutral, positive, and negative are in Appendix A (Table A2). Previous work has shown that very large and/or destructive earthquakes, such as the great Tohoku 2011 (Vo and Collier, 2013), Canterbury 2010 (Becker et al., 2019), and Ridgecrest 2019 (Ruan et al., 2022) earthquakes, cause distress in the affected populations (measured using social media analysis by Vo and Collier, 2013, and Ruan et al., 2022, and “traditional” interview methods by Becker et al., 2019). We show here that earthquakes which are orders of magnitude smaller and cause no physical harm and negligible material damage can still evoke a significant emotional response (Fig. 1b and c). We also show that the level of public interest is dependent on both the intensity of the seismicity (measured here as the number of earthquakes per week) and the proximity of the seismicity to densely populated areas. Figure 1a and b qualitatively show that the number of tweets increases in weeks with a high number of earthquakes, in particular when the earthquakes are located on the Reykjanes Peninsula. To analyse this relationship quantitatively, we plotted the number of earthquake tweets as a function of the number of earthquakes, shown in Fig. 2. For the densely populated Reykjanes Peninsula, there is a correlation of r2=0.66 (Fig. 2a and b). The correlation disappeared when we consider the number of earthquakes in the rest of Iceland, which is relatively sparsely populated (Fig. 2c and d).
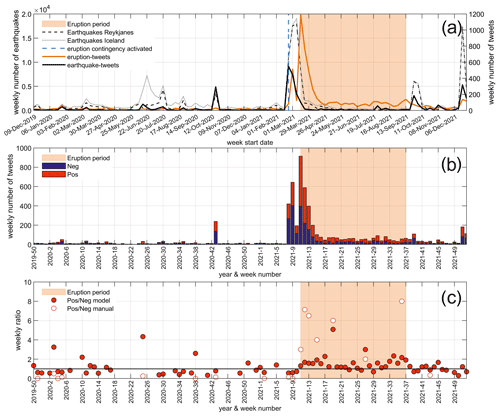
Figure 1Time series plot of Twitter (now known as X) data (n of tweets = 10 341) during the pre-eruptive seismic unrest period (December 2019–March 2021) and the eruption period (March–September 2021, highlighted in orange). The tweets were selected by a set of earthquake- and eruption-related keywords in Icelandic listed in Appendix A. (a) The weekly number of earthquake- and eruption-related tweets together with the weekly number of earthquakes. The number of weekly earthquakes is shown separately for the Reykjanes Peninsula and Iceland as a whole. The magnitude of earthquakes ranged from Mw 0 to 5.6. (b) The weekly number of tweets evaluated as expressing positive (“pos”) or negative (“neg”) sentiments by the NLP model. (c) The average positive : negative (“pos : neg”) weekly ratio, as evaluated by the NLP model in the whole dataset and manually in the data subset. The data shown in (c) include only weeks where the total number of tweets was >10 to avoid bias introduced by very low numbers.
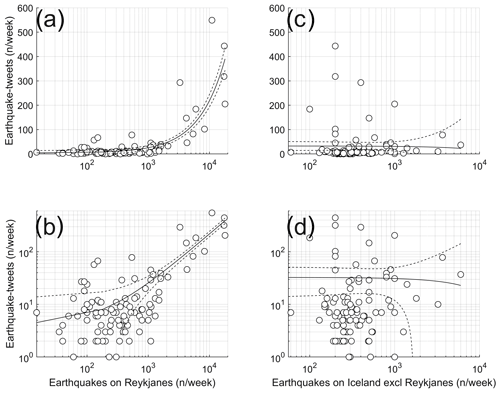
Figure 2Scatterplot showing the number of earthquake-related tweets (Twitter is now known as X) as a function of the number of earthquakes on (a) the Reykjanes Peninsula (earthquake magnitude range Mw 0–5.6, r2=0.69) and (b) the rest of Iceland, excluding the Reykjanes Peninsula (earthquake magnitude range Mw 0–4.8, no statistically significant relationship). The solid line is a linear regression model fit, and the dotted lines are the 5 % significance level.
The seismic unrest period was dominated by negative sentiments with an average weekly positive : negative ratio of 1:1.3. This finding agrees well with contemporary reports in the local media, which described primarily negative experiences, including anxiety (RÚV, 2021b) and disturbed sleep (RÚV, 2021a) caused by the earthquakes. The overall negative sentiments were likely caused by a combination of the physical discomfort of the ground shaking and by the uncertainty about further development. It was already known that the seismicity was being caused by a magma intrusion, but there was uncertainty about whether, when, and especially where an eruption would happen; the areas considered to be at risk to lava flows included a town and major infrastructure (power plants and tourism businesses). The seismic unrest was monitored closely, and scientific interpretations were published continuously in the media. The constant reports would have been impossible to ignore, and conflicting interpretations may have contributed to anxiety and other negative emotions.
There was a statistically significant change () to predominantly positive sentiments associated with the start of the eruption (Fig. 1b and c). The average weekly positive : negative ratio during the eruption was 1.4:1 compared to 1:1.3 prior. It is possible that the increase in positivity was even larger because the NLP evaluation consistently reported a smaller positive : negative ratio during the eruption period compared to the manual analysis (Fig. 1c). To the best of our knowledge, this is the first time that an increase in positive attitudes associated with the start of an eruption has been recorded among local populations. We propose that this positive attitude is best explained by a combination of geophysical and societal factors. Many of the positive sentiments expressed relief that an eruption would bring an end to the near-constant earthquakes and to the associated uncertainty (examples of tweets: “I want it to erupt so that the earthquakes stop”; “There is something absurd about being able to sleep soundly through the night now that there is an eruption in one's backyard”). This agrees with previous studies on highly destructive events where positive statements were found to be associated with relief that the event is over (e.g. the great Tohoku 2011 earthquake; Vo and Collier, 2013) or messages of hope or prayers for rescue and recovery (e.g. Hurricane Harvey; Zou et al., 2019). We also found that the Fagradalsfjall eruption directly evoked positive emotions in the local population, including joy and pleasure. Our findings suggest that the close proximity of the Fagradalsfjall eruption site to populated areas may have been an important factor that enhanced the positive attitudes, as it allowed more people to experience it firsthand (example of a tweet: “The eruption is so fantastic, even on my second visit … I adore seeing all kinds of people there enjoying the nature and being outdoors”). The site was open to the general public and was within an hour's drive from the capital city, followed by a 1 h hike. The public started arriving in large numbers within hours of the eruption, and the total number of visits via the hiking trails equated to 310 000 (volcanic eruption in Geldingadalir, 2021). It is possible that the social and physical isolation caused by the covid-19 pandemic further enhanced the positive experience, as a visit to the eruption site provided both a distraction and an opportunity to socialise and exercise in a relatively covid-safe outdoor setting. Furthermore, the eruption had an unprecedented level of live coverage through multiple high-quality webcams and social media feeds (Wadsworth et al., 2022), allowing people who could not travel in person to participate in and enjoy the phenomenon, thereby potentially kick-starting a new chapter of “remote” volcano tourism (e.g. “It is midday and I have done nothing except watching the eruption [via live streams]. So beautiful!”). Previous studies focusing on tourists in volcanic areas (e.g. Benediktsson et al., 2011; Davis et al., 2013; Donovan, 2018) have reported overwhelmingly positive attitudes, but given their very limited number and type of participants, it is unknown how the findings relate to the general public in local communities. Our results show that eruptions that do not directly endanger lives and infrastructure may cause a measurable increase in positive attitudes on a population-wide level and furthermore suggest that, given the opportunity, people of different ages and physical abilities are keen to experience volcanic activity up close.
Our findings are important for risk assessment and management because we show that even small-sized geohazards without significant material damage can cause a measurable change in expressed sentiments in local populations, which, in turn, may indicate an impact on people's mental health. Pre-eruptive unrest is currently a somewhat “forgotten” volcanic hazard, and its public health impacts have not been researched. Furthermore, it is not included in educational and scientific resources such as the Encyclopedia of Volcanoes (Rymer, 2015) and the VolFilm series (Global Volcanism Program|Video Collections|VOLFilms Collection, 2024). We show that pre-eruptive events (here, seismic unrest) can potentially be more detrimental to mental wellbeing than the actual eruption and should be considered in studies of impacts. In general, the impacts of living with uncertainty due to expected but not yet materialised geohazards need further attention from research and disaster risk reduction (DRR) communities.
Incorporating sentiment analysis of crowd-sourced information, such as social media posts, into local risk management has the potential for immediate and longer-term benefits. While our method does not provide direct measures of mental health states and impacts and is not intended to replace more formal investigations, it can be used to quickly gauge whether communities are under stress and may require additional surveying and/or resources. Alternatively, knowing that the public views an eruption (or other natural phenomena) as a generally enjoyable and attractive event may assist risk managers in choosing the most effective approach to achieve compliance should they need to restrict access to the site.
Our study provides a valuable contribution to longitudinal studies of mental health impacts in the local population. The volcanic–seismic events on Reykjanes were still ongoing at the time of writing (June 2024) and are considered likely to continue for years or potentially decades. Long-duration, dynamically evolving volcanic events are common worldwide, for example at the Kīlauea volcano in Hawaii. The method we demonstrate allows for the capture and analysis of sentiments experienced and expressed in situ, which cannot be reliably reproduced by retrospective investigations. The contemporary meaning of people's lived experiences is essential but ephemeral, and the ability to recall fades and changes over time. With the literal loss of familiar landscapes, and homes, people also lose connection to their memories (Árnason and Hafsteinsson, 2023). Further studies could focus on designing methodologies for quantifying emotional impacts, mental health outcomes, and risk perception changes using social media data in the Reykjanes case study and elsewhere.
Finally, in our quest to reduce the risk posed by geohazards – in this case, a small eruption – we should not dismiss the potential mental health benefits of allowing people to experience them where possible, even though the benefits will be difficult to quantify and weigh up against the (often more obvious) risks.
A1 Theory and previous work on tweet sentiment analysis
Neural language models using Transformer architecture (Vaswani et al., 2017) have had a great impact on the field of natural language processing (NLP). These models are trained using representation learning to embed words (create vector representations for words and their parts) for use in tasks such as machine translation, answering questions, and sentiment analysis. Such models are first trained in an unsupervised manner on raw text to maximise embedding quality across all available contexts. The models, e.g. BERT (Devlin et al., 2019) and T5 (Raffel et al., 2020) – https://jmlr.org/papers/v21/20-074.html (last access: 16 September 2024), can then be adapted using fine-tuning on much smaller labelled datasets for a variety of classification tasks that depend on the context of the text in natural language.
Sentiment analysis is a common area of research in NLP with connections to hate speech analysis and stance detection (Maas et al., 2019; Mohammad et al., 2016; Socher et al., 2013). Here we use it in a traditional positive, negative, and neutral classification setting but with regards to the geohazards surrounding the volcanic eruption in Fagradalsfjall in 2021.
A2 Extended methods
Having gained academic access to historical tweets, we use the Python package TwitterAPI (source code available at https://github.com/geduldig/TwitterAPI, last access: 16 September 2024) to download tweets posted between 9 December 2019 and 31 December 2021. The tweets are filtered for a fixed set of earthquake- and eruption-related keywords in Icelandic (details on keywords below). Duplicates, including retweets, were removed from the data. In total 10 341 individual tweets matched our criteria and were used for further analysis. We set the keywords to include all grammatically correct variations of “eruption” and “earthquake” in Icelandic, including plural words. Spelling errors were not dealt with; this could be addressed with Levenshtein distance but would also increases the risk of false positives. There are common words in Icelandic that differ only slightly in spelling from gos (e.g. gas), and these would have increased the number of false positives. Another approach could have been to automatically correct the tweets, but developed methods target editorial text rather than social media posts (Ingólfsdóttir et al., 2023). We chose this approach because the main keywords, gos and skjálfti (eruption and earthquake), and their grammatical variations are short and easy-to-spell words in Icelandic.
For keywords, tweets were filtered with the morphological variations of eruption and earthquake in Icelandic, along with the emoji for volcano. The full list used is shown below:
Eruption-related keywords include , gos, gosið, gosinu, gossins, eldgos, eldgosið, eldgosi, eldgoss, gjósa, and gaus.
Earthquake-related keywords include skjálfti, skjálfta, skjálftar, skjálftum, skjálftarnir, skjálftana, skjálftanum, skjálftann, skjálftan, skjálftans, and skjálftinn.
A subset of this dataset was manually annotated by sentiment into negative, positive, or neutral. A total of 224 tweets were labelled as negative, 241 as positive, and 171 as neutral. Tweets were selected for manual annotation semi-randomly; outside of the eruption period, we randomly selected a subset of tweets in periods with either highly elevated seismicity or relatively low seismicity levels. During the 6-month-long eruption period, we randomly selected a subset of tweets from each of the first 3 weeks of the eruption when eruption-related tweet frequency was at the highest level. We then randomly selected tweets in eruption weeks 5, 9, 17, and 26 to cover different time periods of the eruption.
To label the other tweets automatically, an already-adapted language model was fine-tuned on the manually annotated data for use in labelling as follows: the data were randomly split into a train and validation set of 493 and 121 tweets, respectively. A pre-trained Icelandic–English language model (Snæbjarnarson and Einarsson, 2022) was first adapted for binary sentiment analysis using the English Stanford Sentiment Treebank (SST) dataset (Socher et al., 2013). The original bilingual model was trained using Fairseq (Ott et al., 2019), from Facebook AI research, but was then ported to the Transformers (Wolf et al., 2020) library and adapted for sentiment analysis. While no explicit Icelandic sentiment analysis dataset exists, the model seems to behave well in general sentiment analysis for Icelandic due to its bilingual pre-training. This behaviour has been well documented before and is referred to as transfer learning (Ruder et al., 2019). The model was then further fine-tuned on the training data for five full iterations (epochs) using a learning rate of and a batch size of eight. As the initial SST fine-tuned model was only trained to classify positive or negative sentiment, the output layer of the neural network was modified to provide three classes with the new classification node initialised by averaging over the prior two.
Initial results showed that the model found that earthquakes were negative and eruptions positive in sentences that should have been labelled as neutral. To mitigate this commonly seen shortcut to labelling (Geirhos et al., 2020), we masked out all of the earthquake- and eruption-related keywords, both during model training and actual evaluation. Using a subset of tweets, we manually verified that the model labelled sentiments correctly as neutral when the keywords were masked out. The drop in performance when masking was minor: there was a drop in accuracy from 71 % to 69 %, and F1 (the harmonic mean of the precision and recall) also dropped from 0.71 to 0.69. Evaluation results are shown in Table A1.
It is worth noting that the representations one can extract from pre-trained foundation models are limited to the relations that can be inferred from their training data. If these do not sufficiently cover the style or terminology one is interested in, then the representations will not be robust. This can be particularly pronounced in the case of idiomatic expressions for low-resource languages when using multilingual models. As we targeted Icelandic, we used a pre-trained model trained on only Icelandic and English to mitigate these issues. If the method is to be followed for other languages, a limiting factor is that the mentioned resources need to be present in some form to cover a given language, i.e. pre-training data or pre-trained models, along with sufficient labelled sentiment data. In these cases, recent advances in general-purpose large-language models (see e.g. OpenAI et al., 2024) may be of value in both direct evaluation and pseudo-labelling training data in low-resource settings. Furthermore, fine-tuning these models using the labelled data can be seen as a way to improve these representations and the resulting decision boundaries. Finally, as improved models and datasets become available, we can expect higher accuracy and lower uncertainty when these are applied to the data we have collected.
Examples of tweets labelled as neutral, positive, and negative are shown in Table A2. Table A2 also shows whether labelling the expressed sentiment was straightforward or ambiguous. The use of humour and sarcasm was a common reason for ambiguous sentiment labelling.
The datasets and code are available in an open-access repository https://github.com/vesteinn/fagradalsfjall_eruption_sentiment (last access: 5 September 2024) for reproducibility and further use by the community.
The key NLP contributions are a new dataset for Icelandic sentiment analysis of tweets about geological events, a fine-tuned Icelandic sentiment model, and an evaluation of masking to prevent shortcut learning in sentiment analysis.
Table A2Examples of tweets (Twitter is now known as X) from the dataset with keywords related to earthquakes (E) and the volcanic eruption (V). The “Label” column shows how the tweet was labelled in the dataset. The “Label certainty” column shows whether labelling the expressed sentiment was straightforward (“clear”) or ambiguous. Where the labelling was ambiguous an explanation is included. The table includes the original tweets in Icelandic and our translation to English. The selected tweets are unchanged from the original posts with one exception. Some tweets included links to the original posts; we removed these to preserve the posters' anonymity.
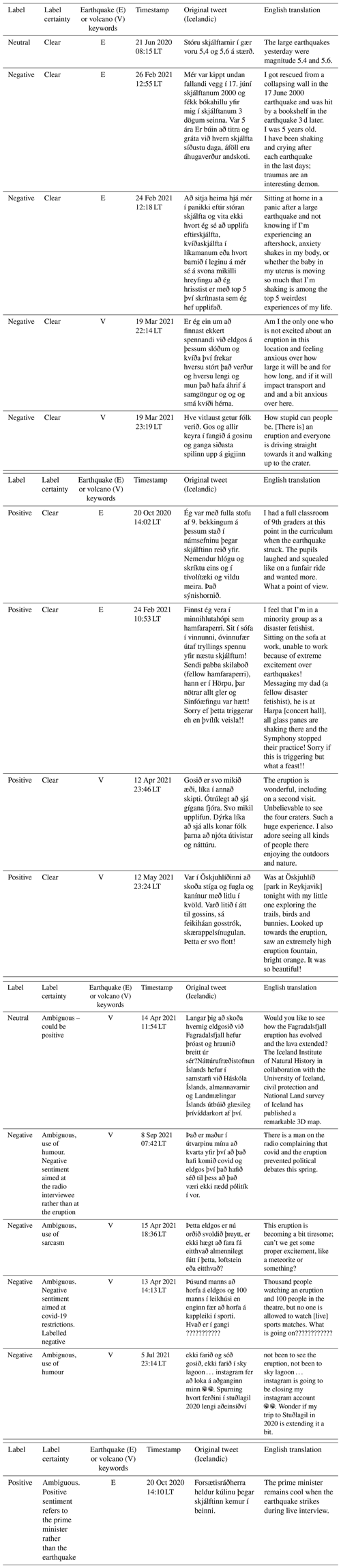
Historical tweets can be obtained from TwitterAPI through academic research access. The code used here for downloading the tweets is a Python package TwitterAPI (source code available at https://github.com/geduldig/TwitterAPI, geduldig, 2024). The datasets and code generated in this study are available in an open-access repository (https://doi.org/10.5281/zenodo.13733750, Snæbjarnarson, 2024).
EI and HKC conceived the study idea. VS obtained and processed the Twitter data and led the NLP analysis with input from EI. EI led the manual Twitter data analysis. EI led the overall writing of the paper with input from all authors.
The contact author has declared that none of the authors has any competing interests.
Publisher's note: Copernicus Publications remains neutral with regard to jurisdictional claims made in the text, published maps, institutional affiliations, or any other geographical representation in this paper. While Copernicus Publications makes every effort to include appropriate place names, the final responsibility lies with the authors.
Twitter data were made available by academic API access of Twitter.
This research has been supported by COMET, the UK Natural Environment Research Council's (NERC) Centre for the Observation and Modelling of Earthquakes, Volcanoes and Tectonics, a partnership between UK universities and the British Geological Survey. Vésteinn Snæbjarnarson was supported by the Pioneer Centre for Artificial Intelligence, DNRF grant number P1.
This paper was edited by Bruce D. Malamud and reviewed by two anonymous referees.
Árnason, A. and Hafsteinsson, S. B.: Technology and Presence in a Museum of Trauma: Therapeutic Effects of Making Danger Real, in: Museums and Technologies of Presence, Routledge, ISBN 9781003334316, 2023.
Becker, J. S., Potter, S. H., McBride, S. K., Wein, A., Doyle, E. E. H., and Paton, D.: When the earth doesn’t stop shaking: How experiences over time influenced information needs, communication, and interpretation of aftershock information during the Canterbury Earthquake Sequence, New Zealand, Int. J. Disaster Risk Reduct., 34, 397–411, https://doi.org/10.1016/j.ijdrr.2018.12.009, 2019.
Benediktsson, K., Lund, K. A., and Huijbens, E.: Inspired by Eruptions? Eyjafjallajökull and Icelandic Tourism, Mobilities, 6, 77–84, https://doi.org/10.1080/17450101.2011.532654, 2011.
Bryan-Smith, L., Godsall, J., George, F., Egode, K., Dethlefs, N., and Parsons, D.: Real-time social media sentiment analysis for rapid impact assessment of floods, Comput. Geosci., 178, 105405, https://doi.org/10.1016/j.cageo.2023.105405, 2023.
Cha, J., Kim, S., and Park, E.: A lexicon-based approach to examine depression detection in social media: the case of Twitter and university community, Humanit. Soc. Sci. Commun., 9, 1–10, https://doi.org/10.1057/s41599-022-01313-2, 2022.
Chatfield, A. and Brajawidagda, U.: Twitter tsunami early warning network: a social network analysis of Twitter information flows, Faculty of Engineering and Information Sciences – Papers: Part A, https://ro.uow.edu.au/eispapers/136/ (last access: 5 September 2024), 2012.
Conneau, A., Khandelwal, K., Goyal, N., Chaudhary, V., Wenzek, G., Guzmán, F., Grave, E., Ott, M., Zettlemoyer, L., and Stoyanov, V.: Unsupervised Cross-lingual Representation Learning at Scale, in: Proceedings of the 58th Annual Meeting of the Association for Computational Linguistics, ACL 2020, Online, 8440–8451, https://doi.org/10.18653/v1/2020.acl-main.747, 2020.
Davis, S., A., A., Aoki, K., Cook, M., Duley, S., Huff, M., and Logan, C.: Managing risk and allure at volcanoes: In Hawaii: How close is too close?, Geoj. Tourism Geosite., 12, 85–93, 2013.
Devlin, J., Chang, M.-W., Lee, K., and Toutanova, K. N.: BERT: Pre-training of Deep Bidirectional Transformers for Language Understanding, in: Proceedings of the 2019 Conference of the North American Chapter of the Association for Computational Linguistics: Human Languages Technologies, June 2–7 June 2019, Minneapolis, Minnesota, https://www.waqasrana.me/assets/papers/N19-1423.pdf (last access: 16 September 2024), 2019.
Donovan, A.: Sublime encounters: Commodifying the experience of the geos, Geo: Geogr. Environ., 5, e00067, https://doi.org/10.1002/geo2.67, 2018.
Earle, P.: Earthquake Twitter, Nat. Geosci., 3, 221–222, https://doi.org/10.1038/ngeo832, 2010.
Eichstaedt, J. C., Smith, R. J., Merchant, R. M., Ungar, L. H., Crutchley, P., Preoţiuc-Pietro, D., Asch, D. A., and Schwartz, H. A.: Facebook language predicts depression in medical records, P. Natl. Acad. Sci. USA 115, 11203–11208, https://doi.org/10.1073/pnas.1802331115, 2018.
Freire, S., Florczyk, A. J., Pesaresi, M., and Sliuzas, R.: An Improved Global Analysis of Population Distribution in Proximity to Active Volcanoes, 1975–2015, ISPRS Int. J. Geo-Inform., 8, 341, https://doi.org/10.3390/ijgi8080341, 2019.
Gallup: Samfélagsmiðlamæling Gallup, Iceland, https://www.gallup.is/frettir/ny-samfelagsmidlamaeling-gallup/ (last access: 3 September 2024), 2021.
geduldig: geduldig/TwitterAPI, GitHub [code], https://github.com/geduldig/TwitterAPI (last access: 16 September 2024), 2024.
Geirhos, R., Jacobsen, J.-H., Michaelis, C., Zemel, R., Brendel, W., Bethge, M., and Wichmann, F. A.: Shortcut learning in deep neural networks, Nat. Mach. Intel., 2, 665–673, https://doi.org/10.1038/s42256-020-00257-z, 2020.
Gissurardóttir, Ó. S., Hlodversdóttir, H., Thordardóttir, E. B., Pétursdóttir, G., and Hauksdóttir, A.: Mental health effects following the eruption in Eyjafjallajökull volcano in Iceland: A population-based study, Scand. J. Public Health, 47, 251–259, https://doi.org/10.1177/1403494817751327, 2019.
Global Volcanism Program|Video Collections|VOLFilms Collection: VOLFilms, https://volcano.si.edu/gallery/VideoCollection.cfm?subject=VOLFilms&language=English (last access: 24 June 2024), 2024.
Guan, X. and Chen, C.: Using social media data to understand and assess disasters, Nat. Hazards, 74, 837–850, https://doi.org/10.1007/s11069-014-1217-1, 2014.
Halldórsson, S. A., Marshall, E. W., Caracciolo, A., Matthews, S., Bali, E., Rasmussen, M. B., Ranta, E., Robin, J. G., Guðfinnsson, G. H., Sigmarsson, O., Maclennan, J., Jackson, M. G., Whitehouse, M. J., Jeon, H., van der Meer, Q. H. A., Mibei, G. K., Kalliokoski, M. H., Repczynska, M. M., Rúnarsdóttir, R. H., Sigurðsson, G., Pfeffer, M. A., Scott, S. W., Kjartansdóttir, R., Kleine, B. I., Oppenheimer, C., Aiuppa, A., Ilyinskaya, E., Bitetto, M., Giudice, G., and Stefánsson, A.: Rapid shifting of a deep magmatic source at Fagradalsfjall volcano, Iceland, Nature, 609, 529–534, https://doi.org/10.1038/s41586-022-04981-x, 2022.
He, P., Gao, J., and Chen, W.: DeBERTaV3: Improving DeBERTa using ELECTRA-Style Pre-Training with Gradient-Disentangled Embedding Sharing, The Eleventh International Conference on Learning Representations, Kigali Rwanda, 1–5 May 2023, https://arxiv.org/pdf/2111.09543 (last access: 16 September 2024), 2022.
Hicks, S. P.: Geoscience analysis on Twitter, Nat. Geosci., 12, 585–586, https://doi.org/10.1038/s41561-019-0425-4, 2019.
Hlodversdottir, H., Thorsteinsdottir, H., Thordardottir, E. B., Njardvik, U., Petursdottir, G., and Hauksdottir, A.: Long-term health of children following the Eyjafjallajökull volcanic eruption: a prospective cohort study, Eur. J. Psychotraumatology, 9, 1442601, https://doi.org/10.1080/20008198.2018.1442601, 2018.
Ingólfsdóttir, S. L., Ragnarsson, P., Jónsson, H., Simonarson, H., Thorsteinsson, V., and Snæbjarnarson, V.: Byte-Level Grammatical Error Correction Using Synthetic and Curated Corpora, in: Proceedings of the 61st Annual Meeting of the Association for Computational Linguistics (Volume 1: Long Papers), ACL 2023, Toronto, Canada, 7299–7316, https://doi.org/10.18653/v1/2023.acl-long.402, 2023.
Kam, J., Park, J., Shao, W., Song, J., Kim, J., Gizzi, F. T., Porrini, D., and Suh, Y.-J.: Data-driven modeling reveals the Western dominance of global public interest in earthquakes, Humanit. Soc. Sci. Commun., 8, 1–9, https://doi.org/10.1057/s41599-021-00914-7, 2021.
Kelley, S. W. and Gillan, C. M.: Using language in social media posts to study the network dynamics of depression longitudinally, Nat. Commun., 13, 870, https://doi.org/10.1038/s41467-022-28513-3, 2022.
Lacassin, R., Devès, M., Hicks, S. P., Ampuero, J.-P., Bossu, R., Bruhat, L., Daryono, Wibisono, D. F., Fallou, L., Fielding, E. J., Gabriel, A.-A., Gurney, J., Krippner, J., Lomax, A., Sudibyo, M. M., Pamumpuni, A., Patton, J. R., Robinson, H., Tingay, M., and Valkaniotis, S.: Rapid collaborative knowledge building via Twitter after significant geohazard events, Geosci. Commun., 3, 129–146, https://doi.org/10.5194/gc-3-129-2020, 2020.
Lan, X., Cheng, Y., Sheng, L., Gao, C., and Li, Y.: Depression Detection on Social Media with Large Language Models, arXiv [preprint] https://doi.org/10.48550/arXiv.2403.10750, 2024.
Maas, A. L., Daly, R. E., Pham, P. T., Huang, D., Ng, A. Y., and Potts, C.: Learning Word Vectors for Sentiment Analysis, ACL, https://aclanthology.org/P11-1015/ (last access: 5 September 2024), 2019.
Malko, A., Duenser, A., Kangas, M., Mollá-Aliod, D., and Paris, C.: Message similarity as a proxy to repetitive thinking: Associations with non-suicidal self-injury and suicidal ideation on social media, Comput. Hum. Behav. Rep., 11, 100320, https://doi.org/10.1016/j.chbr.2023.100320, 2023.
Moghadas, M., Fekete, A., Rajabifard, A., and Kötter, T.: The wisdom of crowds for improved disaster resilience: a near-real-time analysis of crowdsourced social media data on the 2021 flood in Germany, GeoJ., 88, 4215–4241, https://doi.org/10.1007/s10708-023-10858-x, 2023.
Mohammad, S., Kiritchenko, S., Sobhani, P., Zhu, X., and Cherry, C.: SemEval-2016 Task 6: Detecting Stance in Tweets, in: Proceedings of the 10th International Workshop on Semantic Evaluation (SemEval-2016), SemEval 2016, San Diego, California, 31–41, https://doi.org/10.18653/v1/S16-1003, 2016.
Nielsen, A. B., Landwehr, D., Nicolaï, J., Patil, T., and Raju, E.: Social media and crowdsourcing in disaster risk management: Trends, gaps, and insights from the current state of research, Risk Hazards Crisis Public Policy, 15, 104–127, https://doi.org/10.1002/rhc3.12297, 2024.
Oltmanns, J. R., Schwartz, H. A., Ruggero, C., Son, Y., Miao, J., Waszczuk, M., Clouston, S. A. P., Bromet, E. J., Luft, B. J., and Kotov, R.: Artificial intelligence language predictors of two-year trauma-related outcomes, J. Psych. Res., 143, 239–245, https://doi.org/10.1016/j.jpsychires.2021.09.015, 2021.
Olynk Widmar, N., Rash, K., Bir, C., Bir, B., and Jung, J.: The anatomy of natural disasters on online media: hurricanes and wildfires, Nat. Hazards, 110, 961–998, https://doi.org/10.1007/s11069-021-04975-4, 2022.
OpenAI, Achiam, J., Adler, S., Agarwal, S., Ahmad, L., Akkaya, I., Aleman, F. L., Almeida, D., Altenschmidt, J., Altman, S., Anadkat, S., Avila, R., Babuschkin, I., Balaji, S., Balcom, V., Baltescu, P., Bao, H., Bavarian, M., Belgum, J., Bello, I., Berdine, J., Bernadett-Shapiro, G., Berner, C., Bogdonoff, L., Boiko, O., Boyd, M., Brakman, A.-L., Brockman, G., Brooks, T., Brundage, M., Button, K., Cai, T., Campbell, R., Cann, A., Carey, B., Carlson, C., Carmichael, R., Chan, B., Chang, C., Chantzis, F., Chen, D., Chen, S., Chen, R., Chen, J., Chen, M., Chess, B., Cho, C., Chu, C., Chung, H. W., Cummings, D., Currier, J., Dai, Y., Decareaux, C., Degry, T., Deutsch, N., Deville, D., Dhar, A., Dohan, D., Dowling, S., Dunning, S., Ecoffet, A., Eleti, A., Eloundou, T., Farhi, D., Fedus, L., Felix, N., Fishman, S. P., Forte, J., Fulford, I., Gao, L., Georges, E., Gibson, C., Goel, V., Gogineni, T., Goh, G., Gontijo-Lopes, R., Gordon, J., Grafstein, M., Gray, S., Greene, R., Gross, J., Gu, S. S., Guo, Y., Hallacy, C., Han, J., Harris, J., He, Y., Heaton, M., Heidecke, J., Hesse, C., Hickey, A., Hickey, W., Hoeschele, P., Houghton, B., Hsu, K., Hu, S., Hu, X., Huizinga, J., Jain, S., et al.: GPT-4 Technical Report, arXiv [preprint], https://doi.org/10.48550/arXiv.2303.08774, 2024.
Ott, M., Edunov, S., Baevski, A., Fan, A., Gross, S., Ng, N., Grangier, D., and Auli, M.: fairseq: A Fast, Extensible Toolkit for Sequence Modeling, in: Proceedings of the 2019 Conference of the North American Chapter of the Association for Computational Linguistics (Demonstrations), Minneapolis, Minnesota, 48–53, https://doi.org/10.18653/v1/N19-4009, 2019.
Park, G., Schwartz, H. A., Eichstaedt, J. C., Kern, M. L., Kosinski, M., Stillwell, D. J., Ungar, L. H., and Seligman, M. E. P.: Automatic personality assessment through social media language, J. Personal. Social Psychol., 108, 934–952, https://doi.org/10.1037/pspp0000020, 2015.
Pfeiffer, J., Vulić, I., Gurevych, I., and Ruder, S.: MAD-X: An Adapter-Based Framework for Multi-Task Cross-Lingual Transfer, in: Proceedings of the 2020 Conference on Empirical Methods in Natural Language Processing (EMNLP), EMNLP 2020, Online, 7654–7673, https://doi.org/10.18653/v1/2020.emnlp-main.617, 2020.
Pires, T., Schlinger, E., and Garrette, D.: How Multilingual is Multilingual BERT?, in: Proceedings of the 57th Annual Meeting of the Association for Computational Linguistics, ACL 2019, Florence, Italy, 4996–5001, https://doi.org/10.18653/v1/P19-1493, 2019.
Raffel, C., Shazeer, N., Roberts, A., Lee, K., Narang, S., Matena, M., Zhou, Y., Li, W., and Liu, P. J.: Exploring the Limits of Transfer Learning with a Unified Text-to-Text Transformer, J. Mach. Learn. Res., 21, 1−-67, 2020.
Recharla, N., Bolimera, P., Gupta, Y., and Madasamy, A. K.: Depression Severity Detection from Social Media Posts, in: Emergent Converging Technologies and Biomedical Systems, Singapore, Springer, 403–417, https://doi.org/10.1007/978-981-99-8646-0_32, 2024.
Riddell, H. and Fenner, C.: User-Generated Crisis Communication: Exploring Crisis Frames on Twitter during Hurricane Harvey, South. Commun. J., 86, 31–45, https://doi.org/10.1080/1041794X.2020.1853803, 2021.
Ruan, T., Kong, Q., McBride, S. K., Sethjiwala, A., and Lv, Q.: Cross-platform analysis of public responses to the 2019 Ridgecrest earthquake sequence on Twitter and Reddit, Sci. Rep., 12, 1634, https://doi.org/10.1038/s41598-022-05359-9, 2022.
Ruder, S., Peters, M. E., Swayamdipta, S., and Wolf, T.: Transfer Learning in Natural Language Processing, in: Proceedings of the 2019 Conference of the North American Chapter of the Association for Computational Linguistics: Tutorials, Minneapolis, Minnesota, 15–18, https://doi.org/10.18653/v1/N19-5004, 2019.
RÚV Grindvíkingar geti sett sig í spor fólks með kæfisvefn: https://www.ruv.is/frett/2021/03/16/grindvikingar-geti-sett-sig-i-spor-folks-med-kaefisvefn (last access: 30 September 2021), 2021a.
RÚV Skjálftakvíði vegna aðstæðna sem maður ræður ekki við: https://www.ruv.is/frett/2021/03/01/skjalftakvidi-vegna-adstaedna-190 sem-madur-raedur-ekki-vid (last access: 30 September 2021), 2021b.
Rymer, H.: Part VII Volcanic Hazards, in: The Encyclopedia of Volcanoes, 2nd Edn., edited by: Sigurdsson, H., Academic Press, Amsterdam, 895–896, https://doi.org/10.1016/B978-0-12-385938-9.02009-5, 2015.
Saraò, A., Tamaro, A., Sandron, D., Slejko, D., and Rebez, A.: On the crowdsourcing of macroseismic data to characterize geological settings, Int. J. Disast. Risk Reduct., 96, 103934, https://doi.org/10.1016/j.ijdrr.2023.103934, 2023.
Siebert, L., Cottrell, E., Venzke, E., and Andrews, B.: Chapter 12 – Earth's Volcanoes and Their Eruptions: An Overview, in: The Encyclopedia of Volcanoes, 2nd Edn., edited by: Sigurdsson, H., Academic Press, Amsterdam, 239–255, https://doi.org/10.1016/B978-0-12-385938-9.00012-2, 2015.
Sigmundsson, F., Parks, M., Hooper, A., Geirsson, H., Vogfjörd, K. S., Drouin, V., Ófeigsson, B. G., Hreinsdóttir, S., Hjaltadóttir, S., Jónsdóttir, K., Einarsson, P., Barsotti, S., Horálek, J., and Ágústsdóttir, T.: Deformation and seismicity decline before the 2021 Fagradalsfjall eruption, Nature, 609, 523–528, https://doi.org/10.1038/s41586-022-05083-4, 2022.
Snæbjarnarson, V. and Einarsson, H.: Cross-Lingual QA as a Stepping Stone for Monolingual Open QA in Icelandic, arXiv [preprint], https://doi.org/10.48550/arXiv.2207.01918, 2022.
Snæbjarnarson, V., Simonsen, A., Glavaš, G., and Vulić, I.: Transfer to a Low-Resource Language via Close Relatives: The Case Study on Faroese, in: Proceedings of the 24th Nordic Conference on Computational Linguistics (NoDaLiDa), NoDaLiDa 2023, 22–24 May 2023, Tórshavn, Faroe Islands, 728–737, https://doi.org/10.48550/arXiv.2304.08823, 2023.
Snæbjarnarson, V.: vesteinn/fagradalsfjall_eruption_sentiment: v1.0 (v1.0), Zenodo [code and data set], https://doi.org/10.5281/zenodo.13733750, 2024.
Socher, R., Perelygin, A., Wu, J., Chuang, J., Manning, C. D., Ng, A., and Potts, C.: Recursive Deep Models for Semantic Compositionality Over a Sentiment Treebank, in: Proceedings of the 2013 Conference on Empirical Methods in Natural Language Processing, EMNLP 2013, Seattle, Washington, USA, 18–21 October 2013, 1631–1642, https://aclanthology.org/D13-1170 (last access: 16 September 2024), 2013.
Steed, R. J., Fuenzalida, A., Bossu, R., Bondár, I., Heinloo, A., Dupont, A., Saul, J., and Strollo, A.: Crowdsourcing triggers rapid, reliable earthquake locations, Sci. Adv., 5, eaau9824, https://doi.org/10.1126/sciadv.aau9824, 2019.
Vaswani, A., Shazeer, N., Parmar, N., Uszkoreit, J., Jones, L., Gomez, A. N., Kaiser, Ł., and Polosukhin, I.: Attention is All you Need, in: Advances in Neural Information Processing Systems, NIPS'17: Proceedings of the 31st International Conference on Neural Information Processing Systems Long Beach California USA, 4–9 December 2017, https://proceedings.neurips.cc/paper_files/paper/2017/file/3f5ee243547dee91fbd053c1c4a845aa-Paper.pdf (last access: 5 September 2024), 2017.
Venkit, P., Srinath, M., Gautam, S., Venkatraman, S., Gupta, V., Passonneau, R., and Wilson, S.: The Sentiment Problem: A Critical Survey towards Deconstructing Sentiment Analysis, in: Proceedings of the 2023 Conference on Empirical Methods in Natural Language Processing, EMNLP 2023, Singapore, 13743–13763, https://doi.org/10.18653/v1/2023.emnlp-main.848, 2023.
Vo, B.-K. H. and Collier, N.: Twitter Emotion Analysis in Earthquake Situations, IJCLA, 4, 15, 2013.
Volcanic eruption in Geldingadalir: Icelandic Tourism Dashboard, https://www.maelabordferdathjonustunnar.is/en/volcanic-eruption-in-geldingadalir (last access: 30 September 2021), 2021
Wadsworth, F. B., Llewellin, E. W., Farquharson, J. I., Gillies, J. K., Loisel, A., Frey, L., Ilyinskaya, E., Thordarson, T., Tramontano, S., Lev, E., Pankhurst, M. J., Rull, A. G., Asensio-Ramos, M., Pérez, N. M., Hernández, P. A., Calvo, D., Solana, M. C., Kueppers, U., and Santabárbara, A. P.: Crowd-sourcing observations of volcanic eruptions during the 2021 Fagradalsfjall and Cumbre Vieja events, Nat. Commun., 13, 2611, https://doi.org/10.1038/s41467-022-30333-4, 2022.
Wang, C., Engler, D., Li, X., Hou, J., Wald, D. J., Jaiswal, K., and Xu, S.: Near-real-time Earthquake-induced Fatality Estimation using Crowdsourced Data and Large-Language Models, arXiv [preprint], https://doi.org/10.48550/arXiv.2312.03755, 2023.
Wang, Z. and Ye, X.: Social media analytics for natural disaster management, Int. J. Geogr. Inform. Sci., 32, 49–72, https://doi.org/10.1080/13658816.2017.1367003, 2018.
Watson, C. S., Elliott, J. R., Ebmeier, S. K., Biggs, J., Albino, F., Brown, S. K., Burns, H., Hooper, A., Lazecky, M., Maghsoudi, Y., Rigby, R., and Wright, T. J.: Strategies for improving the communication of satellite-derived InSAR data for geohazards through the analysis of Twitter and online data portals, Geosci. Commun., 6, 75–96, https://doi.org/10.5194/gc-6-75-2023, 2023.
Wolf, T., Debut, L., Sanh, V., Chaumond, J., Delangue, C., Moi, A., Cistac, P., Rault, T., Louf, R., Funtowicz, M., Davison, J., Shleifer, S., von Platen, P., Ma, C., Jernite, Y., Plu, J., Xu, C., Le Scao, T., Gugger, S., Drame, M., Lhoest, Q., and Rush, A.: Transformers: State-of-the-Art Natural Language Processing, in: Proceedings of the 2020 Conference on Empirical Methods in Natural Language Processing: System Demonstrations, Online, 38–45, https://doi.org/10.18653/v1/2020.emnlp-demos.6, 2020.
Zimbra, D., Abbasi, A., Zeng, D., and Chen, H.: The State-of-the-Art in Twitter Sentiment Analysis: A Review and Benchmark Evaluation, ACM Trans. Manag. Inf. Syst., 9, 1–29, https://doi.org/10.1145/3185045, 2018.
Zou, L., Lam, N. S. N., Shams, S., Cai, H., Meyer, M. A., Yang, S., Lee, K., Park, S.-J., and Reams, M. A.: Social and geographical disparities in Twitter use during Hurricane Harvey, Int. J. Digit. Earth, 12, 1300–1318, https://doi.org/10.1080/17538947.2018.1545878, 2019.