the Creative Commons Attribution 4.0 License.
the Creative Commons Attribution 4.0 License.
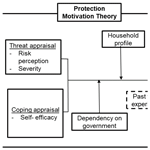
Identifying the drivers of private flood precautionary measures in Ho Chi Minh City, Vietnam
Thulasi Vishwanath Harish
Nivedita Sairam
Liang Emlyn Yang
Matthias Garschagen
Heidi Kreibich
Private flood precautionary measures have proven to reduce flood damage effectively. Integration of these measures into flood response systems can improve flood risk management in high-risk areas such as Ho Chi Minh City (HCMC). Since uptake of such measures is voluntary, it is important to know what drives householders to implement precautionary measures. In this study, we developed a framework representing the uptake of private precautionary measures based on protection motivation theory and the transtheoretical model. Using empirical survey data collected from 1000 flood-prone households in HCMC, we implemented lasso and elastic-net regression to identify the drivers of private precaution. The measures were classified into structural measures and non-structural measures based on whether structural changes to the building were required. The households were classified into proactive and reactive households based on whether their decision to reduce risk (i.e. uptake of precautionary measures) was preceded by experiencing a flood. The data-driven model revealed that the household's level of education, the degree of belief in the government to implement regional flood protection measures and the degree of belief that in case of flooding one has to deal with the consequences of flooding by themselves positively influence the proactive uptake of non-structural measures. Among the households that experienced flooding before implementing the measures, the uptake was found to be driven by the severity of the experienced damage. For the same group of households, perceiving a high severity of future flood impacts was found to negatively influence the uptake of structural flood precautionary measures. These results highlight that efforts to improve the implementation of private precautionary measures should consider the socio-economic characteristics of the members of the household, their past flood experience and their perception of flood risk management for communicating flood risk and incentivizing private precautionary measures.
- Article
(1518 KB) - Full-text XML
- BibTeX
- EndNote
Floods affect 54 million people and cause EUR 58 billion in damage globally every year (Alfieri et al., 2017). Flood damage is predicted to rise further due to socio-economic and climate change (Botzen et al., 2019a). Ho Chi Minh City (HCMC), Vietnam, is one of the cities most exposed to flood risk under current socio-economic conditions (Hallegatte et al., 2013). During the rainy season, a combination of high tide, heavy rains, and high flow volume in the Saigon and Dong Nai rivers results in regular flooding in several parts of Ho Chi Minh City (Woetzel et al., 2020). The flood risk is increasing due to an increasing trend of precipitation events due to climate change, ongoing urbanization, increasing population, and infrastructure density and land subsidence (Duffy et al., 2020; Khoi and Trang, 2016; Phi, 2007; Woetzel et al., 2020).
Reducing flood risk has become a necessity which has led to large investments by the government in extensive flood defence systems (Cao et al., 2021). Based on the design specifications, there is a possibility that conventional large-scale flood protection infrastructure may fail due to rising flood hazard levels. The growing city also poses a challenge to implementing regional measures as new settlements rapidly develop. Hence, a transition to integrated flood risk management strategies is imperative (Botzen et al., 2019a; Nguyen et al., 2021). This means that complementing large-scale protection structures with small-scale private precautionary measures is necessary (Du et al., 2020; Scussolini et al., 2017; Yang et al., 2018).
Private precautionary measures include building elevation, shielding with mobile barriers, waterproof sealing, fortification, flood-adapted use, flood-adapted interior fitting and safeguarding of hazardous substances (Chinh et al., 2016). Elevating and dry-proofing buildings in HCMC were found to reduce expected annual flood damage by 52 %–55 % and 82 %, respectively (Scussolini et al., 2017). Another study conducted in Shanghai by Du et al. (2020) reported a 69 % reduction in expected annual flood damage from wet-proofing. Despite evidence demonstrating the loss-reducing potential of private precautionary measures, their implementation is commonly voluntary, and hardly any official funding is provided (Barendrecht et al., 2020; Chinh et al., 2016; Garschagen, 2015). Past studies have indicated that households are often not willing to take up the responsibility of implementing property-level precautionary measures (Bamberg et al., 2017; Barendrecht et al., 2020). At the household level, certain indicators including education, income, household composition, occupation, social networks and place attachment were identified to influence protective actions (Ji et al., 2021; Okayo et al., 2015).
In order to bridge the knowledge gap in understanding the level of flood preparedness and uptake of private precautionary measures, several studies have applied protection motivation theory (PMT) to identify the drivers that motivate households to take up protective measures (Babcicky and Seebauer, 2019; Bubeck et al., 2018). In order to include a household's willingness to take up measures, the PMT was complemented with the transtheoretical model (TTM) (Weyrich et al., 2020). The TTM is a behavioural-change model which emerged from clinical psychology and represents decision stages which indicate an individual's degree of readiness to act upon danger to protect themselves from a risk (Bočkarjova et al., 2009).
In this study, we develop empirical, data-driven analysis based on the combined PMT–TTM framework to understand what drives households in HCMC, Vietnam, to take up private precautionary measures.
The paper is organized as follows: Sect. 2 explains the data and methods used, specifically, the empirical household survey data used in the study (Sect. 2.1), the PMT–TTM theoretical model (Sect. 2.2) and the statistical analysis (Sect. 2.3); Sect. 3 presents and discusses the results, including the prevalence and cost of the different measures (Sect. 3.1) and drivers of precautionary measures (Sect. 3.2); and Sect. 4 concludes the paper.
2.1 Household survey
The empirical data used in the study were obtained from a structured household survey in selected districts of HCMC during September–October 2020. A total of eight wards in four districts were surveyed, which include Binh Thanh, District 8, Binh Tan and Nha Be as presented in Fig. 1.
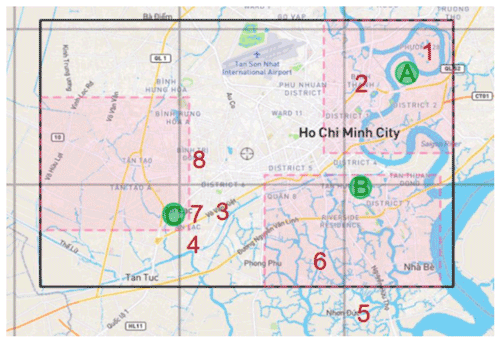
Figure 1Survey areas (n = 8) in Ho Chi Minh City. Red numbers are the sites of the main survey in 2020, and green letters are areas of the pre-test survey in December 2019 (Yang et al., 2020).
The survey collected 1000 valid responses from local households which suffered from floods in the last 10 years. The questions were drafted based on expert knowledge from flood risk researchers, social scientists and local stakeholders in HCMC. The survey areas (Binh Thanh, District 8, Binh Tan and Nha Be) were established in order to cover a broad range of socio-economic profiles and flood types such as tidal, fluvial, pluvial and compound flooding in the city. Within the survey areas, the households were chosen at random. A survey pre-test involving 60 households from three districts (Binh Tan, District 7 and District 2) was conducted in December 2019 in order to test the validity of the questionnaire. The questionnaire was revised based on the responses from the pre-test. The questionnaire covered aspects concerning two past flood events experienced by the households – the most recent and the most serious event in the last 10 years. The questions pertained to the hazard and damage suffered by the households, implementation of precautionary measures, early warning quality and lead time, household's risk perception, and socio-economic profile. In order to maintain consistency, this study uses responses only from the main survey.
2.2 Theoretical framework
The PMT–TTM (protection motivation theory–transtheoretical model) framework is used to conceptualize the cognitive processes driving the uptake of private precaution considering the different risk-reducing decision stages. PMT was first proposed by Rogers (1983) to explain the effect of fear appeals on health-related behaviour in health psychology. Gradually, its application was extended to research in natural and environmental hazards, such as droughts, earthquakes, volcanic hazards, tornadoes, wildfires and flood risks (Babcicky and Seebauer, 2019). PMT comprises two cognitive processes – threat appraisal and coping appraisal – which determine the changes in an individual's coping intentions. Threat appraisal is described as a person's assessment of a threat's damaging potential to valuables, assuming no personal change in behaviour; coping appraisal is described as the person's evaluation of their ability to cope with or avert the threat (Grothmann and Reusswig, 2006). In this study, threat appraisal is represented by how the households perceive current and potential future flood risk; coping appraisal is represented by how able the households feel about resisting the impacts of flooding. PMT is extended to include a household's socio-economic and building characteristics, past flood experiences, and perception of dependency on government protection measures.
The TTM focuses on an individual's decision-making and what changes the behaviour leading to changes in the decision-making stage. The conventionally ordered decision stages are the stages of pre-contemplation, contemplation and action (Block and Keller, 1998; Poussin et al., 2014). In the context of flood preparedness, a TTM represents the households' decision stages, i.e. degree of readiness to implement private precaution to protect themselves from flood impacts. Based on their characteristics, the households with protective behaviour may be categorized into proactive (voluntarily implementing risk reduction measures) and reactive (implementing risk reduction measures as a reaction to experiencing a serious flood event) with respect to specific measures. The combined PMT–TTM has the capability of identifying the factors that motivate households to take up private precaution and the factors that help in changing the decision stages of households (e.g. reactive to proactive households). The PMT–TTM (protection motivation theory–transtheoretical model) was first introduced by Block and Keller (1998). For instance, Weyrich et al. (2020) developed different risk reduction stages to focus on the quality of protective behaviour, while Bočkarjova et al. (2009) implemented intention stages to understand the risk-reducing behavioural intention.
In this study, the framework aims to identify drivers influencing the uptake of flood precautionary measures among households. In this context, we conceptualized two risk-reducing stages, namely, the proactive stage and reactive stage. Households in the proactive stage are those who voluntarily participated in risk reduction measures before experiencing a serious flood event since 2010, i.e. in the last 10 years from the date of the survey. On the other hand, households from the reactive stage undertake protective measures as a response to a serious flood event (Fig. 2). The corresponding question and choices from the household survey are first presented below. In addition to the timeline of implementation, the survey also collects data on the cost of implementing the measure. The corresponding question is similarly presented below.
-
Which of the following precautionary measures (Table 1) have you implemented, and when did you implement them?
-
Before the serious event in the last 10 years
-
Before the recent event
-
Before both events (serious and recent)
-
After both events
-
Did not implement
-
-
If you implemented the measure, how much did it cost to implement the measure? VND million
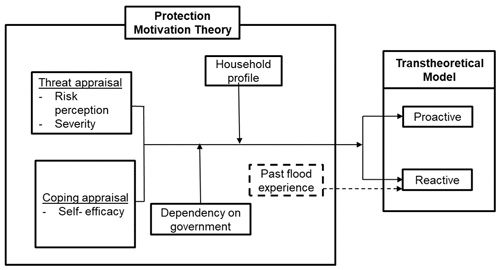
Figure 2Protection motivation theory and transtheoretical model (PMT–TTM) framework consisting of PMT and TTM blocks. Past flood experience is represented as dashed lines since it differentiates the reactive households from proactive households.
Table 1Categorization of private flood precautionary measures into structural and non-structural measures.
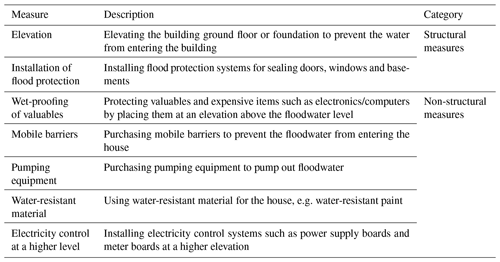
Table 2Household groups classified based on the type of implemented precautionary measure (structural and non-structural measures) and risk-reducing stages (proactive and reactive).

PMT includes six aspects: (1) risk perception, (2) severity, (3) self-efficacy, (4) household profile, (5) dependency on government and (6) past flood experiences (Fig. 2). The survey responses that represent these aspects and potentially influence the uptake of precautionary measures were selected (see Appendix A for the questionnaire). Additionally, since TTM classifies the household based on their risk-reducing stage, households belonged to the TTM groups (proactive and reactive) based on when the measure was implemented. In addition to when the measure was implemented, the implementation cost of each measure is also recorded during the household survey. Each precautionary measure is categorized into the structural or non-structural measures (Table 1). Structural measures require making permanent changes to the construction of the building, e.g. elevating or installing flood protection. These measures have the potential to be included in building codes especially for new construction. On the other hand, non-structural measures do not result in permanent changes to the building structure. The categorization into structural and non-structural measures helps to account for the permanence aspect of the measures in the study. For each precautionary measure category, if a household implemented any one of the measures (1) before the serious event or (3) before both events, the household is grouped into the proactive risk-reducing stage. If a household implemented any one of the measures (2) before the recent event or (4) after both the events, the household is grouped into the reactive risk-reducing stage. Therefore, a household that belongs to the proactive risk-reducing stage for the structural measure category can belong to the reactive risk-reducing stage with respect to the non-structural measure category. From these two levels of classification, risk-reducing stages and precautionary measure categories, four groups of households were formed as illustrated in Table 2.
2.3 Statistical analysis
In order to identify the drivers influencing the uptake of precautionary measures in each household group, responses from the questionnaire survey pertaining to the PMT–TTM framework (see Appendix A) are considered the explanatory variables and regressed against a binary indicator of the uptake of measures (i.e. response variable) (see Sect. 2.2). In this respect, lasso and elastic-net regression models are applied. Since the response variables follow a binomial distribution, a logit regression is implemented. Lasso regression determines the extent of influence by an explanatory variable on the response variable by imposing a λ times L1 penalty on the residual sum of squares to compute the lasso estimate as defined by Eq. (1) (Hastie et al., 2008):
where x represents the explanatory variables, y is the response variables, β0 is the intercept, β represents regression coefficients of explanatory variables, p is the number of input explanatory variables, and N is the number of observations or households interviewed (N = 1000). The L1 penalty is , while λ≥0 is a complexity parameter that controls the amount of regression coefficient shrinkage and is determined by cross-validation. The larger the value of λ is, the greater the shrinkage is (Hastie et al., 2008). Lasso regression performs variable selection while maintaining the stability by imposing a penalty on the size of regression coefficients (Tibshirani, 1996) and shrinking it towards zero when there is low correlation between the explanatory variable and response variable. The nature of this constraint tends to produce some coefficients that are exactly equal to zero and eliminates the explanatory variables corresponding to these coefficients (Tibshirani, 1996). However, when the number of explanatory variables (p) is greater than the number of observations (N), only N variables are selected before lasso saturates, and when a group of variables have high pairwise correlation, then lasso randomly selects one variable from the group. The naive elastic-net regression as illustrated in Eq. (2) overcame this limitation (Zou and Hastie, 2005).
where .
It possesses the characteristic of the L1 penalty term, (lasso regression), and the L2 penalty term, (ridge regression). It overcomes the limitation of lasso regression as the L1 lasso penalty term performs automatic variable selection, while the L2 ridge penalty encourages grouped selection by shrinking together the coefficients of correlated explanatory variables (Hastie et al., 2008). Hyperparameter α estimates the contribution of the L1 and L2 penalty by assigning a value between 0 and 1. However, Eq. (2) was unable to perform satisfactorily as its solution path incurred double shrinkage and did not produce an optimal variance–bias trade-off. Rescaling the naive elastic-net equation by (1+λ2) as shown in Eq. (3) automatically achieved optimality and is known as elastic-net regression (Zou and Hastie, 2005).
Cross-validation is applied to these models to prevent over-fitting. In this study, 10-fold cross-validation is implemented to the available dataset by partitioning 10 disjoint subsets of approximately equal size by randomly sampling data from the dataset without replacement. The model is trained using 9 subsets and validated with the remaining 1 subset. This procedure is repeated until each of the 10 subsets has served as a validation subset. The average of their performance metrics is the model performance. Thereafter, the deviance metric is used to measure the performance of the lasso and elastic-net regression models. Deviance measures the goodness of fit based on the difference in the likelihood between a fitted model and a saturated model (). The likelihood of a saturated model is 1, as the number of estimated parameters is equal to the number of data points. Deviance ranges from 0 to infinity, where a lower deviance value indicates the model has a better data fit. The formula of deviance (D) is as presented in Eq. (4).
The lasso and elastic-net regression models are applied to empirical data pertaining to the four household groups – structural–proactive (SP), non-structural–proactive (NSP), structural–reactive (SR) and non-structural–reactive (NSR). For each household group, the absolute variable coefficient values associated with the lowest deviance value are derived from lasso and elastic-net regression. Thereafter, the weighted median is computed from normalized variable coefficients where the reciprocal of deviance acts as weights. Since the explanatory variables used in the model correspond to the aspects of the PMT–TTM framework, the variable importance based on a weighted-median value greater than 0.5 is considered to drive the uptake of precautionary measures in the household groups.
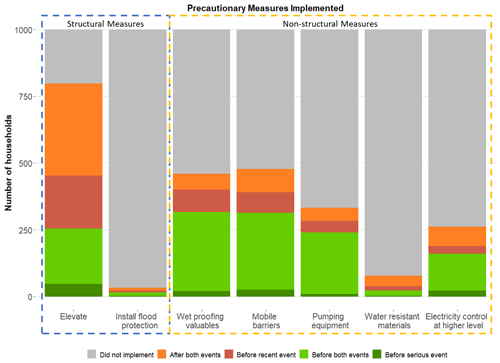
Figure 3Number of households that implemented structural and non-structural private precautionary measures with respect to the temporal precedence considering the most serious event in the last 10 years and the most recent event. The box formed by a yellow dashed line encloses non-structural measures, and the box formed by a blue dashed line encloses structural measures. The shades of green – “before both events” and “before serious event” – indicate proactive households; the shades of orange – “after both events” and “before recent event” – indicate reactive households.
3.1 Implementation of private precautionary measures
In this section, an overview of how many households have implemented specific precautionary measures and the cost of implementation of the measures are presented. The measure “elevation”, i.e. elevating the building ground floor, was implemented the most (Fig. 3), despite the average cost of elevating a building being VND 78 million, which is much higher than implementation costs of all other precautionary measures (Fig. 4). A total of 54.2 % of the households (n = 542) have reactively undertaken this measure (i.e. implementation after experiencing the serious event in the last 10 years and before the recent event or implementation after experiencing the serious and the recent flood event), and 25.5 % (n = 255) have adopted it proactively (i.e. implementation before experiencing the serious event in the last 10 years or implementation before experiencing both the serious and the recent events). The second most often implemented measure is purchasing “mobile barriers” which is closely followed by “wet-proofing of valuables” (i.e. protecting valuables and expensive contents by placing them at elevation above the floodwater level), with an implementation prevalence of 47.7 % (n = 477) and 46 % (n = 460), respectively. Furthermore, 33.3 % (n = 333) of the households have bought pumping equipment to pump out floodwater, and 26.2 % (n = 262) have installed electricity control at a higher level. Only 7.7 % (n = 77) of the households used water-resistant materials. The average cost of purchasing pumping equipment and mobile barriers was VND 3.2 million and 1.4 million, respectively. The average cost of wet-proofing valuables, installing electricity control at a higher level and installing flood protection systems was the lowest amount, totalling to VND 0.5 million, 0.6 million and 0.9 million, respectively. Despite the relatively low implementation cost of installing flood protection (sealing windows, doors), this measure has the lowest implementation prevalence of 3.3 % (n = 33).
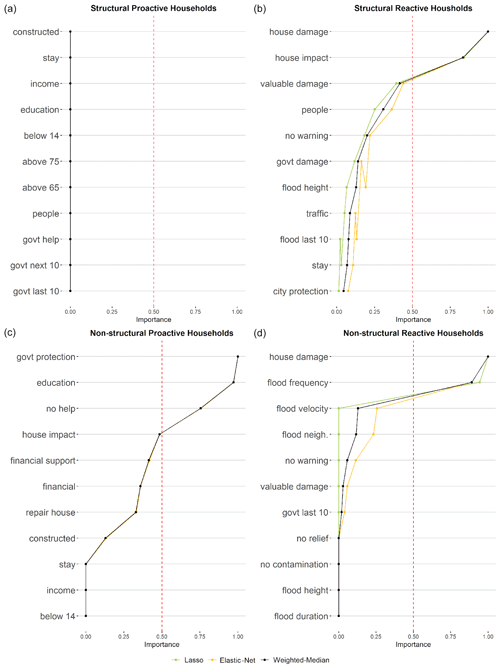
Figure 4Drivers of private precautionary measures for (a) structural–proactive households, (b) structural–reactive households, (c) non-structural–proactive households and (d) non-structural–reactive households (variables on the y axis correspond to variable names in Appendix A).
Among the precautionary measures, the elevation of the building has a special position. Despite the high cost of elevating the house, this measure, which prevents the floodwater from reaching the living area, is very popular in HCMC and helps residents to live with floods. The elevation process can be done to the entire building, or only a new elevated ground floor can be constructed within the building (FEMA, 2007; Garschagen, 2014). Hence, houses are often built elevated or are elevated during renovations, which is frequently done by households in HCMC. It might be decisive that building codes have prescribed a minimum elevation of buildings in Vietnam since 2008 (Garschagen, 2014) and discouraged the implementation of only wet-proofing measures. Most respondents have structurally elevated their houses after experiencing flood events (Fig. 3), which occur frequently, almost during every rainy season in HCMC.
3.2 Drivers of private precautionary measures
The most important drivers of private precaution for the different household groups are identified based on a list of potential influencing variables representing the aspects of the PMT–TTM framework (Appendix A).
Table 3Most important variables influencing implementation of precautionary measures (importance: 0 – no importance, 1 – high importance; coefficients: positive – encourages the uptake of measures, negative – discourages the uptake of measures). All the models resulted in a deviance of approximately 1.3.
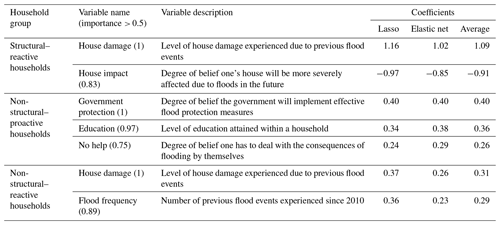
In the case of the structural–reactive household group, the variables “house damage” and “house impact” are identified as the most important influencing variables, with importance values of 1 and 0.83, respectively (Fig. 4b, Table 3). An average coefficient value of house damage computed from lasso and elastic-net regression is 1.09, which implies that experiencing high levels of damage in the past flood events increases the probability of the household adopting structural precautionary measures. On the other hand, the house impact variable with an average coefficient value of −0.91 (note the negative coefficient) indicates that households which strongly believe that their house will be more severely affected by flooding in the future are less likely to adopt structural precautionary measures. The house impact variable relates to the severity factor of threat appraisal (Appendix A). This is in accordance with results of several previous studies which have found that a perceived increase in severe flood damage in the future causes a sense of helplessness and incapacity to adapt further, thus discouraging the implementation of structural measures (Babcicky and Seebauer, 2019; Gebrehiwot and van der Veen, 2015; Grothmann and Reusswig, 2006).
The variables “government protection”, “education” and “no help” are identified to be important for the non-structural–proactive household group with importance values of 1, 0.97 and 0.75, respectively (Fig. 4c, Table 3). Their corresponding regression coefficient values (average) are 0.40, 0.36 and 0.26, respectively. Households with a high belief in government protection (i.e. government will establish effective flood protection measures) are motivated to adopt non-structural measures proactively. These households trust the flood protection measures implemented by the government and also undertake action for protecting their property in case of flooding. Trust in government's flood risk management has been also found to be a driver for protective behavioural intention in the Netherlands (Bočkarjova et al., 2009) and for the uptake of structural measures in New York, NY, USA (Botzen et al., 2019b). Education was found to be the next important driver, indicating that households with higher levels of education are more likely to proactively take up non-structural measures, which requires the householders to understand flood risk and choose appropriate precautionary measures. It has been shown before that the level of education impacts a householder's ability to understand flood risk and capture flood forecasting information (Paul and Hossain, 2013). The variable of no help is the third most important, representing the belief of the households that they have to solely cope with the consequences of a flood event. The households that recognize their responsibility to deal with flood impacts and have a high belief in their abilities to protect themselves often proactively take up private precaution (Botzen et al., 2019b; Gebrehiwot and van der Veen, 2015).
The uptake of non-structural measures in the reactive household group is driven by “house damage” and “flood frequency” with importance values of 1 and 0.89, respectively (Fig. 4d, Table 3). The average regression coefficient of house damage is 0.31 and of flood frequency is 0.29. Similar to the structural–reactive households, house damage (i.e. high damage levels experienced from previous flood events) also drives the decision of implementing non-structural measures. Flood frequency indicates the number of flood events experienced in the last 10 years. Households who experienced a large number of flood events show high uptake of non-structural precautionary measures. These findings verify that experiencing flooding and damage due to flooding encourages protective behaviour among households (Ansari, 2018; Bočkarjova et al., 2009).
A limitation of the analysis is that the structural–proactive household group did not reveal any significant influencing variable (Fig. 4a). One potential reason is that many proactive households that have implemented structural measures would have often implemented them while constructing the house or they might have also bought the house with the measure already implemented. In both these cases, we are not able to ascertain whether the householder made a conscious choice to implement the measure. The study is limited to the householder's independent decision stages based on the questionnaire survey. Hence, there are several external factors such as building code requirements by the government and influence by neighbourhood networks that are not considered in this study. This calls for future research based on a comprehensive participatory approach with institutional stakeholders and private householders to develop a systemic understanding of the external factors influencing the uptake of private precaution. The identified drivers of private precaution in proactive households can be used to better motivate all the households exposed to flooding to take up private precaution. For example, risk communication could focus on the measures undertaken by the government to improve flood protection, enhancing trust in government. Information and guidance on the responsibility of households to protect themselves and deal with their flood damage should be provided. Retrospectively, the self-efficacy of households that experienced flooding may be increased by providing them with information on the effectiveness of private precaution and incentivizing its uptake.
A protection motivation theory–transtheoretical model (PMT–TTM) framework was used to analyse empirical data from a household survey consisting of 1000 households in order to identify the drivers of private precaution in HCMC, Vietnam. The analysis shows that factors which positively influence the uptake of private precaution in proactive groups are the level of education, belief that the government takes actions to reduce flood risk and being aware one has to deal with the consequences of flooding by themselves. Further, the perceived increase in severe flood damage in the future discouraged the reactive implementation of structural measures. A limitation of the study is that no influencing drivers could be identified for undertaking structural precautionary measures proactively. This is attributed to the strong possibility that proactive elevation means that the buildings are built elevated and not a result of decision-making from the householder to structurally alter the building as a precautionary measure. This calls for a participatory research approach to account for external drivers and feedback processes influencing private precaution which are outside the scope of a structured questionnaire survey. Based on the results of this study, we recommend that all households (especially the ones with low levels of education) should be made aware of future risk, protection measures by the government and also their individual responsibility to protect their houses. Risk communication and awareness campaigns covering these aspects have the potential to motivate the households to proactively implement precautionary measures.
The code used for our analysis can be provided upon request.
The survey data will be made available via the HOWAS 21 flood damage database (https://doi.org/10.1594/GFZ.SDDB.HOWAS21, GFZ German Research Centre for Geosciences, 2021) after an embargo time of 3 years. Currently the underlying research data can be provided upon request.
TVH, NS, HK and MG: conceptualization and research design. TVH and NS: data analysis and model development. TVH: visualization. TVH, NS, HK and LEY: interpretation of results. TVH: writing (original draft). TVH, NS, HK, MG and LEY: writing (review and editing). NS and HK: supervision.
The contact author has declared that none of the authors has any competing interests.
Publisher’s note: Copernicus Publications remains neutral with regard to jurisdictional claims in published maps and institutional affiliations.
This article is part of the special issue “Future risk and adaptation in coastal cities”. It is not associated with a conference.
We thank Le Thanh Sang, Tan Do and colleagues at the Southern Institute of Social Sciences (SISS) for the implementation of the household survey in Ho Chi Minh City. Thanks go also to Markus Disse from the Chair of Hydrology and River Basin Management at the Technical University of Munich for his support.
This research has been supported by the German Federal Ministry of Education and Research (BMBF) within the framework of the DECIDER project (grant nos. 01LZ1703G and 01LZ1703A).
The article processing charges for this open-access publication were covered by the Helmholtz Centre Potsdam – GFZ German Research Centre for Geosciences.
This paper was edited by Lindsay Beevers and reviewed by Mohammad Shirvani and one anonymous referee.
Alfieri, L., Bisselink, B., Dottori, F., Naumann, G., de Roo, A., Salamon, P., Wyser, K., and Feyen, L.: Global projections of 360 river flood risk in a warmer world, Earths Future, 5, 171–182, https://doi.org/10.1002/2016EF000485, 2017.
Ansari, M. S.: Protection Motivation of Flood Prone Households in North-Central Bangladesh, MSc thesis, Wageningen University, the Netherlands, https://edepot.wur.nl/463593 (last access: 14 March 2023), 2018.
Babcicky, P. and Seebauer, S.: Unpacking Protection Motivation Theory: evidence for a separate protective and non-protective route in private flood mitigation behavior, J. Risk Res., 22, 1503–1521, https://doi.org/10.1080/13669877.2018.1485175, 2019.
Bamberg, S., Masson, T., Brewitt, K., and Nemetschek, N.: Threat, coping and flood prevention – A meta-analysis, J. Environ. Psychol., 54, 116–126, https://doi.org/10.1016/j.jenvp.2017.08.001, 2017.
Barendrecht, M. H., Sairam, N., Cumiskey, L., Metin, A. D., Holz, F., Priest, S. J., and Kreibich, H.: Needed: A systems approach to improve flood risk mitigation through private precautionary measures, Water Security, 11, 100080, https://doi.org/10.1016/j.wasec.2020.100080, 2020.
Block, L. G. and Keller, P. A.: Beyond protection motivation: An integrative theory of health appeals, J. Appl. Soc. Psychol., 28, 1584–1608, https://doi.org/10.1111/j.1559-1816.1998.tb01691.x, 1998.
Bočkarjova, M., van der Veen, A., and Geurts, P. A. T. M.: A PMT-TTM model of protective motivation for flood danger in the Netherlands, ITC Working Papers Series – Paper 3, University of Twente, ISBN 978-90-6164-279-4, 2009.
Botzen, W. J. W., Deschenes, O., and Sanders, M.: The economic impacts of natural disasters: A review of models and empirical studies, Rev. Env. Econ. Policy, 13, 167–188, https://doi.org/10.1093/reep/rez004, 2019a.
Botzen, W. J. W., Kunreuther, H., Czajkowski, J., and de Moel, H.: Adoption of Individual Flood Damage Mitigation Measures in New York City: An Extension of Protection Motivation Theory, Risk Anal., 39, 2143–2159, https://doi.org/10.1111/risa.13318, 2019b.
Bubeck, P., Wouter Botzen, W. J., Laudan, J., Aerts, J. C. J. H., and Thieken, A. H.: Insights into Flood-Coping Appraisals of Protection Motivation Theory: Empirical Evidence from Germany and France, Risk Anal., 38, 1239–1257, https://doi.org/10.1111/risa.12938, 2018.
Cao, A., Esteban, M., Valenzuela, V. P. B., Onuki, M., Takagi, H., Thao, N. D., and Tsuchiya, N.: Future of Asian Deltaic Megacities under sea level rise and land subsidence: current adaptation pathways for Tokyo, Jakarta, Manila, and Ho Chi Minh City, Curr. Opin. Env. Sust., 50, 87–97, https://doi.org/10.1016/j.cosust.2021.02.010, 2021.
Chinh, D. T., Bubeck, P., Dung, N. V., and Kreibich, H.: The 2011 flood event in the Mekong Delta: preparedness, response, damage and recovery of private households and small businesses, Disasters, 40, 753–778, https://doi.org/10.1111/disa.12171, 2016.
Du, S., Scussolini, P., Ward, P. J., Zhang, M., Wen, J., Wang, L., Koks, E., Diaz-Loaiza, A., Gao, J., Ke, Q., and Aerts, J. C. J. H.: Hard or soft flood adaptation? Advantages of a hybrid strategy for Shanghai, Global Environ. Chang., 61, 102037, https://doi.org/10.1016/j.gloenvcha.2020.102037, 2020.
Duffy, C., Braun, A., and Hochschild, V.: Surface Subsidence in Urbanized Coastal Areas: PSI Methods Based on Sentinel-1 for Ho Chi Minh City, Remote Sens., 12, 4130, https://doi.org/10.3390/rs12244130, 2020.
FEMA: Selecting Appropriate Mitigation Measures for Floodprone Structures, https://www.wbdg.org/FFC/DHS/fema551.pdf (last access: 14 March 2023), 2007.
Garschagen, M.: Risky Change? Vulnerability and Adaptation between Climate Change and Transformation Dynamics in Can Tho City, Vietnam, Steiner, Stuttgart, 432 pp., ISBN 978-3-515-10876-8, 2014.
Garschagen, M.: Risky Change? Vietnam's Urban Flood Risk Governance between Climate Dynamics and Transformation, Pac. Aff., 88, 599–621, https://doi.org/10.5509/2015883599, 2015.
Gebrehiwot, T. and van der Veen, A.: Farmers Prone to Drought Risk: Why Some Farmers Undertake Farm-Level Risk-Reduction Measures While Others Not?, Environ. Manage., 55, 588–602, https://doi.org/10.1007/s00267-014-0415-7, 2015.
GFZ German Research Centre for Geosciences: Flood Damage Database HOWAS 21, Helmholtz Centre Potsdam, https://doi.org/10.1594/GFZ.SDDB.HOWAS21, 2021.
Grothmann, T. and Reusswig, F.: People at risk of flooding: Why some residents take precautionary action while others do not, Nat. Hazards, 38, 101–120, https://doi.org/10.1007/s11069-005-8604-6, 2006.
Hallegatte, S., Green, C., Nicholls, R. J., and Corfee-Morlot, J.: Future flood losses in major coastal cities, Nat. Clim. Change, 3, 802–806, https://doi.org/10.1038/nclimate1979, 2013.
Hastie, T., Tibshirani, R., and Friedman, J: The Elements of Statistical Learning, Data Mining, Inference, and Prediction, in: Springer Series in Statistics, 2nd edn., Springer, New York, NY, https://doi.org/10.1007/978-0-387-84858-7, 2008.
Ji, T., Wei, H. H., Sim, T., Yang, L. E., and Scheffran, J.: Disaggregated validation of disaster-resilience indicators using household survey data: A case study of Hong Kong, Sustain. Cities Soc., 67, 102726, https://doi.org/10.1016/j.scs.2021.102726, 2021.
Khoi, D. N. and Trang, H. T.: Analysis of changes in precipitation and extremes events in Ho Chi Minh City, Vietnam, Procedia Engineer., 142, 229–235, https://doi.org/10.1016/j.proeng.2016.02.036, 2016.
Nguyen, M. T., Sebesvari, Z., Souvignet, M., Bachofer, F., Braun, A., Garschagen, M., Schinkel, U., Yang, L. E., Nguyen, L. H. K., Hochschild, V., Assmann, A., and Hagenlocher, M.: Understanding and assessing flood risk in Vietnam: Current status, persisting gaps, and future directions, J. Flood Risk Manage., 14, e12689, https://doi.org/10.1111/jfr3.12689, 2021.
Okayo, J., Odera, P., and Omuterema, S.: Socio-economic characteristics of the community that determine ability to uptake precautionary measures to mitigate flood disaster in Kano Plains, Kisumu County, Kenya, Geoenviron. Disasters, 2, 26, https://doi.org/10.1186/s40677-015-0034-5, 2015.
Paul, S. K. and Hossain, M. N.: People's Perception about Flood Disaster Management in Bangladesh: A Case Study on the Chalan Beel Area, Stamford J. Environ. Hum. Habitat, 2, 72–86, 2013.
Phi, H. L.: Climate Change and Urban Flooding in Ho Chi Minh City, Third Conference on Climate and Water, 3–6 September 2007, Helsinki, Finland, Finnish Environment Institute, 194–199, ISBN 978-952-11-2790-8, 2007.
Poussin, J. K., Botzen, W. J. W., and Aerts, J. C. J. H.: Factors of Influence on Flood Damage Mitigation Behaviour by Households, Environ. Sci. Policy, 40, 69-77, https://doi.org/10.1016/j.envsci.2014.01.013, 2014.
Rogers, W. R.: Cognitive and physiological processes in fear appeals and attitude change: a revised theory of protection motivation, Social Psychophysiology: A Sourcebook, Guilford Publications, 153–177, ISBN 9780898626261, 1983.
Scussolini, P., Tran, T. T. van, Koks, E., Diaz-Loaiza, A., Ho, P. L., and Lasage, R.: Adaptation to Sea Level Rise: A Multidisciplinary Analysis for Ho Chi Minh City, Vietnam, Water Resour. Res., 53, 10841–10857, https://doi.org/10.1002/2017WR021344, 2017.
Tibshirani, R.: Regression Shrinkage and Selection via the Lasso, J. Roy. Stat. Soc., 58, 267–288, https://doi.org/10.1111/j.2517-6161.1996.tb02080.x, 1996.
Weyrich, P., Mondino, E., Borga, M., Di Baldassarre, G., Patt, A., and Scolobig, A.: A flood-risk-oriented, dynamic protection motivation framework to explain risk reduction behaviours, Nat. Hazards Earth Syst. Sci., 20, 287–298, https://doi.org/10.5194/nhess-20-287-2020, 2020.
Woetzel, J., Pinner, D., Samandari, H., Engel, H., Krishnan, M., Boland, B., and Cooper, P.: Can coastal cities turn the tide on rising flood risk?, McKinsey Global Institue, https://www.mckinsey.com/~/media/mckinsey/business%20functions/sustainability/our%20insights/can%20coastal%20cities%20turn%20the%20tide%20on%20rising%20flood%20risk/mgi-can-coastal-cities-turn-the-tide-on-rising-flood-risk.pdf (last access: 14 March 2023), 2020.
Yang, L. E., Scheffran, J., Süsser, D., Dawson, R., and Chen, Y. D.: Assessment of Flood Losses with Household Responses: Agent-Based Simulation in an Urban Catchment Area, Environ. Model. Assess., 23, 369–388, https://doi.org/10.1007/s10666-018-9597-3, 2018.
Yang, L. E., Garschagen, M., Diez, J. R., and Kreibich, H.: DECIDER Project, Project Newsletter, https://doi.org/10.13140/RG.2.2.23567.41125, 2020.
Zou, H. and Hastie, T.: Regularization and variable selection via the elastic net, J. Roy. Stat. Soc. B, 67, 301–320, https://doi.org/10.1111/j.1467-9868.2005.00503.x, 2005.