the Creative Commons Attribution 4.0 License.
the Creative Commons Attribution 4.0 License.
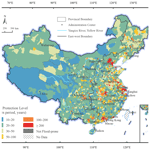
Assessing Chinese flood protection and its social divergence
Dan Wang
Paolo Scussolini
China is one of the most flood-prone countries, and development within floodplains is intensive. However, flood protection levels (FPLs) across the country are mostly unknown, hampering the present assertive efforts on flood risk management. Based on the flood-protection prescriptions contained in the national flood policies, this paper develops a dataset of likely FPLs for China and investigates the protection granted to different demographic groups. The new dataset corresponds to local flood protection designs in 91 (53.2 %) of the 171 validation counties, and in 154 counties (90.1 %) it is very close to the designed FPLs. This suggests that the policy-based FPLs could be a valuable proxy for designed FPLs in China. The FPLs are significantly higher than previously estimated in the FLOPROS (FLOod PROtection Standards) global dataset, suggesting that Chinese flood risk was probably overestimated. Relatively high FPLs (return period of ≥50 years) are seen in 282 or only 12.6 % of the evaluated 2237 counties, which host a majority (55.1 %) of the total exposed population. However, counties with low FPLs (return period of <50 years) host a disproportionate share (52.3 %) of the exposed vulnerable population (children and elders), higher than their share (44.9 %) of the exposed population. These results imply that to reduce social vulnerability and decrease potential casualties, investment in flood risk management should also consider the demographic characteristics of the exposed population.
- Article
(3597 KB) - Full-text XML
-
Supplement
(14605 KB) - BibTeX
- EndNote
Flood protection level (FPL) is the degree to which a flood-prone location is protected against flooding (Scussolini et al., 2016). It is a key determinant of flood risk, making its quantification a prerequisite to reliable risk assessment (Ward et al., 2013). With an increasing focus on large-scale flood risk assessment, which also depends critically on flood protection information (Ward et al., 2017; Alfieri et al., 2017; Winsemius et al., 2018), the necessity of quantifying FPLs has increased in recent years. For example, Jongman et al. (2014) estimated the FPLs in major European river basins by assuming that high-risk areas have high FPLs. Hallegatte et al. (2013) created an FPL dataset for coastal cities by combining design information of flood defenses and expert estimates to improve coastal flood risk assessment. Scussolini et al. (2016) developed FLOPROS (FLOod PROtection Standards), a global database of FPLs based on information included in protection design documents and in protection policy documents, in addition to FPL estimates based on flood risk modeling.
High FPLs reduce the frequency of floods in flood-prone areas and decrease flood risk (Ward et al., 2013). From a cost–benefit view, high FPLs are more economically attractive in areas with a high density of population and economy (Ward et al., 2017). However, high FPLs can have a “levee effect”: creating a sense of security and lowering risk awareness, which boosts floodplain development and population growth and can, in turn, cause catastrophic consequences once a low-probability flood happens (Di Baldassarre et al., 2015; Haer et al., 2020). On the other hand, low FPLs generally mean limited human and financial resources and therefore imply a lower capacity of flood risk reduction (Cheng et al., 2018; Cross, 2001; Han et al., 2020). Moreover, the low FPLs may coincide with a concentration of vulnerable people, e.g., the elders and children, increasing the severity of the human consequences of floods (i.e., more likely fatalities), and more in general exacerbating the local social vulnerability (Birkmann et al., 2016; Gu et al., 2018). Therefore, FPL study is also a key to understand the integrated sociohydrological system.
China is one of the countries that experience the most serious floods and the fastest urbanization. Between 1990 and 2017, floods in China on average affected 149 million people, led to 2165 deaths, and caused economic damage of USD 34 billion per year (Du et al., 2019). Moreover, flood risk changes rapidly due to socioeconomic dynamics (Du et al., 2018) and, in the longer-term, due to climate change (Alfieri et al., 2017; Winsemius et al., 2018). For instance, Du et al. (2018) found that urban lands in the floodplain increased by 26 430 km2, i.e., 542 %, from 1992 to 2015, a process which is still in full swing and thus likely to exacerbate flood risk in the future. Moreover, the urbanization process witnesses an enormous migration from the countryside to cities (Li et al., 2018; Liu and Li, 2017), which selectively leaves the vulnerable population behind and may increase social vulnerability in the countryside (Cheng et al., 2018).
However, little information is available about China's FPLs, which hampers reliable flood risk assessment and challenges risk management. The existing few studies of China's FPLs, to our best knowledge, are only about a specific flood control facility and at local scales. For example, Deng et al. (2015) analyzed the FPL of Taihu Lake levees to inform flood risk management. Zhou (2018) studied the impact of land subsidence on the FPL in lower reaches of the Daqing River. Liu (2017) inferred the FPL in Quzhou through hydrodynamic simulation. Although the aforementioned global FLOPROS database can show an overall FPL for China, it still misses details. For example, in the FLOPROS database the FPLs have a return period of only 20 years in 29 (or 85.3 %) of the 34 Chinese provinces including the capital, Beijing, which is probably incompatible with the massive investment in improving Chinese FPLs in the past decades particularly for metropolises (Du et al., 2019). Therefore, it is reasonable to presume that the FPLs of China are significantly underestimated in the FLOPROS database, especially for urban areas. With such sparse data, the picture of FPLs at the national scale is still unclear, representing a critical knowledge gap in the context of rapid urbanization. This also limits the understanding of the relationship between population exposure, vulnerability, and flood protection.
Therefore, the paper here develops and validates the first FPL dataset for China based on the current Chinese policy on FPLs, the Standard for flood control (standard no. GB 50201-2014), which clearly stated how an area should be protected according to the exposed elements. On this basis, the following questions are addressed. (1) What level of protection against river floods does Chinese policy imply across the country? (2) Since the FPL policy does not consider population demographics, what are the implications for the protection of vulnerable social groups?
2.1 China's flood protection policy and the study framework
FPL data are typically difficult to access at a large scale (Jiang et al., 2020). Scussolini et al. (2016) proposed that FPL can be assessed based on protection design documents and policies or assumed based on hydrodynamic and flood risk simulations and on wealth distribution. Flood protection policies provide an opportunity to establish a large-scale FPL dataset (Mokrech et al., 2015), as they generally contain information on how a region should be protected from floods, although some authors suggest that the actual protection levels could differ from the policy-prescribed protection standard (Jonkman, 2013). Presently, the key policy document for China is the Standard for flood control (standard no. GB 50201-2014), which was released in 2014 by the Ministry of Housing and Urban-Rural Development of the People's Republic of China. It stipulates the FPLs for urban and rural areas depending on three exposure indicators: the amount of exposed population, the per capita gross domestic product (GDP), and the arable lands. Here a framework is developed using spatial information on these indicators of exposure to infer the policy-prescribed FPLs across China (Fig. 1).
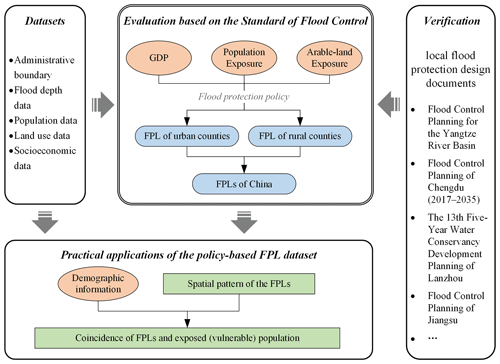
Figure 1The study framework of flood protection level (FPL). Input datasets are indicated in orange circles; the new datasets produced in this study are indicated in blue rounded rectangles; the implications of the new datasets are in green rectangles.
Table 1Urban and rural standards for evaluating the flood protection level (FPL) (source: Standard for flood control, standard no. GB 50201-2014).
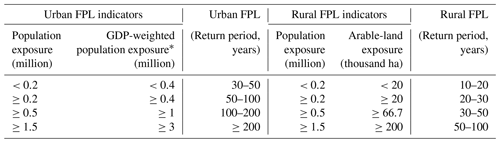
Note: ∗ GDP-weighted population exposure is the population exposure multiplied by the ratio between the relative per capita gross domestic product (GDP) and the national average.
This is conceptually akin to the policy layer of the FLOPROS dataset, but the framework yields information at the much finer spatial scale of the county. In the study framework, based on the relevant datasets, the FPL of an urban county (in Chinese: shi or qu) is evaluated by population exposure and GDP-weighted population exposure; the FPL of a rural county (xian) is evaluated by the population exposure and arable-land exposure (Table 1), as prescribed in the Standard for flood control (standard no. GB 50201-2014). Additionally, local flood protection design documents are collected to verify the policy-based FPL dataset. At the same time, the spatial pattern of the FPLs is identified using spatial statistics techniques, and the FPLs of the exposed population, including the elders, children, and rural and urban population are evaluated by combing the FPLs with demographic information. Note that the vulnerable population is mainly comprised of the elders and children.
2.2 Data
Six datasets are employed. First, an administrative boundary is adopted from He et al. (2016), which considered administrative-boundary adjustments from 1990 to 2010. Second, an undefended fluvial flood depth map with a return period of 100 years is provided by the CIMA (Centro Internazionale in Monitoraggio Ambientale) Research Foundation (Rudari et al., 2015), which is accessible from the Global Risk Data Platform (http://preview.grid.unep.ch/, last access: 19 February 2021). It has a spatial resolution of 1 km and has been used for analyzing China's urban land expansion (Du et al., 2018) and population dynamics in floodplains (Fang et al., 2018). Third, population density maps for 1990 and 2015 are acquired from the China Temporal Datasets of the Harvard Dataverse, published by the WorldPop program (http://www.worldpop.org.uk, last access: 19 February 2021). It originally has a spatial resolution of 100 m and is aggregated to a 1 km resolution to match the flood depth data. Fourth, the demographic information of 1990 and 2015 is obtained from China's national census data (National Bureau of Statistics of China, 2015). It includes the proportions of children (aged ≤14 years) and elders (aged ≥65 years) and the rural and urban population to the county-level total population, which are used to calculate the vulnerable-population exposure. Fifth, the land use data of China for 2015 comes from the Data Center for Resources and Environmental Sciences, Chinese Academy of Sciences, which is accessible from the Resource and Environment Data Cloud Platform (http://www.resdc.cn/, last access: 19 February 2021). It has a resolution of 1 km and is used to extract arable lands in floodplains. Besides, the county-level GDP in 2015 (Division of Urban Social and Economic Survey of National Bureau of Statistics, 2016) is used to calculate the GDP-weighted population exposure.
2.3 Assessment of flood protection level
Three exposure indicators are employed to assess the FPL of a certain flood-prone county: population exposure (PopE), GDP-weighted PopE, and arable-land exposure (ArableE). For county i, the PopE is calculated as the population in the floodplain, which is defined as the maximum extent (i.e., where flood depth is >0 cm) of the undefended 100-year flood map, following previous flood exposure analyses (Du et al., 2018; Fang et al., 2018; Jongman et al., 2012). The calculation is conducted by overlaying the flood depth map and the population density maps using a geographical information system (Fang et al., 2018). Then, for an urban county i, the PopE is transformed into the GDP-weighted PopE using the relative factor of the county's GDP per capita to the national average GDP per capita, following Eq. (1),
where Gi refers to the GDP per capita in county i and Ga refers to the national average of GDP per capita in China.
For a rural county i, the ArableE is calculated as the area of arable lands in the 100-year floodplain by overlaying the flood depth and land use maps. Based on the three calculated indicators, the FPL can be estimated by applying the criteria specified in the Standard for flood control (standard no. GB 50201-2014) (Table 1). The FPL of an urban county is the larger value between FPLs based on the PopE criteria FPL(PopE,i) and based on the GDP-weighted PopE criteria FPL(GDP-weighted PopE,i), while the FPL of a rural county is the larger value between FPL(PopE,i) and the FPL based on the ArableE criteria FPL(ArableE,i), following Eq. (2),
The FPLs are assessed for 2237 counties that fully or partially fall within the 100-year floodplain. The result is presented by six FPLs: ≥200, 100–200, 50–100, 30–50, 20–30, and 10–20 years. Further, FPLs of ≥50 years are summarized as relatively high FPLs, while FPLs of <50 years are summarized as low FPLs.
2.4 Verification of the flood protection levels
Local documents of flood protection design are collected to verify the policy-based FPL results. The validation counties are selected based on the date of the flood protection design: the data should represent the flood protection of the year 2015. A raw sample of 304 counties is found from accessible flood protection documents dating from 1998 to 2019. Two-round selection is further implemented. For the first round, we only select the relatively new documents released from 2007 to 2012, which are neither so old that the FPL information may be outdated nor so new that the FPL may be uncompleted and unqualified. Those documents are kept in the validation data only if they state that the design would be completed between 2010 and 2015. A sample of 110 counties is selected from this round. For the second round, new flood protection design documents starting from 2015 are researched, and these are kept only if they state the current (2015) flood protection standards. Another 61 counties are then selected. Therefore, the validation sample size is 171 counties, representing 7.6 % of the surveyed Chinese counties, 34.0 % of the exposed population, and 13.0 % of Chinese exposed arable lands. Assuming that the designed flood protection standards reflect the reality of flood protection implemented in practice, the agreement between the policy-based FPLs and those derived from the local design documents is checked for the 171 counties, and the overall accuracy is further calculated.
2.5 Pattern clustering of flood protection level
The spatial pattern of FPLs, i.e., the clustering of counties with a homogenous FPL, is essential to understand regional flood risk distribution. It also informs on indirect dimensions of flood risk, as, e.g., high-FPL counties may suffer indirect flood risk if their surrounding counties have low FPLs and thus suffer severe flooding (Cheng et al., 2018; Han et al., 2020). The spatial pattern of the FPL data is then identified using the LISA (local indicator of spatial association) or local Moran's I (Anselin, 1995). The local Moran's I statistic is calculated as follows (Chakravorty et al., 2003):
where Ii is the local Moran's I in county i; xi and xj refer to the FPL of county i and its neighboring county j, respectively; is the mean FPL across all counties; Wi,j is a n-by-n weight matrix defining the spatial contiguity between county i and any county j, where if county i and county j share a border and otherwise ; and s2 is the variance of FPLs across all flood-prone counties.
A positive value for the local Moran's I statistic indicates that the FPL in a county is similar to those in its neighboring counties, while a negative I value indicates dissimilar values (Zhu et al., 2018; Frigerio et al., 2018; Shen et al., 2019). The local Moran's I is calculated by applying the queen contiguity matrix in the GeoDa software program (version 1.12), which is available from http://geodacenter.github.io (last access: 19 February 2021). The significance is evaluated at an alpha level of 0.05. Four different LISA clustering patterns of FPLs are identified. (1) High–high: both the county and its neighbors have high FPLs. (2) High–low: the FPL is high in a county, while it is low in its neighboring counties. (3) Low–high: FPL is low in a county, while it is high in its neighboring counties. (4) Low–low: both a county and its neighbors have low FPLs.
2.6 Dynamic analysis of population exposure and vulnerable-population exposure
PopE refers to the population exposure in a certain county, which is calculated as the population in the floodplain by overlaying the flood depth and the population density maps (Sect. 2.3). The exposed vulnerable population comprises the exposed children and elders because children and elders are generally considered more vulnerable to flooding, due to limited mobility and physical resistance (Gu et al., 2018; Salvati et al., 2018). Assuming that the proportion of exposed children to the total population is spatially homogeneous within a county, the exposed children are calculated in each county using Eq. (4),
where PopE refers to the population exposure in the county and children and total population are respectively the numbers of children and total population in a county. Similarly, the exposed elders can be calculated. The exposed vulnerable population is the sum of exposed children and exposed elders. Additionally, the exposed rural and urban population are estimated following the method.
Equation (5) is used to calculate the growth rate of population exposure from 1990 to 2015,
where PopE2015 and PopE1990 refer to the population exposure in 2015 and 1990, respectively. Similarly, the growth rates of exposed children, elders, and the vulnerable population are calculated, as well as the growth rates of the exposed rural and urban population.
3.1 Validation of the new policy-based FPL dataset
The policy-based FPL dataset matches to a good degree the information from protection design documents. In 91 (53.2 %) out of the 171 verification counties, the FPLs agree with the local official protection design documents (Tables S1 and S2 in the Supplement). The FPLs in the dataset are overestimated in 20 counties (11.7 %) and underestimated in 60 counties (35.1 %). Most (90.1 %) of the overestimations and underestimations are only off by one FPL. In comparison, the FPLs of the global FLOPROS database match the protection design documents in 50 (29.2 %) out of the 171 counties and underestimate the FPLs in almost all other counties, with 32.7 % of the underestimations being off by only one FPL. Therefore, the policy-based FPL dataset constitutes a substantial improvement on previous knowledge of Chinese FPLs.
3.2 Spatial pattern of flood protection level
According to the prescriptions of the Standard for flood control (standard no. GB 50201-2014) policy, a majority (87.4 % or 1955) of Chinese counties have FPLs of <50 years that are defined hereafter as relatively low FPLs (Fig. 2), while only 282 counties (12.6 %) have high FPLs (≥50 years). A considerable proportion (33.1 % or 741) of the evaluated Chinese counties are protected with an FPL of ≥30 years, which is much higher than that in the global FLOPROS database (Scussolini et al., 2016), in which only 5 (14.7 %) out of 34 provinces have FPLs of ≥30 years. Therefore, Chinese FPLs are probably underestimated in the previous study by Scussolini et al. (2016).
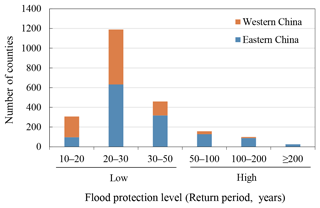
Figure 2The number of counties with different flood protection levels. (The map of western and eastern China is shown in Fig. 3).
The FPLs show significant divergence between eastern and western China (Figs. 2 and 3), reflecting general differences in population exposure and in economic performance. A dominant portion (85.5 % or 241) of high-FPL counties are located in eastern China. Particularly, all the 25 counties with the highest FPL (≥200 years) are located in the east. In contrast, only 4.3 % (or 41) of the western Chinese counties have high FPLs, which is much lower than the share in eastern China (18.7 % or 241). The majority (68.4 % or 210) of the lowest-FPL counties (10–20 years) are located in the west. In sum, western China is disproportionally protected with low FPLs.
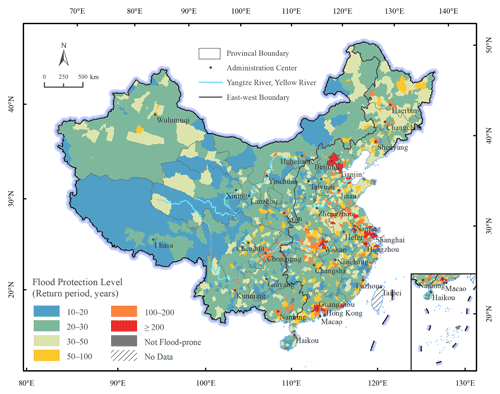
Figure 3Flood protection levels (FPLs) for the study area. The FPL is limited to the scope of floodplains but plotted to cover all counties. The data should only be viewed as a proxy of the designed FPLs and do not equate to the actual FPLs. The Shapefile format data are available in the Supplement.
High–high FPL clusters include 112 counties. They are mainly located in the three primary urban agglomerations of Beijing–Tianjin–Hebei, the Yangtze River Delta, and the Pearl River Delta (Fig. 4). The three primary urban agglomerations are home to most of the counties with the highest FPLs of ≥200 years (Fig. 3). Besides, the high–high FPL clusters are also located in the middle Yangtze River reaches. The low–high FPL clusters include a total of 66 counties, surrounding the high–high FPL clusters. These counties within the low–high FPL clusters can be vulnerable to floods when they are needed to sacrifice to protect their surrounding large cities that are more expensive to be flooded (Wang et al., 2016). For instance, in China, flood detention zones are planned in rural areas to protect surrounding cities in the Yangtze River and Huai River basins of China (Du et al., 2021). Low–low FPL clusters include 158 counties, which are mainly located in southwestern China and scattered along a belt from Hohhot to Kunming. Surrounding the low–low FPL clusters, 48 counties have relatively high FPLs and form high–low clusters.
3.3 Protection levels of the exposed (vulnerable) population in 2015
A majority (55.1 % or 231.1 million) of the total exposed population is found in a minority of 282 counties with high FPLs (≥50 years) (Table 2). Particularly, 23.3 % (97.8 million) of the total exposed population are protected by an FPL of ≥200 years. In contrast, 188.4 million (44.9 %) of the flood-exposed people are in low-FPL counties, lower than that in the high-FPL counties.
A majority (52.3 % or 38.3 million) of the exposed vulnerable population is concentrated in low-FPL (<50 years) counties, higher than these counties' share of the total exposed population (44.9 %) (Table 2). These low-FPL counties host 52.9 % (19.4 million) of the exposed children and 51.6 % (18.8 million) of the exposed elders. Particularly, counties with an FPL of 20–30 years host the largest exposed vulnerable population (19.9 million or 27.1 %) across all the six FPLs, including 10.1 million (27.5 %) children and 9.8 million (26.8 %) elders.
The ratio of the vulnerable population to the total exposed population is as high as 20.3 % in low-FPL counties, while it is 15.1 % in high-FPL counties (Table 2). Both exposed children and elders are found disproportionally in the low-FPL counties. Specifically, the children's share of the total exposed population is 10.3 % in the low-FPL counties, higher than in the high-FPL counties (7.5 %); similarly, the elders' share is 10.0 % in the low-FPL counties, higher than in the high-FPL counties (7.6 %). Therefore, the low-FPL counties have a disproportionally higher share of the vulnerable population than the high-FPL counterparts, in terms of both exposed children and elders.
Table 2Exposed population (total, vulnerable, children, and elders) for each flood protection level (FPL), in absolute amounts and as percentage of the whole exposed population. The rightmost column reports the ratio of the vulnerable to the total exposed population.
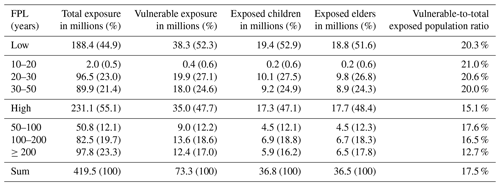
The protection divergence also happens between the exposed rural and urban population. A majority (66.8 % or 107.3 million) of the exposed rural population are protected by low FPLs, while this proportion is only 31.3 % (81.1 million) for the exposed urban population (Table S3 in the Supplement). This implies that the rural people are not equally protected against flooding compared with urban inhabitants.
3.4 Changes in the exposed (vulnerable) population across protection levels
The total exposed population has grown by 60.3 %, rapidly from 1990 to 2015 in counties that are presently protected by high FPLs, while it has remained relatively stable in the low-FPL counties (2.34 %) (Fig. 5a). In 1990, the exposed population was primarily located in counties with FPLs of 20–30 years (95.0 million or 28.9 %), while in 2015 it is primarily in counties with ≥200 years FPLs (97.8 million or 23.3 %).
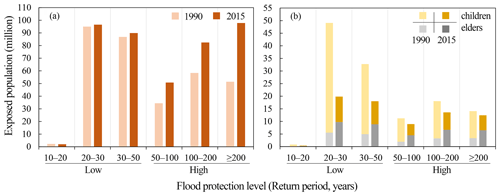
Figure 5Changes in exposed total population (a) and vulnerable population (b) across different flood protection levels from 1990 to 2015.
The exposed vulnerable population has decreased by 41.9 %, from 126.0 million in 1990 to 73.3 million in 2015, and decreased more sharply (by 53.7 %) in the low-FPL counties (Fig. 5b). The decrease of the exposed vulnerable population is mainly caused by a sharply declining exposed population of children. The exposed children, in total, have decreased by 65.6 % from 106.8 million in 1990 to 36.8 million in 2015. The exposed children's share to the total exposed population has declined rapidly across all FPLs, which decreases the ratio of the vulnerable population to the total exposed from 38.4 % in 1990 to 17.5 % in 2015 (Fig. 6).
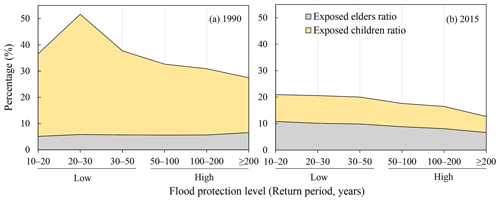
Figure 6The ratios of the exposed vulnerable population, exposed children, and exposed elders to the total exposed population across different flood protection levels in 1990 (a) and 2015 (b). Note that flood protection levels refer to the situation of 2015 and keep constant between 1990 and 2015.
In contrast, the exposed elders have increased across all six FPLs, with a total growth of 90.2 % from 19.2 million to 36.5 million (Fig. 5b). This trend reflects China's aging population. Moreover, the elders' share of the total exposed population has risen from 5.9 % in 1990 to 8.7 % in 2015 (Fig. 6). Particularly in the low-FPL counties, it has increased from 5.7 % in 1990 to 10.0 % in 2015 with a growth of 4.3 %, much higher than that in the high-FPL counties (1.7 %).
The exposed rural population has decreased by 26.5 % from 218.6 million in 1990 to 160.7 million in 2015 and has decreased more (30.1 %) in the low-FPL counties (Table S3). However, there is a consistent disproportional distribution of the rural population in low-FPL counties. Meanwhile, the exposed urban population (177.7 million) of the high-FPL counties in 2015 is more than twice of that in 1990 (79.2 million). However, the low-FPL areas also witness a rapid increase in exposed urban population by 68.5 %.
4.1 Residual flood risk is nonstationary and should be effectively managed
Chinese FPLs should be much higher than that in previous studies, according to the prescriptions of the Standard for flood control (standard no. GB 50201-2014) policy. The newly developed data show that almost one-third (33.1 %, 741) of the evaluated Chinese counties should be protected with an FPL of ≥30 years, while this FPL is only in 5 (14.7 %) out of 34 provinces in the FLOPROS database (Scussolini et al., 2016). Particularly, the newly developed data show that a considerable proportion (12.6 % or 282) of Chinese counties have FPLs of ≥50 years that are defined as relatively high FPLs. Moreover, the high FPLs protect the majority (55.1 % or 231.1 million) of the exposed Chinese population. Those high-FPL counties are concentrated in eastern China, particularly in the urban agglomerations (Bai et al., 2014). The underestimation of Chinese FPLs can at least partially explain the high level of flood risk in previous studies (Alfieri et al., 2017; Willner et al., 2018). For instance, global flood risk assessments show huge flood risk across Chinese provinces both in current conditions and future scenarios (Willner et al., 2018), which are considered to further propagate a devastating indirect impact to other countries through the global trade and supply network (Willner et al., 2018). However, those global assessments are based on the FLOPROS database, which is significantly lower than the policy-required FPLs as indicated by the paired sample t test (p<0.01, Table S4 in the Supplement). For instance, FLOPROS presented Beijing with an FPL of 20 years, while it should be 200 years according to the Chinese protection policy (Table S1). The real flood risk should thus be much lower than the estimates in previous studies if the new FPL is considered.
However, high-level flood protection does not represent absolute safety. On the contrary, low-probability floods can still occur, and flood protection structures may technically fail, causing residual flood risk (Haer et al., 2020). Particularly, levee breaches can cause a catastrophe for the areas with a high density of population and assets (Jongman, 2018). In high-FPL counties, a sense of safety brought by the flood protection structures can reduce the perception of risk and cause the levee effect – boosting floodplain development and increasing flood exposure (Cheng and Li, 2015; Kates et al., 2006). The possibility of a similar outcome should be considered in China, as suggested by the faster increasing trend of the exposed population in the high-FPL counties than in the low-FPL counties. The rapid increase in the exposed population can exacerbate residual flood risk, rendering these high-FPL areas vulnerable to low-probability and high-impact floods (Koks et al., 2015; Di Baldassarre et al., 2013). The residual risk can be further aggravated by future climate change (Alfieri et al., 2017; Winsemius et al., 2018). Alfieri et al. (2017) indicated that the future annual expected economic losses in China may be the highest of all countries, rising by 1.5-fold to 3.4-fold and reaching EUR 50 billion to 110 billion per year, based on global warming scenarios of 1.5 to 4∘, respectively.
The residual flood risk can be higher if the real-world flood protection lags behind the policy requirement and design. In fact, the policy-based FPL dataset only reflects how a county should be protected according to the flood protection policy, which also stipulates that the flood protection should be updated along with population growth and economic development. Unfortunately, a survey in 2013 found that 44 % (284) of the 642 Chinese cities did not update their flood protection planning according to their socioeconomic growth (Cheng and Li, 2015). A neglect of the real-world flood protection lagging behind the policy-based flood protection can distort the selection of adaptation measures.
Flood risk management will inevitably face an ongoing challenge from population growth when reducing the residual risk. It is predicted that, in the next 10 years, the Chinese urban population will increase by 17 % (United Nations, 2018), which will increase residual flood risk in the high-FPL counties because high-FPL counties are usually urban areas. The flood protection structures should be upgraded along with socioeconomic development and climate change to keep the residual flood risk to an acceptable level (Kwadijk et al., 2010). Non-structural measures such as early warning systems, land use planning, building codes, and insurance and reinsurance can be a complement to the flood protection structures for effectively managing flood risk (Aerts et al., 2014; Jongman, 2018; Du et al., 2020).
4.2 Demographics should be included in the flood protection policy
Although the low-FPL counties see a lower exposed population, the majority (52.3 % or 38 million) of exposed vulnerable population are concentrated there. Particularly, the elders' share of the total exposed population was increasing rapidly in these low-FPL counties. These findings are consistent with other studies (Cheng et al., 2018). Meanwhile, a great number of exposed rural people are found to be concentrated in these low-FPL counties. The low-FPL counties located in rural areas have experienced an economic downturn and have insufficient job opportunities, which causes a large number of young adults to temporarily migrate to cities for work opportunities (He et al., 2016; Meng, 2014). Therefore, it may be difficult for these low-FPL counties to respond to and recover from flooding due to economic backwardness and labor shortages.
Hence with more vulnerable people, a higher potential casualty rate caused by floods is expected in these low-FPL counties. The elder Chinese population is predicted to more than double from 128 million in 2015 to 348 million in 2050 (The World Bank, 2019), implying an increase of exposed elders. This will further increase social vulnerability and challenge flood risk management, particularly in the low-FPL counties. However, the Standard for flood control (standard no. GB 50201-2014) policy neglects the demographic characteristics of the exposed population. It is economically reasonable to employ a relatively low FPL for areas that have a low density of population and economy. Such a strategy, however, may aggravate flood risk because the less protected areas coincide with the high social vulnerability that is caused by a disproportional distribution of vulnerable people, particularly elders.
Therefore, local demographic characteristics should be considered for an economically and socially beneficial strategy of flood adaptation (Koks et al., 2015). The low-FPL areas can employ decentralized and soft adaptation measures, such as elevation of buildings, wet flood proofing, and dry flood proofing to reduce flood vulnerability (Aerts, 2018; Du et al., 2020), since structural flood protections are generally less cost-effective in areas with a lower exposed population (Ward et al., 2017; Jongman, 2018). Considering the relative concentration of exposed vulnerable population in the low-FPL counties, flood risk information, adaptation measures, and emergency plans should be made accessible and understandable to children and elders (De Boer et al., 2014). Communities should pay more attention to children and elders during early warning, evacuation, and resettlement periods, and a one-on-one assistance scheme can be developed at the community level to help the vulnerable people. Emergency planning and flood adaptation design should consider the particular needs of children and elders, which can be promoted by their participation in the planning and designing processes (Liang et al., 2017).
4.3 Limitations and future perspectives
The newly developed FPL dataset reflects how China should be protected against river floods according to the flood protection policy. It does not report actual FPLs, although it generally agrees with local flood protection design documents. Given the scarcity of the real-world flood protection data, the new dataset could be considered a valuable proxy of designed FPLs and can assist efforts to understand, evaluate, and manage flood risk in the following ways. (1) Authorities can use this database to check if the relevant counties are protected properly. (2) Flood risk assessment could be further conducted considering the developed FPL dataset. (3) The policy-based FPLs can be an important foundation for relevant researchers to develop a more reliable FPL dataset of China and the world. (4) It can also help to reveal potential social divergence by combining the policy-based FPLs with some social data, which can further improve the flood protection policy, as indicated by the relevant analyses in this study. It is worth noting that the real-world FPLs are not fixed but are plausibly updated along with socioeconomic development and climate change. Therefore, relevant departments, communities, and users are invited to use, verify, and improve the newly developed FPL result (which is accessible in the Supplement in Shapefile format). With the wide participation of public stakeholders, the flood protection data can be much improved in the future.
Limitations also come with our data and methods. The exposed population is calculated based on a gridded population dataset from the WorldPop program, which is a disaggregation result of census population using auxiliary variables such as land use conditions and nightlight brightness. However, neither the disaggregation methods nor the auxiliary data are free of uncertainty and error (Smith et al., 2019). Moreover, due to a lack of gridded demographic data, the exposed vulnerable population is calculated assuming that its proportion to the total population is spatially homogeneous within a county. In fact, the demographic characteristics can be spatially heterogeneous (Han et al., 2007; Qiang, 2019). Nowadays, crowd-sourcing population data are emerging, thanks to social media (Goodchild and Glennon, 2010; Smith et al., 2019) and mobile phone records (Wu et al., 2012). These new data can help to improve the exposed (vulnerable) population accuracy and in turn, the FPL estimates. Meanwhile, it should be noted that Sect. 3.4 only analyzed changes in the exposed (vulnerable) population across the current protection levels, which was not equal to the exposure and vulnerability changes along with different-period FPLs. Besides, only some aspects of social divergence have been analyzed, due to data limitation. Under the FPL policy, other factors of social divergence, such as sex, income, and education level (Tian and Lemos, 2018; Huang et al., 2020), need to be further studied. For example, the education levels across different FPLs may help relevant authorities to develop suitable flood adaptation strategies or help researchers to further analyze the FPL policy's influence on social divergence.
A framework is developed to assess the county-level FPLs in China based on the flood protection policy and relevant socioeconomic variables of floodplains. The produced FPL dataset shows a match ratio of 53.2 % with the designed FPLs included in specific flood protection documents in a sample of 171 counties. The policy-based FPL dataset constitutes a substantial improvement on previous knowledge, and the dataset is relatively accurate. This study thus agrees with the argument of Scussolini et al. (2016) that flood protection policy could be a valuable proxy for FPLs. However, there still may be significant differences between the policy-based FPLs and the actual flood protection because the latter may be behind or ahead of the policy-required FPLs. The FPL dataset was thus made open access to encourage relevant users to check and improve it.
The produced FPL dataset shows that western China is dominated by low FPLs, while high-FPL counties are concentrated in the east. There are 282 counties with a high FPL (≥50 years), which account for only 12.6 % of the total flood-prone counties but host 55.1 % (231.1 million) of the total exposed population. In contrast, more of the exposed vulnerable population (52.3 % or 38 million) are concentrated in the low-FPL counties. Moreover, exposed population grows rapidly (by 60.3 %) in the high-FPL counties, while the proportion of elders increases more rapidly in the low-FPL counties than in the high-FPL counties. These findings imply that the flood protection policy has a relatively efficient strategy to protect the majority of the exposed population within a minority of well-protected counties. However, the rapid growth of the exposed population can increase residual flood risk. Moreover, the disproportional concentration and rapid increase of the exposed vulnerable population, particularly the elders, in the low-FPL counties can probably increase the places' vulnerability.
Therefore, diversified adaptation measures including both structural flood defenses and non-structural solutions should be employed to reduce flood risk in both the high- and low-FPL counties. Local demographic characteristics should be considered for an economically and socially beneficial strategy of flood adaptation. Particularly, the vulnerable population in the low-FPL counties should receive dedicated attention. This study shows that combining FPLs and demographic information is critical to understand and manage flood risk.
The Chinese flood protection data are available in the Supplement. Supporting data are accessible through the associated references.
The supplement related to this article is available online at: https://doi.org/10.5194/nhess-21-743-2021-supplement.
SD designed this study. DW implemented data processing and analysis. DW, PS, and SD prepared the paper with contributions from all co-authors.
The authors declare that they have no conflict of interest.
This research has been supported by the National Natural Science Foundation of China (grant nos. 41871200, 41730642, and 51761135024), the National Key Research and Development Program of China (grant no. 2017YFC1503001), and the Netherlands Organisation for Scientific Research (grant no. ALWOP.164).
This paper was edited by Heidi Kreibich and reviewed by three anonymous referees.
Aerts, J.: A Review of Cost Estimates for Flood Adaptation, Water, 10, 33, https://doi.org/10.3390/w10111646, 2018.
Aerts, J. C. J. H., Botzen, W. J. W., Emanuel, K., Lin, N., de Moel, H., and Michel-Kerjan, E. O.: Evaluating Flood Resilience Strategies for Coastal Megacities, Science, 344, 472–474, https://doi.org/10.1126/science.1248222, 2014.
Alfieri, L., Bisselink, B., Dottori, F., Naumann, G., de Roo, A., Salamon, P., Wyser, K., and Feyen, L.: Global projections of river flood risk in a warmer world, Earths Future, 5, 171–182, https://doi.org/10.1002/2016ef000485, 2017.
Anselin, L.: Local Indicators of Spatial Association – LISA, Geogr. Anal., 27, 93–115, https://doi.org/10.1111/j.1538-4632.1995.tb00338.x, 1995.
Bai, X., Shi, P., and Liu, Y.: Realizing China's urban dream, Nature, 509, 158–160, https://doi.org/10.1038/509158a, 2014.
Birkmann, J., Welle, T., Solecki, W., Lwasa, S., and Garschagen, M.: Boost resilience of small and mid-sized cities, Nature, 537, 605–608, https://doi.org/10.1038/537605a, 2016.
Chakravorty, S., Koo, J., and Lall, S. V.: Metropolitan industrial clusters; patterns and processes, the World Bank, the United States, 2003.
Cheng, X. and Li, C.: The Evolution Trend, Key Features and Countermeasures of Urban Flood Risk, China Flood & Drought Management, 25, 6–9, https://doi.org/10.16867/j.cnki.cfdm.2015.03.002, 2015.
Cheng, X., Wan, H., Huang, S., Li, C., and Zhang, H.: Lessons learned from the 2016 Yangtze River flood in Anhui province, China, International Journal of River Basin Management, 16, 307–314, https://doi.org/10.1080/15715124.2018.1437741, 2018.
Cross, J. A.: Megacities and small towns: different perspectives on hazard vulnerability, Global Environmental Change Part B: Environmental Hazards, 3, 63–80, https://doi.org/10.1016/S1464-2867(01)00020-1, 2001.
De Boer, J., Botzen, W. J. W., and Terpstra, T.: Improving Flood Risk Communication by Focusing on Prevention-Focused Motivation, Risk Anal., 34, 309–322, https://doi.org/10.1111/risa.12091, 2014.
Deng, Y., Wu, H., Sun, D., and Zhao, Z.: Flood protection capacity analysis on levee of Taihu Lake, China Flood Drought Management, 25, 72–75, https://doi.org/10.16867/j.cnki.cfdm.2015.05.022, 2015.
Di Baldassarre, G., Viglione, A., Carr, G., Kuil, L., Salinas, J. L., and Blöschl, G.: Socio-hydrology: conceptualising human-flood interactions, Hydrol. Earth Syst. Sci., 17, 3295–3303, https://doi.org/10.5194/hess-17-3295-2013, 2013.
Di Baldassarre, G., Viglione, A., Carr, G., Kuil, L., Yan, K., Brandimarte, L., and Bloeschl, G.: DebatesPerspectives on socio-hydrology: Capturing feedbacks between physical and social processes, Water Resour. Res., 51, 4770–4781, https://doi.org/10.1002/2014wr016416, 2015.
Division of Urban Social and Economic Survey of National Bureau of Statistics: Statistical Yearbook of Chinese Cities, China Statistical Press, Beijing, 2016.
Du, S., Cheng, X., Huang, Q., Chen, R., Ward, P. J., and Aerts, J. C. J. H.: Brief communication: Rethinking the 1998 China floods to prepare for a nonstationary future, Nat. Hazards Earth Syst. Sci., 19, 715–719, https://doi.org/10.5194/nhess-19-715-2019, 2019.
Du, S., Scussolini, P., Ward, P. J., Zhang, M., Wen, J., Wang, L., Koks, E., Diaz-Loaiza, A., Gao, J., Ke, Q., and Aerts, J. C. J. H.: Hard or soft flood adaptation? Advantages of a hybrid strategy for Shanghai, Global Environ. Chang., 61, 102037, https://doi.org/10.1016/j.gloenvcha.2020.102037, 2020.
Du, S., Shen, J., Fang, J., Fang, J., Liu, W., Wen, J., Huang, X., and Chen, S.: Policy delivery gaps in the land-based flood risk management in China: A wider partnership is needed, Environ. Sci. Policy, 116, 128–135, https://doi.org/10.1016/j.envsci.2020.11.005, 2021.
Du, S. Q., He, C. Y., Huang, Q. X., and Shi, P. J.: How did the urban land in floodplains distribute and expand in China from 1992–2015?, Environ. Res. Lett., 13, 034018, https://doi.org/10.1088/1748-9326/aaac07, 2018.
Fang, Y. Q., Du, S. Q., Scussolini, P., Wen, J. H., He, C. Y., Huang, Q. X., and Gao, J.: Rapid Population Growth in Chinese Floodplains from 1990 to 2015, Int. J. Env. Res. Pub. He., 15, 1602, https://doi.org/10.3390/ijerph15081602, 2018.
Frigerio, I., Carnelli, F., Cabinio, M., and De Amicis, M.: Spatiotemporal Pattern of Social Vulnerability in Italy, Int. J. Disast. Risk Sc., 9, 249–262, https://doi.org/10.1007/s13753-018-0168-7, 2018.
Goodchild, M. F. and Glennon, J. A.: Crowdsourcing geographic information for disaster response: a research frontier, Int. J. Digit. Earth, 3, 231–241, https://doi.org/10.1080/17538941003759255, 2010.
Gu, H., Du, S., Liao, B., Wen, J., Wang, C., Chen, R., and Chen, B.: A hierarchical pattern of urban social vulnerability in Shanghai, China and its implications for risk management, Sustain. Cities Soc., 41, 170–179, https://doi.org/10.1016/j.scs.2018.05.047, 2018.
Haer, T., Husby, T. G., Botzen, W. J. W., and Aerts, J. C. J. H.: The safe development paradox: An agent-based model for flood risk under climate change in the European Union, Global Environ. Chang., 60, 102009, https://doi.org/10.1016/j.gloenvcha.2019.102009, 2020.
Hallegatte, S., Green, C., Nicholls, R. J., and Corfee-Morlot, J.: Future flood losses in major coastal cities, Nat. Clim. Change, 3, 802–806, https://doi.org/10.1038/nclimate1979, 2013.
Han, J., Zhang, Z., and Qi, Q.: Analysis and Visualization of the Uneven Distribution of Population in China, Geo-Information Science, 9, 14–19, https://doi.org/10.3969/j.issn.1560-8999.2007.06.003, 2007.
Han, Y. P., Huang, Q. X., He, C. Y., Fang, Y. Q., Wen, J. H., Gao, J., and Du, S. Q.: The growth mode of built-up land in floodplains and its impacts on flood vulnerability, Sci. Total Environ., 700, 134462, https://doi.org/10.1016/j.scitotenv.2019.134462, 2020.
He, C., Huang, Q., Dou, Y., Wei, T., and Liu, J.: The population in China's earthquake-prone areas has increased by over 32 million along with rapid urbanization, Environ. Res. Lett., 11, 074028, https://doi.org/10.1088/1748-9326/aa5dec, 2016.
Huang, Q., Yin, D., He, C., Yan, J., Liu, Z., Meng, S., Ren, Q., Zhao, R., and Inostroza, L.: Linking ecosystem services and subjective well-being in rapidly urbanizing watersheds: Insights from a multilevel linear model, Ecosyst. Serv., 43, 101106, https://doi.org/10.1016/j.ecoser.2020.101106, 2020.
Jiang, Y., Zhi, Y., Zhao, H., Liang, L., Cao, Y., and Gu, J.: Research status and prospects on water conservancy big data, Journal of Hydroelectric Engineering, 39, 1–32, https://doi.org/10.11660/slfdxb.20201001, 2020.
Jongman, B.: Effective adaptation to rising flood risk COMMENT, Nat. Commun., 9, 1986, https://doi.org/10.1038/s41467-018-04396-1, 2018.
Jongman, B., Ward, P. J., and Aerts, J. C. J. H.: Global exposure to river and coastal flooding: Long term trends and changes, Global Environ. Chang., 22, 823–835, https://doi.org/10.1016/j.gloenvcha.2012.07.004, 2012.
Jongman, B., Hochrainer-Stigler, S., Feyen, L., Aerts, J., Mechler, R., Botzen, W. J. W., Bouwer, L. M., Pflug, G., Rojas, R., and Ward, P. J.: Increasing stress on disaster-risk finance due to large floods, Nat. Clim. Change, 4, 264–268, https://doi.org/10.1038/nclimate2124, 2014.
Jonkman, S. N.: Advanced flood risk analysis required, Nat. Clim. Change, 3, 1004, https://doi.org/10.1038/nclimate2031, 2013.
Kates, R. W., Colten, C. E., Laska, S., and Leatherman, S. P.: Reconstruction of New Orleans after Hurricane Katrina: A research perspective, P. Natl. Acad. Sci. USA, 103, 14653–14660, https://doi.org/10.1073/pnas.0605726103, 2006.
Koks, E. E., Jongman, B., Husby, T. G., and Botzen, W. J. W.: Combining hazard, exposure and social vulnerability to provide lessons for flood risk management, Environ. Sci. Policy, 47, 42–52, https://doi.org/10.1016/j.envsci.2014.10.013, 2015.
Kwadijk, J. C. J., Haasnoot, M., Mulder, J. P. M., Hoogvliet, M. M. C., Jeuken, A. B. M., van der Krogt, R. A. A., van Oostrom, N. G. C., Schelfhout, H. A., van Velzen, E. H., van Waveren, H., and de Wit, M. J. M.: Using adaptation tipping points to prepare for climate change and sea level rise: a case study in the Netherlands, Wires Clim. Change, 1, 729–740, https://doi.org/10.1002/wcc.64, 2010.
Li, Y. H., Jia, L. R., Wu, W. H., Yan, J. Y., and Liu, Y. S.: Urbanization for rural sustainability – Rethinking China's urbanization strategy, J. Clean Prod., 178, 580–586, https://doi.org/10.1016/j.jclepro.2017.12.273, 2018.
Liang, P., Xu, W., Ma, Y., Zhao, X., and Qin, L.: Increase of Elderly Population in the Rainstorm Hazard Areas of China, Int. J. Env. Res. Pub. He., 14, 963, https://doi.org/10.3390/ijerph14090963, 2017.
Liu, S.: Calculation of Flood Control Capacity in Quzhou under Current Conditions, Journal of Zhejiang University of Water Resources and Electric Power, 29, 27–31, available at: https://kns.cnki.net/kcms/detail/detail.aspx?FileName=ZJSL201705007&DbName=CJFQ2017 (last access: 22 February 2021), 2017.
Liu, Y. S. and Li, Y. H.: Revitalize the world's countryside, Nature, 548, 275–277, https://doi.org/10.1038/548275a, 2017.
Meng, X.: People Flocking to China's Cities, Science, 343, 138–139, https://doi.org/10.1126/science.1244814, 2014.
Mokrech, M., Kebede, A. S., Nicholls, R. J., Wimmer, F., and Feyen, L.: An integrated approach for assessing flood impacts due to future climate and socio-economic conditions and the scope of adaptation in Europe, Climatic Change, 128, 245–260, https://doi.org/10.1007/s10584-014-1298-6, 2015.
National Bureau of Statistics of China: China Statistical Yearbook 2014, Beiijing, China, 2015.
Qiang, Y.: Disparities of population exposed to flood hazards in the United States, J. Environ. Manage., 232, 295–304, https://doi.org/10.1016/j.jenvman.2018.11.039, 2019.
Rudari, R., Silvestro, F., Campo, L., Rebora, N., Boni, G., and Herold, C.: Improvement of the global food model for the GAR 2015, United Nations Office for Disaster Risk Reduction (UNISDR), Centro Internazionale in Monitoraggio Ambientale (CIMA), UNEP GRID, Arendal, 69, 2015.
Salvati, P., Petrucci, O., Rossi, M., Bianchi, C., Pasqua, A. A., and Guzzetti, F.: Gender, age and circumstances analysis of flood and landslide fatalities in Italy, Sci. Total Environ., 610, 867–879, https://doi.org/10.1016/j.scitotenv.2017.08.064, 2018.
Scussolini, P., Aerts, J. C. J. H., Jongman, B., Bouwer, L. M., Winsemius, H. C., de Moel, H., and Ward, P. J.: FLOPROS: an evolving global database of flood protection standards, Nat. Hazards Earth Syst. Sci., 16, 1049–1061, https://doi.org/10.5194/nhess-16-1049-2016, 2016.
Shen, J., Du, S. Q., Huang, Q. X., Yin, J., Zhang, M., Wen, J. H., and Gao, J.: Mapping the city-scale supply and demand of ecosystem flood regulation services-A case study in Shanghai, Ecol. Indic., 106, 8, https://doi.org/10.1016/j.ecolind.2019.105544, 2019.
Smith, A., Bates, P. D., Wing, O., Sampson, C., Quinn, N., and Neal, J.: New estimates of flood exposure in developing countries using high-resolution population data, Nat. Commun., 10, 7, https://doi.org/10.1038/s41467-019-09282-y, 2019.
The World Bank: Health Nutrition and Population Statistics: Population estimates and projections, the World Bank, the United States, 2019.
Tian, Q. and Lemos, M. C.: Household Livelihood Differentiation and Vulnerability to Climate Hazards in Rural China, World Dev., 108, 321–331, https://doi.org/10.1016/j.worlddev.2017.10.019, 2018.
United Nations: World Urbanization Prospects: The 2018 Revision, United Nations, the United States, 2018.
Wang, Z., Yu, Y., Ji, Y., and He, X.: Discussion on the mechanism of temporary settlement and supply guarantee for residents in the detention basin, Journal of Economics of Water Resources, 34, 75–78+82, available at: https://kns.cnki.net/kcms/detail/detail.aspx?FileName=SLJJ201605018&DbName=CJFQ2016 (last access: 22 February 2021), 2016.
Ward, P. J., Jongman, B., Weiland, F. S., Bouwman, A., van Beek, R., Bierkens, M. F. P., Ligtvoet, W., and Winsemius, H. C.: Assessing flood risk at the global scale: model setup, results, and sensitivity, Environ. Res. Lett., 8, 044019, https://doi.org/10.1088/1748-9326/8/4/044019, 2013.
Ward, P. J., Jongman, B., Aerts, J., Bates, P. D., Botzen, W. J. W., Loaiza, A. D., Hallegatte, S., Kind, J. M., Kwadijk, J., Scussolini, P., and Winsemius, H. C.: A global framework for future costs and benefits of river-flood protection in urban areas, Nature Clim. Change, 7, 642–646, https://doi.org/10.1038/nclimate3350, 2017.
Willner, S. N., Levermann, A., Zhao, F., and Frieler, K.: Adaptation required to preserve future high-end river flood risk at present levels, Sci. Adv., 4, 1914, https://doi.org/10.1126/sciadv.aao1914, 2018.
Winsemius, H. C., Jongman, B., Veldkamp, T. I. E., Hallegatte, S., Bangalore, M., and Ward, P. J.: Disaster risk, climate change, and poverty: assessing the global exposure of poor people to floods and droughts, Environ. Dev. Econ., 23, 328–348, https://doi.org/10.1017/s1355770x17000444, 2018.
Wu, J., Huang, L., Liu, Y., Peng, J., Li, W., Gao, S., and Kang, C.: Traffic Flow Simulation Based on Call Detail Records, Acta Geogr. Sin., 67, 1657–1665, https://doi.org/10.11821/xb201212007, 2012.
Zhou, Z.: Research on Influence of Land Subsidence on Flood Control Capacity in the Downstream Area of Daqing River, Yellow River 40, 43–48, https://doi.org/10.3969/j.issn.1000-1379.2018.06.010, 2018.
Zhu, B., Fu, Y., Liu, J. L., He, R. X., Zhang, N., and Mao, Y.: Detecting the priority areas for health workforce allocation with LISA functions: an empirical analysis for China, BMC Health Serv. Res., 18, 14, https://doi.org/10.1186/s12913-018-3737-y, 2018.