the Creative Commons Attribution 4.0 License.
the Creative Commons Attribution 4.0 License.
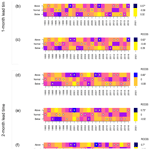
Seasonal fire danger forecasts for supporting fire prevention management in an eastern Mediterranean environment: the case of Attica, Greece
Konstantinos V. Varotsos
Christos Giannakopoulos
Panagiotis P. Nastos
Maria Hatzaki
Forest fires constitute a major environmental and socioeconomic hazard in the Mediterranean. Weather and climate are among the main factors influencing forest fire potential. As fire danger is expected to increase under changing climate, seasonal forecasting of meteorological conditions conductive to fires is of paramount importance for implementing effective fire prevention policies. The aim of the current study is to provide high-resolution (∼9 km) probabilistic seasonal fire danger forecasts, utilizing the Canadian Forest Fire Weather Index (FWI) for the Attica region, one of the most fire-prone regions in Greece and the Mediterranean, employing the fifth-generation ECMWF seasonal forecasting system (SEAS5). Results indicate that, depending on the lead time of the forecast, both the FWI and ISI (Initial Spread Index) present statistically significant high discrimination scores and can be considered reliable in predicting above-normal fire danger conditions. When comparing the year-by-year fire danger predictions with the historical fire occurrence recorded by the Hellenic Fire Service database, both seasonal FWI and ISI forecasts are skilful in identifying years with a high number of fire occurrences. Overall, fire danger and its subcomponents can potentially be exploited by regional authorities in fire prevention management regarding preparedness and resources allocation.
- Article
(6955 KB) -
Supplement
(1062 KB) - BibTeX
- EndNote
The Mediterranean region includes more than 25×106 ha of forests and about 50×106 ha of other wooded lands that make vital contributions to rural development, poverty alleviation and food security, as well as to the agriculture, water, tourism and energy sectors (FAO and Plan Bleu, 2018). The Mediterranean is considered a high-fire-risk region where fires cause severe environmental and economic losses and even loss of human lives (MedECC, 2020). Severe forest fires have consistently affected Europe since the beginning of the century, especially regarding the five European Mediterranean countries of Portugal, Spain, Italy, Greece and France, which on average collectively account for approximately 85 % of the total burnt area in Europe per year (Costa et al., 2020).
Weather and climate, vegetation conditions and composition, and human activities play an essential role in fire regimes (Costa et al., 2020). According to Rogers et al. (2020), climate highly affects fuel properties and short-term weather patterns determine fuel moisture and physical conditions necessary for fire spread. Regarding the Mediterranean, the combination of extreme drought with extreme winds or heatwaves has been identified as a crucial factor for the occurrence of wildfires (Ruffault et al., 2020). Under changing climatic conditions, future fire danger and the frequency and the extent of large wildfires are expected to increase throughout the Mediterranean basin (Dupuy et al., 2020; Ruffault et al., 2020; Turco et al., 2018). According to Moreira et al. (2020), burnt areas may be further amplified by land use and management changes that increase fuel load and continuity.
Fire management strategies in Mediterranean Europe place an emphasis on fire suppression, which can indeed lead to a higher fuel load and fuel connectivity as encapsulated in the term “firefighting trap”, which culminates in hindering suppression under extreme fire weather, ultimately leading to more severe and usually larger fires (Moreira et al., 2020). Fire management should be enriched, comprising also prevention and adaptation measures (Alcasena et al., 2019; Fernandes, 2013). This holistic point of view has been included in the new EU Forest Strategy for 2030 (European Commission, 2021) that explicitly considers fire prevention as an integral component for maintaining and enhancing the resilience of European forests. Further underlining this, in the recent report on wildfires of the United Nations Environment Programme (2022), a radical change in government spending on wildfires was called for, with the aim to rebalance governments' investment from reaction and response to prevention and preparedness.
Seasonal forecasting of weather conditions conducive to fires (fire weather) is of paramount importance for implementing effective fire prevention. The prediction of unfavourable conditions prior to each fire season may support policymakers and civil protection agencies in implementing adequate fuel management policies in vulnerable regions, along with optimizing firefighting resources to mitigate the adverse effects of forest fires (Turco et al., 2019).
For the relationship between meteorological conditions and fire danger, different indices are used worldwide that assess fire danger for research and operational purposes with the Canadian Forest Fire Weather Index (FWI) being one of the most widely used systems (Field et al., 2015). The FWI has been shown to correlate well with fire activity globally (Abatzoglou et al., 2018; Bedia et al., 2015) and regionally, including parts of Europe (e.g. Dupuy et al., 2020; Karali et al., 2014; Ruffault et al., 2020). Since 2007, the FWI has been adopted at the EU level by the European Forest Fire Information System (EFFIS), a component of the Copernicus Emergency Management Service (CEMS), to assess fire danger level in a harmonized way throughout Europe after several tests on its validity and robustness for the European domain (San-Miguel-Ayanz et al., 2012). EFFIS provides short-term FWI forecasts, as well as monthly and seasonal forecasts of temperature and rainfall anomalies that are expected to prevail over European and Mediterranean areas for a time window of 7 months. To the best of our knowledge, only two studies so far have assessed seasonal fire danger predictions for Europe. The first study is by Bedia et al. (2018), in which the authors provided seasonal probabilistic predictions of the FWI for Mediterranean Europe by utilizing the ECMWF System 4, focusing on the calibration of model outputs prior to forecast verification, as well as on the analysis of FWI forecast quality compared to reference observational values. In the second study by Costa-Saura et al. (2022), the performance of different seasonal forecasting systems to predict several indicators relevant to forestry and agriculture for central Europe and the Mediterranean, including the FWI, was assessed.
The current study aims to provide high-resolution probabilistic FWI seasonal forecasts for Attica, Greece, employing the methodology of Bedia et al. (2018) and further expanding it through statistical downscaling. Moreover, it aims to assess the ability of these forecasts to provide robust information and support fire management decisions in the Attica region. Attica encompasses the entire metropolitan area of Athens, the country's capital and largest city with approximately 3.8 million inhabitants (census of 2021). It is one of the country's most vulnerable regions to rural and peri-urban forest fires due to its complex topography, flammable vegetation, high concentration of population and activities as well as its extensive wildland–urban interface (WUI) (Mitsopoulos et al., 2020; Salvati and Ranalli, 2015).
The catastrophic fires that took place in Attica during the summer of 2021 that burnt more than 150 000 ha (Evelpidou et al., 2022) of forests and arable land underpinned the timeliness and need for this study. These fires broke out during the most severe and the longest heatwave (maximum daily temperature reached 43.9 ∘C, while heatwave conditions prevailed for 10 d) to have occurred in Attica in the last decades according to the meteorological records of the National Observatory of Athens. Our assessment includes the verification of the FWI–SEAS5 (ECMWF's seasonal forecast system) forecasts against gridded observations using a probabilistic tercile-based approach and a qualitative comparison of predicted years with above-normal fire danger conditions using historical fire occurrence data.
The paper is organized as follows. In the next section, the data and methods are introduced. In Sect. 3, the results of the forecast performance of the FWI, its subcomponents and the input meteorological variables to the FWI system for Attica region are presented, together with the results of the qualitative evaluation of above-normal fire danger conditions against historical fire occurrence data. In Sect. 4, the performance of the single meteorological variables, the impact of spin-up and lead time on fire danger forecast performance, and the qualitative evaluation of fire danger forecasts are discussed. Finally, in Sect. 5, the main conclusions and suggestions for future work are discussed.
2.1 Canadian Forest Fire Weather Index (FWI)
The FWI is a daily meteorologically based system used worldwide to estimate fire danger in a generalized fuel type (mature pine forest; van Wagner, 1987). According to Wotton (2009), fire danger refers to the assessment of both the static and dynamic factors of the fire environment which determine the ease of ignition, rate of spread, difficulty of control and impact of a fire. The meteorological inputs to the system are daily noon values of air temperature, relative humidity, wind speed and 24 h precipitation (Stocks et al., 1989). The FWI system consists of six subcomponents each measuring a different aspect of fire danger (van Wagner, 1987). The first three primary sub-indices are fuel moisture codes, which are numeric ratings of the moisture content of the forest floor and other dead organic matter. The Fine Fuel Moisture Code (FFMC) is a numeric rating of the moisture content of litter and other cured fine fuels. The FFMC is an indicator of the relative ease of ignition and the flammability of fine fuel, having a fast response to weather variations (approximately 0.5 d under “standard” conditions, i.e. noon temperature of 25 ∘C, relative humidity of 30 % and wind speed of 10 km h−1). The Duff Moisture Code (DMC) is a numeric rating of the average moisture content of loosely compacted organic layers of moderate depth. This code gives an indication of fuel consumption and is characterized by a medium-term response to weather variations (approximately 10 d). The Drought Code (DC) is a numeric rating of the average moisture content of deep, compact organic layers. The DC has a long-term response (about 50 d) to weather variations and is a useful indicator of seasonal drought effects on forest fuels, as well as the amount of smouldering in deep duff layers and large logs. The two intermediate sub-indices, the Initial Spread Index (ISI) and Buildup Index (BUI), are fire behaviour indices. The ISI is a numerical rating of the expected fire rate of spread which combine the effect of wind and the FFMC. The BUI is a numerical rating of the total amount of fuel available for combustion that combines the DMC and DC. The resulting index is the Canadian Forest Fire Weather Index (FWI), which combines the ISI and BUI. The FWI represents frontal fire intensity (van Wagner, 1987) and can be used as a general index of fire danger (Wotton, 2009). Each component of the FWI System has its own scale, but for all of them a higher value indicates more severe burning conditions (de Groot, 1987). A more analytical description of the FWI system and its subcomponents can be found in van Wagner (1987) and Wotton (2009). The structure of the index and the meteorological variables needed for its calculation are presented in Fig. S1 in the Supplement.
2.2 Seasonal forecast data and reference observations
2.2.1 ECMWF SEAS5 dataset
In the framework of the current study, the fifth-generation ECMWF seasonal forecasting system (SEAS5) (Johnson et al., 2019), available in the Copernicus Climate Change Service (C3S) Climate Data Store (CDS) (https://doi.org/10.24381/cds.181d637e; Copernicus Climate Change Service, 2018), was utilized. SEAS5 has been operational since November 2017, replacing System 4. The system includes updated versions of the atmospheric (Integrated Forecasting System, IFS) and ocean (Nucleus for European Modelling of the Ocean, NEMO) models with the addition of the interactive sea ice model LIM2 (Louvain-la-Neuve sea ice model; Johnson et al., 2019). The set of re-forecasts (hindcasts) available in the CDS starts on the first day of every month for the years 1993–2016 and contains 25 ensemble members. The data from these re-forecasts are used to verify the forecasting system and calibrate real-time forecast products. Real-time forecasts (from 2017 onwards) consist of a 51-member ensemble initialized every month and integrated for 7 months. The seasonal forecasts are initialized with atmospheric conditions from ERA-Interim (Dee et al., 2011) until 2016 and the ECMWF operational analysis since 2017. Re-forecast and forecast data are available at a global grid.
For the daily FWI calculations, the SEAS5 instantaneous outputs at 12:00 UTC for 2 m air temperature, northward and eastward 10 m wind components, 2 m dew point temperature, and daily accumulated precipitation were used. 12:00 UTC was used as a proxy for local noon values required as input to the FWI as proposed by several previous studies for the Mediterranean and Greece (e.g. Bedia et al., 2012, 2018; Herrera et al., 2013; Papagiannaki et al., 2020). Additionally, according to Papagiannaki et al. (2020), during the fire season the meteorological conditions at 12:00 UTC (i.e. 15:00 LST) are highly conductive to the occurrence and spread of fires as corroborated by the Hellenic Fire Service; thus, the respective fire danger predictions are considered to be particularly useful from an operational perspective. Moreover, relative humidity needed for FWI calculations was computed from air and dew point temperatures. Concerning precipitation, data correspond to the accumulated values since the initialization time; therefore differences with the previous day's values were computed (de-accumulation) to obtain daily accumulated values for each grid point.
It should be noted that in order to commence the calculations of the FWI, default initial values of the FFMC, DMC and DC were used. This means that a spin-up period was required to minimize the effects of errors in the initial conditions used in its calculation. Given that the longest time lag of the fuel moisture codes, as described above, is about 50 d, a spin-up period of up to 2 months was considered sufficient for both the FWI and/or its subcomponents. A fire season spanning from May to September (MJJAS) that coincides with the dry season in Attica according to the records of the Hellenic National Meteorological Service was considered, and six different experimental setups for FWI calculations were implemented. In particular, we performed SEAS5 MJJAS fire danger forecasts initialized in March and April (2 months and 1 month in advance of the target fire season, respectively), without and with spin-up, using both SEAS5 and ERA5-Land data (Fig. 1). In the case of spin-up, in 1-month (2-month) lead time forecasts, the FWI time series for April (March and April) were firstly calculated for the index to stabilize and were then removed from the analysis.
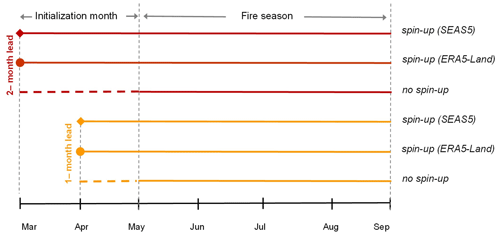
Figure 1Experimental setups used for FWI calculations. Forecasts are initialized in April (1-month lead time, in yellow) and March (2-month lead time, in red), while three different experiments concerning the spin-up period (a) with no spin-up (dashed line), (b) with spin-up implanting the ERA5-Land data (solid line–circle symbol) and (c) with spin-up using the SEAS5 model data (solid line–diamond symbol) are shown.
2.2.2 ERA5-Land reanalysis dataset
As a reference observational dataset, the state-of-the-art global reanalysis dataset ERA5-Land of the Copernicus CDS (https://doi.org/10.24381/cds.e2161bac; Muñoz-Sabater, 2019) was used. ERA5-Land comes with a series of improvements compared to ERA5 making it more accurate for all types of land applications. The dataset provides a total of 50 variables describing the water and energy cycles over land globally and hourly and at a spatial resolution of 9 km from 1950 to present (Muñoz-Sabater et al., 2021). To be consistent with the SEAS5 data, 2 m air temperature, 2 m dew point temperature, 10 m northward and eastward wind components at 12:00 UTC, and daily accumulated precipitation were used for the calculation of daily FWI values.
2.3 Statistical downscaling of seasonal forecasts
To statistically downscale the seasonal forecasts at the ERA5-Land horizontal resolution, a two-step approach was followed. In particular, the seasonal forecast meteorological variables used to calculate the FWI were initially regridded to the ERA5-Land grid by means of bilinear interpolation, and next, bias correction was applied using empirical quantile mapping (EQM). This two-step approach is the reversed order of the framework of bias correction and spatial disaggregation, which has been previously used to statistically downscale global and/or regional models for both climate change and seasonal forecast studies (Lorenz et al., 2021; Marcos et al., 2018; Varotsos et al., 2022). Regarding the bias correction method, EQM works by adjusting the 1st–99th percentiles of the predicted empirical probability density function (PDF) based on the observed empirical PDF, while for lower or higher values falling outside this range, a constant extrapolation is applied using the correction obtained for the 1st or 99th percentile, respectively. For more information on how EQM works, the reader may refer to the studies of Manzanas et al. (2018, 2019), Manzanas (2020) and Bedia et al. (2018).
In this study bias correction was applied using daily data for the period May to September using a moving window width of 31 d to adjust the intra-seasonal biases originating from the model's behaviour (i.e. model drift, Manzanas, 2020, and references therein). Following Bedia et al. (2018), the FWI was bias-corrected after its calculation from the regridded fields of temperature, relative humidity, wind speed and precipitation to avoid unrealistic FWI trends that could occur by calculating the FWI from the bias-corrected meteorological variables. Nevertheless, results of the statistically downscaled temperature, relative humidity, wind speed and precipitation are also presented in the following sections.
2.4 Metrics and methodology of fire danger forecast verification
According to WMO (2020), measures of historical predictive skill are an essential component of seasonal forecasts as they provide the users an indication of the trustworthiness of the real-time forecasts. There are many different skill measures describing the quality of specific forecast attributes that are estimated by calculating the corresponding properties of the set (e.g. discrimination, reliability) of hindcasts paired with reference observations (WMO, 2020). In the framework of the current study, the probabilistic relative operating characteristic (ROC) skill score, measuring forecast discrimination, together with the reliability diagrams were used to assess the potential skill and usefulness of fire danger seasonal forecasts after spatial disaggregation and bias adjustment.
2.4.1 ROC skill score (ROCSS)
ROC skill measures the frequency of occasions when the system correctly distinguished between events occurring and not occurring (Jolliffe and Stephenson, 2003). The ROC is based on the ratio between the hit rate and the false alarm rate and is evaluated separately for each category (above normal, normal or below normal). The ROC skill score (ROCSS) ranges from −1 (perfectly bad discrimination) to 1 (perfectly good discrimination). A value of zero indicates no skill compared to a random prediction or the climatological value.
As in previous studies (e.g. Bedia et al., 2018; Manzanas et al., 2014; Mercado-Bettín et al., 2021), a tercile-based probabilistic approach for forecast verification was applied. In order to assess fire danger forecast performance, the easyVerification (MeteoSwiss, 2017), SpecsVerification (Siegert, 2020) and visualizeR (Frías et al., 2018) R packages were used for skill calculation and visualization. The ROCSSs were calculated at each grid point for the different tercile categories depending on the examined parameter, e.g. the upper tercile for the FWI, temperature and wind speed or the lower tercile for relative humidity and precipitation, averaged over the verification period, and maps depicting the spatial variations in their skill scores for the different initialization times were constructed.
Moreover, tercile plots for the FWI (and its subcomponents) for Attica were built to complement the spatial analysis provided by the ROCSS maps, presenting the performance of the seasonal forecast along the hindcast period. In order to build a tercile plot for a given variable, the observations along with the bias-corrected multi-member ensemble predictions were categorized into three tercile categories, considering values above (upper tercile), between (middle tercile) or below (lower tercile) the respective climatological values within the 1993–2016 period. Subsequently, a probabilistic forecast was computed year by year considering the number of members falling within each category. Moreover, the observed category according to the ERA5-Land dataset is provided in the plot to facilitate a visual comparison of hits and misses of the forecast system along the hindcast period.
2.4.2 Reliability diagrams
Reliability diagrams are diagnostic tools measuring how closely the forecast probabilities of a specific event (for instance a particular tercile category) correspond to the observed frequency of that event (Weisheimer and Palmer, 2014). According to WMO (2020), in the context of decision-making, forecast reliability plays an important role in making a prior assessment of the benefits of using seasonal forecast information. A construction of a reliability diagram involves binning forecasts by probability category and plotting these values against the observed frequencies (WMO, 2020). For a perfectly reliable forecasting system, the line obtained would match the diagonal (perfect reliability line). The reliability line that best fits the points in the diagram is calculated, applying least-squares regression weighted by the number of forecasts in each probability bin. Based on the slope of the reliability line and the uncertainty associated with it, six easy-to-interpret categories can be defined: perfect, still very useful, marginally useful+, marginally useful, not useful and dangerously useless (Manzanas et al., 2018). The marginally useful+ category differentiated those cases for which the reliability line lies within the skill region (Brier skill score >0, shaded in grey). The reader can refer to Frías et al. (2018) and Manzanas et al. (2018) for more information on the construction of the reliability diagrams.
It should be noted that concerning the FWI (and its subcomponents), both in the tercile maps/plots and the reliability diagrams, only the results of the above-normal conditions (upper-tercile category) are discussed in the main body of the paper, as high FWI (and its subcomponents) values are related to increased fire danger conditions and, hence, to increased wildfire activity (e.g. Urbieta et al., 2015).
2.5 Qualitative evaluation of above-normal fire danger conditions against historical fire occurrence
A qualitative evaluation of the ability of FWI hindcasts to predict actual fire occurrence as obtained by historical fire records was performed. To this aim, records of national wildfire time series data for the period between 2000 and 2016 were obtained from the Hellenic Fire Service online database (https://www.fireservice.gr/el_GR/synola-dedomenon, last access: 10 November 2022). As these data concern both forest and urban fires, only fire events that burnt at least 1 ha of forest or forested areas were extracted from the database.
Burnt areas less than 1 ha were excluded from our analysis to limit the uncertainties associated with the recording of small fires in fire databases as was reported in previous studies (Jiménez-Ruano et al., 2017; Turco et al., 2013). Regarding the number of fires and the respective burnt areas, these were constrained for the months covering the fire season as defined in the current study (i.e. from May to September). We decided to exclude the fire data for the hindcast years between 1993 and 1999, as they were recorded by the Hellenic Forest Service following a different methodology that is not compatible with the Fire Service's one.
Regarding the approach to the qualitative evaluation, the years between 2000 and 2016 were characterized as high-fire-activity years since the number of fire events for the entire Attica domain for each year was greater than the median of the fire events observed for the whole period. Moreover, only the years with fire danger (based on ERA5-Land) in the upper-tercile category (above-normal conditions) were selected from the tercile plots for Attica, and the relevant proportion of ensemble members predicting upper-tercile values was recorded. Consequently, the number of fires per year was shown along with the abovementioned proportion.
The Results section is organized in two parts presenting the following: (a) the forecast performance of the FWI, its subcomponents and the input meteorological variables to the FWI system and (b) the qualitative evaluation of above-normal fire danger conditions against historical fire occurrence data.
3.1 Forecast performance of meteorological variables and fire danger components
The quality of the downscaled fire danger hindcasts for Attica was initially assessed via the ROCSS. In Fig. 2, the spatial distribution of the ROCSS for the upper-tercile category of the FWI for the MJJAS fire season for both lead times, with and without the performance of spin-up, are presented. Statistically significant ROCSS values greater than 0.4 were found almost in the entire domain for the 1-month lead time experiments, while higher scores (>0.6) were found for the 2-month lead time experiments. In order to complement this spatial analysis, Fig. 3 depicts the tercile plots of the FWI averaged over Attica, for both lead time experiments providing a year-to-year visual comparison between hindcast tercile categories and the corresponding observed values as obtained by ERA5-Land. These spatially averaged predictions for the upper tercile of the FWI for all experiments indicate a statistically significant positive ROCSS resonating the spatial analysis results (Fig. 2). Reaching increasingly higher values, for the 1-month lead time forecasts, the ROCSS was 0.45 for the no spin-up experiment, 0.57 with spin-up using the SEAS5 model data and 0.62 when the ERA5-Land data were implanted in the spin-up procedure. For the 2-month lead time forecasts, higher ROCSSs were calculated for all spin-up experiments compared to a 1-month lead (the attained values were 0.66, 0.73 and 0.7, respectively) with the SEAS5 performing slightly better than the observations. Regarding the temporal performance on a year-by-year basis, both lead time experiments depicted high agreement (60 %–80 %) among the members for half of the years with observed above-normal conditions.
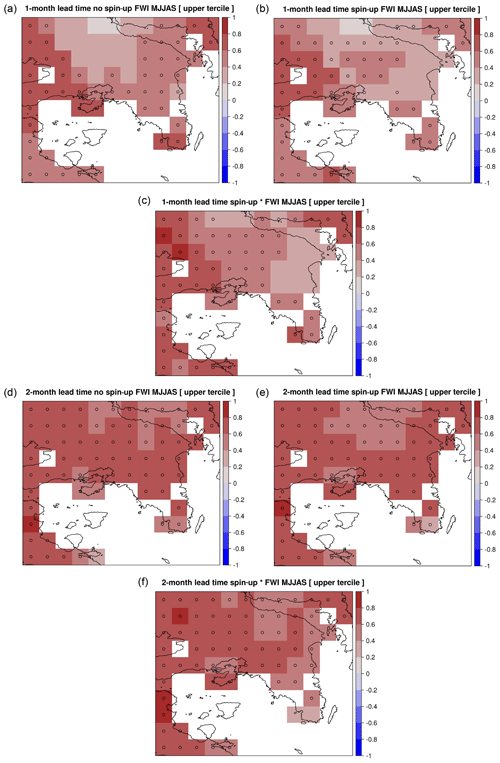
Figure 2ROC skill scores (ROCSSs) of the upper-tercile SEAS5 FWI predictions for a 1-month lead time – (a) with no spin-up, (b) with spin-up using the SEAS5 data and (c) with spin-up implanting the ERA5-Land data – and a 2-month lead time – (d) with no spin-up, (e) with spin-up using the SEAS5 data and (f) with spin-up implanting the ERA5-Land data. The grid points with significant ROCSS values are indicated by circles (α=0.05).
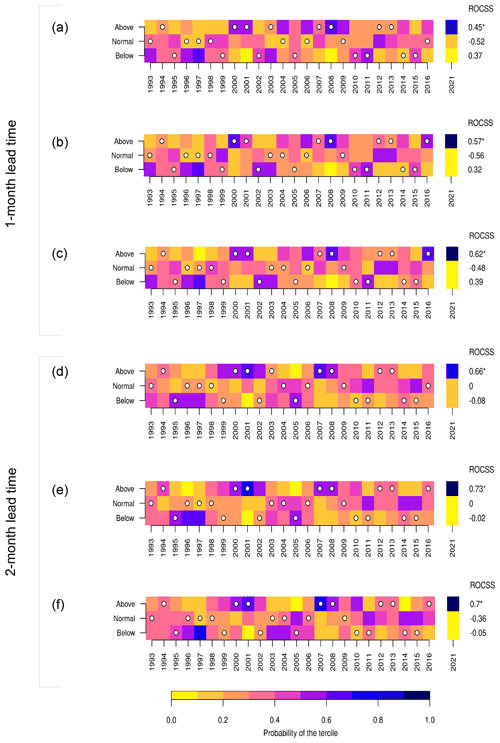
Figure 3Tercile plots for May–September FWI predictions covering the hindcast period (1993–2016) for a 1-month lead time – (a) with no spin-up, (b) with spin-up using the SEAS5 model data and (c) with spin-up implanting the ERA5-Land data – and for a 2-month lead time – (d) with no spin-up, (e) with spin-up using the SEAS5 model data and (f) with spin-up implanting the ERA5-Land data. Forecast probabilities for the three tercile categories are codified in a scale ranging from yellow (0, no member forecasts in one category) to blue (1, all the members in the same category). The white bullets represent the observed category according to the ERA5-Land dataset. ROCSS values obtained from the hindcast period are shown on the right side of each category, and the asterisk indicates significant values (α=0.05).
To further elaborate on the fire danger forecast verification, the reliability diagrams are presented in Figs. S2–S3. The upper-tercile FWI predictions for 2-month lead time experiments were classified as perfectly reliable, while predictions fell in the marginally useful+ category for 1-month lead time experiments.
Considering the forecasted meteorological variables used in FWI calculations, the ROCSSs were calculated for 1-month and 2-month lead time forecasts only when the variable indicates high-fire-danger conditions, i.e. high air temperature, low relative humidity, low total precipitation and high wind speed. Thus, the ROCSS for the upper-tercile category of air temperature and wind speed, as well as the lower-tercile category of relative humidity and total precipitation, for the different lead times, are presented in Fig. 4. Both lead time forecasts of relative humidity and wind speed exhibited high discrimination skills and temperature exhibited low skill almost for the entire domain, while precipitation showed no skill for both experiments. In particular, for relative humidity, statistically significant ROCSSs, greater than 0.6 for the 1-month lead time forecast, were attained for the entire domain, while the ROCSS ranges between 0.6 and 0.8 for the 2-month lead time forecast. For wind speed, statistically significant discrimination skill scores between 0.4 and 1.0 were attained for the 1-month lead time, while lower values (0.4–0.6) were found for the 2-month lead time forecast mainly in the eastern part of the area of interest. Overall, the highest skills averaged over the study domain were found for the lower tercile of relative humidity (0.73, perfect), the upper tercile of wind (0.45, marginally useful+) and the upper tercile of temperature (0.34, marginally useful+) for 1-month lead time forecasts (not shown).
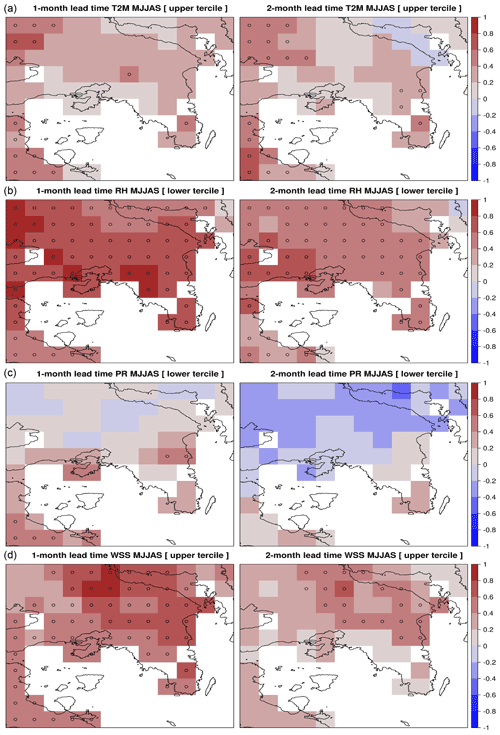
Figure 4ROCSSs of the FWI input variables for 1-month (left column) and 2-month (right column) lead time forecasts that correspond to high-fire-danger values: (a) upper tercile of air temperature (T2M), (b) lower tercile of air relative humidity (RH), (c) lower tercile of total precipitation (PR) and (d) upper tercile of wind speed (WSS). The grid points with significant ROCSS values are indicated by circles (α=0.05).
Given that relative humidity and wind speed demonstrated high discrimination skill for both lead time experiments, the ROCSSs of the FWI subcomponents that directly depend on these variables were further investigated. In particular, the ROCSSs for the Fine Fuel Moisture Code (FFMC) and Duff Moisture Code (DMC), which receive relative humidity as input variable, as well as the Initial Spread Index (ISI), which integrates the fuel moisture of fine fuels (FFMC) and near-surface wind speed, were assessed. All fuel moisture subcomponents presented poor discrimination scores (ROCSS <0.3 averaged over the area) for both lead time experiments and depending on the spin-up experiment were classified as not useful or dangerously useless (not shown). The only exceptions are the 1-month lead time FFMC forecast without spin-up and the 2-month lead time DC with spin-up using observations, which were classified as marginally useful+ (not shown). The ISI differs as can be seen in Fig. 5, showing the spatial distribution of the ROCSS, where statistically significant scores (>0.4) were found almost in the entire domain for both lead time experiments, with higher scores depicted for the 1-month lead time. Moreover, the spatial pattern of the ROCSS does not differ within each lead time experiment between the different spin-up experiments. Looking into the tercile plots (Fig. 6), it is evident that the highest ROCSSs for ISI upper-tercile predictions are found for the 1-month lead time experiments, having minor differences between the different spin-up experiments (0.85–0.87). Lower values were found for the 2-month lead time experiments; however, the ROCSSs remain high (0.6). From the interannual perspective, concerning 1-month lead time ISI forecasts, most of the observed above-normal years were fairly predicted by SEAS5 (by 50 %–60 % of the members). For 2-month lead time experiments, ISI hindcasts tend to underestimate the observed above-normal events, as less than 40 % of the above-normal years were predicted by most of the members (by more than 60 % of the members). Moreover, the FWI and ISI forecast probabilities for the 2021 fire season are presented in Figs. 3 and 6. Here, most of the ensemble members (>70 %) predict above-normal conditions for both the FWI and ISI for a year with elevated fire activity, supporting the case of their usefulness for providing fire danger forecasts under operational usage. Lastly, according to the reliability diagrams, the ISI predictions for 1-month lead time experiments are classified as perfectly reliable, while 2-month lead time experiments fall in the marginally useful+ category (Figs. S4–S5).
3.2 FWI and ISI predictions against fire occurrence
In this section, the focus will only be on the FWI and ISI as these were found to perform better with respect to their ROCSSs and respective reliability. The qualitative evaluation of above-normal fire danger conditions against historical fire occurrence was thus implemented for the FWI and ISI subcomponent, for both lead times and only for the spin-up experiments with the highest discrimination scores, as discussed in the previous section.
In order to decide which fire occurrence aspect should be considered, the correlation between FWI and ISI hindcasts with burnt areas and the number of fires for the years 2000–2016 was calculated and revealed moderate correlation between the FWI and ISI with the number of fires (r=0.55 and 0.45, respectively; p value <0.05) and no statistically significant correlation with burnt areas. Similar results were reported in a recent study of Galizia et al. (2021), suggesting that fire-prone pyro-regions, with Greece and Attica categorized as such, present moderate (>0.4) and strong (>0.6) positive correlations of the number of fires with the FWI and ISI, respectively. Thus, the number of fires instead of burnt area was eventually favoured as the variable of choice for the qualitative evaluation of fire danger hindcasts.
Figure 7 depicts the number of fires (with burnt area greater than 1 ha) per year, for the years between 2000 and 2016 of the hindcast period and the respective proportion of ensemble members predicting above-normal FWI and ISI values as obtained by the tercile plots averaged over the entire Attica domain (Figs. 3 and 6). Concerning both the FWI and ISI, the prediction of years with increased fire activity (i.e. the years with total number of fires greater than the 2000–2016 median based on the fire records) was clearly dependent on the lead time of the forecasts. It should be reiterated that only the years with observed (based on ERA5-Land) fire danger in the upper-tercile category (above-normal conditions) were taken into account. This includes also the 2003, 2009 and 2010 high-fire-activity years which according to the ERA5-Land observations fall in the middle (2003, 2009) and lower (2010) terciles.
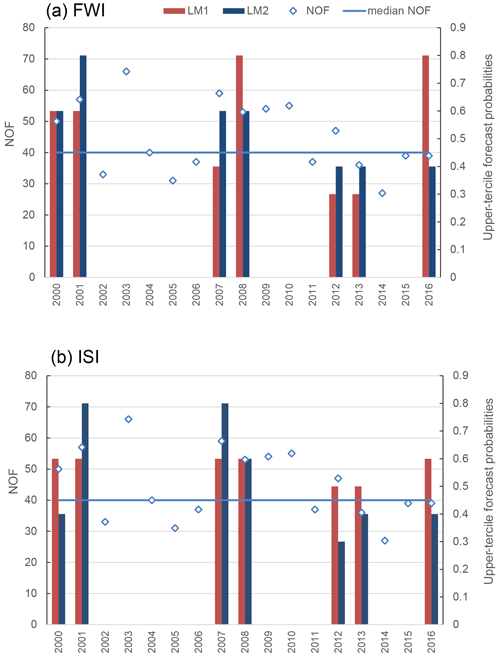
Figure 7Annual number of fires (NOF) in Attica (blue diamonds), median of fire events for 2000–2016 (blue line), and upper-tercile forecast probabilities of the (a) FWI and (b) ISI for the best-performing 1-month (red columns; LM1) and 2-month (blue columns; LM2) lead time spin-up experiments.
As seen in Fig. 7, half of the remaining years with increased fire activity are indeed captured by more than 60 % of the ensemble members by at least one of the 1-month or 2-month lead time FWI forecasts. The high fire activity of 2007 is captured only by 2-month lead time experiment, while 2012 is missed by both lead time experiments. Moreover, 2016 is overshot by the 1-month lead time experiment. Regarding the ISI, more than half of the years are captured with the percentage of ensemble members varying between 50 % and 80 % by at least one of the lead time experiments. Lastly, the high fire activity of the 2000 and 2012 fire seasons is not captured by 2-month lead time forecasts.
4.1 Prediction skill of single meteorological variables
Before delving into the indicators more directly associated with fire danger, the individual meteorological variables that serve as input to the FWI system were examined. As discussed in Sect. 3.1, it appears that relative humidity and wind speed exhibit high discrimination skills and temperature exhibits low skill, while precipitation showed no skill for both lead time experiments. For all meteorological variables, the forecast performance declines as the forecast lead time (i.e. the period between the target fire season and the initialization date of the forecast) increases, which is in line with previous studies (e.g. Doblas-Reyes et al., 2013; van den Hurk et al., 2012).
Looking at the individual variables, according to Mishra et al. (2019), limited predictive skill of seasonal temperature and very low skill of seasonal precipitation was found over all of Europe based on the EUROSIP (European Multimodel Seasonal to Interannual Prediction) multi-model framework, including the ECMWF System 4, the predecessor of SEAS5. Regarding the area under study, it is part of the Mediterranean region which is considered an area of transition between subtropical and mid-latitudes, where seasonal forecasts are challenging; therefore, the assessment of the added value and the identification of limitations of seasonal forecast products are of paramount importance when developing climate services (Calì Quaglia et al., 2022). The same study found statistically significant temperature anomaly correlations over the eastern Mediterranean between the SEAS5 and the ERA5 reference dataset; however, summer ROC skill score was not discussed in that study. Additionally, summer precipitation showed limited skill, located mainly at the western part of the Mediterranean. In general, the climate of the western Mediterranean is more predictable than the eastern part of the domain, probably due to the influence of El Niño–Southern Oscillation (ENSO) and North Atlantic Oscillation (NAO) teleconnections (Calì Quaglia et al., 2022; Frías et al., 2010). Concerning relative humidity, our results are in line with previous studies (Bedia et al., 2018; Bett et al., 2018) that found significant skill over the eastern Mediterranean using the ECMWF System 4 forecasting system. Finally, wind speed can be considered a promising variable regarding skill, as it is more closely related to the larger-scale atmospheric circulation than more complex processes like precipitation. According to Bett et al. (2022), the wind skill was found to be patchy throughout Europe especially during summer however using the System 4 forecasting system. The high wind skill for Attica empowers the discussion of the next section as the FWI is highly sensitive to wind speed (Karali et al., 2014). In addition, Kassomenos (2010) found that the Etesians (dry north winds prevailing during summer) are very often associated with the development of extreme wildfires in Greece, while Paschalidou and Kassomenos (2016) pointed out that mesoscale and local systems can play an important role on fire development, as they interact with and may exacerbate the larger-scale circulation patterns.
4.2 Impact of lead time and spin-up on fire danger forecast performance
Concerning the performance of the FWI and its subcomponents, according to the results presented in Sect. 3.1, it appears that the lead time of the forecast highly affects their skill scores and especially those of the FWI and ISI which attained the highest ROCSSs and are therefore discussed here. As far as the ISI is concerned, the highest ROCSSs were found for the 1-month lead time forecasts and can be attributed to the high performance of wind speed for this exact lead time experiment. ISI ROCSSs (Fig. 5) also indicate that the specific subcomponent is insensitive to spin-up as it remains unaffected between experiments and its performance is mostly controlled by the skill of the meteorological variables used for its calculations for the different lead time forecasts. This can be attributed to the fact that the ISI is calculated by solely combining wind speed with the FFMC, the latter having a fast response (less than 1 d) to weather variations as presented in Sect. 2.1.
Concerning the FWI, its high complexity and the non-linear relationships between its input meteorological variables and subcomponents make it difficult to attribute its performance to a single variable and/or subcomponent. According to the results, the FWI performs better in 2-month lead time experiments (even in the no spin-up experiment), even though the forecast performance of the single variables as discussed in Sect. 4.1 is decreased. A potential reason could be the higher scores of the DC and, therefore, of the BUI subcomponent (Fig. S6), compared to 1-month lead, which may be attributed to the memory of the DC subcomponent associated with soil moisture. The improved BUI of a 2-month lead time (Fig. S6), combined with the relatively high ISI (Fig. 5) skill scores, lead to a high level of FWI performance. The spin-up impact on the FWI, which can be seen in Fig. 3, is positive as higher discrimination scores were achieved in both 1-month and 2-month lead time experiments, without however altering the reliability class. From the terciles plots, it is also evident that although spin-up alters the discrimination skill of FWI forecasts, the choice between the model or observations in the spin-up procedure plays a minor role.
In summary, depending on the lead time of the forecasts, both the FWI and ISI were found to be useful tools in decision-making for the region under study as the scores imply. As several subcomponents of the FWI system (such as the ISI, BUI and FFMC) can be used by fire management authorities (Wotton, 2009), further research could be directed to utilizing multi-model ensembles in order to study potential improvements in the scores of the FWI and these subcomponents.
4.3 Qualitative evaluation of fire danger forecasts to predict fire occurrence based on fire statistics
The qualitative evaluation of the best-performing forecast experiments of the ISI and FWI has been carried out against fire occurrence data presented in Sect. 3.2. Both FWI and ISI forecasts managed to capture high-fire-activity years adequately (for the period 2000–2016), while the forecast probabilities were found to be highly dependent on the lead time. For half of the high-fire-activity years, both indices managed to capture high-fire-activity fire seasons with forecast probabilities greater than 0.6 (>60 % of the ensemble members for both lead time experiments). This implies that at least for high-fire-activity seasons, the seasonal approach for these two indices can be useful for complementing current fire management tools.
Regarding the misses discussed in Sect. 3.2, the reason is twofold. On one hand it should be considered that fire activity is not only driven by climate but also by interactions among climate, vegetation and human activities (Galizia et al., 2021). Thus, a climate-only approach, as proposed here, may prove insufficient for certain years. Disasters such as forest fires arise from a complex interplay between hazard, vulnerability and exposure (GIZ and EURAC, 2017; IPCC, 2022). The integration of seasonal forecast information (i.e. constituting the hazard component of risk) with other types of information, describing the natural and human capital as well as the vulnerability of the exposed system (Bacciu et al., 2021), is critical in order to enhance planning and decision-making regarding fire prevention and preparedness. On the other hand, the misses highlight the sensitivity of the results to the ERA5-Land dataset which was used to statistically downscale and evaluate the seasonal forecasts output (Herrera et al., 2019; Mavromatis and Voulanas, 2021). Therefore, further research is needed to investigate the impact of the selected reference dataset on the statistically downscaled forecasts.
As climate plays an important role in fire dynamics and climate change is increasing the frequency and severity of fire weather, the ability to forecast fire danger conditions prior to the beginning of the fire season can enhance preparedness and support decision-making in fire management for fire-prone areas. Moreover, the resilience of the forestry sector may be enhanced by developing dedicated climate services, such as fire danger seasonal forecasts, in order to reduce risks and offer opportunities for long-term reduction in wildfire disasters. The aim of this study is to provide high-resolution probabilistic seasonal fire danger forecasts, utilizing the Canadian Forest Fire Weather Index (FWI) for Attica, Greece, and verify these forecasts using probabilistic verification measures for skill assessment (ROC skill score, reliability diagrams). The ultimate goal is to explore whether these forecasts can support disaster management and relevant regional authorities by incorporating such fire risk assessment indicators in prevention and preparedness plans (Oom et al., 2022). The analysis focuses on the predictability of above-normal (upper-tercile) FWI years which have been associated in several studies with increased fire occurrence. Moreover, the study tried to assess the ability of fire danger forecasts to capture years with increased fire activity, by comparing hindcast years of above-normal fire danger conditions with historical fire occurrence data obtained by the Hellenic Fire Service. Our results suggest that depending on the lead time of the forecast, both the FWI and ISI present statistically significant high discrimination scores and can be considered reliable in predicting above-normal fire danger conditions. Therefore, they can be viewed as valuable climate-related alarms of increased fire danger and fire occurrence and may be further exploited by regional authorities in fire management regarding prevention, preparedness and resources allocation in the Attica region and other fire-prone regions and sub-regions in the Mediterranean.
Future work should focus on the assessment of large ensemble approaches utilizing different forecasting systems available in the Copernicus CDS, as well as alternative pathways to enhance the skill of seasonal fire danger predictions to be applicable to the whole Greek or even Mediterranean-wide domain. Finally, the impact of the selected reference dataset, here ERA5-Land, on the statistically downscaled forecasts should also be explored.
The post-processed datasets generated during the current study can be made available by the corresponding author on reasonable request.
The supplement related to this article is available online at: https://doi.org/10.5194/nhess-23-429-2023-supplement.
AK and KVV conceptualized and developed the methodology. AK and KVV performed the formal analysis. AK, KVV and MH wrote the original manuscript draft. CG acquired resources and funding. AK, KVV, MH, CG and PPN reviewed and edited the final manuscript.
The contact author has declared that none of the authors has any competing interests.
Publisher’s note: Copernicus Publications remains neutral with regard to jurisdictional claims in published maps and institutional affiliations.
The authors would like to thank the Copernicus Climate Change Service for making freely available the SEAS5 seasonal forecast and ERA5-Land datasets.
The authors would like to acknowledge funding from C3S European Tourism as well as the H2020 FIRE-RES projects.
This research has been supported by C3S European Tourism (contract no. C3S_422_Lot2_TEC) and the European Union's Horizon 2020 research and innovation project FIRE-RES (grant no. 101037419).
This paper was edited by Ricardo Trigo and reviewed by two anonymous referees.
Abatzoglou, J. T., Williams, A. P., Boschetti, L., Zubkova, M., and Kolden, C. A.: Global patterns of interannual climate–fire relationships, Glob. Change Biol., 24, 5164–5175, https://doi.org/10.1111/gcb.14405, 2018.
Alcasena, F. J., Ager, A. A., Bailey, J. D., Pineda, N., and Vega-García, C.: Towards a comprehensive wildfire management strategy for Mediterranean areas: Framework development and implementation in Catalonia, Spain, J. Environ. Manage., 231, 303–320, https://doi.org/10.1016/j.jenvman.2018.10.027, 2019.
Bacciu, V., Hatzaki, M., Karali, A., Cauchy, A., Giannakopoulos, C., Spano, D., and Briche, E.: Investigating the Climate-Related Risk of Forest Fires for Mediterranean Islands' Blue Economy, Sustainability, 13, 10004, https://doi.org/10.3390/su131810004, 2021.
Bedia, J., Herrera, S., Gutiérrez, J. M., Zavala, G., Urbieta, I. R., and Moreno, J. M.: Sensitivity of fire weather index to different reanalysis products in the Iberian Peninsula, Nat. Hazards Earth Syst. Sci., 12, 699–708, https://doi.org/10.5194/nhess-12-699-2012, 2012.
Bedia, J., Herrera, S., Gutiérrez, J. M., Benali, A., Brands, S., Mota, B., and Moreno, J. M.: Global patterns in the sensitivity of burned area to fire-weather: Implications for climate change, Agr. Forest Meteorol., 214–215, 369–379, https://doi.org/10.1016/j.agrformet.2015.09.002, 2015.
Bedia, J., Golding, N., Casanueva, A., Iturbide, M., Buontempo, C., and Gutiérrez, J. M.: Seasonal predictions of Fire Weather Index: Paving the way for their operational applicability in Mediterranean Europe, Climate Services, 9, 101–110, https://doi.org/10.1016/j.cliser.2017.04.001, 2018.
Bett, P., Thornton, H., and Troccoli, A.: Skill assessment of energy-relevant climate variables in a selection of seasonal forecast models. Report using final data sets, Met Office, 58pp., https://doi.org/10.5281/zenodo.1293863, 2018.
Bett, P. E., Thornton, H. E., Troccoli, A., De Felice, M., Suckling, E., Dubus, L., Saint-Drenan, Y.-M., and Brayshaw, D. J.: A simplified seasonal forecasting strategy, applied to wind and solar power in Europe, Climate Services, 27, 100318, https://doi.org/10.1016/j.cliser.2022.100318, 2022.
Calì Quaglia, F., Terzago, S., and von Hardenberg, J.: Temperature and precipitation seasonal forecasts over the Mediterranean region: added value compared to simple forecasting methods, Clim. Dynam., 58, 2167–2191, https://doi.org/10.1007/s00382-021-05895-6, 2022.
Copernicus Climate Change Service: Seasonal forecast daily and subdaily data on single levels, ECMWF SEAS5, Copernicus Climate Change Service (C3S) Climate Data Store (CDS) [data set], https://doi.org/10.24381/cds.181d637e, 2018.
Costa, H., Rigo, D., Libertà, G., Houston Durrant, T., and San-Miguel-Ayanz, J.: European wildfire danger and vulnerability in a changing climate: towards integrating risk dimensions, Technical report by the Joint Research Centre: JRC PESETA IV project: Task 9 forest fires, Publications Office of the European Union, Luxembourg, https://doi.org/10.2760/46951, 2020.
Costa-Saura, J., Mereu, V., Santini, M., Trabucco, A., Spano, D., and Bacciu, V.: Performances of climatic indicators from seasonal forecasts for ecosystem management: The case of Central Europe and the Mediterranean, Agr. Forest Meteorol., 319, 108921, https://doi.org/10.1016/j.agrformet.2022.108921, 2022.
Dee, D. P., Uppala, S. M., Simmons, A. J., Berrisford, P., Poli, P., Kobayashi, S., Andrae, U., Balmaseda, M. A., Balsamo, G., Bauer, P., Bechtold, P., Beljaars, A. C. M., van de Berg, L., Bidlot, J., Bormann, N., Delsol, C., Dragani, R., Fuentes, M., Geer, A. J., Haimberger, L., Healy, S. B., Hersbach, H., Hólm, E. V., Isaksen, L., Kållberg, P., Köhler, M., Matricardi, M., McNally, A. P., Monge-Sanz, B. M., Morcrette, J.-J., Park, B.-K., Peubey, C., de Rosnay, P., Tavolato, C., Thépaut, J.-N., and Vitart, F.: The ERA-Interim reanalysis: configuration and performance of the data assimilation system, Q. J. Roy. Meteor. Soc., 137, 553–597, https://doi.org/10.1002/qj.828, 2011.
de Groot, W. J.: Interpreting the Canadian forest fire weather index (FWI) system, in: Proceedings of the Fourth Central Regional Fire Weather Committee Scientific and Technical Seminar; Canadian Forest Service, Edmonton, Alberta, Canada, 3–14 pp., 1987.
Doblas-Reyes, F. J., García-Serrano, J., Lienert, F., Biescas, A. P., and Rodrigues, L. R. L.: Seasonal climate predictability and forecasting: status and prospects, WIREs Clim. Change, 4, 245–268, https://doi.org/10.1002/wcc.217, 2013.
Dupuy, J., Fargeon, H., Martin-StPaul, N., Pimont, F., Ruffault, J., Guijarro, M., Hernando, C., Madrigal, J., and Fernandes, P.: Climate change impact on future wildfire danger and activity in southern Europe: a review, Ann. Forest Sci., 77, 35, https://doi.org/10.1007/s13595-020-00933-5, 2020.
European Commission: Communication from the Commission to the European Parliament, the Council, the European Economic and Social Committee and the Committee of the Regions-New EU Forest Strategy for 2030, European Commission, COM(2021) 572 final, https://eur-lex.europa.eu/legal-content/EN/TXT/?uri=CELEX%3A52021DC0572 (last access: 24 January 2023), 2021.
Evelpidou, N., Tzouxanioti, M., Gavalas, T., Spyrou, E., Saitis, G., Petropoulos, A., and Karkani, A.: Assessment of Fire Effects on Surface Runoff Erosion Susceptibility: The Case of the Summer 2021 Forest Fires in Greece, Land, 11, 21, https://doi.org/10.3390/land11010021, 2022.
FAO and Plan Bleu: State of Mediterranean Forests 2018, FAO & Plan Bleu, Rome, Italy, 308 pp., 2018.
Fernandes, P. M.: Fire-smart management of forest landscapes in the Mediterranean basin under global change, Landscape Urban Plan., 110, 175–182, https://doi.org/10.1016/j.landurbplan.2012.10.014, 2013.
Field, R. D., Spessa, A. C., Aziz, N. A., Camia, A., Cantin, A., Carr, R., de Groot, W. J., Dowdy, A. J., Flannigan, M. D., Manomaiphiboon, K., Pappenberger, F., Tanpipat, V., and Wang, X.: Development of a Global Fire Weather Database, Nat. Hazards Earth Syst. Sci., 15, 1407–1423, https://doi.org/10.5194/nhess-15-1407-2015, 2015.
Frías, M. D., Herrera, S., Cofiño, A. S., and Gutiérrez, J. M.: Assessing the Skill of Precipitation and Temperature Seasonal Forecasts in Spain: Windows of Opportunity Related to ENSO Events, J. Climate, 23, 209–220, https://doi.org/10.1175/2009JCLI2824.1, 2010.
Frías, M. D., Iturbide, M., Manzanas, R., Bedia, J., Fernández, J., Herrera, S., Cofiño, A. S., and Gutiérrez, J. M.: An R package to visualize and communicate uncertainty in seasonal climate prediction, Environ. Modell. Softw., 99, 101–110, https://doi.org/10.1016/j.envsoft.2017.09.008, 2018.
Galizia, L. F., Curt, T., Barbero, R., Rodrigues, M., Galizia, L. F., Curt, T., Barbero, R., and Rodrigues, M.: Understanding fire regimes in Europe, Int. J. Wildland Fire, 31, 56–66, https://doi.org/10.1071/WF21081, 2021.
GIZ and EURAC: Risk Supplement to the Vulnerability Sourcebook. Guidance on how to apply the Vulnerability Sourcebook's approach with the new IPCC AR5 concept of climate risk, GIZ, Bonn, 64 pp., https://www.adaptationcommunity.net/wp-content/uploads/2017/10/GIZ-2017_Risk-Supplement-to-the-Vulnerability-Sourcebook.pdf (last access: 24 January 2023), 2017.
Herrera, S., Bedia, J., Gutiérrez, J. M., Fernández, J., and Moreno, J. M.: On the projection of future fire danger conditions with various instantaneous/mean-daily data sources, Climatic Change, 118, 827–840, https://doi.org/10.1007/s10584-012-0667-2, 2013.
Herrera, S., Kotlarski, S., Soares, P. M. M., Cardoso, R. M., Jaczewski, A., Gutiérrez, J. M., and Maraun, D.: Uncertainty in gridded precipitation products: Influence of station density, interpolation method and grid resolution, Int. J. Climatol., 39, 3717–3729, https://doi.org/10.1002/joc.5878, 2019.
IPCC: Climate Change 2022: Impacts, Adaptation and Vulnerability. Contribution of Working Group II to the Sixth Assessment Report of the Intergovernmental Panel on Climate Change, edited by: Pörtner, H.-O., Roberts, D. C., Tignor, M., Poloczanska, E. S., Mintenbeck, K. Alegría, A., Craig, M., Langsdorf, S., Löschke, S., Möller, V., Okem, A., and Rama, B., Cambridge University Press. Cambridge University Press, Cambridge, UK and New York, NY, USA, 3056 pp., 2022.
Jiménez-Ruano, A., Rodrigues Mimbrero, M., and de la Riva Fernández, J.: Exploring spatial–temporal dynamics of fire regime features in mainland Spain, Nat. Hazards Earth Syst. Sci., 17, 1697–1711, https://doi.org/10.5194/nhess-17-1697-2017, 2017.
Johnson, S. J., Stockdale, T. N., Ferranti, L., Balmaseda, M. A., Molteni, F., Magnusson, L., Tietsche, S., Decremer, D., Weisheimer, A., Balsamo, G., Keeley, S. P. E., Mogensen, K., Zuo, H., and Monge-Sanz, B. M.: SEAS5: the new ECMWF seasonal forecast system, Geosci. Model Dev., 12, 1087–1117, https://doi.org/10.5194/gmd-12-1087-2019, 2019.
Jolliffe, I. T. and Stephenson, D. B.: Forecast Verification: A Practitioner's Guide in Atmospheric Science, John Wiley & Son, 240 pp., ISBN 0-471-49759-2, 2003.
Karali, A., Hatzaki, M., Giannakopoulos, C., Roussos, A., Xanthopoulos, G., and Tenentes, V.: Sensitivity and evaluation of current fire risk and future projections due to climate change: the case study of Greece, Nat. Hazards Earth Syst. Sci., 14, 143–153, https://doi.org/10.5194/nhess-14-143-2014, 2014.
Kassomenos, P.: Synoptic circulation control on wild fire occurrence, Phys. Chem. Earth Parts A/B/C, 35, 544–552, https://doi.org/10.1016/j.pce.2009.11.008, 2010.
Lorenz, C., Portele, T. C., Laux, P., and Kunstmann, H.: Bias-corrected and spatially disaggregated seasonal forecasts: a long-term reference forecast product for the water sector in semi-arid regions, Earth Syst. Sci. Data, 13, 2701–2722, https://doi.org/10.5194/essd-13-2701-2021, 2021.
Manzanas, R.: Assessment of Model Drifts in Seasonal Forecasting: Sensitivity to Ensemble Size and Implications for Bias Correction, J. Adv. Model. Earth Sy., 12, e2019MS001751, https://doi.org/10.1029/2019MS001751, 2020.
Manzanas, R., Frías, M. D., Cofiño, A. S., and Gutiérrez, J. M.: Validation of 40 year multimodel seasonal precipitation forecasts: The role of ENSO on the global skill, J. Geophys. Res.-Atmos., 119, 1708–1719, https://doi.org/10.1002/2013JD020680, 2014.
Manzanas, R., Lucero, A., Weisheimer, A., and Gutiérrez, J. M.: Can bias correction and statistical downscaling methods improve the skill of seasonal precipitation forecasts?, Clim. Dynam., 50, 1161–1176, https://doi.org/10.1007/s00382-017-3668-z, 2018.
Manzanas, R., Gutiérrez, J. M., Bhend, J., Hemri, S., Doblas-Reyes, F. J., Torralba, V., Penabad, E., and Brookshaw, A.: Bias adjustment and ensemble recalibration methods for seasonal forecasting: a comprehensive intercomparison using the C3S dataset, Clim. Dynam., 53, 1287–1305, https://doi.org/10.1007/s00382-019-04640-4, 2019.
Marcos, R., Llasat, M. C., Quintana-Seguí, P., and Turco, M.: Use of bias correction techniques to improve seasonal forecasts for reservoirs — A case-study in northwestern Mediterranean, Sci. Total Environ., 610–611, 64–74, https://doi.org/10.1016/j.scitotenv.2017.08.010, 2018.
Mavromatis, T. and Voulanas, D.: Evaluating ERA-Interim, Agri4Cast, and E-OBS gridded products in reproducing spatiotemporal characteristics of precipitation and drought over a data poor region: The Case of Greece, Int. J. Climatol., 41, 2118–2136, https://doi.org/10.1002/joc.6950, 2021.
MedECC: Climate and Environmental Change in the Mediterranean Basin – Current Situation and Risks for the Future, First Mediterranean Assessment Report, edited by: Cramer, W., Guiot, J., and Marini, K., Union for the Mediterranean, Plan Bleu, UNEP/MAP, Marseille, France, 632 pp., https://doi.org/10.5281/zenodo.4768833, ISBN: 978-2-9577416-0-1, 2020.
Mercado-Bettín, D., Clayer, F., Shikhani, M., Moore, T. N., Frías, M. D., Jackson-Blake, L., Sample, J., Iturbide, M., Herrera, S., French, A. S., Norling, M. D., Rinke, K., and Marcé, R.: Forecasting water temperature in lakes and reservoirs using seasonal climate prediction, Water Res., 201, 117286, https://doi.org/10.1016/j.watres.2021.117286, 2021.
MeteoSwiss, Bhend, J., Ripoldi, J., Mignani, C., Mahlstein, I., Hiller, R., Spirig, C., Liniger, M., Weigel, A., Jimenez, J. B., Felice, M. D., Siegert, S., and Sedlmeier, K.: easyVerification: Ensemble Forecast Verification for Large Data Sets, CRAN [code], https://cran.r-project.org/web/packages/easyVerification/index.html (last access: 24 January 2023), 2017.
Mishra, N., Prodhomme, C., and Guemas, V.: Multi-model skill assessment of seasonal temperature and precipitation forecasts over Europe, Clim. Dynam., 52, 4207–4225, https://doi.org/10.1007/s00382-018-4404-z, 2019.
Mitsopoulos, I., Mallinis, G., Dimitrakopoulos, A., Xanthopoulos, G., Eftychidis, G., and Goldammer, J. G.: Vulnerability of peri-urban and residential areas to landscape fires in Greece: Evidence by wildland-urban interface data, Data in Brief, 31, 106025, https://doi.org/10.1016/j.dib.2020.106025, 2020.
Moreira, F., Ascoli, D., Safford, H., Adams, M. A., Moreno, J. M., Pereira, J. M. C., Catry, F. X., Armesto, J., Bond, W., González, M. E., Curt, T., Koutsias, N., McCaw, L., Price, O., Pausas, J. G., Rigolot, E., Stephens, S., Tavsanoglu, C., Vallejo, V. R., Wilgen, B. W. V., Xanthopoulos, G., and Fernandes, P. M.: Wildfire management in Mediterranean-type regions: paradigm change needed, Environ. Res. Lett., 15, 011001, https://doi.org/10.1088/1748-9326/ab541e, 2020.
Muñoz Sabater, J.: ERA5-Land hourly data from 1981 to present, Copernicus Climate Change Service (C3S) Climate Data Store (CDS) [dataset], https://doi.org/10.24381/cds.e2161bac, 2019.
Muñoz-Sabater, J., Dutra, E., Agustí-Panareda, A., Albergel, C., Arduini, G., Balsamo, G., Boussetta, S., Choulga, M., Harrigan, S., Hersbach, H., Martens, B., Miralles, D. G., Piles, M., Rodríguez-Fernández, N. J., Zsoter, E., Buontempo, C., and Thépaut, J.-N.: ERA5-Land: a state-of-the-art global reanalysis dataset for land applications, Earth Syst. Sci. Data, 13, 4349–4383, https://doi.org/10.5194/essd-13-4349-2021, 2021.
Oom, D., de Rigo, D., Pfeiffer, H., et al.: Pan-European wildfire risk assessment, Publications Office of the European Union, Luxembourg, EUR 31160 EN, https://doi.org/10.2760/9429, ISBN 978-92-76-55137-9, 2022.
Papagiannaki, K., Giannaros, T. M., Lykoudis, S., Kotroni, V., and Lagouvardos, K.: Weather-related thresholds for wildfire danger in a Mediterranean region: The case of Greece, Agr. Forest Meteorol., 291, 108076, https://doi.org/10.1016/j.agrformet.2020.108076, 2020.
Paschalidou, A. K. and Kassomenos, P. A.: What are the most fire-dangerous atmospheric circulations in the Eastern-Mediterranean? Analysis of the synoptic wildfire climatology, Sci. Total Environ., 539, 536–545, https://doi.org/10.1016/j.scitotenv.2015.09.039, 2016.
Rogers, B. M., Balch, J. K., Goetz, S. J., Lehmann, C. E. R., and Turetsky, M.: Focus on changing fire regimes: interactions with climate, ecosystems, and society, Environ. Res. Lett., 15, 030201, https://doi.org/10.1088/1748-9326/ab6d3a, 2020.
Ruffault, J., Curt, T., Moron, V., Trigo, R. M., Mouillot, F., Koutsias, N., Pimont, F., Martin-StPaul, N., Barbero, R., Dupuy, J.-L., Russo, A., and Belhadj-Khedher, C.: Increased likelihood of heat-induced large wildfires in the Mediterranean Basin, Sci. Rep., 10, 13790, https://doi.org/10.1038/s41598-020-70069-z, 2020.
Salvati, L. and Ranalli, F.: `Land of Fires': Urban Growth, Economic Crisis, and Forest Fires in Attica, Greece, Geogr. Res., 53, 68–80, https://doi.org/10.1111/1745-5871.12093, 2015.
San-Miguel-Ayanz, J., Schulte, E., Schmuck, G., Camia, A., Strobl, P., Libertà, G., Giovando, C., Boca, R., Sedano, F., Kempeneers, P., McInerney, D., Withmore, C., de Oliveira, S. S., Rodrigues, M., Houston Durrant, T., Corti, P., Oehler, F., Vilar, L., and Amatulli, G.: Comprehensive monitoring of wildfires in Europe: The European Forest Fire Information System (EFFIS), in: Approaches to Managing Disaster - Assessing Hazards, Emergencies and Disaster Impacts, edited by: Tiefenbacher, J., IntechOpen, 87–108, https://doi.org/10.5772/28441, 2012.
Siegert, S., Bhend, J., Kroener, I., and Felice, M. D.: SpecsVerification: Forecast Verification Routines for Ensemble Forecasts of Weather and Climate, CRAN [code], https://cran.r-project.org/web/packages/SpecsVerification/index.html (last access: 24 January 2023), 2020.
Stocks, B. J., Lynham, T. J., Lawson, B. D., Alexander, M. E., Wagner, C. E. V., McAlpine, R. S., and Dubé, D. E.: The Canadian Forest Fire Danger Rating System: An Overview, Forest. Chron., 65, 450–457, https://doi.org/10.5558/tfc65450-6, 1989.
Turco, M., Llasat, M. C., Tudela, A., Castro, X., and Provenzale, A.: Brief communication Decreasing fires in a Mediterranean region (1970–2010, NE Spain), Nat. Hazards Earth Syst. Sci., 13, 649–652, https://doi.org/10.5194/nhess-13-649-2013, 2013.
Turco, M., Rosa-Cánovas, J. J., Bedia, J., Jerez, S., Montávez, J. P., Llasat, M. C., and Provenzale, A.: Exacerbated fires in Mediterranean Europe due to anthropogenic warming projected with non-stationary climate-fire models, Nat. Commun., 9, 3821, https://doi.org/10.1038/s41467-018-06358-z, 2018.
Turco, M., Marcos-Matamoros, R., Castro, X., Canyameras, E., and Llasat, M. C.: Seasonal prediction of climate-driven fire risk for decision-making and operational applications in a Mediterranean region, Sci. Total Environ., 676, 577–583, https://doi.org/10.1016/j.scitotenv.2019.04.296, 2019.
United Nations Environment Programme: Spreading like Wildfire – The Rising Threat of Extraordinary Landscape Fires, A UNEP Rapid Response Assessment, Nairobi, 126 pp., https://wedocs.unep.org/handle/20.500.11822/38372 (24 January 2023), 2022.
Urbieta, I. R., Zavala, G., Bedia, J., Gutiérrez, J. M., Miguel-Ayanz, J. S., Camia, A., Keeley, J. E., and Moreno, J. M.: Fire activity as a function of fire–weather seasonal severity and antecedent climate across spatial scales in southern Europe and Pacific western USA, Environ. Res. Lett., 10, 114013, https://doi.org/10.1088/1748-9326/10/11/114013, 2015.
van den Hurk, B., Doblas-Reyes, F., Balsamo, G., Koster, R. D., Seneviratne, S. I., and Camargo, H.: Soil moisture effects on seasonal temperature and precipitation forecast scores in Europe, Clim. Dynam., 38, 349–362, https://doi.org/10.1007/s00382-010-0956-2, 2012.
van Wagner, C. E.: Development and structure of a Canadian forest fire weather index system, Canadian Forestry Service, Ottawa, Forestry Tech. Rep. 35, 35 pp., ISBN 0-662-15198-4, 1987.
Varotsos, K. V., Dandou, A., Papangelis, G., Roukounakis, N., Kitsara, G., Tombrou, M., and Giannakopoulos, C.: Using a new local high resolution daily gridded dataset for Attica to statistically downscale climate projections, Clim. Dynam., online first, https://doi.org/10.1007/s00382-022-06482-z, 2022.
Weisheimer, A. and Palmer, T. N.: On the reliability of seasonal climate forecasts, J. Roy. Soc. Interface, 11, 20131162, https://doi.org/10.1098/rsif.2013.1162, 2014.
WMO: Guidance on Operational Practices for Objective Seasonal Forecasting, WMO, Geneva, WMO-No. 1246, 91 pp., ISBN 978-92-63-11246-9, 2020.
Wotton, B. M.: Interpreting and using outputs from the Canadian Forest Fire Danger Rating System in research applications, Environ. Ecol. Stat., 16, 107–131, https://doi.org/10.1007/s10651-007-0084-2, 2009.