the Creative Commons Attribution 4.0 License.
the Creative Commons Attribution 4.0 License.
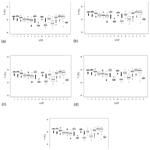
Assessing heat exposure to extreme temperatures in urban areas using the Local Climate Zone classification
Anna Deluca
Dirk Lauwaet
Joan Ballester
Jordi Corbera
Maria Carmen Llasat
Trends of extreme-temperature episodes in cities are increasing (in frequency, magnitude and duration) due to regional climate change in interaction with urban effects. Urban morphologies and thermal properties of the materials used to build them are factors that influence spatial and temporal climate variability and are one of the main reasons for the climatic singularity of cities. This paper presents a methodology to evaluate the urban and peri-urban effect on extreme-temperature exposure in Barcelona (Spain), using the Local Climate Zone (LCZ) classification as a basis, which allows a comparison with other cities of the world characterised using this criterion. LCZs were introduced as input of the high-resolution UrbClim model (100 m spatial resolution) to create daily temperature (median and maximum) series for summer (JJA) during the period 1987 to 2016, pixel by pixel, in order to create a cartography of extremes. Using the relationship between mortality due to high temperatures and temperature distribution, the heat exposure of each LCZ was obtained. Methodological results of the paper show the improvement obtained when LCZs were mapped through a combination of two techniques (land cover–land use maps and the World Urban Database and Access Portal Tools – WUDAPT – method), and the paper proposes a methodology to obtain the exposure to high temperatures of different LCZs in urban and peri-urban areas. In the case of Barcelona, the distribution of temperatures for the 90th percentile (about 3–4 ∘C above the average conditions) leads to an increase in the relative risk of mortality of 80 %.
- Article
(5808 KB) - Full-text XML
- BibTeX
- EndNote
Alterations to the natural environment associated with urban activity mean that climate variability in urban landscapes is more complex than in peri-urban and rural areas. Urban landscapes are home to more than half the world's population, and projections show that two-thirds of the world's population will live in cities by 2050 (UN, 2015). Urban areas are certainly more exposed and vulnerable to the negative effects of climate change due to their non-sustainable relationship with surrounding areas and environments. The Urban Climate Change Research Network's Second Assessment Report on Climate Change in Cities (ARC3.2) (Rosenzweig et al., 2018) places the average annual temperature increase ratio per decade between 0.1 and 0.5 ∘C in the period from 1961 to 2010 in the cities it analysed. And it is estimated that the temperature will rise between 1.3 and 3 ∘C in the middle of the 21st century (2040–2070) and between 1.7 and 4.9 ∘C at the end (2070–2100).
Urban landscapes are particularly sensitive to rising temperatures at all timescales (Pachauri et al., 2014). Heat waves (HWs) are one of the deadliest weather events, and their frequency, intensity and duration are expected to increase in the future due to climate change (Li and Bou-Zeid, 2013; De Jarnett and Pittman, 2017; Sheridan and Dixon, 2016) and the urban-heat-island (UHI) effect. Consequently, the related health impacts are of emerging environmental-health concern (Wolf and McGregor, 2013). In Europe, the growing urbanisation along with the impacts of the increase in extreme temperature causes increased heat-related mortality (Smid et al., 2019; Ingole et al., 2020).
There are many factors that influence the spatial and temporal climate variability in urban areas, such as different urban morphologies and the thermal properties of the materials used to build such areas (Geletič et al., 2016; Li et al., 2016). One of the main topics usually studied to characterise the urban climate is the extreme temperatures in cities due to the UHI effect, which was first discussed back in the 1940s (Balchin and Pye, 1947). Historically, a considerable body of research has been published on the phenomenon (i.e. Oke, 1982; Lo et al., 1997; Arnfield, 2003; Voogt and Oke, 2003; Chen et al., 2006; Mirzaei and Haghighat, 2010; Giannaros et al., 2014; Lehoczky et al., 2017; Sobrino and Irakulis, 2020). However, certain methodological inconsistencies have been revealed when comparing different urban climate studies. One of the main reasons is the lack of standardisation to compare the properties that affect specific urban thermal behaviour (Stewart, 2011). Moving forward from this premise, a new methodology based on the urban climate zones defined by Oke (2004) and called the Local Climate Zone (LCZ) classification has emerged (Stewart and Oke, 2012). LCZ classification establishes a system of standardisation for urban and rural areas and their thermal responses. It proposes a classification with a total of 17 measurable categories based on a combination of geometric, thermal, radiative and metabolic parameters that characterise urban and peri-urban areas. By using this classification, it is possible to study the effects of urban climate in more spatial and temporal detail (Bechtel et al., 2015). Combinations of built environment (Benzie et al., 2011; Inostroza et al., 2016) are well encompassed by the LCZ approach, and, along with socio-demographic factors (Nayak et al., 2018), this allows us to develop a geospatial distribution of heat exposure (Dickson et al., 2012; Drobinski et al., 2014). Along the same line of research, the international project called World Urban Database and Access Portal Tools (WUDAPT) has created a portal with guidelines based on Earth observation data, with the aim of building a worldwide database of cities, using the LCZ classification. This standardisation will allow comparisons between cities while providing better data for meteorological and climate models (Brousse et al., 2016; Ching et al., 2018). Currently, the available, validated layer for Barcelona on the WUDAPT portal is the one made in our studio to fill in the Metropolitan Area of Barcelona (AMB), as explained in more detail in Sect. 3.1.
Due to LCZ classification being originally designed to mainly describe the thermal characteristics of the different land covers and land uses, it is useful to apply it to estimate the level of heat exposure (Vicedo-Cabrera et al., 2014; Lowe et al., 2015; Achebak et al., 2019) to adverse climate conditions that is one of the main goals of this paper. There are a wide range of definitions for the term “vulnerability” (UNISDR, 2009; Cutter, 1996; Llasat et al., 2009), which depend on different physical and social factors (Cutter et al., 2000; Tromeur et al., 2012; Nakamura and Llasat, 2017). In this framework heat vulnerability is understood as a combination of heat exposure (based on high temperatures) and sensitivity (Wolf and McGregor, 2013; Bao et al., 2015; Inostroza et al., 2016), where the last is related to population characteristics and coping capacities. Although there are some publications that study risk on an urban scale for extreme heat events (Xu et al., 2012; Weber et al., 2015; Krstic et al., 2017; Eum et al., 2018), few have been studied from an LCZ perspective. This paper therefore aims to assess heat exposure using the LCZ classification in a coastal Mediterranean metropolitan region. Barcelona constitutes a good example of a Mediterranean coastal megacity (port cities with a population greater than 1 million in 2005) (Hanson et al., 2011) that can be severely affected by climate change impacts. In effect, the annual mean temperature increase in the Mediterranean Basin is higher than the world average (1.5 ∘C above 1880–1899 levels in 2018) and could be above 2.2 ∘C in 2040 without additional mitigation (Lionello et al., 2014; Cramer et al., 2018; MedECC, 2019). Direct impacts on health produced by the frequency and intensity increase in heat waves and tropical nights will be amplified by the urban-heat-island effect, which is particularly important in Barcelona (Baccini et al., 2011; Martin-Vide and Moreno, 2020). Associated with this temperature increase, by 2050, for the lower-sea-level-rise scenarios and current adaptation measures, cities in the Mediterranean will account for half of the 20 global cities with the highest increase in average annual damage (Hallegate et al., 2013).
This study is a starting point for new research lines with three objectives in mind: (a) making changes to urban land cover and observing the changes in heat exposure to high temperatures without having to resort to climate modelling, (b) downscaling the temperature outputs of urban models to resolutions of under 100 m using the LCZ maps, and (c) applying this methodology to climate change scenarios.
2.1 Study area
The Metropolitan Area of Barcelona (AMB) and its surroundings have been selected for the application of the LCZ classification. AMB involves the city of Barcelona and 35 adjoining municipal areas (Fig. 1). The AMB is situated in the northwest of the Mediterranean Basin and covers an area of 636 km2 with a population of around 3.2 million. The city of Barcelona (∼1.6 million) is in its centre, between the rivers Llobregat (south) and Besòs (north), the Catalan Coastal Range (west), and the Mediterranean Sea (east) (Fig. 1b).
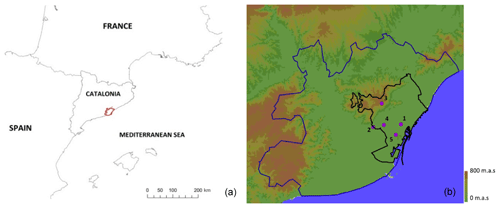
Figure 1(a) Location of the Metropolitan Area of Barcelona (AMB), (b) Domain used to run the UrbClim model. The blue line marks the border of the AMB, while the black line shows the municipality of Barcelona. The numbers indicate the weather stations used to assess the LCZ–T relationship.
The Barcelona municipality has been selected to analyse the effect of high temperatures and apply the proposed methodology approach on a neighbourhood scale. Barcelona is divided into 10 districts, which are subdivided into 73 neighbourhoods. It covers an area of 101 km2 and has a population density of over 15 000 inh./km2, which is higher than New York City, Tokyo or New Delhi. In terms of climate, Barcelona and its surroundings are characterised by hot summers (25–27 ∘C average temperature), and the thermal stress of high temperatures is accentuated by the proximity of the sea, which results in a humid atmosphere. Total precipitation in Barcelona is around 600 mm per year. Autumn is the wettest season and has a highly irregular distribution of precipitation, in many cases causing episodes of urban flooding (Gilabert and Llasat, 2017; Cortès et al., 2018).
2.2 Methodology design
In order to carry out this study, we followed the workflow shown below:
-
LCZ mapping. A geographic information system methodology based on land cover and land use (LCLU) maps has been applied to the entire AMB to improve the precision of the international WUDAPT method. The WUDAPT method has also been applied to all the area shown in Fig. 1b, both inside and outside AMB, that will be used as input of the climate model.
-
Climate characterisation. The climate of the median- and extreme-temperature distribution in Barcelona was characterised from the outputs of the UrbClim model.
-
Heat exposure thresholds. Heat exposure thresholds were defined based on the epidemiological temperature–mortality model proposed by Achebak et al. (2018).
-
Thermal characterisation. A methodology was developed for the thermal characterisation of the LCZs and its assessment.
Each one of these steps will be explained in detail in the following sections in order to simplify the understanding of this methodology in which each part is based on the results of the previous one. The overall methodology followed constitutes a result of this work.
2.3 LCZ mapping
2.3.1 Data from official thematic cartography, satellite images and weather stations
In order to create the LCZ cartography, data shown in Table 1 have been used. The LCZs were represented following two methods, as explained in Sect. 3. The land cover–land use method was based on using all the layers presented in Table 1, except for the Landsat 8 image, which was only used with the WUDAPT methodology and the orthophoto to make the training areas.
2.3.2 Land cover and land use method and WUDAPT method
There are several proposals for mapping LCZs, whether with a bottom-up or top-down approach (Brousse et al., 2016; Lelovics et al., 2014; Wang et al., 2017; Mitraka et al., 2015). Each LCZ is defined by 10 variables (geometric, radiative and metabolic), which were tested and standardised by Stewart and Oke (2012) and are applied in this study.
Our study features an LCZ map that combines two different mapping techniques (Fig. 2). For the administrative region of the AMB (with a more extensive and detailed source of data), a methodology based on land cover and land use (LCLU) data was used that departs from the reclassification of the land use key for the existing high-resolution maps. The LCLU data were combined with lidar data, which allowed us to define the height of the buildings. There are other techniques that use similar methodologies to show LCZs, like those by Geletič and Lehnert (2016) or Skarbit et al. (2017). For the area outside the AMB, the international WUDAPT methodology was used, based on satellite Earth observation data (Bechtel et al., 2015). This study improved accuracy through a population map and high-resolution orthophotos provided by the Cartographic and Geological Institute of Catalonia (ICGC). Both methodologies are summarised below.
The LCLU method is based on different land cover and land use maps (see Table 1), such as the Land Cover Map of Catalonia (LCLU-Cat), which uses both an extensive classification of up to 241 categories made by the Centre for Research on Ecology and Forestry Applications and the Urban Atlas (UA). The first thematic map was used to define the land cover types and density of vegetation. The UA distinguishes 20 categories of urban areas and discerns between urban fabric type and density, which is why it is very useful for the first 10 categories of LCZ classification. Each LCLU category corresponds to one of the descriptions of the different morphological parameters that define the LCZs. Building heights is another layer of the map and was made with a lidar sensor, which was also used to discern between the different building types of each LCZ.
Figure 3 shows the difference between the total coverage of each LCZ when obtained from the LCLU and from WUDAPT maps in AMB (Fig. 2). In the WUDAPT approach, 52.8 % of the surface area of the AMB consists of urban areas (LCZs 1–10 and E), while in the high-resolution map (LCLU approach), the same type of coverage occupies just 37.3 %. It is a consequence of the difference in the LCZ characterisation processes that both methods follow. Although 17 LCZs are distinguished in the two methods, WUDAPT uses the spectral radiance provided by satellite images and applies a supervised classification based on a random-forest generalisation method based on training zones (Bechtel et al., 2015). On the contrary, the method of LCLU proposed here analyses the intrinsic variables that characterises each category of LCZ classification, and consequently it has major integrity and quality. That is to say, it has a better resolution. In both methods, we can see that the natural-forest category (LCZ A) is the most common, accounting for 24.1 % and 18.4 % of the land respectively. This is due to the fact that the Metropolitan Area of Barcelona includes Serra de Collserola Natural Park in the coastal mountain range. The next most common class is LCZ C, which corresponds to scrubland and bush. Dealing with land classified as urban, the most common types include industrial estates (LCZ 8); areas with dense buildings less than 25 m tall (LCZ 2); and category LCZ 6, which consists of open arrangements of mid-rise buildings. The WUDAPT map suffers from a lack of characterisation of urban areas, which is not the case for the LCLU map.
The resulting LCZ map is a high-resolution thematic vector base map (Fig. 2b), in which each polygon that makes up the urban fabric is attributed to an LCZ category (Gilabert et al., 2016). Finally, it was rasterised at a resolution of 100 m, applying an all shape filter, so that it could be used as an input for the UrbClim model. The method we followed is shown in the workflow diagram (Fig. 4). There are similar examples in the literature, such as the LCZ map for Île-de-France (http://www.institutparisregion.fr, last access: 23 July 2020) or the LCZ LCLU map of Vienna (Hammerberg et al., 2018).
The WUDAPT method (Bechetel et al., 2015) allows us to create a 100 m × 100 m raster map based on Earth observation data from remote sensing. The representative regions of interest are chosen for proposed LCZ categories from Earth observation satellite data, with the use of very high resolution aerial orthophotos as the ground truth. The LCZ map, made by the first author of this paper using the WUDAPT proposal, is officially presented on the project portal and is available for download (http://www.wudapt.org, last access: 23 July 2020). This method has been applied to an extended area as is shown in Fig. 5.
A multi-resolution grid shape file (62.5, 125 and 250 m) containing information on the population as registered in 2016 made by the Statistical Institute of Catalonia (Generalitat de Catalunya and Institut d'Estadística de Catalunya, 2015) was used to correct the peri-urban areas of AMB where rural activities cannot be well identified. The orthophoto was used to check and correct any categories and the limits between them.
Figure 5 shows the resulting map combining the LCLU method (in raster format) for the administrative region of the AMB and the WUDAPT method for the rest of the study area with a final resolution of 100 m.
2.4 Weather stations
Table 2 shows the weather stations within the municipality of Barcelona that have been used to evaluate and compare the characterisation of the LCZ with the daily average temperature outputs of the UrbClim model. LCZ and height information are also attached.
2.5 UrbClim model simulation
UrbClim is an urban boundary layer climate model specifically designed to simulate temperature at a very high spatial resolution (here at 100 m; De Ridder et al., 2015). The model consists of a land surface scheme with simplified urban physics coupled to a 3D atmospheric boundary layer. UrbClim is faster than high-resolution mesoscale climate models by at least 2 orders of magnitude (García-Díez et al., 2016), making the very long runs that are necessary for climate-change-related studies possible. UrbClim has been recently validated in several European cities, including Barcelona (García-Díez et al., 2016). Currently, within the framework of the Pan-European Urban Climate Service (PUCS) project (H2020, 2017–2019), the urban climate of Barcelona has been modelled until 2100, keeping in mind different representative concentration pathways (RCPs) to observe the consequences of climate change on an urban scale. Barcelona was chosen, among other European cities, and VITO and ISGlobal were the organisations responsible for modelling this city.
UrbClim model uses a land surface and a soil–vegetation–atmosphere transfer scheme that is designed to deal with urban surfaces. Each surface grid cell in the model is made up of portions of vegetation, bare soil and urban surface cover, which are all represented using LCZ mapping. A set of transfer equations, together with appropriate parameter values for albedo, emissivity, and aerodynamic and thermal roughness length, is used to simulate the heat transfer in each surface grid cell. The large-scale atmospheric conditions are used as lateral and upper boundary conditions. The 3D boundary layer model represents a simplified atmosphere by using the continuity equations for horizontal momentum, potential temperature, specific humidity and mass.
The simulations for the 1987–2016 period were used for this period. The UrbClim simulations cover a large domain containing 401 × 401 horizontal grid points at a 100 m resolution (40×40 km approximately) and 19 vertical levels within the lower 3 km of the troposphere. It covers the entire geographical area of the Metropolitan Area of Barcelona, including the neighbouring highly populated cities. The driving model data are updated every 3 h using ERA-Interim reanalysis (Dee et al., 2011), which runs at a spatial resolution of T255 (approximately 70–80 km). The UrbClim model directly downscales the ERA-Interim reanalysis data to a 100 m resolution. The climate distributions of the daily mean temperature (Tmean), maximum temperature and dew point temperatures were calculated for all the summer months (JJA). The maximum temperature provides an estimate of the worst conditions that can be expected. This is important for risk management and avoiding heatstroke, which usually occurs during the hours of the day when the temperature reaches its highest value. The dew point temperature (Tdew) was used as a starting point to calculate the humidex (humidity index; Eq. 1), which describes the perceived thermal feeling of a person, by combining the effect of heat and humidity (Masterton and Richardson, 1979). Barcelona has quite high relative humidity during the summer months, which means that the humidex increases considerably.
2.6 Quantifying heat exposure by temperature
The next step consists of reclassifying the maps of the proposed distributions for the daily mean temperature, keeping in mind the impact that they can have on health. This was carried out using the results provided in the study by Achebak et al. (2018), in which a distributed-lag nonlinear model was used to model the short-term delayed relation between daily summer temperature and mortality data from cardio-respiratory diseases in Barcelona (and 46 other cities), over a similar period of time modelled (Fig. 6). This makes it possible to objectively establish the thresholds for health relative risks (RRs), based on temperature. For instance, an RR value of 1.20 means that the relative risk of mortality is 20 % higher at a given level of temperature exposure compared to a baseline optimum temperature (e.g. temperature of minimum mortality, when RR = 1). Relative risks are statistically significant when the lower bound of the confidence interval is greater than 1.
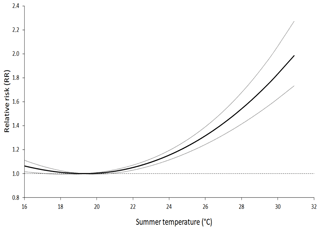
Figure 6Relative risk (RR) curve based on mortality due to summer daily temperature (JJA) in Barcelona for the 1980–2015 period (Achebak et al., 2018).
We are assuming that the curve is applicable to all districts of the city (Achebak et al., 2018). Table 3 has been built for RR intervals of 0.2 (20 %) following Fig. 6. Each RR interval has been associated with a heat exposure index (HEI) that includes temperature intervals based on the curve of Achebak et al. (2018). Barcelona deals with an HEI value of 1 for temperatures between 18 and 20 ∘C up to an HEI value of 7 for temperatures above 31.1 ∘C which would mean a very high relative risk of mortality associated with high temperatures. The use of seven HEI categories has the advantage that it can be applied to any city by adjusting them to the temperature values of that city and to the RR curve considered.
3.1 UrbClim temperature outputs and HEI maps
In order to analyse the impact of the different LCZs in the distribution of high temperatures in summer, the maps of maximum and daily mean temperature corresponding to percentiles P50, P75, P90, P95 and P99 have been built (Fig. 7). Barcelona has a high relative humidity due to proximity to the sea that increases warm perception, and, for this reason, the cartography of the average daily humidex value has also been represented.
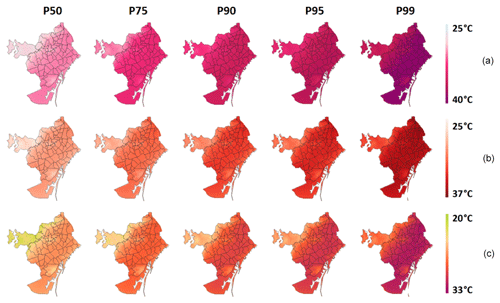
Figure 7Climatological conditions in summer modelled by UrbClim (1987–2016): (a) humidex, (b) daily maximum temperature and (c) daily mean temperature, for the different distributions (P50, P75, P90, P95 and P99).
As we can see in Fig. 7, there is a very similar spatial distribution pattern. The lowest temperatures are in the most remote area of the coast, and they are mainly associated with categories LCZ A and LCZ 9 (mainly covering areas of woodland or very low density buildings). A cooling effect can also be noted in the most important parks in the city, as well as on the seafront, because of the sea breeze (the UrbClim model underestimates the sea breeze effect in Barcelona; García-Diez et al., 2016). The highest temperatures can be found in the centre of the city, with a tendency to increase in a north-easterly direction.
We saw that P99 of the humidex reached 39 ∘C. In Barcelona, without taking humidity into account, the average temperature in the city can reach above 30 ∘C. Even so, normal temperatures during the summer are around 27 ∘C. In Mediterranean cities, relative humidity is important since it is usually high, a fact that affects temperature (Diffenbaugh et al., 2007). In this sense, we observe that the humidex can register temperatures on the order of 5 ∘C higher than the sensible temperature. This study has focused on sensible temperature because the curve that defines the heat exposure index has been made for sensible temperature. In any case, we must bear in mind that the temperature or heat stress may be higher due to the greater humidex.
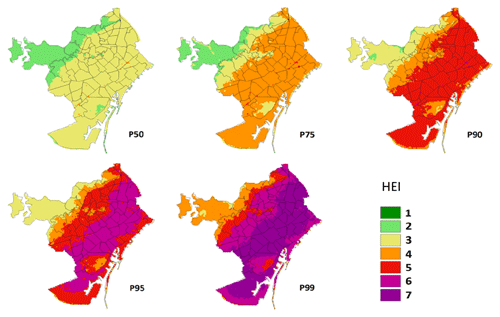
Figure 8Maps of HEI for the different probability distributions proposed (P50, P75, P90, P95 and P99).
Figure 8 shows maps of HEI distribution reclassified with the UrbClim output of daily mean temperature according to the proposed thresholds shown in Sect. 2.6. This reclassification turns the extreme-temperature maps or hazard maps into heat exposure maps. It can be seen that the HEI is lower in areas with higher altitude and in inter-urban parks (such as the Montjuïc park located in the SE of the map); although when P90 is surpassed, the HEI value goes over level 5 for most of the urban fabric. Note that P50 shows an increase in the relative risk of mortality of 40 %.
3.2 Thermal characterisation of the LCZs
In this section we aim to match up each LCZ with a determined thermal behaviour to create a methodology that will allow us to estimate the heat exposure to high temperatures from these data.
First, for each climatic percentile (P50, P75, P90, P95 and P99) of daily mean temperature (although it could also be performed for the maximum temperature and humidex) we analysed the thermal response of the LCZ (LCZ–T) (Fig. 7). To do so we compared, pixel by pixel, the temperature maps with the LCZ maps, and we built a box plot for each LCZ (Fig. 9).
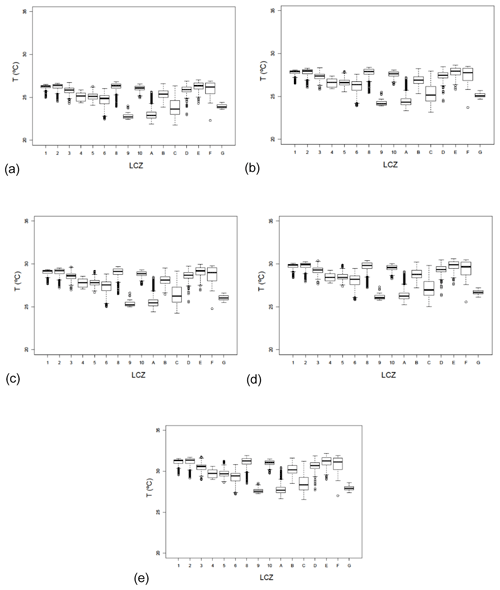
Figure 9Box plots for the thermal characterisation of the LCZ for different distributions: (a) P50, (b) P75, (c) P90, (d) P95 and (e) P99.
In order to characterise each LCZ, we tested its normality and the differentiated behaviour of each probability density curve adjusted to each LCZ. The results of the normality tests (based on the central limit theorem) and comparable variations on the relation between LCZ–T indicated that ANOVA may be used for testing whether the differences in LCZ mean temperatures outlined above are significant or not (Geletic et al., 2016). LCZs C, F and 6 do not follow a normal distribution (at 95 %) although they tend towards it. This is due to the high thermal variability in these categories. There were statistically significant differences in mean land surface temperatures between most LCZs, but LCZs 4 and 5 were recognised as zones that were less distinguishable from other LCZs. Once we had the temperature distribution it was possible to map the HEI.
Transposing the model onto LCZ maps allowed us to map heat exposure distributions for Barcelona. This methodology has the advantage that it can be transferred to other cities because it relates each LCZ with an HEI value. It is only necessary to have the LCZ map and know some temperature values in the city to calibrate the model. In the case that there is not an RR–T curve available, the same HEI as in this paper could be applied.
Figure 9 shows that LCZs 8 (large low-rise buildings), 1 (compact high-rise), E (asphalt) and 2 (compact mid-rise) (from highest to lowest) usually have the highest temperatures. These LCZs in general terms correspond to the categories with high admittance and high permeability (Stewart and Oke, 2012). In contrast, the lowest temperatures correspond to LCZs 9 (sparsely built), A (dense trees), C (bushes) and G (water), which are wooded areas and parks on the outskirts of the city. On the other hand, crops and bare land (LCZs C and F) show very variable behaviour, as during the day they tend to be surfaces that store and retain heat, while during the night their behaviour registers temperatures below the average of the sample. These surfaces are characterised by a large temperature range given the marked contrast between day and night.
Table 4 shows that the more extreme the percentile, the larger the standard deviation, as expected. Besides this, the more marked deviations correspond to LCZs C and F, which correspond to wooded or bare areas and which show less thermal inertia. On the other hand, category C is very highly influenced (in the case of Barcelona) by orientation, as there are zones located in shaded parts of valleys while other zones are in the sunny ones, which has a direct impact on the deviation. In the case of category C, we observed that it corresponds to land use that is not very representative in spatial terms.
3.3 Mapping the heat exposure with LCZs
Figure 10 shows the average behaviour of the LCZs for different temperature percentiles (P50, P75, P90, P95, P99). The values corresponding to the range between the 25th and 75th percentiles of each LCZ for each probability scenario have been adjusted to a logarithmic curve that can be very useful for building heat exposure maps for high temperatures based on the thermal properties of the LCZ. Knowing the temperature distribution for each category and scenario allows for performing a simulation of the impact on temperature distribution of potential modifications to the urban morphology.
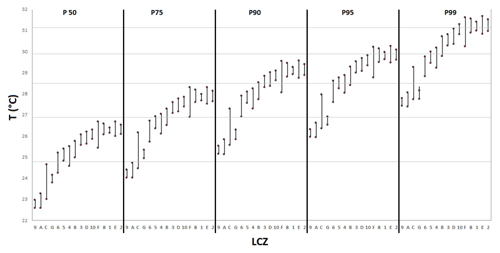
Figure 10Characterisation of every LCZ with the daily mean temperature (1987–2016) for each probability scenario. Each bar shows P25 and P75, around the median for each LCZ (ordered from lowest to highest temperature). The horizontal grey lines are the different HEI scenarios (2 to 7, low to high).
As explained in the methodology, seven ranges of temperature have been defined according to different relative risk thresholds (Table 3) established by the curve proposed in the study by Achebak et al. (2018) (Fig. 6). By characterising the LCZs from the model represented in Fig. 11, the maps of the heat exposure index associated with high temperatures for different probabilistic scenarios have been built. The scenario corresponding to P75 of the temperature would imply a ratio of relative risk of mortality increase of 60 %, and this would be 80 % in a scenario according to P90.
3.4 Assessment and comparison of the LCZ–T relationship
The results of the LCZ–T relationship as well as the results of the urban climate model (UC) have been compared with the distribution of temperature obtained from series of over 10 years for five weather stations (Table 2) located in different LCZs in the municipality of Barcelona. Root mean square errors (RMSEs), the differences between the output of both sets of results (UrbClim model and LCZ–T relationship) and observations have been obtained in order to compare the results (Tables 5 and 6). We want to highlight that UrbClim has already been validated in Barcelona by García-Díez et al. (2016) as outlined in Sect. 2.6. Table 5 shows that differences in absolute value are lower than 1.2 ∘C. In all the cases they are equal to or below 0.5 ∘C for the percentile of 50, and this is also the case for the percentile of 75 with the exception of the Raval station, which is placed in the oldest part of the city. It should also be kept in mind that a stand-alone observation is not the same as an aerial 100×100 m observation, and this fact is particularly important when the weather station is surrounded by buildings.
Table 5Temperature for each distribution scenario (DIST) and weather station observed (OB), modelled by UrbClim (UC) and estimated from the distribution of temperature (the mean value is taken) for each LCZ (LCZ–T). The difference (Δ) between them is also shown. All the values are expressed in degrees Celsius. ZU denotes Zona Universitària.
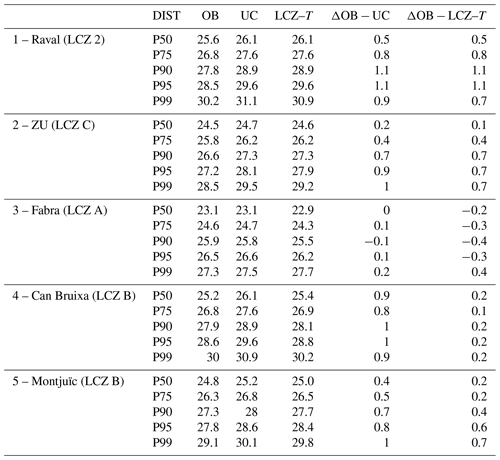
Table 6Number of pixels where the HEI obtained through the LCZ–T model (Fig. 11) underestimates, overestimates or coincides with the HEI provided by the urban climate model (Fig. 6) for the different scenarios. Percentage of coincidences and RMSE are also shown.
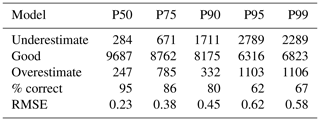
The HEI maps drawn up using the LCZs were compared with the map based on temperature distribution created by UrbClim (Table 6). Coincidences between pixels for both models are above 80 % for percentiles P50, P75 and P90, and they are more than 60 % in all cases.
This paper presents a methodology to characterise the distribution of daily mean temperature in reference to the Local Climate Zone (LCZ) mapping in different temperature scenarios in summer (June–July–August). The climate percentiles have been obtained for the period 1987–2016 and applied at a 100 m resolution to the city of Barcelona. Although other authors have already worked with the relationship between thermal behaviour and LCZ category (Stewart et al., 2014; Skarbit et al., 2015; Geletič et al., 2016; Verdonck et al., 2018), they have usually applied land surface temperature satellite images for the summer months and short time periods. Other characterisations of LCZ classification using weather stations can also be found in Alexander and Mills (2014) and Kotharjar and Bagade (2018). In these cases, these authors have worked with climate series from observational data. The advantage of the methodology proposed here, in which the LCZ distribution has been compared with the outputs of a high-resolution climate model (UrbClim), is that the relationship has been established from long-term climate series and for the entire selected region. Currently, there are multiple studies characterising LCZs using urban model outputs (Aminipouri et al., 2019; Beck et al., 2018; Geletič et al., 2018; Kwok et al., 2019; Unger et al., 2018), but there are not many studies with climatic ouputs that span as many years.
The results of this methodology applied to the Metropolitan Area of Barcelona have shown a major difference between the thermal response in summer for the different LCZs than that obtained from some satellite images. In terms of land use, LCZs A and C, which belong to the most prevalent categories, show the lowest temperatures, consistent with the majority of studies carried out (e.g. Geletič et al., 2016). In our case, category C shows a wider interquartile range than the other types. This is because this category is found in different altitudes along the Catalan Coastal Range and in areas with different orientations. Regarding category B, attributed to the majority of inter-urban parks, it shows temperatures below those of the most typical urban zones.
The highest daily mean summer temperatures in Barcelona are concentrated in LCZs 2, E, 1, 8, F and 10, with LCZs 2, 1 and E being the most representative of urban planning in the city centre. With regard to LCZs 8 and 10, these are zones that tend to record high temperatures due to the nature of the activities and materials on the land cover (in most cases, metal structures). The urban LCZ with the lowest temperatures is 9, which is almost non-existent in Barcelona and is located mainly in zones in the Catalan Coastal Range with a significant altitudinal slope. Another urban LCZ with low relative temperatures commonly found in the city is 6, which is mainly located in the neighbourhoods furthest away from the coast and closer to the mountain. These neighbourhoods have a higher percentage of urban green cover, less dense buildings and some of the highest gross domestic products per capita in the city.
The paper has also introduced the heat exposure index (HEI), which evaluates the increase in the risk of mortality ratio as a consequence of heat exposure in reference to the model proposed by Achebak et al. (2018) which connects relative risk of mortality caused by cardio-respiratory failure with the effects of high temperatures. This index, associated with each LCZ once the temperature has been associated with it, allows for the mapping of the HEI. The comparison between the heat exposure index maps elaborated directly from the temperature outputs produced by the UrbClim model and those produced from LCZ cartography is well suited to simulating heat exposure index maps for scenarios corresponding to percentiles of temperature between 50 % and 90 %, and, in the case in which there is no coincidence between the HEI value in the pixel, it is more usual to have underestimation than overestimation. In the case of Barcelona, the distribution of temperatures for P90 (about 3–4 ∘C compared to average conditions) leads to an increase in the relative risk of mortality of 80 %, and the increase is 40 % in the case of P50.
This paper also provides comparison of two methodologies to map LCZs: WUDAPT and the land cover–land use (LCLU) method based on land use maps. The international standard method WUDAPT is exclusively based on satellite Earth observation data (Ching et al., 2018). LCLU is based on land use maps, the Urban Atlas, lidar measurements and orthophotos. LCLU has been applied to the Metropolitan Area of Barcelona, and WUDAPT has been applied to the entire region (inside and outside) the AMB. The WUDAPT map suffers from a lack of characterisation of different types of urban areas, which is not the case for the land cover–land use method. Therefore, when the required data are available, it is better to apply the LCLU methodology than the WUDAPT one. In this study, the curve of Achebak et al. (2018) was taken into account as representative of the whole of Barcelona city. In the future, it would be good to have a similar curve for different districts of the city. In addition to this, future work could include mapping sensitivity, taking into account coping capacities based on gross domestic product (GDP), or the social structure of the neighbourhood. This would include vulnerability.
In conclusion, the LCZ–T relation based on the characterisation of the average temperature for each LCZ corresponding to different percentile distributions allows us to consider adaptive methods, proposing changes to more sustainable urban planning, for example the use of green or white cover. The advantage of the proposed methodology is that it allows us to obtain a heat exposure distribution for summer temperatures without having to resort to climate models, by applying the model of temperature distribution associated with each LCZ. It can also be useful to perform different experiments modifying land use and land coverage applied to the cartography and, consequently, the LCZ distribution and their associated heat exposure index. Another possibility is being able to separate the heat exposure levels on an LCZ map with higher spatial resolutions from those used in weather models and climate models.
The satellite data to apply the WUDAPT methodology were obtained from the official USGS EarthExplorer website: https://earthexplorer.usgs.gov/ (Geological Survey (U.S.) and EROS Data Center, 1980). The Google Earth Pro and SAGA open-source software were used to reproduce the WUDAPT workflow proposal. The data to map the LCZs at a vector resolution are public data – the Urban Atlas and the LCLU-Cat obtained from the following URLs: https://www.creaf.uab.es/mcsc/ (CREAF, 2009) and https://land.copernicus.eu/local/urban-atlas/urban-atlas-2012 (EEA, 2012) . Lidar data belong to the ICGC and must be requested. The data were obtained in the framework of the Industrial Doctorate programme (ref. 2015-DI-038). The orthophoto was obtained using the WMS link, https://www.icgc.cat/es/Administracion-y-empresa/Servicios/Servicios-en-linea-Geoservicios/WMS-y-teselas-Cartografia-de-referencia/WMS-Mapas-y-ortofotos-vigentes (Institut Cartogràfic i Geològic de Catalunya, 2015), and the population data were obtained from the public URL, https://biblio.idescat.cat/publicacions/Record/21104 (Generalitat de Catalunya and Institut d'Estadística de Catalunya, 2014). The meteorological data to validate the model were provided by the two official and public services in Catalonia: AEMET and SMC. The UrbClim model used public ERA-Interim reanalysis data from https://apps.ecmwf.int/datasets/data/interim-full-daily/levtype=sfc/ (Berrisford et al., 2009). The model was run at the VITO centre under the PUCS project (no. 730004)). The RR model was based on the model presented in the article of Achebak et al. (2018).
JG conceived the study, designed and carried out the data analysis, and wrote the paper. MCL, JC and JB participated in defining the analysis and methodology and contributed to interpreting the results and to writing the paper. DL and AdL ran the UrbClim model and prepared the output data.
The authors declare that they have no conflict of interest.
Our thanks go to M-CostAdapt (TM2017-83655-C2-2-R) research projects (MINECO/AEI/FEDER, UE), an ERC Consolidator Grant awarded to Gara Villalba (818002-URBAG), and the Water Research Institute (IdRA) at the University of Barclona. The authors would like to thank the European Environment Agency (EEA), Centre for Ecological Research and Forestry Applications (CREAF) and Metropolitan Area of Barcelona for making the land use maps available. We would also like to thank the State Meteorological Agency (AEMET) and the Meteorological Service of Catalonia (SMC) for the weather station data. Finally, we want to thank Hicham Achebak for giving us the RR model.
Joan Ballester gratefully acknowledges funding from the European Union's Horizon 2020 research and innovation programme under grant agreement nos. 865564 (European Research Council Consolidator Grant EARLY-ADAPT), 727852 (project Blue-Action) and 730004 (project PUCS) and from the Ministry of Science and Innovation (MCIU) under grant agreement nos. RYC2018-025446-I (programme Ramón y Cajal) and EUR2019-103822 (project EURO-ADAPT).
This research has been supported by the Industrial Doctorate programme (ref. 2015-DI-038) between the University of Barcelona and the Cartographic and Geological Institute of Catalonia.
This paper was edited by Ricardo Trigo and reviewed by two anonymous referees.
Achebak, H., Devolder, D. and Ballester, J.: Heat-related mortality trends under recent climate warming in Spain: A 36-year observational study, PLoS Med., 15, e1002617, https://doi.org/10.1371/journal.pmed.1002617, 2018.
Achebak, H., Devolder, D., and Ballester, J.: Trends in temperature-related age-specific and sex-specific mortality from cardiovascular diseases in Spain: a national time-series analysis, Lancet Planet. Health, 3, e297–e306, 2019.
Alexander, P. and Mills, G.: Local climate classification and Dublin's urban heat island, Atmosphere-Basel, 5, 755–774, 2014.
Aminipouri, M., Knudby, A. J., Krayenhoff, E. S., Zickfeld, K., and Middel, A.: Modelling the impact of increased street tree cover on mean radiant temperature across Vancouver's local climate zones, Urban For. Urban Gree., 39, 9–17, 2019.
Arnfield, A. J.: Two decades of urban climate research: a review of turbulence, exchanges of energy and water, and the urban heat island, Int. J. Climatol., 23, 1–26, 2003.
Baccini, M., Kosatsky, T., Analitis, A., Anderson, H. R., D'Ovidio, M., Menne, B., Michelozzi, P., and Biggeri, A.: Impact of heat on mortality in 15 European cities: attributable deaths under different weather scenarios, J. Epidemiol. Commun. H., 65, 64–70, 2011.
Balchin, W. G. V. and Pye, N.: A micro-climatological investigation of bath and the surrounding district, Q. J. Roy. Meteor. Soc., 73, 297–323, 1947.
Bao, J., Li, X., and Yu, C.: The construction and validation of the heat vulnerability index, a review, Int. J. Env. Res. Pub. He., 12, 7220–7234, 2015.
Bechtel, B., Alexander, P. J., Böhner, J., Ching, J., Conrad, O., Feddema, J., Mills, G., See, L., and Stewart, I.: Mapping local climate zones for a worldwide database of the form and function of cities, ISPRS Int. Geo.-Inf., 4, 199–219, 2015.
Beck, C., Straub, A., Breitner, S., Cyrys, J., Philipp, A., Rathmann, J., Schneider, A., Wolf, K., and Jacobeit, J.: Air temperature characteristics of local climate zones in the Augsburg urban area (Bavaria, southern Germany) under varying synoptic conditions, Urban Clim., 25, 152–166, 2018.
Benzie, M., Burningham, K., and Hodgson, N.: Vulnerability to Heat Waves and Drought: Case Studies of Adaptation to Climate Change in South-West England, York, UK, The Joseph Rowntree Foundation, 2011.
Berrisford, P., Dee, D. P. K. F., Fielding, K., Fuentes, M., Kallberg, P., Kobayashi, S., and Uppala, S.: The ERA-interim archive. ERA report series, 1–16, available at: https://apps.ecmwf.int/datasets/data/interim-full-daily/levtype=sfc/ (last access: 23 July 2020), 2009.
Brousse, O., Martilli, A., Foley, M., Mills, G., and Bechtel, B.: WUDAPT, an efficient land use producing data tool for mesoscale models? Integration of urban LCZ in WRF over Madrid, Urban Clim., 17, 116–134, 2016.
Chen, X. L., Zhao, H. M., Li, P. X., and Yin, Z. Y.: Remote sensing image-based analysis of the relationship between urban heat island and land use/cover changes, Remote Sens. Environ, 104, 133–146, 2006.
Ching, J., Mills, G., Bechtel, B., See, L., Feddema, J., Wang, X., Ren, C., Brousse, O., Martilli, A., Neophytou, M., Mouzoudires, P., Stewart, I., Hanna, A., Ng, E., Foley, M., Alexander, P., Aliaga, D., Niyogi, D., Shreevastava, A., Bhalachandran, S., Masson, V., Hidalgo, J., Fung, J., de Fatima Andrad, M., Baklanov, A., Wei Dai, D., Milcinski, G., Demuzere, M., Brunsell, N., Pesaresi, M., Miao, S., Mu, Q., Chen, F., and Theeuwes, N.: World urban data base and access portal tools (WUDAPT), an urban weather, climate and environmental modelling infrastructure for the Anthropocene, B. Am. Meteorol. Soc., 99, 1907–1924, 2018.
Cortès, M., Llasat, M. C., Gilabert, J., Llasat-Botija, M., Turco, M., Marcos, R., Martin Vide, J. P., and Falcón L.: Towards a better understanding of the evolution of the flood risk in Mediterranean urban areas: the case of Barcelona, Nat. Hazards, 93, 39–60, 2018.
CREAF: Generalitat de Catalunya, Mapa de cobertes del sòl de Catalunya, available at: https://www.creaf.uab.es/mcsc/ (last access: 23 July 2020), 2009.
Cutter, S. L.: Vulnerability to environmental hazards, Prog. Hum. Geogr., 20, 529–539, 1996.
Cutter, S. L., Mitchell, J. T., and Scott, M. S.: Revealing the vulnerability of people and places: a case study of Georgetown County, South Carolina, Ann. Am. Assoc. Geogr., 90, 713–737, 2000.
Cramer, W., Guiot, J., Fader, M., Garrabou, J., Gattuso, J. P., Iglesias, A., Lange, M. A., Lionello, P., Llasat, M. C., Paz, S., Peñuelas, J., Snoussi, M., Toreti, A., Tsimplis, M. N., and Xoplaki, E.: Climate change and interconnected risks to sustainable development in the Mediterranean, Nat. Clim. Change, 8, 972–980, https://doi.org/10.1038/s41558-018-0299-2, 2018.
Dee, D. P., Uppala, S. M., Simmons, A. J., Berrisford, P., Poli, P., Kobayashi, S., Andrae, U., Balmaseda, M. A., Balsamo, G., Bauer, P., Bechtold, P., Beljaars, A. C. M., van de Berg, L., Bidlot, J., Bormann, N., Delsol, C., Dragani, R., Fuentes, M., Geer, A. J., Healy S. B., Hersbach, H., Hólm, E. V., Isaksen, L., Kallberg, P., Kölher, M., Matricardi, M., McNally, A. P., Morcrette, J. J., Park, B. K., Peubey, C., de Rosnay, P., Tavolato, C., Thépaut, J. N., and Vitart, F.: The ERA-Interim reanalysis: Configuration and performance of the data assimilation system, Q. J. Roy. Meteor. Soc., 137, 553–597, 2011.
DeJarnett, N. and Pittman, M.: Protecting the Health and Wellbeing of Communities in a Changing Climate, Proceedings of a Workshop – in Brief, The National Academies Press, Washington, DC, https://doi.org/10.17226/24797, 8, 2017.
De Ridder, K., Lauwaet, D., and Maiheu, B.: UrbClim–A fast urban boundary layer climate model, Urban Clim., 12, 21–48, 2015.
Dickson, E., Baker, J. L., and Hoornweg, D.: Urban risk assessments: understanding disaster and climate risk in cities, The World Bank Publications, Washington DC, 2012.
Diffenbaugh, N. S., Pal, J. S., Giorgi, F., and Gao, X.: Heat stress intensification in the Mediterranean climate change hotspot, Geophys. Res. Lett., 34, L11706, https://doi.org/10.1029/2007GL030000, 2007.
Drobinski, P., Ducrocq, V., Alpert, P., Anagnostou, E., Béranger, K., Borga, M., Braud, I., Chanzy, A., Davolio, S., Delrieu, G., Estournel, C., Filali Boubrahmi, N., Font, J., Grubisic, V., Gualdi, S., Homar, V., Ivancan-Picek, B., Kottmeier, C., Kotroni, V., Lagouvardos, K., Lionello, P., Llasat, M. C., Ludwig, W., Lutoff, C., Mariotti, A., Richard, E., Romero, R., Rotunno, R., Roussot, O., Ruin, I., Somot, S., Taupier-Letage, I., Tintore, J., Uijlenhoet, R., and Wernli, H.: HyMeX, a 10-year multidisciplinary program on the Mediterranean water cycle, B. Am. Meteor. Soc., 95, 1063–1082, 2014.
Eum, J. H., Kim, K., Jung, E. H., and Rho, P.: Evaluation and Utilization of Thermal Environment Associated with Policy: A Case Study of Daegu Metropolitan City in South Korea, Sustainability, 10, 1179, https://doi.org/10.3390/su10041179, 2018.
European Environment Agency (EEA): Copernicus Programme, Urban Atlas LCLU, EU, available at; https://land.copernicus.eu/local/urban-atlas/urban-atlas-2012 (last access: 23 July 2020), 2012.
García-Díez, M., Lauwaet, D., Hooyberghs, H., Ballester, J., De Ridder, K., and Rodó, X.: Advantages of using a fast urban boundary layer model as compared to a full mesoscale model to simulate the urban heat island of Barcelona, Geosci. Model Dev., 9, 4439–4450, https://doi.org/10.5194/gmd-9-4439-2016, 2016.
Geletič, J. and Lehnert, M.: GIS-based delineation of local climate zones: The case of medium-sized Central European cities, Morav. Geogr. Rep., 24, 2–12, 2016.
Geletič, J., Lehnert, M., and Dobrovolný, P.: Land Surface Temperature Differences within Local Climate Zones, Based on Two Central European Cities, Remote Sens., 8, 788, https://doi.org/10.3390/rs8100788, 2016.
Geletič, J., Lehnert, M., Savic, S., and Miloševic, D.: Modelled spatiotemporal variability of outdoor thermal comfort in local climate zones of the city of Brno, Czech Republic, Sci. Total Environ., 624, 385–395, 2018.
Generalitat de Catalunya and Institut d'Estadística de Catalunya: Població De Catalunya Georeferenciada a 1 De Gener De 2014 Barcelona, available at: https://biblio.idescat.cat/publicacions/Record/21104 (last access: 23 July 2020), 2014.
Geological Survey (U.S.) and EROS Data Center: EarthExplorer, Reston, Va., U.S. Dept. of the Interior, U.S. Geological Survey, available at: https://earthexplorer.usgs.gov/ (last access: 23 July 2020), 1980.
Giannaros, T. M., Melas, D., Daglis, I. A., and Keramitsoglou, I.: Development of an operational modeling system for urban heat islands: an application to Athens, Greece, Nat. Hazards Earth Syst. Sci., 14, 347–358, https://doi.org/10.5194/nhess-14-347-2014, 2014.
Gilabert, J. and Llasat, M. C.: Circulation Weather Types associated with extreme flood events in Northwestern Mediterranean, Int. J. Climatol. 38, 1864–1876, 2017.
Gilabert, J., Tardà, A., Llasat, M. C., and Corbera, J.: Assessment of Local Climate Zones over Metropolitan Area of Barcelona and added value of Urban Atlas, Corine Land Cover and Copernicus Layers under INSPIRE Specifications, INSPIRE Conference, 26 September 2016, Barcelona, 2016.
Hallegatte, S., Green, C., Nicholls, R. J., and Corfee-Morlot, J.: Future flood losses in major coastal cities, Nat. Clim. Change, 3, 802–806, 2013.
Hammerberg, K., Brousse, O., Martilli, A., and Mahdavi, A.: Implications of employing detailed urban canopy parameters for mesoscale climate modelling: a comparison between WUDAPT and GIS databases over Vienna, Austria, Int. J. Climatol., 38, 1241–1257, 2018.
Hanson, S., Nicholls, R., Ranger, N., Hallegatte, S., Cofree-Morlot, J., Herweijer, C., and Chateau, J.: A global ranking of port cities with high exposure to climate extremes, Climatic Change, 104, 89–111, 2011.
Ingole, V., Marí-Dell'Olmo, M., Deluca, A., Quijal, M., Borrell, C., Rodríguez-Sanz, M., Achebak, H., Lauwet, D., Gilabert, J., Murage, P., Hajat, S., Basagaña, X., and Ballester, J.: Spatial Variability of Heat-Related Mortality in Barcelona from 1992–2015: A Case Crossover Study Design, Int. J. Env. Res. Pub. He., 17, 2553, https://doi.org/10.3390/ijerph17072553, 2020.
Inostroza, L., Palme, M., and de la Barrera, F.: A heat vulnerability index: spatial patterns of exposure, sensitivity and adaptive capacity for Santiago de Chile, Plos One, 11, e0162464, https://doi.org/10.1371/journal.pone.0162464, 2016.
Kotharkar, R. and Bagade, A.: Local Climate Zone classification for Indian cities: A case study of Nagpur, Urban Clim., 24, 369–392, 2018.
Krstic, N., Yuchi, W., Ho, H. C., Walker, B. B., Knudby, A. J., and Henderson, S. B.: The Heat Exposure Integrated Deprivation Index (HEIDI): A data-driven approach to quantifying neighborhood risk during extreme hot weather, Environ. Int., 109, 42–52, 2017.
Kwok, Y. T., Schoetter, R., Lau, K. K. L., Hidalgo, J., Ren, C., Pigeon, G., and Masson, V.: How well does the local climate zone scheme discern the thermal environment of Toulouse (France)? An analysis using numerical simulation data, Int. J. Climatol., 39, 5292–5315, 2019.
Lehoczky, A., Sobrino, J. A., Skoković, D., and Aguilar, E.: The Urban Heat Island Effect in the City of Valencia: A Case Study for Hot Summer Days, Urban Science, 1, 9, https://doi.org/10.3390/urbansci1010009, 2017.
Lelovics, E., Unger, J., and Gál, T.: Design of an urban monitoring network based on Local Climate Zone mapping and temperature pattern modelling, Clim. Res., 60, 51–62, 2014.
Li, D. and Bou-Zeid, E.: Synergistic interactions between urban heat islands and heat waves: The impact in cities is larger than the sum of its parts, J. Appl. Meteorol. Clim., 52, 2051–2064, https://doi.org/10.1175/JAMC-D-13-02.1, 2013.
Li, X., Li, W., Middel, A., Harlan, S. L., Brazel, A. J., and Turner, B. L.: Remote sensing of the surface urban heat island and land architecture in Phoenix, Arizona: Combined effects of land composition and configuration and cadastral- demographic- economic factors, Remote Sens. Environ., 174, 233–243, 2016.
Lionello, P., Abrantes, F., Gacic, M., Planton, S., Trigo, R., and Ulbrich, U.: The climate of the Mediterranean region: research progress and climate change impacts, Reg. Environ. Change, 14, 1679–1684., 2014.
Llasat, M. C., Llasat-Botija, M., and López, L.: A press database on natural risks and its application in the study of floods in Northeastern Spain, Nat. Hazards Earth Syst. Sci., 9, 2049–2061, https://doi.org/10.5194/nhess-9-2049-2009, 2009.
Lo, C. P., Quattrochi, D. A., and Luvall, J. C.: Application of high-resolution thermal infrared remote sensing and GIS to assess the urban heat island effect, Int. J. Remote Sens., 18, 287–304, 1997.
Lowe, R., Ballester, J., Creswick, J., Robine, J. M., Herrmann, F. R., and Rodó, X.: Evaluating the performance of a climate-driven mortality model during heat waves and cold spells in Europe, Int. J. Env. Res. Pub. He., 12, 1279–1294, 2015.
Martin-Vide, J. and Moreno-Garcia, M. C.: Probability values for the intensity of Barcelona's urban heat island (Spain), Atmos. Res., 240, 104877, https://doi.org/10.1016/j.atmosres.2020.104877, 2020.
Masterton, J. M. and Richardson, F. A.: Humidex: a method of quantifying human discomfort due to excessive heat and humidity, Environment Canada, Atmospheric Environment, National government publication, 1979.
MedECC: Risks associated to climate and environmental changes in the Mediterranean region. A preliminary assessment by the MedECC Network, Science-policy interface – 2019, 36 pp., 2019.
Mirzaei, P. A. and Haghighat, F.: Approaches to study urban heat island–abilities and limitations, Build. Environ., 45, 2192–2201, 2010.
Mitraka, Z., del Frate, F., Chrysoulakis, N., and Gastellu-Etchegorry, J. P.: Exploiting earth observation data products for mapping local climate zones, 2015 Joint Urban Remote Sensing Event (JURSE), Lausanne, 30 March–1 April, 15201755, https://doi.org/10.1109/JURSE.2015.7120456, 2015.
Nakamura, I. and Llasat, M. C.: Policy and systems of flood risk management: a comparative study between Japan and Spain, Nat. Hazards, 87, 919–943, 2017.
Nayak, S. G., Shrestha, S., Kinney, P. L., Ross, Z., Sheridan, S. C., Pantea, C. I., Hsu, W. H., Muscatiello, N., and Hwang, S. A.: Development of a heat vulnerability index for New York State, Public Health, 161, 127–137, 2018.
Oke, T. R.: The energetic basis of the urban heat island, Q. J. Roy. Meteor. Soc., 108, 1–24, 1982.
Oke, T. R.: Urban observations, World Meteorological Organization, IOM Report No. 81, WMO/TD no. 1250, WMO, Geneva, 2004.
Institut Cartogràfic i Geològic de Catalunya: Ortofoto de Catalunya, available at: https://www.icgc.cat/es/Administracion-y-empresa/Servicios/Servicios-en-linea-Geoservicios/WMS-y-teselas-Cartografia-de-referencia/WMS-Mapas-y-ortofotos-vigentes (last access: 23 July 2020), 2015.
Pachauri, R. K., Allen, M. R., Barros, V. R., Broome, J., Cramer, W., Christ, R., Church J. A., Clarke, L., Dahe, Q., Dasgupta, P., Dubash, N. K., Edenhofer, O., Elgizouli, I., Field, C. B., Forster, P., Friedlingstein, P., Fuglestvedt, J., Gomez-Echeverri, L., Hallegatte, S., Hegerl, G., Howden, M., Jiang, K., Jimenez Cisneroz, B., Kattsov, K., Lee, H., Mach, K. J., Marotzke, J., Mastrandrea, M. D., Meyer, L., Minx, J., Mulugetta, Y., O'Brien, Oppenheimer, M., Pereira, J. J., Pichs-Madruga, R., Plattner, G. K., Pörtner, H. O., Power, S. B., Preston, B., Ravindranath, N. H., Reisinger, A., Riahi, K., Rusticucci, M., Scholes, R., Seyboth, K., Sokona, Y., Stavins, R., Stocker, T. F., Tschakert, P., van Vuuren, D., and van Ypserle, J. P.: Climate change 2014: synthesis report. Contribution of working groups I, II and III to the fifth assessment report of the intergovernmental panel on climate change, edited by: Pachauri, R. and Meyer, L., IPCC, Geneva, Switzerland, ISBN: 978-9-2916-9143-2, 2014.
Rosenzweig, C., Solecki, W. D., Romero-Lankao, P., Mehrotra, S., Dhakal, S., and Ali Ibrahim, S.: Climate change and cities. Second assessment report of the urban climate change research network, Cambridge University Press, New York, 2018.
Sheridan, S. C. and Dixon, P. G.: Spatiotemporal trends in human vulnerability and adaptation to heat across the United States, Anthropocene, 20, 61–73, 2016.
Skarbit, N., Gal, T., and Unger, J.: Airborne surface temperature differences of the different Local Climate Zones in the urban area of a medium sized city, Joint Urban Remote Sensing Event (JURSE), Lausanne, 30 March–1 April, 15215553, https://doi.org/10.1109/JURSE.2015.7120497, 2015.
Skarbit, N., Stewart, I. D., Unger, J., and Gál, T.: Employing an urban meteorological network to monitor air temperature conditions in the `local climate zones' of Szeged, Hungary, Int. J. Climatol., 37, 582–596, 2017.
Smid, M., Russo, S., Costa, A. C., Granell, C., and Pebesma, E.: Ranking European capitals by exposure to heat waves and cold waves, Urban Climate, 27, 388–402, 2019.
Sobrino, J. A. and Irakulis, I.: A Methodology for Comparing the Surface Urban Heat Island in Selected Urban Agglomerations Around the World from Sentinel-3 SLSTR Data, Remote Sens., 12, 2052, https://doi.org/10.3390/RS12122052, 2020.
Stewart, I. D.: A systematic review and scientific critique of methodology in modern urban heat island literature, Int. J. Climatol., 31, 200–217, 2011.
Stewart, I. D. and Oke, T. R.: Local climate zones for urban temperature studies, B. Am. Meteorol. Soc., 93, 1879–1900, 2012.
Stewart, I. D., Oke, T. R., and Krayenhoff, E. S.: Evaluation of the “local climate zone” scheme using temperature observations and model simulations, Int. J. Climatol., 34, 1062–1080, 2014.
Tromeur, E., Ménard, R., Bailly, J.-B., and Soulié, C.: Urban vulnerability and resilience within the context of climate change, Nat. Hazards Earth Syst. Sci., 12, 1811–1821, https://doi.org/10.5194/nhess-12-1811-2012, 2012.
UN: World population prospects: The 2015 revision, United Nations Econ. Soc. Aff., 33, 1–66, 2015.
Unger, J., Skarbit, N., and Gál, T.: Evaluation of outdoor human thermal sensation of local climate zones based on long-term database, Int. J. Biometeorol., 62, 183–193, 2018.
UNISDR, UNOFDRR: Terminology on disaster risk reduction, Geneva, Switzerland, 30 pp., 2009.
Verdonck, M. L., Demuzere, M., Hooyberghs, H., Beck, C., Cyrys, J., Schneider, A., Dewulf, R., and Van Coillie, F.: The potential of local climate zones maps as a heat stress assessment tool, supported by simulated air temperature data, Landscape Urban Plan., 178, 183–197, 2018.
Vicedo-Cabrera, A. M., Iñíguez, C., Barona, C., and Ballester, F.: Exposure to elevated temperatures and risk of preterm birth in Valencia, Spain, Environ. Res., 134, 210–217, 2014.
Voogt, J. A. and Oke, T. R.: Thermal remote sensing of urban climates, Remote Sens. Environ, 86, 370–384, 2003.
Wang, R., Ren, C., Xu, Y., Lau, K. K. L., and Shi, Y.: Mapping the local climate zones of urban areas by GIS-based and WUDAPT methods: A case study of Hong Kong, Urban Clim., 24, 567–576, 2017.
Weber, S., Sadoff, N., Zell, E., and de Sherbinin, A.: Policy-relevant indicators for mapping the vulnerability of urban populations to extreme heat events: A case study of Philadelphia, Appl. Geogr., 63, 231–243, 2015.
Wolf, T. and McGregor, G.: The development of a heat wave vulnerability index for London, United Kingdom, Weather and Climate Extremes, 1, 59–68, 2013
Xu, Z., Sheffield, P. E., Hu, W., Su, H., Yu, W., Qi, X., and Tong, S.: Climate change and children's health- A call for research on what works to protect children, Int. J. Env. Res. Pub. He., 9, 3298–3316, 2012.