the Creative Commons Attribution 4.0 License.
the Creative Commons Attribution 4.0 License.
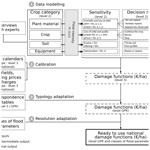
Process-based flood damage modelling relying on expert knowledge: a methodological contribution applied to the agricultural sector
Anne-Laurence Agenais
Frédéric Grelot
Claire Richert
Flood damage assessment is crucial for evaluating flood management policies. In particular, properly assessing damage to agricultural assets is important because they are complex economic systems particularly exposed to floods. The modelling approaches used to assess flood damage are of several types and can be fed by damage data collected post-flood, from experiments or based on expert knowledge. The process-based models fed by expert knowledge are the subject of research and also widely used in an operational way. Although identified as potentially transferable, they are in reality often case-specific and difficult to reuse in time (updatability) and space (transferability). In this paper, we argue that process-based models, based on a rigorous modelling process, can be suitable for application in different contexts. We propose a methodological framework aimed at verifying the conditions necessary to develop these models in a spirit of capitalisation by relying on four axes which are (i) the explicitation of assumptions, (ii) the validation, (iii) the updatability, (iv) the transferability. The methodological framework is then applied to the model we have developed in France to produce national damage functions for the agricultural sector. We show in this paper that the proposed methodological framework facilitates an explicit description of the modelling assumptions and data used, which is necessary to consider for a reuse in time or for transfer to another geographical area. In this sense, this methodological framework constitutes a solid basis for considering the validation, transfer, comparison and capitalisation of data collected around models based on processes relying on expert knowledge. In conclusion, we identify research tracks to be implemented so as to pursue this improvement in a spirit of capitalisation and international cooperation.
- Article
(3590 KB) -
Supplement
(854 KB) - BibTeX
- EndNote
Worldwide, flooding causes huge damage (van Loenhout et al., 2020) estimated at EUR 58 billion (USD 75 billion) per year (Alfieri et al., 2017). The EU Floods Directive (Directive 2007/60/EC) requires Member States first to map the flood extent and assets at risk, and second to coordinate measures to reduce this flood risk. All Member States are confronted with this challenge to decrease total flood damage while urban assets continue developing in flood-prone areas (Rojas et al., 2013). To face this challenge, flood management usually mixes several types of approaches at the river basin level. Agricultural areas globally generate less damage than urban areas (1 % only of the total damage in Europe (Alfieri et al., 2017)). As a consequence, protection measures such as dykes are usually dedicated to protecting urban areas. Farmers are seen as potential contributors to reduce flood risk either by changing their practices (O'Connell et al., 2007; Posthumus and Morris, 2010) or by using agricultural lands to give more room for water flooding, which involves increasing their exposure (Morris et al., 2010). However, the second type of measure raises many questions on acceptability and compensation (Zandersen et al., 2020; Erdlenbruch et al., 2009; Posthumus et al., 2008, 2010). Thus, properly evaluating flood damage in agriculture becomes a real issue for two reasons. First, evaluating flood damage in agriculture is necessary so as to justify the efficiency of the policy and then the choice to be made between several options. This is usually done by performing cost–benefit analysis, which requires developing flood damage functions (Jonkman et al., 2008; Merz et al., 2010). Second, even if the project is efficient, the acceptability of these measures requires involving farmers (Posthumus et al., 2008) and introducing compensation payments (Erdlenbruch et al., 2009). To reach this goal, developing a comprehensive model to evaluate flood damage on farms is necessary. In particular, to discuss and build a trusting relationship with farmers who may be over-exposed, this model needs to reflect as much as possible what happens to them in the case of flooding.
Several classifications of the methods used to model flood damage can be found in the literature (Jongman et al., 2012; Davis and Skaggs, 1992; Merz et al., 2010; Molinari et al., 2020; Malgwi et al., 2021). However, these classifications are not operative because they mix the modelling methods and the data necessary to feed the models. Presenting the modelling methods separately from the data needed to feed them provides greater clarity. The strategies generally adopted to model flood damage are (i) data-driven modelling, (ii) conceptual modelling and (iii) process-based modelling. To feed these models, different types of data can be used: (i) damage observation data, (ii) data from expert knowledge, (iii) data from experiments. Data-driven modelling approaches requires damage observation data. Conceptual modelling is more often used to evaluate indirect damage with input–output (IO) models (Hallegatte, 2008; Van der Veen et al., 2003; Hallegatte, 2014; Crawford-Brown et al., 2013; Xie et al., 2012) or computable general equilibrium (CGE) models (Xie et al., 2014; Rose and Liao, 2005; OCDE, 2014). They are appropriate for indirect and large-scale damage evaluation but not for sectoral damage evaluation at micro and meso scales. Process-based modelling can be fed by expert knowledge or experimental data. Experiments require significant monetary and time investments. Most often process-based modelling approaches are fed with expert knowledge. To do so, it is recommended to have experienced interviewers, who also have some knowledge of making damage estimates (Davis and Skaggs, 1992). To illustrate these categories of modelling approaches, let us take the example of flood damage assessment models developed in Germany and the United Kingdom. In Germany, a huge effort to collect post-flood damage data has been made (Thieken et al., 2017) and the models developed for residential (FLEMO-ps) (Thieken et al., 2008a) and economic assets (FLEMO-c) (Kreibich et al., 2010) are data-driven models. On the contrary, the flood damage functions that have been established in the United Kingdom by the Flood Hazard Research Centre (FHRC) are process-based models fed with expert knowledge (Penning-Rowsell and Chatterton, 1977; Penning-Rowsell et al., 1992, 2005, 2013; Priest et al., 2021b). The flood damage models INSYDE (Dottori et al., 2016) in Italy or floodam.building (Grelot and Richert, 2019) in France are also part of this category. Each of these methods has its advantages and drawbacks. For data-based approaches, it remains difficult to systematically collect individual data on a large scale. For process-based approaches, the understanding of processes often remains too incomplete (Merz et al., 2010; Meyer et al., 2012, 2013). Moreover, it is often pointed out that process-based modelling approaches are context specific and do not enable capitalisation of modelling efforts to other contexts.
For the agricultural sector, no data-driven models were found in the literature. In Germany, no model such as FLEMOps or FLEMOc exists for agriculture (Thieken et al., 2008b). To evaluate agricultural damage in the MEDIS project, Förster et al. (2008) extrapolated yield loss estimation based on one specific flood in Germany. This can be explained by the fact that few sinistrality data are available for the agricultural sector. The penetration rate of private insurance for flood crop losses is low (Priest et al., 2021a; Browne, 2000; Vozinaki et al., 2015) and no private insurance for overall agricultural damage exists as, for example for soil erosion. Conceptual models are not suitable for assessing damage at the watershed or farm level (Meyer et al., 2013). Flood damage in economic activities such as farming is classically estimated by the loss of added value (Penning-Roswell et al., 2005; Brémond and Grelot, 2010), which corresponds to the decrease in product minus the variation in production costs due to flooding (Brémond et al., 2013). Because of flood impacts, the farmer will make some choices which will lead to variation in production costs. Some may be saved (harvest) while others may increase (treatment, tillage, for instance). Thus, damage to agricultural assets results both from complex biophysical processes and from repair and recovery actions taken by farmers, which need to be explained for the damage to be assessed (Brémond et al., 2013; Brémond, 2011; Durant et al., 2018; Priest et al., 2021a). For this purpose, a process-based modelling approach seems to be the most promising. As experimental data on flood damage on farms are scarce and context specific (Satrapa et al., 2012), feeding expert knowledge into the models seems most suitable. However, a literature review of 42 studies on flood damage modelling for agricultural activities (Brémond et al., 2013) showed that many simplifications are usually made: (i) most methods considered only the loss of yield; (ii) the loss of yield was estimated as a function of period of the year which hinders the transferability to other geographical context; (iii) the biophysical processes considered were not explicit; (iv) the implications of flooding for farmers' actions were not explicitly considered or were not transferable; (v) the implications of flooding for perennial crops were not taken into account; (vi) the modelling assumptions were not validated.
In this article, we analyse and discuss the methodological aspects required to develop process-based damage assessment models in a spirit of capitalisation. We propose a framework for the development of damage assessment models based on expert knowledge and illustrate its use around the model floodam.agri that we have developed and used to produce flood damage functions for the agricultural sector in France. Two questions are addressed: (i) is the methodological framework we propose useful for developing flood damage assessment models in the spirit of capitalisation? (ii) What methodological efforts are needed to develop process-based models that are not only context specific in this capitalisation and cooperation perspective? In Sect. 2, based on a state of the art, the proposed methodological framework is detailed around its four axes: (i) explicit assumptions, (ii) validation, (iii) updatability and (iv) transferability. In Sect. 3, the case study, i.e. the context and main steps of development of floodam.agri are presented. Then, in Sect. 4, the methodological framework is applied to floodam.agri. In Sect. 5, the usefulness and limitations of the framework are discussed. Finally, Sect. 6 concludes by outlining the research avenues to be developed for the improvement of process-based models.
2.1 Proposition of a methodological framework
Based on a review of the literature as well as on our own modelling experience, we propose the methodological framework presented in Table 1. It is presented in the form of questions that are as many conditions to be respected for the development of process-based models from a capitalisation perspective. We detail the conditions of each axis in Sect. 2.2 to 2.5.
2.2 Axis 1: explicit assumptions – system boundaries, biophysical processes and decisions
Gerl et al. (2016) reviewed 47 flood damage models (process based or data driven) in order to create a basis for harmonisation and benchmarking. One of their main conclusions is that this requires profound insight into the model structures, mechanisms and underlying assumptions. In the following, we highlight which assumptions need to be made explicit.
Flood damage is usually classified into four types: direct tangible (e.g. physical damage due to contact with water), indirect tangible (e.g. loss of production and income), direct intangible (e.g. loss of life) and indirect intangible (Jongman et al., 2012; Merz et al., 2010; Priest et al., 2021b). To evaluate the flood effects on economic activities, defining the limits of the system considered is crucial to distinguish between direct and indirect damage since the flood impacts not only the property directly affected. As an example, in agricultural assets, Brémond and Grelot (2012) identified damage induced at the farm scale due to the links between farm plots and buildings. Nortes Martínez et al. (2020) shows the importance of interactions betweens farms and the cooperative at a winery cooperative scale and the consequences on flood damage estimation. Therefore, a clear definition of the boundaries and components of the system under consideration is necessary in order to avoid errors of double counted or uncounted damage. This refers to condition EA1 in Table 1.
Then, process-based models try to reflect physical or biophysical processes that occur in the considered system and which generate flood impacts. These processes are numerous, depend on the component of the system considered and may depend on different flood parameters (Kelman and Spence, 2004). Explicit assumptions regarding which processes are considered as well as which components of the system and which flood parameters are involved are essential in process-based models (Davis and Skaggs, 1992). Condition EA2 (Table 1) is developed in sub-conditions that help to detail how the biophysical processes due to a flood in the considered system are taken into account.
Finally, flood damage results in an interaction between the flood impacts and human behaviour (Middelmann-Fernandes, 2010). At the end, evaluating the damage in monetary terms requires knowledge of the repair and restoration choices made by the people affected and their costs. In data-driven modelling, these choices are implicitly included in the damage data collected. In process-based models, the property damage avoided technique is used (Shabman and Stephenson, 1996). The repair choices and their costs are hypothetical and fed with expert knowledge. As a consequence, explicit assumptions on the decision rules considered are also critical to properly describe a process-based damage model. This refers to condition EA3 (Table 1).
2.3 Axis 2: validation
Although the research community has put a lot of effort into improving flood damage models, Molinari et al. (2019a) point to the lack of validation and identify three modalities for the validation of flood damage models, which are (i) the comparison with observed data, (ii) the comparison with other models, (iii) the use of expert judgement. In the methodological framework (Table 1), condition V1 questions the possibility to compare the outputs with observed damage data and condition V2 to compare the models between them. However, for all sectors, and especially for the agricultural one, a lack of data to fully implement the first modality is commonly observed. In addition, sinistrality data should be considered with caution as they may only represent part of the damage that one wishes to compare. The insurance coverage of the different types of damage, in particular in agriculture, is not complete. As for the second one, a lot of work is being done to compare the different existing models (Gerl et al., 2016; Molinari et al., 2020; Malgwi et al., 2021) in order to have a better idea of the uncertainties. However, the difficulties encountered are often related to the lack of explicit assumptions used in the approaches and modelling choices, which brings us back to the importance of properly addressing axis 1 of our methodological framework. The third modality is a validation related to the operationality and use of the model. We state that two perspectives should be distinguished: (i) adequacy with the stakeholders' expectations (condition V3), which is related to the use of the model in practice (V4), (ii) validation with the experts involved in the modelling process (V5). As for the second point, few experiences and methods have been found. Let us mention the experience of Dias et al. (2018), who discussed with experts the data collected for the construction of damage functions in buildings. The methodology for validating the models with experts remains to be consolidated. Based on our own experience, we detail in condition V5, the sub-conditions which seem to us necessary for the validation by the experts involved in the modelling process in the following steps: (i) discussion of the modelling assumptions about processes and recovery actions, (ii) discussion of the monetisation values; (iii) discussion of the outputs.
2.4 Axis 3: updatability
Although some research exists on updating flood hazard models, for example by integrating climate change (Hattermann et al., 2016), the update of flood damage models remains minimally investigated but is necessary (Comiskey, 2005). Updatability is defined as the possibility of updating and should be understood as the anticipation in the modelling process of the possibility of updating the calibration data of the model. This notion is different from the update, which corresponds to updating the model outputs. It can be achieved through the updatability of the source data or through simplified methods of actualisation of the outputs. The update when it is addressed, concerns the values enabling the monetisation as, for example in the last version of the multi-coloured handbook (Priest et al., 2021b). In general, the databases used are rarely made explicit and even less so the vintages. It is therefore important to verify whether the types of data and their sources are made explicit (condition U1, Table 1), whether the database vintages used are specified (condition U2) and whether the databases are tracked over time (condition U3).
2.5 Axis 4: transferability
Transferring the flood damage model is a challenging issue (Molinari et al., 2020; Jongman et al., 2012; Cammerer et al., 2013). As we dealt with updating in Sect. 2.4, we focus here on transfer in space and on improvements of the model. Improving modelling techniques to transfer data-driven flood damage models has been widely explored (Wagenaar et al., 2018, 2021). However, the transfer of process-based model is very challenging mainly because it requires in-depth understanding of the origin, calibration, assumptions and field of application, which brings us back again to the central issue of explicit modelling assumptions (Sect. 2.2). Although process-based modelling approaches seem to be the most promising in terms of transferability, the lack of explicit assumptions hinders this and the models developed remain context-specific (Vozinaki et al., 2015). For example, for the development of AGRIDE-c (Molinari et al., 2019b; Scorzini et al., 2020), floodam.agri was partially transferred. In particular, the yield loss coefficients were directly used after discussions with local experts. However, the part concerning the validation by experts remains poorly detailed in Molinari et al. (2019b) and Scorzini et al. (2020). We are not sure that all the central assumptions of floodam.agri, namely biophysical processes and farmers' decisions, were sufficiently detailed in Agenais et al. (2013) to enable transferability. Condition T1 (Table 1) checks whether the adaptation, improvement or transfer conditions have been taken into account and described at the time of the model design. Condition T2 refers to the effective transfer of the model.
3.1 Context of development and implications
In France, since 2011, it has been mandatory for local flood risk managers to conduct cost–benefit analysis (CBA) of their flood management projects to make them eligible for financial support from the State. Meanwhile, as support, the French ministry in charge of the environment proposed a methodology to fulfil CBA (Rouchon et al., 2018). A working group of engineers and researchers of which we were part was charged with developing damage functions usable at a national scale. The idea was that the consulting firms in charge of the realisation of CBA for local flood risk managers could use these resources whatever the context. Two strategies were possible: reuse and adapt damage functions to the French context or develop our own functions. For the agricultural sector, among existing process-based models, the AGDAM model developed by the USACE (1985) and the model developed by the FHRC (Hess and Morris, 1988; Morris and Hess, 1988; Penning-Roswell et al., 2005; Priest et al., 2021a) stood out as being the most advanced. The possibility of adapting AGDAM or the FHRC damage functions was investigated. However, a review of the literature (Brémond et al., 2013) revealed that no existing damage function could meet the operational needs. Indeed, the ministry needed ready-to-use French National Damage Functions (i) that cover the vast majority of French agricultural crops and that were compatible with the databases used to locate them (Graphical Plot Register, GPR, which is the French Land Parcel Identification System, LPIS, connected to the Common Agricultural Policy), (ii) that are applicable at a national scale but can be adjusted to local specificities if needed (specific culture, selling price, etc.) and (iii) that are updatable, i.e. based on values from identified databases that can be tracked over time as far as possible. Then, the option selected was to develop our own damage functions. As in other countries, the lack of sinistrality data quickly led us to choose process-based models based on expert knowledge. In this paper, we focus on the floodam.agri model that we developed and that was used to produce the national damage functions. However, ready-to-use national flood damage functions have been developed for the residential sector, public infrastructures, agricultural sector, and commercial and industrial sectors. They are all available online (https://www.ecologie.gouv.fr/levaluation-economique-des-projets-gestion-des-risques, last access: 13 October 2022). In practice, since 2013, over 200 flood management projects have been analysed by CBA using this method and flood damage functions.
3.2 Methodology to develop floodam.agri and produce national damage functions
The floodam.agri model includes generic parts and can produce damage functions at different scales, depending on the calibration. We illustrate in this paper the use of floodam.agri to produce damage functions at the national scale. This methodology has followed seven stages (Fig. 1).
First, the conceptual framework was established. A crop category is broken down into elementary components and for each component, the damage is estimated based on the biophysical processes at work due to the flood and the actions carried out by farmers after the flood.
Second, to inform the conceptual framework, 30 individual surveys (Fig. 2) with agricultural experts working in regional technical institutes were carried out. They usually had expertise at the level of a crop family that encompasses several categories (Appendix A, Table A1). Some had expertise in several families of crops. Among the experts, six were specialists in grain and oleaginous crops, eight in vegetable crops, four in vines, three in fruit trees, and eight in meadows and feeding crops. The experts worked in geographical areas where crops had been impacted by at least once flood since 2005. We focused on five areas that differ in terms of hydrological and agricultural contexts: two Mediterranean areas, an area composed of alluvial plains and mountains, an oceanic area, and a rural area composed of plains and plateaus.
A questionnaire was designed (Supplement 1) to conduct semi-structured interviews that lasted about 1 h. It was structured in two parts in order to collect information (i) on the impacts on farm components and (ii) on the consequences for farmers' practices. Prior to every interview, production cycles in terms of physiological stages and agricultural work calendar were established based on the literature, for the categories of crop corresponding to the expert interviewed. This information was presented and discussed with the experts too.
Third, floodam.agri implementation can be summarised as shown in Fig. 3. The crops for which damage can be estimated with floodam.agri are defined in a three-level classification (Appendix A, Table A1). All the crops that belong to the same category are associated with a similar vulnerability to floods, but can differ in terms of their other characteristics (yield, selling price, crop calendar, intermediate consumption). For each component, based on the interviews, the sensitivity to flooding is modelled, i.e. a proportion of loss or level of deterioration of a component is associated with flood parameters; for example for the crop component, the sensitivity determines a loss of yield in percent of the standard yield. Decision rules associate behaviours with the proportion of loss or level of deterioration of a component. The floodam.agri model was implemented using R language.
Fourth, floodam.agri must be calibrated with data such as agricultural calendars, yields, and sales prices to produce flood damage functions. The level of data specification should be appropriate for the scale at which the damage functions are to be produced. For the national damage functions, we used data at the national level.
Fifth, a validation was carried out through focus groups bringing together the experts consulted in individual interviews for each crop family. This step occurred on average 1 year after the first interview. In total, five focus group were organised (see Sect. 4.2).
Sixth, based on focus group discussions, some corrections were made.
Seventh, the process resulted in ready-to-use flood damage functions. To produce them, two more steps (3 and 4 in Fig. 3) were achieved: (3) adapting the damage functions to fit the typology used to locate the crops (GPR), (4) adapting the resolution of the functions to fit the available data that pertain to flood parameters. The ranges of values considered for each parameter in floodam.agri and the grouping choices for the period of occurrence and flood duration categories chosen for ready-to-use damage functions are specified in Appendix B (respectively Tables B1–B3). In addition for the national application, to manage rotations if necessary on the application territory, we proposed to create a mixed function. For example, if the 3-year rotation is wheat, wheat, rape, the weight assigned to the wheat function is and the weight assigned to rape is .
3.3 Overview of French national damage functions
Ready-to-use national damage functions were produced for 15 of the 28 sorts of crop of the GPR typology (Supplement 2). These 15 sorts accounted for 89 % of agricultural areas located in flood-prone areas in metropolitan France in 2010, according to the GPR database. They indicate the estimated expected value of damage in euros by hectare, depending on the water depth, submersion duration, season of occurrence of the flood, and flow speed. The maximum expected damage is the lowest by hectare for sunflower crops (EUR 1611) and the highest for arboriculture and orchards (EUR 93 549) (Table A2).
For illustrative purpose, Fig. 4 shows the damage function of the arboriculture. How the hazard parameters were aggregated to produce these graphical outputs is specified in Appendix B. The damage increases with the flow speed, the submersion duration and the water depth. It is generally the highest in spring and the lowest in winter.
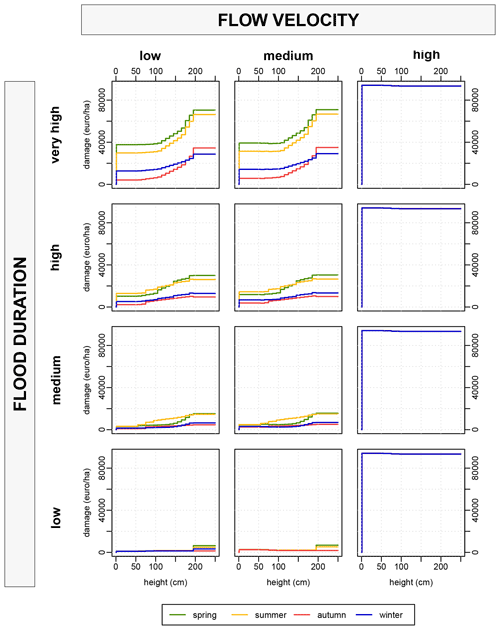
Figure 4Example of the national flood damage function developed using floodam.agri for the category “arboriculture”.
The threshold effects in the relationship between the damage and the water depth correspond to the water depths at which new types of plant organs are reached by water (e.g. leaves, fruits).
In this section, the methodological framework (Table 1) is applied to floodam.agri. The objective is to analyse the extent to which the framework makes the modelling process explicit and allows for the transfer of the model to other study cases. A detailed illustration is given for apple crop.
4.1 Axis 1: explicit assumptions
4.1.1 EA1: what are the boundaries and components of the system considered?
The floodam.agri model is a conceptual model developed on the basis of the literature and previous works (Brémond et al., 2013; Brémond, 2011; Nortes Martínez, 2019a). Flood impacts on the agricultural sector can be evaluated by the variation of added value through the production process. Figure 5 represents the links between economic entities that may impact the variation in added value. Each economic entity is composed of physical components such as building and parcels that can be directly affected by a flood. The farmer makes choices for the production process and recovery if a flood occurs. At farm level, the growing process can be impacted either directly by the flood or indirectly if the farm buildings are impacted. In the same way, flood impacts on suppliers may interfere in the production process. The components in dark grey are those that are currently considered in floodam.agri. It takes into account the physical components related to the land plots, namely crops, plant material, soil and equipment such as irrigation systems, fences and trellis depending on the crop type. It also takes into account the farmer's decision in terms of adaptation of production tasks (crop management sequence) and recovery tasks. Using floodam.agri requires specifying some data on these components to produce the damage functions. These assumptions represent a national vision for the development of national damage functions but can be specified at other scales. They were made in collaboration with the experts consulted. For example, we had to set the physiological stages to the weeks of the year (example for the apple in Fig. 6). Similarly, assumptions were made about certain physical characteristics (trunk heights, first fruits). Finally, we also specified the crop management sequences for each crop (according to the physiological stages and based on the weeks of the year for the national application).
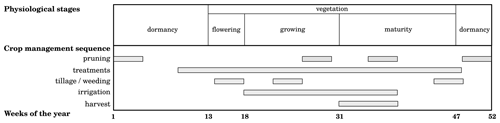
Figure 6Distribution of the physiological stages and crop management sequence of apple crop over the weeks of a year selected for the national functions.
Interviews were conducted on the vulnerability of farm buildings and their contents (equipment and stock) as well as cattle. However, these elements have not been integrated into floodam.agri to date. Furthermore, floodam.agri also does not consider damage induced at the farm scale, i.e. damage induced on farm activity due to direct damage on farm equipment, for example as evaluated in Brémond and Grelot (2012) or indirect damage at the scale of the area affected by a flood as, for example damage propagation on cooperatives as evaluated in Nortes Martínez (2019a) and described in Nortes Martínez (2019b). Indeed, in an operational way, it remains very difficult to obtain information concerning the links between farm buildings and parcels of the same farm or the links between farms and cooperatives.
Equations (1)–(4) describe the translation of this conceptual framework in economic terms. The total damage to a plot (D in Euro per hectare) is the sum of the costs of the actions needed to restore the plot (C in Euro per hectare) and of the loss of added value (ΔAV in Euro per hectare). It is calculated as the sum of the damage to each component of the plot (Dc): (i) plant material (for perennial crops), (ii) the crop production, (iii) the soil, and (iv) equipment. The crop component is defined as the part of the plant that is harvested.
The added value is the difference between the outcome of the plot (O) and the intermediate consumption due to its management (IC). The outcome is the product of the yield (Y) and the selling price (P), while the intermediate consumption is the consumption in terms of input, material, and labour. The loss of added value is the difference between the usual added value and the added value following a flood.
4.1.2 EA2: what are the biophysical processes that cause the damage considered?
The methodological framework proposes to discuss this question following two sub-questions.
-
Are the biophysical processes that cause the damage taken into account in the model explicitly considered?
-
Are the links between biophysical processes and flood parameters clearly defined?
For each component, Table 2 summarises the processes at work in the formation of damage, the major flood parameters involved, whether the process is considered or not in floodam.agri and if yes how it is estimated. These processes have been identified based on the literature and during the individual interviews.
Table 2Biophysical processes considered or not in the national flood damage functions produced with floodam.agri.
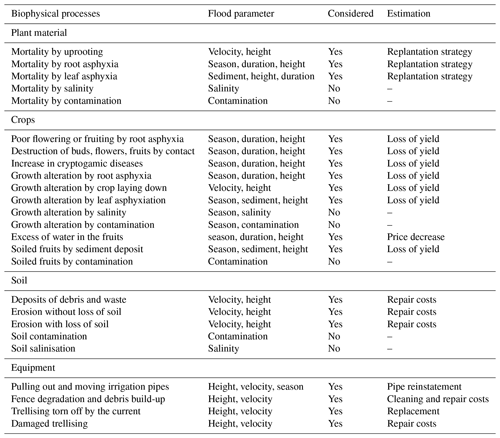
The parameters used to characterise the floods are (i) the height, (ii) the duration of submersion, (iii) the velocity, and (iv) the season. Flood impacts on crops were described as a function of physiological stages instead of time of the year to maintain the adaptability of our model to different contexts. The relevance of the choice of these physiological stages to the sensitivity of the component to flooding was discussed with the experts. For apple, for example (Fig. 6), to qualify the sensitivity of the plant material (tree), two stages were defined (dormancy and vegetation) and for the crop, five stages (dormancy, bud-break, flowering, growing, maturity). The effects of water level are defined taking into account crop data (trunk height, fruit height and maximum height). For apple trees, the height of the trunk has been set at 80 cm and the maximum height of the trees at 200 cm.
Plant material
Table 2 shows that the main processes that cause mortality of plant material, i.e. tree or vine, are uprooting or asphyxia. Table 3 details how the processes discussed with the experts were modelled into a mortality rate (β) for a low-velocity flood. For the example of arboriculture, trees are considered to be uprooted for high velocity. The diagrams represent the mortality rate (β) as a function of the flood parameters (duration and height) for the two physiological stages defined with experts. The proportion of trees suffering from asphyxia increases with the water depth and submersion duration. There is no uprooting for low or medium velocity.
Crop
For perennial crops, on a plot, the crop borne by the destroyed plant material (β) is considered destroyed as well. The crop loss (α) on the undamaged plant material (1−β) is then determined. Table 2 synthesises the processes identified with experts which contribute to yield loss (α) or quality loss (γ) for annual and perennial crops. Table 4 illustrates for the apple crop how the processes were associated with yield loss. In addition to yield losses, flooding can cause a deterioration in the quality of the remaining fruit (e.g. reduced shelf-life potential) and generate a lower selling price. Based on the interviews with experts, it was considered for the example of apples that the selling price (Pu) is reduced by 10 % when the flooding occurs at the maturity stage with a height of more than 80 cm and a duration of more than 2 d. This effect is added to the loss of yield as described in Eq. (11).
Soil
The flood impacts on the soil component taken into account in floodam.agri are erosion and littering (Table 2). Erosion depends on the flow speed, and the quantity of material carried by flood water depends on the water depth. How the damage processes were related to the flooding parameters based on the interviews for the arboriculture example is detailed in Table 8. Currently, the phenomena of organic matter loss or pollution are not taken into account. This is mainly due to the fact that the experts we met have not been confronted with these problems in a systematic way. The salinisation phenomena are the subject of an adaptation which is in progress.
Equipment
Equipment on the plots (i.e. irrigation systems, fences, greenhouses, and trellis) can be deteriorated or destroyed (Table 2). The deterioration or destruction of equipment depends on the flow speed, which influences the number of devices that move during the flood, and on the water depth that is linked to the number of devices immersed.
4.1.3 EA3: what are the assumptions on farmers' decisions?
The assumptions made on the decision rules of farmers after the flood are linked to the damage endured and the physiological stage of the crops. They are detailed for each component below.
Behaviour in standard situation
The behaviour of farmers in the standard situation is defined by the crop management sequence, which is the logical and orderly sequence of tasks that must be performed to achieve the set yield (Sébillote and Soler, 1990). The periods in which tasks must be performed are defined on the basis of physiological stages (example for the apple, Fig. 6). These sequences of tasks were used as a basis to discuss with the experts the change in farmers' behaviour due to flooding. The list of potential additional or cancelled tasks is presented in Table 5.
Decisions related to plant material
Direct (DPM) and delayed () damage to plant material is estimated. Direct damage to plant material does not depend on the farmer's decisions and is estimated by the loss of outcome due to the loss of plants:
with β the proportion of plants lost by hectare, Yu the mean usual yield by hectare, and Pu the mean usual selling price.
Then, delayed damage () is estimated taking into account the farmer's decision. Depending on the tree mortality rate (β), three possible strategies are considered in Table 6: (i) no replanting (Eq. 7), (ii) replanting only the missing trees (Eq. 8), (iii) grubbing and replanting the entire plot (Eq. 9). Each strategy is associated with costs and their mathematical formulation. Table 6 gives the values of (β) associated with the three strategies that were collected from the experts of arboriculture.
Decisions related to crops
The possible strategies following the loss of yield are different depending on whether the crop is perennial or annual. Table 7 summarises the possible strategies and the associated equation to calculate damage.
In floodam.agri, farmers of perennial crops have only two choices: continue (Eq. 11) or stop (Eq. 12) the crops. In all cases, the basic assumption is that of a continuity of the production of the current crop. That is to say that no radical change in the orientation of the farm's production is envisaged. Most of the time they decide to continue the crop management sequence also because leaving rotten fruit in the orchard or vineyard could lead to disease development. For example, for apple crops, the harvest is always carried out unless the total yield losses, i.e. combining yield losses alone and plant material losses, are (i) more than 95 % and the flooding took place before the maturity stage, (ii) more than 75 % and the flooding takes place at the maturity stage (a chemical treatment is then carried out). Moreover, if they continue, for the case of apple crops, there is no variation of intermediate consumptions because the treatments are already very regular in normal situations.
Regarding annual crops, farmers generally have to modify their usual crop management plan then the additional expenses in terms of treatments to avoid moisture-related diseases (Eq. 10). They can also decide to stop the crop (Eq. 12).
Two additional strategies are possible for annuals crops as a function of the period of occurrence of the flood and the loss of yield. It is possible to re-sow the same crop if the flood occurs early enough in the crop's development cycle (e.g. up to the emergence stage for winter and summer field crops). In this case, the damage is expressed in terms of yield loss due to the later seeding plus the additional seeding costs (Eq. 13). The possibility of planting another catch crop is also being considered. This is particularly the case when the flooding occurs too late on a winter cereal for the same crop to be re-sown. Grain farmers may then consider planting a spring or summer cereal. This alternation is part of the crop rotation that they practice on a multi-year basis. In this case, the damage is expressed in terms of the possible loss of product linked to the realisation of this new crop to which is added the cost of a new sowing (Eq. 14).
Decisions related to the soil and equipment
For the soil and equipment, the repair and replacement actions have been defined with experts as a function of flood impacts on the component. The damage to the soil component (Dsoil) relates only to the year of the flood. It is equal to the costs of tilling the soil to correct for erosion and picking up litter, which depend on the labour and mechanisation costs:
with dtilling the amount of time needed to till one hectare of soil, dcleaning the amount of time needed by hectare to pick up litter, Clabour the labour cost, and Cmecha the mechanisation cost.
For the case of orchards, Table 8 summarises the actions to be carried out and the estimated work times that have been defined with the experts. The damage to the soil was defined in the same way for each crop family.
Table 8Illustration of assumptions elaborated with experts for soil damage in the case of orchards for the national damage functions.
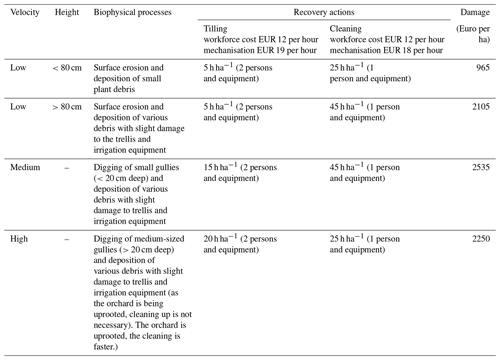
The damage to equipment (Deq) relates only to the year of the flood. It is equal to the replacement and repair costs, which include labour and material costs:
with I the set of devices that need to be replaced, J the set of devices that need to be repaired, Cmat the material cost to replace or repair a device, and drepair the amount of time needed to repair a device.
4.2 Axis 2: validation
In this section, the methodological framework (Table 1) is used to describe the validation process implemented for floodam.agri.
4.2.1 V1: is it possible to compare the model results with sinistrality data?
As specified in Sect. 3, to date, it is not possible to compare flood damage models developed for the agricultural sector with sinistrality data since no such data exist (Priest et al., 2021a; Vozinaki et al., 2015). In France, sinistrality data on the agricultural sector are very limited and unsuitable for comparison with the damage functions developed. Indeed, the penetration rate of private insurance is very low. Compensation is mainly paid through the National Agricultural Risk Guarantee Fund (FNGRA). However, this system compensates only part of the crop losses (e.g. losses of grapes or cereals are not covered) and, moreover, it is a compensation system based on a declarative estimate of losses at the time of the flood. It does not take into account, as we have tried to do in this study, the deferred losses and the variations in expenses linked to farmers' decisions.
4.2.2 V2: is it possible to compare the results of the model with other similar models?
To date, no comparison of floodam.agri has been made with other models. To our knowledge, this has not been done for any flood damage assessment model for agriculture. Comparing floodam.agri with existing flood damage model for agriculture such as the flood damage functions developed by the FHRC in the United Kingdom or AGDAM in the United States would required a common case study. No such initiative has been done yet. We hope that the effort of explicitness made in this article contributes toward this direction. As a first step, Table C1 uses the methodological framework we propose to compare floodam.agri, the FHRC method and the AGDAM method. This comparison was made on the basis of the documents we had at our disposal, namely the AGDAM users manual (USACE, 1985) and the different versions of the multicoloured manual (Penning-Roswell et al., 2005; Priest et al., 2021b). On the basis of existing documents, a certain amount of information remains incomplete (the number of applications, transfers that may have not been published, etc.). This table should not be considered as a result in itself but it highlights that the framework proposed in this article constitutes a basis for discussion for the comparison and transfer of process-based models.
4.2.3 V3: does the model meet stakeholders' expectations?
The national flood damage functions that were produced using floodam.agri were used by stakeholders (engineering firms and project developers) between 2014 and 2022 in more than 200 CBAs. This proves that floodam.agri has met the expectations of the stakeholders involved in the process, namely the Ministry of the Environment, the local authorities in charge of the project and the consulting firms that carry out the CBA.
4.2.4 V4: has the model been presented and discussed with the experts involved for the development?
Within the framework of the development of floodam.agri, we implemented a specific methodology allowing us to discuss and validate in groups during workshops the setting in the model of the information collected in individual interviews. This qualitative research method is the focus group. The aim of these workshops is multiple. They allow the coherence of the information collected in individual interviews to be verified and discussed collectively. Above all, they allow the results of the overall modelling chain (loss of plant material, yield, associated behaviours) to be presented to the experts who were interviewed separately on the different components of the model and to allow them to readjust their assumptions if necessary.
The following topics were discussed using illustrations (Fig. 7):
-
the biophysical processes considered for each component,
-
the ranges of yield loss in function of flood parameter,
-
the determination of impacts for each components in function of flood parameter,
-
the farmers' strategies for crop continuation,
-
the additional or cancelled tasks and as a consequence the variation in crop expenses,
-
the replanting strategies,
-
the list of recovery tasks and their estimated cost (hours of work, equipment).
Each assumption was discussed until all experts agreed to validate them. Following this work, the list of changes to be made was established (Supplement 3) and implemented.
4.3 Axis 3: updatability
In this section, the methodological framework (Table 1) is used to describe the updatability of floodam.agri.
4.3.1 U1: are all the data used in the model and their sources made explicit?
To produce flood damage functions, floodam.agri requires (i) an estimate of usual yields, (ii) an estimate of selling prices, (iii) an estimate of intermediate consumptions and (iv) the physiological stages and crop management sequence. Table 9 lists all the data and their source used in floodam.agri. There is no homogeneous database that provides information on all the technical and economic data of the crops. We had to collect this information from different databases depending on the crop and sometimes complete this information based on expert opinion. It is therefore all the more important to be rigorous about making the data used explicit.
Table 9Data sources.
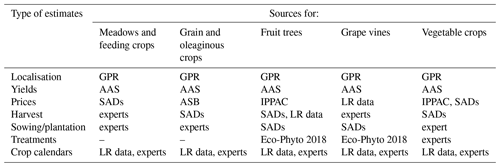
GPR: Graphical Plot Register; AAS: Annual Agricultural Statistics database; SAD: Scales of Agricultural Disasters; ASB: Agricultural Situation Bulletin; IPPAC: Index of Producer Prices of Agricultural Commodities; LR data: technical and economic memento of the main agricultural productions in Languedoc–Roussillon and fact sheets on the Languedoc–Roussillon region.
4.3.2 U2: are the vintages of the data used in the model specified?
The vintage used and the frequency of updates are specified in Table 10. Since the databases used are heterogeneous, the vintages of the databases are also heterogeneous.
4.3.3 U3: are the data used tracked over time?
Table 10 shows the update frequency of the databases used. Updating the data that are published annually is easy. On the other hand, to update data from documents whose publication frequency is not predetermined requires checking for each datum if a new edition has been produced. If not, a validation with experts should be renewed.
To sum up, Tables 9 and 10 show that the updatability of data is not homogeneous. Three modalities can be distinguished:
-
input data come from a single database which tracked over time (e.g. yields),
-
input data come from different databases with different update frequencies (e.g. selling prices and intermediate consumptions),
-
input data come from expert knowledge (e.g. physiological stages).
4.4 Axis 4: transferability
In this section, the methodological framework (Table 1) is used to describe the conditions on transferability.
4.4.1 T1: are the conditions for adaptations, improvements and transfers described?
The possibility to adapt floodam.agri to different contexts was a requirement and has been anticipated in the modelling process. The different steps for adaptation from the simplest to the most demanding are identified according to the differences between the context in which floodam.agri was developed and the context in which it could be transferred. Methodological proposals are made for each of these steps (Fig. 8).
Adjusting damage functions resolution (step 1)
The first possibility of adaptation concerns the compatibility between the flood damage functions produced with floodam.agri and existing hydraulic and hydrological models in terms of resolutions. As the resolution of flood parameters is higher in floodam.agri, it can easily generate flood damage functions with a higher resolution. For example, for the national application, it was proposed to simplify the season parameter and we defined four seasons (Appendix B, Table B1). If hydrological models provide a more precise definition of flood seasonality, given that the time step is the week in floodam.agri, adapted damage functions can be produced.
Adjusting the typology (step 2)
To generate national damage functions, we had to adapt the damage function typology developed in level 3 (Appendix A, Table A1) to make it compatible with the GPR (Appendix A, Table A2). It is possible to adapt this typology and make other crop categories from level 3.
Adjusting to local context (step 3)
This step encompasses two aspects. First, the adjustment of crop technical–economic data (yields or selling prices) requires ensuring that data listed in Sect. 4.3 exist for the study area. The problems encountered in this case may be related to the typology of crops that will have to be adapted too. Second, locally, it will be necessary to adjust the crop calendars (Fig. 6) of each crop. Since the physiological stages have been calibrated on a weekly basis, these calendars can be adapted to a new context on the basis of existing bibliographical and technical data on the area of application and/or on the basis of interviews with agricultural experts, taking care to cover the diversity of crops.
Adjusting sensitivity and decision rules in the case of flooding (step 4)
In the context of application, some biophysical processes or particular behaviours of farmers in cases of flooding that have not been considered in floodam.agri may appear. In this case, it will be necessary to consolidate the modelling (sensitivity and decision rules) with local experts.
Adding a new crop (step 5)
If a crop is to be added to the list of 53 existing crops in floodam.agri, two options should be considered. First, it is necessary to determine whether the crop can be assigned to a vulnerability category. If so, it is necessary to calibrate the physiological stages, crop management sequence, yield and price of the crop. If not, it will be necessary to create a new crop category and to add new sensitivity and decision rules functions. For this, data collection from agricultural experts will be necessary. Moreover, agro-economic data will have to be collected to calibrate the functions.
Taking into account new hazard parameters (step 6)
This is the most demanding level of adaptation because it requires repeating for each crop category all the biophysical processes and the impact on farmers' decisions. This type of transfer necessarily requires work with experts.
4.4.2 T2: has the model been transferred to another context?
To date, some adjustments have been made to the resolutions (step 1) or to the local data (step 2) in the frame of the mandatory CBA of flood management projects. In Mao (2019), an adaptation of the flood damage functions was made at the regional level (step 2) using regional data. Based on Agenais et al. (2013), floodam.agri has been partially transferred to the Italian context (Molinari et al., 2019b; Scorzini et al., 2020), but the way in which the experts' knowledge was collected and formalised for the transfer is not made explicit, particularly with regard to the assumptions made about the processes and behaviours of the farmers. Moreover, work is underway to adapt floodam.agri to coastal flooding (step 6).
5.1 A crucial contribution to the clarification of assumptions
The proposed framework clarifies the components, interactions and decision entities that are or are not considered in the damage assessment model. In economic systems, added value is produced on spatial entities (plots in the agricultural case) and depends on production factors (material, labour, input) and decision rules. In the case of agriculture, the added value increases on the plots and is then stored and transformed in other spatial entities on or off the farm. Nortes Martínez et al. (2020) show the importance of these interactions for avoiding over- and underestimation in damage assessment. Because of the complexity of these mechanisms of localisation of added value in a production chain, the FHRC recommends, in an operational way, not to take into account the indirect effects (Penning-Roswell et al., 2005). However, making the limits of the modelled system explicit remains fundamental in the classification of damage between direct and indirect effects. The larger the system considered, the more it will include effects that could be considered indirect. Developing models that locate and characterise interactions between several components in the field is time consuming. Depending on operational needs, this approach may be required (resilience analysis of a sector affected by a project) or not (large-scale damage assessment on all the issues).
From the modelling experience presented in this article around floodam.agri, the proposed framework concerning the explicitation of assumptions appears to us to be effective for two main reasons. Firstly, the explanation of the assumptions facilitated the collection of information from the experts. Indeed, we found that the logic we proposed to deconstruct the biophysical processes and the decisions made by farmers was consistent with the cognitive approach of damage assessment of the experts. In this sense, the application of the framework reduces the uncertainties surrounding the collection of expert knowledge. Secondly, the explicitness of the assumptions appears to be a necessary condition for the implementation of the other axes, namely validation, updatability and transferability. For example, it is essential to know which processes have been taken into account in determining yield losses. Studies carried out in the context of drainage may only take into account processes such as root asphyxiation, which will be predominant, but in the case of floods with significant velocity effects, it is essential to integrate also the processes of uprooting or laying down. This effort to clarify assumptions is also necessary for capitalisation.
5.2 Consolidate the validation
The proposed framework allows for a clear improvement in the validation methodology with experts involved in the modelling process. However, we are aware of the need to consolidate this aspect. Two avenues are usually identified: first, the comparison of model results with each other; second, the comparison with claims data (Molinari et al., 2019a). A third avenue is to consider the geographical transfer of models as an opportunity to capitalise on expert knowledge by involving new experts and being able to clearly present the modelling assumptions to them. We consider that the clarification of the assumptions is a prerequisite for both avenues and the framework presented here is a step towards the possibility of comparing models with each other. We have made a first proposal in Table C1 based on the existing literature. This should not be considered a result but a discussion support to facilitate exchanges on methods with a view to capitalisation. Concerning the collection of ex post damage data, in particular for the agricultural sector, this is a real challenge that requires a long-term effort. Some interesting initiatives are to be highlighted such as, for example the validation carried out by Chau et al. (2015) or Shrestha et al. (2021). The modelling effort we have carried out to develop floodam.agri has highlighted the importance of acquiring knowledge both on biophysical and human processes in order to be able to assess damage in economic terms. This implies that the data to be collected post-flood in order to validate a model such as floodam.agri must be of different nature, ranging from biophysical impacts (yield loss, mortality of plant material, soil erosion, etc.) to monetary damage, including the chain of behaviours of recovery and continuation of crop management sequence. But this type of post-flood data collection is very time consuming. Most of the time, in large-scale events, the primary objective will be to obtain an overall damage assessment fairly quickly and not to carry out a detailed characterisation of the damage formation processes. In this case, it could be used to estimate damage in monetary terms from hazard parameters. It could also be used to estimate damage in monetary terms from partial post-flood data collection such as yield losses, which corresponds to the practice of the insurance system in France. This type of use would provide a more complete picture of the damage on the basis of the current modelling assumptions, but would not validate or only partially validate the estimated values. On the contrary, such a characterisation makes sense for small-scale events for which, however, various levels of impact can be encountered on an individual scale. In this case, the collection of data allows for validation. For this, the implementation of observatories is an interesting approach.
5.3 Capitalise over time with updatability
The proposed methodological framework requires the specification of all the data used, their source and their vintage. This makes it possible to consider updating the models produced for a given context over time. This is the case for the damage functions produced thanks to floodam.agri. This effort allows us to consider the transfer by comparison of existing databases from one context to another. A difficulty persists for data that are not tracked over time, and in this case we recommend either updating the data on the basis of expert opinions or using a discount rate whose value must be specified.
5.4 Anticipate the transferability to capitalise in space
Transferability needs to be anticipated right from the design stage. We are convinced that process-based models have generic parts that can be transposed and specified in other contexts. The methodological framework has proven useful to describe these aspects and their specification. In particular, we propose a reflection with experts on the basis of vegetative cycles rather than on a monthly basis as was done by Vozinaki et al. (2015) for the evaluation of yield losses due to flash floods in Greece. We believe that this approach has two major advantages. First, discussing biophysical impacts (yield and plant material losses), and decisions to continue cultivation, with experts on this basis fits better with their cognitive approach and reduce uncertainty in data collection. Second, it makes it possible to transfer this method to other contexts, by calibrating vegetative cycles of crops.
5.5 Development prospects around process-based models
The proposed methodological framework also provides a basis for future improvements. In this sense, the explicitness of the assumptions (biophysical processes, decision rules) should not be fixed but should be fed. This suggests the possibility of pooling efforts at an international scale. The tracks of improvement which we consider be a priority concern taking into account (i) other biophysical processes, (ii) agricultural buildings, (iii) breeding systems and (iv) adaptations of the trajectories of farms to floods.
Some biophysical processes such as pollution, salinisation or degradation of soil quality remain scarcely studied and should be consolidated.
For agricultural buildings, a similar approach by breaking down the basic components of the farm building (structure, equipment, input) could be conducted using the model floodam.building (Grelot and Richert, 2019). It will then be necessary to specify the sensitivity and reparation costs of these components with experts. The challenge remains to determine the location associated with the use and technical orientation of buildings, which is not specified in existing databases in France.
Regarding livestock systems, the work carried out by the FHRC is a solid base that should be consolidated by addressing the issue of delayed effects related to the loss of animals as has been integrated through the loss of plant material for crops.
Finally, an important challenge remains to take into account the adaptive capacities of farmers in the long term. Collecting data from agricultural experts who have witnessed flooding on a large number of farms allows us to model a standard behaviour. However, we are aware that this average view does not reflect the diversity of individual vulnerability situations at the farm level. Thus, at the individual scale, decisions, especially those concerning long-term issues such as replanting, will depend on individual parameters such as investment dynamics, the age of the farm manager, the farm's trajectory etc. While it would be possible to assess the economic relevance of certain measures in terms of damage avoided using floodam.agri (e.g. assessment of the damage avoided by establishing a grassland in place of a vineyard), the determinants of these adaptation decisions are much more complex at the level of individuals and in particular farms. Understanding the internal and external determinants of adaptation implementation would require a different approach and investigation at the individual level (Richert et al., 2017).
Process-based flood damage assessment models relying on expert knowledge are widely researched and used operationally. However, it is often observed that this work cannot be capitalised on because the models are too attached to their development context. In this paper, we argue that process-based models, based on a rigorous modelling process, can be suitable for application in different contexts. We show that following a rigorous modelling process can contribute to their capitalisation and transferability. We propose a framework that improves the development of process-based flood damage models by meeting the properties of assumption explicitness, validation, updatability and transferability. We show that respecting these properties could help structure a common modelling effort at the international level.
By applying the proposed methodological framework to floodam.agri, we show that it is possible to describe explicitly the modelling assumptions. Given the complexity of the phenomena (biophysical and decisional processes) and the diversity of the data sources, we argue that the methodological framework is useful for structuring and anticipating from the beginning of the development process a spirit of capitalisation in time and space. This rigorous work is a necessary condition to consider the possibility of improvement in the long term and of cooperation around the development at an international scale. The framework proposed here thus opens up prospects for cooperation in improving and transferring existing models, particularly agricultural models. In terms of research, this work of methodological improvement must be carried out in parallel with the improvement of observation and data collection on the impacts of floods in terms of monetary damage but also with improvement of the understanding of biophysical damage processes, repair decisions and adaptation in the long term.
Different typologies had to be used in the development of floodam.agri. To work with the experts on the sensitivity of the crops, we used the families (level 1), categories (level 2) and subcategories (level 3) described in Table A1.
Level 1 corresponds to five crop families. It brings together 24 categories of crops usually grouped in agronomy. However, this level is not fine enough to define homogeneous damage processes. The crop category (level 2) is the level where damage process is homogeneous. The crop sub-category (level 3) represents a total of 53 crops that can be related to the second level. For instance, winter wheat, barley, and rye are three types of crops that belong to the winter wheat category and to the grain and oleaginous crops family.
Then, we produced the ready-to-use national damage functions by adjusting the typology to be compatible with the Graphic Plot Register (GPR level, Table A2) which is the database for locating agricultural assets based on farmers' declarations to benefit from the European Common Agricultural Policy subsidies.
Table A2Categories of crops in the RPG database, area in flood-prone areas, and maximum damage estimated with floodam.agri.
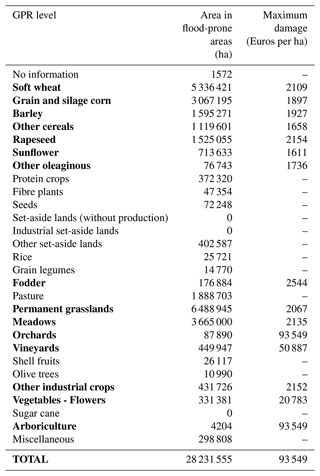
The areas in flood-prone regions were estimated using the approximate potential flood extent (EAIP), which was estimated for the whole country within the frame of the first national flood risk assessment between 2011 and 2017. The maximum values of damage are calculated taking into account all possible combinations of flood parameters. The categories in bold are linked to a damage function produced with floodam.agri.
The resolution of the model is given in Table B1. For the production of the ready-to-use national flood damage functions, groupings were made to give duration classes (Table B2) and to calibrate the four seasons (Table B3.
We present in Table C1 a proposal for using the methodological framework to describe and compare three process-based models for agricultural damage assessment based on the existing literature. Table C1 provides an overview of what is and is not included in the models. For example, it allows us to see that the floodam.agri and AGDAM models could only be compared on cereal crops.
(Mao, 2019)Molinari et al., 2019bScorzini et al., 2020floodam.agri has been implemented in R language is available at http://www.floodam.org/floodam.agri/ (Grelot, 2022) and floodam.building is available at: http://www.floodam.org/floodam.building/ (Grelot et al., 2022) as a package.
The supplement related to this article is available online at: https://doi.org/10.5194/nhess-22-3385-2022-supplement.
FG and PB developed the conceptual damage model. ALA, PB and FG collected expert knowledge. ALA collected secondary data. FG and ALA implemented floodam.agri in R language. ALA and CR wrote a detailed description of the damage model in English and a first draft of the paper. PB proposed the methodological framework detailed in the paper. PB wrote the first complete version that was reviewed by all authors. All authors contributed significantly to the figures.
The contact author has declared that none of the authors has any competing interests.
Publisher's note: Copernicus Publications remains neutral with regard to jurisdictional claims in published maps and institutional affiliations.
This work benefited from the support of the French Ministry of Environment under the funding agreement no. 2200752351. The authors thank the two referees for their reviews that contributed to improving the quality of the paper. We are grateful to Katrin Erdlenbruch for her contribution to the first discussions on farm vulnerability to flooding.
This research has been supported by the French Ministry of Environment (grant no. 2200752351).
This paper was edited by Heidi Kreibich and reviewed by two anonymous referees.
Agenais, A.-L., Grelot, F., Brémond, P., and Erdlenbruch, K.: Dommages des inondations au secteur agricole. Guide méthodologique et fonctions nationales, Groupe de travail national acb inondation, IRSTEA, https://hal.inrae.fr/hal-02600061/file/pub00041499.pdf (last access: 13 October 2022), 2013. a, b
Alfieri, L., Bisselink, B., Dottori, F., Naumann, G., de Roo, A., Salamon, P., Wyser, K., and Feyen, L.: Global projections of river flood risk in a warmer world, Earth's Future, 5, 171–182, https://doi.org/10.1002/2016EF000485, 2017. a, b
Brémond, P.: Caractérisation et évaluation économique de la vulnérabilité des exploitations agricoles aux inondations, Thèse de doctorat, spécialité sciences Économiques, Université de Montpellier 1, Montpellier, France, https://tel.archives-ouvertes.fr/tel-00656442v1 (last access: 13 October 2022), 2011. a, b
Brémond, P. and Grelot, F.: Comparison of a systemic modelling of farm vulnerability and classical methods to appraise flood damage on agricultural activities, in: 11th biennal conference of the International Society for Ecological Economics (ISEE) – Advancing sustainability in a time of crisis, p. 20, https://hal.archives-ouvertes.fr/hal-00615462/file/MO2010-PUB00031884.pdf (last access: 13 October 2022), 2010. a
Brémond, P. and Grelot, F.: Taking into account recovery to assess vulnerability: application to farms exposed to flooding, in: International Congress on Environmental Modelling and Software – Managing Resources of a Limited Planet, edited by: Seppelt, R., Voinov, A. A., Lange, S., and Bankamp, D., IEMSs, p. 9, https://hal.inrae.fr/hal-02598034 (last access: 13 October 2022), 2012. a, b
Brémond, P., Grelot, F., and Agenais, A. L.: Review Article: Economic evaluation of flood damage to agriculture – review and analysis of existing methods, Nat. Hazards Earth Syst. Sci., 13, 2493–2512, https://doi.org/10.5194/nhess-13-2493-2013, 2013. a, b, c, d, e
Browne, M. J.: The Demand for Flood Insurance: Empirical Evidence, J. Risk Uncertain., 20, 291–306, https://doi.org/10.1023/A:1007823631497, 2000. a
Cammerer, H., Thieken, A. H., and Lammel, J.: Adaptability and transferability of flood loss functions in residential areas, Nat. Hazards Earth Syst. Sci., 13, 3063–3081, https://doi.org/10.5194/nhess-13-3063-2013, 2013. a
Chau, V. N., Cassells, S., and Holland, J.: Economic impact upon agricultural production from extreme flood events in Quang Nam, central Vietnam, Nat. Hazards, 75, 1747–1765, https://doi.org/10.1007/s11069-014-1395-x, 2015. a
Comiskey, J. J.: Overview of Flood Damages Prevented by U.S. Army Corps of Engineers Flood Control Reduction Programs and Activities, J. Contemp. Water Res. Educ., 130, 13–19, https://doi.org/10.1111/j.1936-704X.2005.mp130001003.x, 2005. a
Crawford-Brown, D., Syddall, M., Guan, D., Hall, J., Li, J., Jenkins, K., and Beaven, R.: Vulnerability of London's Economy to Climate Change: Sensitivity to Production Loss, J. Environ. Protect., 4, 548–563, 2013. a
Davis, S. A. and Skaggs, L. L.: Catalog of residential depth-damage functions used by the Army Corps of Engineers in flood damage estimation, Tech. Rep. ADA255462, US Army Corps of Engineers, Institute for Water Resources, https://apps.dtic.mil/sti/pdfs/ADA255462.pdf (last access: 13 October 2022), 1992. a, b, c
Dias, P., Arambepola, N., Weerasinghe, K., Weerasinghe, K., Wagenaar, D., Bouwer, L. M., and Gehrels, H.: Development of damage functions for flood risk assessment in the city of Colombo (Sri Lanka), Procedia Eng., 212, 332–339, https://doi.org/10.1016/j.proeng.2018.01.043, 2018. a
Dottori, F., Figueiredo, R., Martina, M. L. V., Molinari, D., and Scorzini, A. R.: INSYDE: a synthetic, probabilistic flood damage model based on explicit cost analysis, Nat. Hazards Earth Syst. Sci., 16, 2577–2591, https://doi.org/10.5194/nhess-16-2577-2016, 2016. a
Durant, D., Kernéïs, E., Meynard, J.-M., Choisis, J.-P., Chataigner, C., Hillaireau, J.-M., and Rossignol, C.: Impact of storm Xynthia in 2010 on coastal agricultural areas: the Saint Laurent de la Prée research farm's experience, J. Coast. Conserv., 22, 1177–1190, https://doi.org/10.1007/s11852-018-0627-8, 2018. a
Erdlenbruch, K., Thoyer, S., Grelot, F., Kast, R., and Enjolras, G.: Risk-sharing policies in the context of the French Flood Prevention Action Programmes, J. Environ. Manage., 91, 363–369, 2009. a, b
Förster, S., Kuhlmann, B., Lindenschmidt, K.-E., and Bronstert, A.: Assessing flood risk for a rural detention area, Nat. Hazards Earth Syst. Sci., 8, 311–322, https://doi.org/10.5194/nhess-8-311-2008, 2008. a
Gerl, T., Kreibich, H., Franco, G., Marechal, D., and Schröter, K.: A Review of Flood Loss Models as Basis for Harmonization and Benchmarking, PLOS ONE, 11, 1–22, https://doi.org/10.1371/journal.pone.0159791, 2016. a, b
Grelot, F.: floodam.agri: Creation of flood damage functions for cultivated plots, R package version 0.1.0.1, floodam.agri [code], http://www.floodam.org/floodam.agri/, last access: 13 October 2022. a
Grelot, F. and Richert, C.: floodam – Modelling Flood Damage functions of buildings – Manual for floodam v1.0.0, Report, IRSTEA, https://hal.inrae.fr/hal-02609309/file/pub00061355.pdf (last access: 13 October 2022), 2019. a, b
Grelot, F., Gontrand, F., Richert, C., Boisgontier, H., and Nortes Martinez, N.: floodam.building: Creation of flood damage functions to buildings, R package version 1.0.0.2, floodam.building [code], http://www.floodam.org/floodam.building/, last access: 13 October 2022. a
Hallegatte, S.: An Adaptive Regional Input-Output Model and its Application to the Assessment of the Economic Cost of Katrina, Risk Anal., 28, 779–799, 2008. a
Hallegatte, S.: Modeling the Role of Inventories and Heterogeneity in the Assessment of the Economic Costs of Natural Disasters, Risk Anal., 34, 152–167, 2014. a
Hattermann, F. F., Huang, S., Burghoff, O., Hoffmann, P., and Kundzewicz, Z. W.: Brief Communication: An update of the article “Modelling flood damages under climate change conditions – a case study for Germany”, Nat. Hazards Earth Syst. Sci., 16, 1617–1622, https://doi.org/10.5194/nhess-16-1617-2016, 2016. a
Hess, T. M. and Morris, J.: Estimating the Value of Flood Alleviation on Agricultural Grassland, Agr. Water Manage. 15, 141–153, 1988. a
Jongman, B., Kreibich, H., Apel, H., Barredo, J. I., Bates, P. D., Feyen, L., Gericke, A., Neal, J., Aerts, J. C. J. H., and Ward, P. J.: Comparative flood damage model assessment: towards a European approach, Nat. Hazards Earth Syst. Sci., 12, 3733–3752, https://doi.org/10.5194/nhess-12-3733-2012, 2012. a, b, c
Jonkman, S. N., Bockarjova, M., Kok, M., and Bernardini, P.: Integrated hydrodynamic and economic modelling of flood damage in the Netherlands, Ecol. Econ., 66, 77–90, 2008. a
Kelman, I. and Spence, R.: An overview of flood actions on buildings, Eng. Geol., 73, 297–309, https://doi.org/10.1016/j.enggeo.2004.01.010, 2004. a
Kreibich, H., Seifert, I., Merz, B., and Thieken, A. H.: Development of FLEMOcs – a new model for the estimation of flood losses in the commercial sector, Hydrolog. Sci. J., 55, 1302–1314, 2010. a
Malgwi, M. B., Schlögl, M., and Keiler, M.: Expert-based versus data-driven flood damage models: A comparative evaluation for data-scarce regions, Int. J. Disast. Risk Reduct., 57, 102148, https://doi.org/10.1016/j.ijdrr.2021.102148, 2021. a, b
Mao, G.: Estimation des coûts économiques des inondations par des approches de type physique sur exposition, Phd thesis, École doctorale Sciences économiques et gestion, Lyon, http://www.theses.fr/2019LYSE1192/document (last access: 13 October 2022), 2019. a, b
Merz, B., Kreibich, H., Schwarze, R., and Thieken, A.: Review article “Assessment of economic flood damage”, Nat. Hazards Earth Syst. Sci., 10, 1697–1724, https://doi.org/10.5194/nhess-10-1697-2010, 2010. a, b, c, d
Meyer, V., Becker, N., Markantonis, V., Schwarze, R., Aerts, J. C. J. H., van den Bergh, J. C. J. M., Bouwer, L. M., Bubeck, P., Ciavola, P., Daniel, V. E., Genovese, E., Green, C. H., Hallegatte, S., Kreibich, H., Lequeux, Q., Lochner, B., Logar, I., Papyrakis, E., Pfurtscheller, C., Poussin, J., Przyluski, V., Thieken, A. H., Thompson, P. M., and Viavattene, C.: Costs of Natural Hazards – A Synthesis, Conhaz – wp09 final report, UFZ, Leipzig, Germany, https://www.ufz.de/export/data/2/122169_CONHAZ_WP09_1_Synthesis_Report_final.pdf (last access: 13 October 2022), 2012. a
Meyer, V., Becker, N., Markantonis, V., Schwarze, R., van den Bergh, J. C. J. M., Bouwer, L. M., Bubeck, P., Ciavola, P., Genovese, E., Green, C., Hallegatte, S., Kreibich, H., Lequeux, Q., Logar, I., Papyrakis, E., Pfurtscheller, C., Poussin, J., Przyluski, V., Thieken, A. H., and Viavattene, C.: Review article: Assessing the costs of natural hazards – state of the art and knowledge gaps, Nat. Hazards Earth Syst. Sci., 13, 1351–1373, https://doi.org/10.5194/nhess-13-1351-2013, 2013. a, b
Middelmann-Fernandes, M. H.: Flood damage estimation beyond stage-damage functions: an Australian example, J. Flood Risk Manage., 3, 88–96, https://doi.org/10.1111/j.1753-318X.2009.01058.x, 2010. a
Molinari, D., De Bruijn, K. M., Castillo-Rodríguez, J. T., Aronica, G. T., and Bouwer, L. M.: Validation of flood risk models: Current practice and possible improvements, Int. J. Disast. Risk Reduct., 33, 441–448, https://doi.org/10.1016/j.ijdrr.2018.10.022, 2019a. a, b
Molinari, D., Scorzini, A. R., Gallazzi, A., and Ballio, F.: AGRIDE-c, a conceptual model for the estimation of flood damage to crops: development and implementation, Nat. Hazards Earth Syst. Sci., 19, 2565–2582, https://doi.org/10.5194/nhess-19-2565-2019, 2019b. a, b, c, d
Molinari, D., Scorzini, A. R., Arrighi, C., Carisi, F., Castelli, F., Domeneghetti, A., Gallazzi, A., Galliani, M., Grelot, F., Kellermann, P., Kreibich, H., Mohor, G. S., Mosimann, M., Natho, S., Richert, C., Schroeter, K., Thieken, A. H., Zischg, A. P., and Ballio, F.: Are flood damage models converging to “reality”? Lessons learnt from a blind test, Nat. Hazards Earth Syst. Sci., 20, 2997–3017, https://doi.org/10.5194/nhess-20-2997-2020, 2020. a, b, c
Morris, J. and Hess, T. M.: Agricultural flood alleviation benefit assessment: A case study, J. Agricult. Econ., 39, 402–412, 1988. a
Morris, J., Tim, H., and Helena, P.: Agriculture's Role in Flood Adaptation and Mitigation, OECD, https://doi.org/10.1787/9789264083578-9-en, 2010. a
Nortes Martínez, D.: Taking into account multi-scale analysis in economic modeling of vulnerability to floods. Study of Cooperative Winery Systems by a Multi-Agent Model, SupAgro Montpellier, https://hal.inrae.fr/tel-03587907/file/19-0003_Nortes_Martinez.pdf, (last access: 13 October 2022), 2019a. a, b
Nortes Martínez, D.: COOPER – Flood impacts over Cooperative Winemaking, Computer program, IRSTEA, https://www.comses.net/codebases/6038/releases/1.0.1/ (last access: 13 October 2022), 2019b. a
Nortes Martínez, D., Grelot, F., Brémond, P., Farolfi, S., and Rouchier, J.: Are interactions important in estimating flood damage to economic entities? The case of wine-making in France, Nat. Hazards Earth Syst. Sci., 21, 3057–3084, https://doi.org/10.5194/nhess-21-3057-2021, 2021. a, b
OCDE: Seine Basin, Île-de-France, 2014: Resilience to Major Floods, OCDE, 204 pp., https://doi.org/10.1787/9789264208728-en, 2014. a
O'Connell, P. E., Ewen, J., O'Donnell, G., and Quinn, P.: Is there a link between agricultural land-use management and flooding?, Hydrol. Earth Syst. Sci., 11, 96–107, https://doi.org/10.5194/hess-11-96-2007, 2007. a
Penning-Roswell, E., Johnson, C., Tunstall, S., Tapsell, S., Morris, J., Chatterton, J., and Green, C.: Appraisal of flood risk management for agriculture, in: The benefits of flood and coastal risk management: a handbook of assessment techniques, Flood Hazard Research Centre, Middlesex University Press, UK, 61–70, ISBN 1904750516, 2005. a, b, c, d
Penning-Rowsell, E. C. and Chatterton, J. B.: The benefits of flood alleviation – A manual of assessment techniques (The Blue Manual), Saxon House, Farnborough, UK, ISBN 056600190X, 1977. a
Penning-Rowsell, E. C., Green, C. H., Thompson, P. M., Coker, A. M., Tunstall, S. M., Richards, C., and Parker, D. J.: The economics of coastal management: a manual of benefit assessment techniques, The Yellow Manual, Belhaven Press, London, UK, ISBN 047194744X, 1992. a
Penning-Rowsell, E. C., Johnson, C. L., Tunstall, S. M., Tapsell, S., Morris, J., Chatterton, J., and Green, C. H.: The Benefits of Flood and Coastal Risk Management: A Handbook of Assessment Techniques, in: Multi Coloured Handbook, Flood Hazard Research Centre, Middlesex University Press, ISBN 1904750516, 2005. a
Penning-Rowsell, E. C., Priest, S. J., Parker, D. J., Morris, J., Tunstall, S. M., Viavattene, C., Chatterton, J., and Owen, D.: Flood and Coastal Erosion Risk Management: A Manual for Economic Appraisal, Routledge, ISBN 9780203066393, https://doi.org/10.4324/9780203066393, 2013. a
Posthumus, H. and Morris, J.: Implications of CAP reform for land management and runoff control in England and Wales, Land Use Policy, 27, 42–50, 2010. a
Posthumus, H., Hewett, C. J. M., Morris, J., and Quinn, P. F.: Agricultural land use and flood risk management: Engaging with stakeholders in North Yorkshire, Agr. Water Manage., 95, 787–798, 2008. a, b
Posthumus, H., Rouquette, J. R., Morris, J., Gowing, D. J. G., and Hess, T. M.: A framework for the assessment of ecosystem goods and services; a case study on lowland floodplains in England, Ecol. Econ., 69, 1510–1523, 2010. a
Priest, S., Viavattene, C., Penning-Rowsell, E., Parker, D., Joyce, J., Morris, J., and Chatterton, J.: Appraisal of Flood Risk Management for Agriculture, in: Flood and coastal erosion risk management, Flood Hazard Research Centre, Middlesex University, p. 12, https://www.mcm-online.co.uk/chapter-9/ (last access: 13 October 2022), 2021a. a, b, c, d
Priest, S., Viavattene, C., Penning-Rowsell, E., Parker, D., Joyce, J., Morris, J., and Chatterton, J.: Handbook for economic appraisal : Flood and coastal erosion risk management, Flood Hazard Research Centre, Middlesex University, https://www.mcm-online.co.uk (last access: 13 October 2022), 2021b. a, b, c, d
Richert, C., Erdlenbruch, K., and Figuières, C.: The determinants of households' flood mitigation decisions in France – on the possibility of feedback effects from past investments, Ecol. Econ., 131, 342–352, https://doi.org/10.1016/j.ecolecon.2016.09.014, 2017. a
Rojas, R., Feyen, L., and Watkiss, P.: Climate change and river floods in the European Union: Socio-economic consequences and the costs and benefits of adaptation, Global Environ. Change, 23, 1737–1751, https://doi.org/10.1016/j.gloenvcha.2013.08.006, 2013. a
Rose, A. and Liao, S. Y.: Modeling Regional Economic Resilience to Disasters: A Computable General Equilibrium Analysis of Water Service Disruptions, J. Reg. Sci., 45, 75–112, 2005. a
Rouchon, D., Peinturier, C., Christin, N., and Nicklaus, D.: Analyse multicritère des projets de prévention des inondations – Guide méthodologique 2018, Report, MTES, 2018. a
Satrapa, L., Fošumpaur, P., Horský, M., Broček, M., and Nešvarová, P.: Posuzování účinnosti akcí protipovodňové ochrany v rámci Činnosti strategického experta programu Prevence před povodněmi v ČR – Assessing the effectiveness of flood protection in the work Expert Strategic Flood Prevention Program in the Czech Republic, Report, Czech Technical University, https://www.vuvh.sk/download/manazmentpovodi_rizik/zbornikprispevkov/konferencia/Prispevky/SekciaB/Satrapa_a_kol.pdf (last access: 13 October 2022), 2012. a
Scorzini, A. R., Di Bacco, M., and Manella, G.: Regional flood risk analysis for agricultural crops: Insights from the implementation of AGRIDE-c in central Italy, Int. J. Disast. Risk Reduct., https://doi.org/10.1016/j.ijdrr.2020.101999, in press, 2020. a, b, c, d
Sébillote, M. and Soler, L. G.: Les processus de décision des agriculteurs, in: Modélisation systémique et système agraire – Déécision et organisation, INRA, Paris, 93–117, ISBN 978-2-7380-0267-9, 1990. a
Shabman, L. and Stephenson, K.: Searching for the Correct Benefit Estimate: Empirical Evidence for an Alternative Perspective, Land Econ., 72, 433–449, 1996. a
Shrestha, B. B., Kawasaki, A., and Zin, W. W.: Development of flood damage functions for agricultural crops and their applicability in regions of Asia, J. Hydrol.: Reg. Stud., 36, 100872, https://doi.org/10.1016/j.ejrh.2021.100872, 2021. a
Thieken, A., Olschewski, A., Kreibich, H., Kobsch, S., and Merz, B.: Development and evaluation of FLEMOps – a new Flood Loss Estimation MOdel for the private sector, in: Flood Recovery, Innovation and Response I, edited by: Proverbs, D., Brebbia, C. A., and Penning-Rowsell, E., WIT Press, 315–324, ISBN 978-1-84564-132-0, 2008a. a
Thieken, A. H., Ackermann, V., Elmer, F., Kreibich, H., Kuhlmann, B., Kunert, U., Maiwald, H., Merz, B., Müller, M., Piroth, K., Schwarz, J., Schwarze, R., Seifert, I., and Seifert, J.: Methods for the evaluation of direct and indirect flood losses, in: RIMAX Contributions at the 4th International Symposium on Flood Defence, Deutsches GeoForschungs Zentrum GFZ, https://gfzpublic.gfz-potsdam.de/pubman/item/item_6063_7/component/file_6064/98_Thieken.pdf?mode=tme (last access: 13 October 2022), 2008b. a
Thieken, A., Kreibich, H., Müller, M., and Lamond, J.: Data Collection for a Better Understanding of What Causes Flood Damage – Experiences with Telephone Surveys, in: chap. 7, AGU – American Geophysical Union, 95–106, https://doi.org/10.1002/9781119217930.ch7, 2017. a
USACE: AGDAM, Agricultural Flood Damage Analysis – User's Manual (Provisionnal), Computer Program Documentation CPD-48, US Army Corps of Engineers, Institute for Water Resources, HEC – Hydrologic Engineering Center, Davis, CA, https://www.hec.usace.army.mil/publications/ComputerProgramDocumentation/AGDAM_UsersManual_(CPD-48).pdf (last access: 13 October 2022), 1985. a, b
Van der Veen, A., Steenge, A E., Bockarjova, M., and Logtmeijer, C. J. J.: Structural economic effects of large scale inundation: a simulation of the Krimpen dike breakage, Tech. rep., University of Twente, Twente, https://ris.utwente.nl/ws/portalfiles/portal/5159901/Veen03structural.pdf (last access: 13 October 2022), 2003. a
van Loenhout, J., Below, R., and McClean, D.: The human cost of disasters: an overview of the last 20 years (2000–2019), Tech. rep., Centre for Research on the Epidemiology of Disasters (CRED) and United Nations Office for Disaster Risk Reduction (UNISDR), https://www.preventionweb.net/files/74124_humancostofdisasters20002019reportu.pdf (last access: 13 October 2022), 2020. a
Vozinaki, Anthi-Eirini, K., Karatzas, G. P., Sibetheros, I. A., and Varouchakis, E. A.: An agricultural flash flood loss estimation methodology: the case study of the Koiliaris basin (Greece), February 2003 flood, Nat. Hazards, 79, 899–920, https://doi.org/10.1007/s11069-015-1882-8, 2015. a, b, c, d
Wagenaar, D., Lüdtke, S., Schröter, K., Bouwer, L. M., and Kreibich, H.: Regional and Temporal Transferability of Multivariable Flood Damage Models, Water Resour. Res., 54, 3688–3703, https://doi.org/10.1029/2017WR022233, 2018. a
Wagenaar, D., Hermawan, T., van den Homberg, M. J. C., Aerts, J. C. J. H., Kreibich, H., de Moel, H., and Bouwer, L. M.: Improved Transferability of Data-Driven Damage Models Through Sample Selection Bias Correction, Risk Anal., 41, 37–55, https://doi.org/10.1111/risa.13575, 2021. a
Xie, W., Li, N., Wu, J.-D., and Liu, X.-Q.: Evaluation of indirect loss from hypothetical catastrophes in two regions with different economic development levels in China, Nat. Hazards Earth Syst. Sci., 12, 3325–3335, https://doi.org/10.5194/nhess-12-3325-2012, 2012. a
Xie, W., Li, N., Wu, J.-D., and Hao, X.-L.: Modeling the economic costs of disasters and recovery: analysis using a dynamic computable general equilibrium model, Nat. Hazards Earth Syst. Sci., 14, 757–772, https://doi.org/10.5194/nhess-14-757-2014, 2014. a
Zandersen, M., Oddershede, J. S., Pedersen, A. B., Nielsen, H. O., and Termansen, M.: Nature Based Solutions for Climate Adaptation – Paying Farmers for Flood Control, Ecol. Econ., 179, 106705, https://doi.org/10.1016/j.ecolecon.2020.106705, 2020. a
- Abstract
- Introduction
- Methodological framework for capitalising on modelling efforts
- Case study: the development of floodam.agri to produce national damage functions for agriculture in France
- Application of the methodological framework to floodam.agri
- Discussion
- Conclusions
- Appendix A: Families and categories of crops considered in floodam.agri
- Appendix B: Resolution of the flood parameters in floodam.agri and categories chosen for the production of national flood damage functions
- Appendix C: Conceptual comparison of three process-based models to estimate agricultural damage
- Code and data availability
- Author contributions
- Competing interests
- Disclaimer
- Acknowledgements
- Financial support
- Review statement
- References
- Supplement
- Abstract
- Introduction
- Methodological framework for capitalising on modelling efforts
- Case study: the development of floodam.agri to produce national damage functions for agriculture in France
- Application of the methodological framework to floodam.agri
- Discussion
- Conclusions
- Appendix A: Families and categories of crops considered in floodam.agri
- Appendix B: Resolution of the flood parameters in floodam.agri and categories chosen for the production of national flood damage functions
- Appendix C: Conceptual comparison of three process-based models to estimate agricultural damage
- Code and data availability
- Author contributions
- Competing interests
- Disclaimer
- Acknowledgements
- Financial support
- Review statement
- References
- Supplement