the Creative Commons Attribution 4.0 License.
the Creative Commons Attribution 4.0 License.
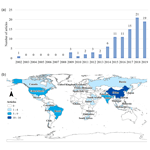
Review article: A systematic review and future prospects of flood vulnerability indices
Luana Lavagnoli Moreira
Mariana Madruga de Brito
Masato Kobiyama
Despite the increasing body of research on flood vulnerability, a review of the methods used in the construction of vulnerability indices is still missing. Here, we address this gap by providing a state-of-art account on flood vulnerability indices, highlighting worldwide trends and future research directions. A total of 95 peer-reviewed articles published between 2002–2019 were systematically analyzed. An exponential rise in research effort is demonstrated, with 80 % of the articles being published since 2015. The majority of these studies (62.1 %) focused on the neighborhood followed by the city scale (14.7 %). Min–max normalization (30.5 %), equal weighting (24.2 %), and linear aggregation (80.0 %) were the most common methods. With regard to the indicators used, a focus was given to socioeconomic aspects (e.g., population density, illiteracy rate, and gender), whilst components associated with the citizen's coping and adaptive capacity were slightly covered. Gaps in current research include a lack of sensitivity and uncertainty analyses (present in only 9.5 % and 3.2 % of papers, respectively), inadequate or inexistent validation of the results (present in 13.7 % of the studies), lack of transparency regarding the rationale for weighting and indicator selection, and use of static approaches, disregarding temporal dynamics. We discuss the challenges associated with these findings for the assessment of flood vulnerability and provide a research agenda for attending to these gaps. Overall, we argue that future research should be more theoretically grounded while, at the same time, considering validation and the dynamic aspects of vulnerability.
- Article
(1061 KB) -
Supplement
(220 KB) - BibTeX
- EndNote
- Included in Encyclopedia of Geosciences
Floods affect billions of people worldwide (Zarekarizi et al., 2020). Indeed, according to the Emergency Events Database (CRED, 2019), around 50 000 people died and approximately 10 % of the world population was affected by floods between 2009 and 2019. Due to population growth and climate change, more frequent and widespread floods are anticipated (Hirsch and Archfield, 2015; Leung et al., 2019). Therefore, flood risk management is required for mitigating potential damages.
Nowadays there is a consensus that risk (i.e., the potential for adverse impacts) is not driven solely by natural hazards (e.g., floods, droughts) but depends on the interactions between hazards, exposure, and vulnerability (IPCC, 2012, 2014). In this regard, vulnerability plays an important role in flood risk assessment. It encompasses multiple social, economic, physical, cultural, environmental, and institutional characteristics which influence the susceptibility of the exposed elements to the impact of hazards (Birkmann et al., 2013; UNDRR, 2017). Due to its importance, the need to understand and assess flood vulnerability has been highlighted by international initiatives such as the Sendai Framework for Disaster Risk Reduction 2015–2030 (UNISDR, 2015).
In response to this, numerous studies have been undertaken to better understand flood vulnerability. Nevertheless, both the terminology and methodology used in these assessments are still a subject of discussion (Aroca-Jiménez et al., 2020; Kelman, 2018). In fact, some consider vulnerability as being a function of exposure and susceptibility (Balica et al., 2009; IPCC, 2001; Turner et al., 2003; UNDP, 2014), while others separate these concepts (Dilley et al., 2005; Fedeski and Gwilliam, 2007) as it is possible to be exposed to a hazard and not be vulnerable. For instance, a person may live in an area prone to natural hazards but have sufficient alternatives to modify the structure of their house to prevent potential losses (Cardona et al., 2012).
A wide range of approaches have been proposed for assessing flood vulnerability. The most commonly used methods are stage damage functions (Papathoma-Köhle et al., 2012, 2017; Tarbotton et al., 2015), damage matrices (Bründl et al., 2009; Papathoma-Köhle et al., 2017), and vulnerability indices (Birkmann, 2006; de Brito et al., 2017; Kappes et al., 2012; Moreira et al., 2021). The first two methods assess only the physical vulnerability, neglecting the social vulnerability of their inhabitants (Koks et al., 2015). However, the capacity of households to cope, adapt, and respond to hazards is equally important for assessing the potential impacts of floods (de Brito et al., 2018). Therefore, given the importance of holistic studies on vulnerability to ensure a better representation of reality, the use of vulnerability indices is recommended (Balica et al., 2013; Birkmann et al., 2013; Fuchs et al., 2011; Nasiri et al., 2016). Indices serve as a summary of complex and multidimensional issues to assist decision-makers, to facilitate the interpretation of a phenomenon, and to increase public interest through a summary of the results. Flood vulnerability indices are, therefore, a tool for measuring the vulnerability degree throughout the aggregation of several indicators or variables. Despite their advantages, indices can present misleading messages if they are poorly constructed or misinterpreted. Hence, a clear understanding of the normalization, weighting, and aggregation methods used to build an index is required (Moreira et al., 2021).
Over the past few years, several review articles about flood vulnerability have been published. For instance, Rufat et al. (2015) reviewed 67 articles to identify the leading drivers of social vulnerability to floods. Rehman et al. (2019) and Fatemi et al. (2017) reviewed different methodologies used for assessing flood vulnerability. Jurgilevich et al. (2017) systematically reviewed 42 climate risk and vulnerability assessments. More recently, Diaz-Sarachaga and Jato-Espino (2020) evaluated 72 articles related to the appraisal of vulnerability in different types of hazards in urban areas. Some studies also analyzed different methods and indexed construction designs to understand which decisions have the greatest influence on the vulnerability outcomes. For instance, Nasiri et al. (2016) compared damage curves, computer modeling, and indicators to evaluate flood vulnerability. Similarly, Schmidtlein et al. (2008) and Tate (2012, 2013) examined the sensitivity of the results to changes in the construction of the vulnerability index.
Notwithstanding these advances, to the best of our knowledge, no study has conducted a systematic review of flood vulnerability indices with a focus on the different stages involved in the construction of flood vulnerability indices. The investigation of the methods used for normalizing, weighting, aggregation, and validation and the implications for each choice for vulnerability assessment have received little attention so far. In addition, even though there have been recent advancements in the field (e.g., Cutter and Derakhshan, 2020), the temporal dynamics of flood vulnerability have not been tackled by the existing reviews. This is particularly important given that certain adaptation policies and strategies may reduce short-term risk probability but increase long-term vulnerability and exposure (Cardona et al., 2012). Therefore, a better understanding of the methods used in each step of the index construction, the vulnerability temporal dynamics (e.g., pre- and post-flood), and the uncertainty involved is needed for advancing research on flood vulnerability assessments.
Considering the aforementioned gaps, and given the proliferation of methods for building vulnerability indices, it is pertinent to review the development of this field. Hence, here, we carried out a systematic literature review of indices used to assess flood vulnerability. The focus is given to urban and riverine floods. The following questions guided the analysis: (1) which spatial scale was considered? (2) Which indicators were most commonly used to measure flood vulnerability? (3) How were the temporal dynamics of vulnerability addressed (e.g., pre- or post-flood event)? (4) Which methods were most commonly applied in each stage of the index building process (i.e., normalization, weighting, or aggregation)? (5) To which extent did these studies conduct validation and apply uncertainty and sensitivity analysis? In addition to highlighting existing challenges, we also point out directions for further research.
In general, indicators consist of various pieces of data capable of synthesizing the characteristics of a system. When these indicators are aggregated, they are called index or composite indicators (Saisana and Tarantola, 2002). Overall, the construction of an index comprehends seven steps (Fig. 1). First, the phenomenon to be measured is defined, so that the index results can provide a clear understanding of this phenomenon (Nardo et al., 2008). Then, the indicators used to measure the phenomenon are selected. This should be done carefully as the results reflect the quality of the selected indicators.
Table 1Characteristics of the main normalization methods used for building indices.
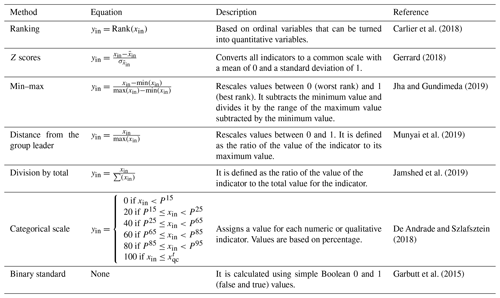
Note: y is the transformed variable of x for indicator i for unit n. Pi is the ith percentile of the distribution of the indicator xin, and p is an arbitrary threshold around the mean.
In the third step, the relationships between the selected indicators are identified. Indicators with similar characteristics can be grouped, aiming to reduce the number of variables. To this end, statistical analysis (e.g., principal component analysis – PCA) or expert knowledge can be used to decide whether the indicators are sufficient or appropriate for describing the phenomenon (Nardo et al., 2008). After selecting the indicators, they need to be normalized to a common scale before being aggregated into an index as they usually have different units of measurement (see Table 1 for the main normalization methods). By doing so, problems with outliers can also be reduced (Jacobs et al., 2004).
The fifth step comprises the weighting and aggregation of the indicators. Weights can be assigned to indicators to demonstrate their importance in relation to the studied phenomenon (see Table 2 for the main weighting methods). Given that it may be difficult to find an acceptable weighting scheme, equal weights are often used, which implies that all criteria are worth the same (de Brito et al., 2018). Alternatively, an equal weighting scheme could be the result of a lack of knowledge about the indicators' importance. After the indicators are weighted, they are aggregated. The most common aggregation methods are linear and geometric. The linear method consists of the weighted and normalized sum of indicators, whereas the geometric aggregation represents the output of the indicators for which the exponent is their assigned weight (Nardo et al., 2008).
The sixth step consists of sensitivity and uncertainty analyses (see Table 3 for the main uncertainty and sensitivity methods). The first evaluates the contribution of the uncertainty source of each indicator to the variance of the results, while the latter focuses on how the uncertainty of each indicator propagates through the index structure and affects the outputs (Saisana et al., 2005; Saisana and Tarantola, 2002).
Table 3Characteristics of the main methods for uncertainty and sensitivity analysis used for building indices.
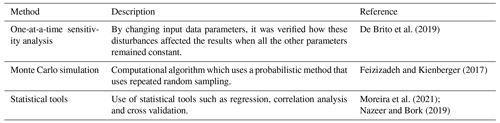
The final step comprises the validation of the index results. This is crucial for verifying if they are consistent with the real system and have a satisfactory precision range. Validation can be achieved by using independent secondary data that refer to observable outcomes. Since vulnerability is not a directly observable phenomenon, its validation requires the use of proxies such as mortality and built environment damage (Schneiderbauer and Ehrlich, 2006), post-event surveys (Fekete, 2009), number of disasters (Debortoli et al., 2017), and emergency service requests (Kontokosta and Malik, 2018).
A bibliographic search was performed by focusing on studies that constructed flood vulnerability indexes. The Web of Science (WoS) database was used to identify peer-reviewed articles published since 1945, using the following Boolean keywords: (“flood” OR “flooding”) AND (“index” OR “composite indicator”) AND (“vulnerability” NOT “coast*”). Only the abstract, title, and keywords were searched. This narrowed the search space substantially.
These queries elicited over 348 articles published between January 2002 and December 2019. At first, the title, abstract, and keywords were screened manually to exclude articles that were not useful for the purpose of the present study. After this preselection, the full text of 84 selected papers was revised in detail. An additional 11 key articles were included. They were not found in our original search even though they built vulnerability indices. This occurred because the keywords “index” or “composite indicator” were not mentioned in the article's abstract, title, or keywords. Hence, this limitation should be acknowledged, as relevant articles may have been disregarded.
Following their selection, the articles were classified according to (1) publication year, (2) study area country, (3) spatial scale (e.g., neighborhood, household, or city), (4) region classification (e.g., urban, rural,1 or both), (5) number of indicators, (6) whether or not there was a reduction in the indicators (e.g., PCA and expert knowledge), (7) temporal dynamics (pre- or post-flood), (8) normalization, aggregation, and weighting methods used, and (9) if there uncertainty and validation analysis were performed. A complete list of the reviewed papers is presented in the Supplement.
4.1 Flood vulnerability indices at a glance
An increasing number of studies that built flood vulnerability indices can be observed in recent years, with about 80 % (n=76) of the articles being published since 2015 (Fig. 2a), which is also the year that the Sendai Framework for Disaster Risk Reduction (UNISDR, 2016) was agreed among several member states. Therefore, the growing number of publications may result from the increasing awareness of flood disaster prevention and reduction policies. The increasing number of vulnerability indices studies could also be attributed to the ease of using indices to address complex and multidimensional issues such as flood vulnerability in contrast to methods that demand more data (e.g., damage curves). Alternatively, this increase may just match a general rise in published papers. To investigate this, we calculated the increase in flood vulnerability studies in relative terms, based on a normalization according to the number of all flood publications in the WoS database. Results show that the increase in research on flood vulnerability indices is significantly greater than the increase in published flood articles (Appendix A; Fig. A1).
Overall, most of the assessments were conducted in Asia (45.3 %), followed by the Americas (24.2 %), and encompassing 38 countries (Fig. 2b). This was expected as, according to the Emergency Events Database (EM-DAT) statistics, between 2002 and 2019 Asia showed the highest number of deaths caused by floods (1027 deaths; CRED, 2019). As such, the studies are highly concentrated in a few countries, namely China (n=14), Brazil (n=8), India (n=6), Pakistan (n=6), and the United States (n=6). Meanwhile, there were fewer studies in East and West Africa, despite the frequent occurrence of floods and the high mortality they cause across these regions.
In terms of spatial scale, most of the studies were conducted at the neighborhood scale (62.1 %), followed by city (14.7 %), household (12.6 %), group of cities (7.4 %), various scales (2.1 %), and state scale (1.1 %). Similar outcomes were obtained by Diaz-Sarachaga and Jato-Espino (2020), who found out that vulnerability studies at national and regional scales are infrequent. The neighborhood scale was the dominant scale in all continents (Fig. 3) as it is the smallest unit for which data are available for large areas, generally through census data. Only eight studies (8.4 %) were conducted at the basin level (i.e., group of cities), and a few articles (n=2) conducted assessments across various scales. For instance, Balica et al. (2009) evaluated the vulnerability at the basin, subbasin, and city scales. Similarly, Remo et al. (2016) compared three scales (i.e., census blocks, communities, and counties) and found out that the results generally mirrored each other. None of the considered articles draw conclusions at the national or global level.
Around 40.0 % of the studies were applied to urban areas, 15.8 % to rural areas, and 44.2 % to both. The high prevalence of studies that consider both urban and rural areas is related to the data availability, as the census tracks usually encompass the entire perimeter of a municipality. At the neighborhood scale, most studies considered only urban areas (53.4 %; Fig. 4). Conversely, studies at the household scale were developed mainly in rural areas (58.3 %). This can be explained by a lower availability of detailed geospatial data in rural areas worldwide (Zhang and Zhu, 2018; Zielstra and Zipf, 2010). Therefore, in these cases, it is necessary to collect data via household surveys.
4.2 Indicators used to characterize flood vulnerability
Table 4 shows the most frequent indicators grouped into social, economic, physical, and coping capacity dimensions. In summary, social and economic indicators such as population density (37.9 %), illiteracy rate (32.6 %), unemployment rate (29.5 %), female rate (28.4 %), per capita income (25.3 %), and elderly rate (22.1 %) were the most commonly used vulnerability indicators (Table 4). This is similar to the results obtained by Rufat et al. (2015), who found out that the most used indicators are poverty and deprivation, per capita income, unemployment rate, the elderly, and children. Nevertheless, widely used indicators found by the authors were not identified or were rarely used in our sample. These include, for example, stress and mental health, hygiene and sanitation, social networks, and experience with floods (Schneiderbauer and Ehrlich, 2006).
Table 4Most commonly used flood vulnerability indicators. Only indicators used in at least five articles are shown here. This cut-off point was defined for clarity purposes as more than 600 different indicators were mentioned in the 95 reviewed articles.
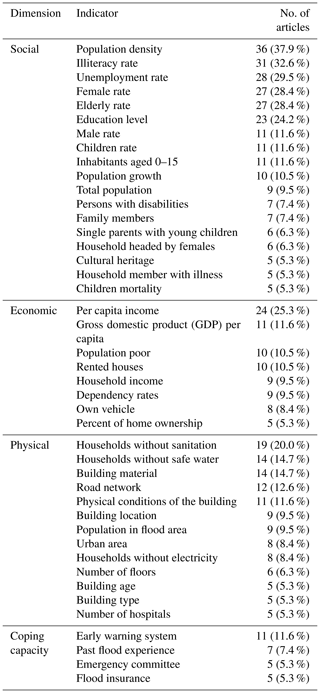
The studies used a median of 16 indicators. Although 32.6 % (n=31) of the studies used more than 20 indicators (e.g., Sam et al., 2017), most of them (58.0 %) did not apply any method for reducing the number of variables. Among the studies which conducted reduction, the most used technique was the PCA, which was applied to 35.5 % (n=11) of the indices that used more than 20 indicators (e.g., Aroca-Jimenez et al., 2017; Grosso et al., 2015; Török, 2018). In addition to PCA, some studies used other approaches, for example, based on expert opinion (e.g., de Brito et al., 2018) or based on indicators with a high Pearson correlation (e.g., Kotzee and Reyers, 2016).
4.3 Temporal dynamics
In order to identify if the articles included the temporal dynamics of vulnerability, the indices were classified into pre-event (before), event (during) and post-event (after; Kobiyama et al., 2006). Most of the studies focused on assessing past vulnerability trends (88.4 %) and only 12.6 % explored post-event flood vulnerability (e.g., Carlier et al., 2018; Miguez and Veról, 2017). None focused on the vulnerability during the event or elaborated on projections for future vulnerabilities.
The indicators used are different according to the temporal scale considered. Post-event indices encompassed human, economic, and material damages to quantify the flood vulnerability (Table 5). Variables such as mitigation, damages, and coping behavior after experiencing a flood were often considered (Abbas et al., 2018). For instance, Rogelis et al. (2016) compared the results of the most vulnerable areas by ranking the basins according to the observed impacts from highest to lowest damage in terms of number of fatalities, injured people, evacuated people, and number of affected houses.
4.4 Indicator normalization, weighting, and aggregation
Concerning the indicators normalization, the most used approach was min–max (30.5 %), followed by Z score (12.6 %) and distance from the group leader (12.6 %; Table 6a). A total of five studies applied other methods. For example, Aroca-Jimenez et al. (2017, 2018) expressed the indicators' values in percentage or per capita value, and de Brito et al. (2018) used fuzzy functions to normalize the indicators. It is important to note that most papers did not specify the normalization method used (11.6 %), which limits the reproducibility of the study results.
Among the weighing approach types, statistical methods were the most applied (30.5 %), especially the PCA method (21.1 %). The high use of PCA can be attributed to the pioneering work by Cutter et al. (2003), who recommended the use of a factor analytic approach. Other less common statistical methods include dividing the indicator values by the total or maximum value (Okazawa et al., 2011), hot spot analysis (Kubal et al., 2009), and the unequal weighting method (Kablan et al., 2017).
Many authors recommend the use of participatory methods for weighing the indicators, such as the use of multicriteria decision-making (MCDM) tools (Evers et al., 2018). It is assumed that, if practitioners and experts are involved in creating an index that they find useful, it is more likely that they will trust its results (Oulahen et al., 2015). Furthermore, participation is believed to be a key component in fostering effective disaster risk reduction (Fekete et al., 2021). In the present study, the analytical hierarchy process (AHP) was the most common MCDM technique, which corroborates the results by de Brito and Evers (2016). These authors attributed this preference to the fact that AHP is a straightforward and flexible method. This method was applied separately in 10 papers and together with other methods in five papers, totaling 16.0 % of the reviewed articles. Among the less common MCDM methods, Promethee (Preference Ranking Organization METHod for Enrichment of Evaluations; Daksiya et al., 2017) and the analytical network process (de Brito et al., 2018) techniques are worth mentioning.
A total of seven articles used other weighting methods, including the entropy method (Lianxiao and Morimoto, 2019), Delphi technique (Yang et al., 2018b), and expert scoring (Wu et al., 2015). Furthermore, about one-quarter (24.2 %) of the papers attributed equal weighting, and 6.3 % did not specify the weighting method used (Table 6b). Some authors preferred not to weight indicators because they assumed that these indicators are equally important for the vulnerability calculation (Yoon, 2012), whereas others pointed out that there is insufficient evidence to attribute importance to one factor over another (Fekete, 2011).
In terms of aggregation, the majority of the articles (80.0 %) used linear aggregation, followed by geometric aggregation (10.5 %) and mixed methods (4.2 %). The linear method is useful when all indicators have the same unit or after they have been normalized. The geometric aggregation is preferred when the interest is assessing the degree of non-compensation between the indicators. In linear aggregation, compensation is constant, while in geometric aggregation the compensation is lower for indices with low values (Nardo et al., 2008). Nevertheless, the geometric method has a limitation when indicators with very low scores are compensated by indicators with high scores (Gan et al., 2017).
It is important to mention other aggregation methods used (5.3 %). For instance, Abebe et al. (2018) used the Bayesian belief network, which is formed by a graphical network representing the cause–effect relationships between the different indicators (Pearl, 1988). Yang et al. (2018b, a) applied the Shannon entropy method. More recently, Amadio et al. (2019) used a non-compensatory aggregation method to compensate for the low performance of one indicator with some higher performance of another indicator. Finally, Chiu et al. (2014) used the fuzzy comprehensive evaluation method to aggregate the indicators.
4.5 Uncertainty, sensitivity, and validation
Each step in the construction of flood vulnerability indices carries uncertainty (Saisana et al., 2005), which is added to the ones associated with the randomness of flood events (Merz et al., 2008). Therefore, to ensure a better index quality and verify the results' robustness, uncertainty analysis should be conducted. Despite its importance, only three (3.2 %) of the reviewed papers performed uncertainty analysis. Nazeer and Bork (2019) observed variations in the final results that changed input variables, and Feizizadeh and Kienberger (2017) and Guo et al. (2014) applied a Monte Carlo simulation and set pair analysis, respectively.
With respect to the sensitivity analysis (SA), only nine papers (9.5 %) performed it. Most articles applied local SA by comparing the results obtained by changing input methods, such as choosing different weights (Müller et al., 2011; Nazeer and Bork, 2019; Rogelis et al., 2016), aggregation methods (Fernandez et al., 2016; Nazeer and Bork, 2019), or indicators (Rogelis et al., 2016; Zhang and You, 2014). In addition, Abebe et al. (2018) quantified sensitivity through variance reduction and mutual information. Spatial SA was conducted by de Brito et al. (2019) by computing the vulnerability class switches when the indicator weights were changed. Only Feizizadeh and Kienberger (2017) performed the global sensitivity analysis.
Although the number of flood vulnerability studies has increased, few studies attempted to validate the obtained outcomes (Fekete, 2009). Of the reviewed articles, only 11 (11.6 %) validated the results, mostly using maps with flooded areas (n=7), flood damage (n=3), and expert opinion (n=1).
Despite the increasing amount of research on flood vulnerability indices since 2015, a series of persistent knowledge gaps of methodological nature were found in our systematic review (Table 7). Here, we summarize these gaps and provide a research agenda with needs that should be addressed in the future.
The first challenge refers to the spatial scale, as vulnerability is not only site specific but also scale dependent (Ciurean et al., 2013). The choice of the spatial scale in the reviewed articles was mostly driven by data availability, and hence, most of them were applied at the neighborhood level using census tracks. Despite the availability of census data at the country level, there were no studies at the national level, and only eight papers (8.4 %) constructed vulnerability indices using data at the basin scale. Nevertheless, these scales are often used for flood risk management and are necessary to enable the comparability of regions and to define hot spot areas where intervention is needed (Balica et al., 2009; Fekete et al., 2010). Conversely, studies at the household level were rare in our sample (n=12). Yet, aspects related to the citizens' coping capacities can only be captured at this spatial scale.
An additional issue is the problem of down- or upscaling that implies different levels of generalization. Hence, multilevel and cross-scale studies are needed. They allow for a better understanding of scale implications, including their benefits and drawbacks. A better understanding of urban–rural linkages is also required, instead of studying them in isolation. To this end, the framework proposed by Jamshed et al. (2020) could be used. This framework considers, either qualitatively or quantitatively, how rural–urban linkages can influence the occurrence of floods and how these shape the vulnerability of rural households. It considers rural areas not as secluded units but rather as being interlinked with cities.
A further gap is that indicators related to the populations' coping and adaptive capacity were rarely used. The focus was given to social indicators that increase people's vulnerability. Similar to the scale choice, the preference for these indicators is driven due to data availability, as social indicators (e.g., age and gender) are easily accessible. Nevertheless, the capacity of people to anticipate, cope with, resist, and recover from disasters is equally important for understanding the risk. In fact, even poor and vulnerable people have the capacity to cope (Wisner et al., 2012). Therefore, when dealing with flood vulnerability, other relevant indicators, such as risk perception (Carlier et al., 2018) and past flood experience (Beringer and Kaewsuk, 2018), are important. However, data on these are often not readily available, thus requiring local research, which demands time, financial resources, and a multidisciplinary team. Indeed, information on citizens' adaptive behavior and perception requires longitudinal or quasi-experimental studies that allow the capturing of behavioral dynamics over time (Kuhlicke et al., 2020). For instance, recent advancements have been made by applying geostatistical methods to psychosocial survey data (Guardiola-Albert et al., 2020). As an alternative, people's risk perception could be derived from widely available data sources, including, for instance, Google trends (e.g., Kam et al. 2019) and Twitter statistics (Dyer and Kolic, 2020). Nevertheless, it should be noted that such approaches can be considered only where the use of social media and search engines are prevalent across the society. In countries where the use of digital technologies is not widespread, there is the risk that the marginalized population is left out of the analysis.
Table 7Summary of the identified knowledge gaps and need for building flood vulnerability indicators.
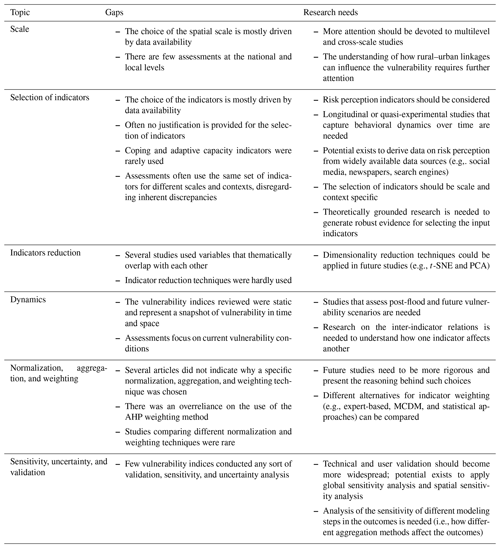
Still with regard to the indicators used, many studies used variables that thematically overlap with each other. In this context, some indices used more than 20 indicators to measure flood vulnerability and did not apply any technique (e.g., PCA or expert based) to reduce this number. This can decrease the explanatory power of the index. In this context, besides PCA, the potential exists to apply dimensionality reduction techniques such as the t-distributed stochastic neighbor embedding (t-SNE; Anowar et al., 2021). A further issue is that the reason for variable selection was often not given, or it was justified based on previous studies, without taking into consideration the study area specificities or conceptual frameworks. Due to the difficulty and time involved in developing indicators, low-quality databases are normally used (Freudenberg, 2003). For adequate indicator selection, the analytical soundness, measurability, relevance to the phenomenon being measured, and the relationships to each other (e.g., interrelationships and feedback loops) should be taken into account. Furthermore, more theoretically grounded research is needed to generate robust evidence for selecting the input indicators.
All of the vulnerability indices reviewed here are static and represent a snapshot of vulnerability. Hence, they do not capture the complexities and temporal dynamics of vulnerability. Few studies focused on measuring flood vulnerability post-event. Nevertheless, the drivers of vulnerability can vary considerably over time. Results by Kuhlicke et al. (2011) and Reiter et al. (2018) show that the exposed citizens (e.g., the elderly and children) may be less vulnerable during the preparatory phase of a flood but highly vulnerable during the recovery phase. Hence, incorporating the phase of the flood disaster is key for improving the validity of vulnerability indices (Rufat et al., 2015). To account for temporal context, the indicators can be differentiated according to the different phases of a disaster, i.e., preparedness, response, and recovery phases. Such a phase-oriented approach could inform variable selection and weighting. In addition to this, there is a need for research looking into future vulnerabilities, and a forward-looking perspective is needed for preventive flood risk reduction (Birkmann et al., 2013; Garschagen and Kraas, 2010). These could make use of, for instance, population growth projections or employ tools such as qualitative futuring techniques (Hoffman et al., 2021). Nevertheless, it is important to notice that this can further increase the uncertainty of the vulnerability modeling outcomes. Still, exercises like this can provide a glimpse of plausible futures.
Similar to the selection of the indicators, several articles did not indicate why a specific normalization and weighting technique was chosen. Additionally, some did not explicitly specify any normalization (11.6 %) or weighting (6.3 %) method. Nevertheless, the use of arbitrary techniques without testing different methods and their assumptions increases the subjective judgment error. Hence, it is imperative for future studies to be more rigorous and present the reasoning behind such choices. Furthermore, there was an overreliance on the use of the AHP weighting method, and studies comparing different normalization and weighting techniques were rare (7.4 %). Future research should tackle this by exploring different alternatives for evaluating indicator weights (e.g., expert-based, MCDM, or statistical approaches) and compare the findings by means of validation and sensitivity analyses.
A final persisting gap is that few vulnerability indices conducted any sort of validation, sensitivity, or uncertainty analysis. Fewer than 14 % of the studies validated the obtained results. To this end, impact data were often used (e.g., Rezende et al., 2019). Only 9.5 % conducted sensitivity or uncertainty analysis. This can lead to vulnerability outputs that are incoherent with the local reality by either over- or underestimating the vulnerability. This, in turn, has direct implications for flood risk management. In this regard, Fekete (2009) points out the difficulty in finding empirical evidence about vulnerability because vulnerability is multidimensional and not directly observable. Thus, further research is needed on the validation of vulnerability outcomes (including technical and user validation) and analysis of the sensitivity of the contribution of individual indicators to the obtained results. The potential exists to apply a global sensitivity analysis, which is already widely applied for building composite indicators for other fields of study (Luan et al., 2017; Saisana and Saltelli, 2008).
Besides the aforementioned methodological gaps, it is important to emphasize that the theoretical framework adopted influences the methodological choices that are made when constructing vulnerability indices. Even though we have not analyzed the theoretical constructs used by each study, when reading the articles it became clear that several of them do not specify how they conceptualize vulnerability. Furthermore, there are ambiguities in how vulnerability is understood (Kelman, 2018). For instance, some authors consider coping and adaptive capacity as components of flood vulnerability (e.g., de Brito et al., 2018; Feizizadeh and Kienberger, 2017). Others include flood hazard characteristics or exposure (e.g., Carlier et al., 2018; Chaliha et al., 2012) as part of vulnerability. Hence, we argue that a stronger theoretical underpinning of research is needed for producing scientifically rigorous and comparable research. Within this context, future work could investigate how different terminologies and theoretical constructs are defined and applied across different flood vulnerability case studies. Future reviews could also look into the methodology used to collect information on vulnerability indicators (e.g., surveys and public databases) as this influences the choices that can be made at each stage of the index construction.
The present study reviewed 95 articles from 38 countries that constructed flood vulnerability indices. In summary, despite the increasing number of studies and advances made, the review has revealed and reconfirmed a number of persistent knowledge gaps. Temporal dynamic aspects of vulnerability were often disregarded. Only 11.6 % of the studies focused on indicators that address post-event conditions related to flood damage and consequences, and none of them investigated future vulnerabilities. Coping and adaptive capacity indicators were frequently ignored, as obtaining these data demands time and effort. Most did not apply sensitivity (90.5 %) and uncertainty analyses (96.8 %) nor did they perform results validation (86.3 %). This demonstrates a limitation in the reliability of these indices. It is clear from the literature that the challenge for further research is to foster the development of dynamic vulnerability assessments that consider the coping capacity of citizens and take the uncertainty involved in all steps of the index building process into account, including the selection of indicators, normalization, weighting, and aggregation. This is required in order to advance our understanding of flood vulnerability and support pathways towards flood risk reduction.
The underlying research data are available in the Supplement.
The supplement related to this article is available online at: https://doi.org/10.5194/nhess-21-1513-2021-supplement.
LLM contributed to the conceptualization, data curation, investigation, methodology and writing (original draft preparation and review and editing). MMB contributed to the methodology, supervision, validation and writing (review and editing). MK contributed to project administration, methodology, supervision and writing (review and editing).
The authors declare that they have no conflict of interest.
The authors thank CNPq for the research scholarship.
This research has been supported by the Conselho Nacional de Desenvolvimento Científico e Tecnológico (grant no. 141387/2019-0).
The article processing charges for this open-access publication were covered by the Helmholtz Centre for Environmental Research – UFZ.
This paper was edited by Sven Fuchs and reviewed by three anonymous referees.
Abbas, A., Amjath-Babu, T. S., Kächele, H., Usman, M., Amjed Iqbal, M., Arshad, M., Adnan Shahid, M., and Müller, K.: Sustainable survival under climatic extremes: linking flood risk mitigation and coping with flood damages in rural Pakistan, Environ. Sci. Pollut. R., 25, 32491–32505, https://doi.org/10.1007/s11356-018-3203-8, 2018.
Abebe, Y., Kabir, G., and Tesfamariam, S.: Assessing urban areas vulnerability to pluvial flooding using GIS applications and Bayesian Belief Network model, J. Clean. Prod., 174, 1629–1641, https://doi.org/10.1016/j.jclepro.2017.11.066, 2018.
Ahmad, D. and Afzal, M.: Household vulnerability and resilience in flood hazards from disaster-prone areas of Punjab, Pakistan, Nat. Hazards, 99, 337–354, https://doi.org/10.1007/s11069-019-03743-9, 2019.
Amadio, M., Mysiak, J., and Marzi, S.: Mapping Socioeconomic Exposure for Flood Risk Assessment in Italy, Risk Anal., 39, 829–845, https://doi.org/10.1111/risa.13212, 2019.
Anowar, F., Sadaoui, S., and Selim, B.: Conceptual and empirical comparison of dimensionality reduction algorithms (PCA, KPCA, LDA, MDS, SVD, LLE, ISOMAP, LE, ICA, t-SNE), Comput. Sci. Rev., 40, 100378, https://doi.org/10.1016/j.cosrev.2021.100378, 2021.
Aroca-Jimenez, E., Bodoque, J. M., Garcia, J. A., and Diez-Herrero, A.: Construction of an integrated social vulnerability index in urban areas prone to flash flooding, Nat. Hazards Earth Syst. Sci., 17, 1541–1557, https://doi.org/10.5194/nhess-17-1541-2017, 2017.
Aroca-Jiménez, E., Bodoque, J. M., García, J. A., and Díez-Herrero, A.: A quantitative methodology for the assessment of the regional economic vulnerability to flash floods, J. Hydrol., 565, 386–399, https://doi.org/10.1016/j.jhydrol.2018.08.029, 2018.
Aroca-Jiménez, E., Bodoque, J. M., and García, J. A.: How to construct and validate an Integrated Socio-Economic Vulnerability Index: Implementation at regional scale in urban areas prone to flash flooding, Sci. Total Environ., 746, 140905, https://doi.org/10.1016/j.scitotenv.2020.140905, 2020.
Baeck, S. H., Choi, S. J., Choi, G. W., and Lee, D. R.: A study of evaluating and forecasting watersheds using the flood vulnerability assessment index in Korea, Geomatics, Nat. Hazards Risk, 5, 208–231, https://doi.org/10.1080/19475705.2013.803268, 2014.
Balica, S. F., Douben, N., and Wright, N. G.: Flood vulnerability indices at varying spatial scales, Water Sci. Technol., 60, 2571–2580, https://doi.org/10.2166/wst.2009.183, 2009.
Balica, S. F., Popescu, I., Beevers, L., and Wright, N. G.: Parametric and physically based modelling techniques for flood risk and vulnerability assessment: A comparison, Environ. Model. Softw., 41, 84–92, https://doi.org/10.1016/j.envsoft.2012.11.002, 2013.
Beringer, A. L. and Kaewsuk, J.: Emerging livelihood vulnerabilities in an urbanizing and climate uncertain environment for the case of a secondary city in Thailand, Sustainability, 10, 1452, https://doi.org/10.3390/su10051452, 2018.
Bertilsson, L., Wiklund, K., de Moura Tebaldi, I., Rezende, O. M., Veról, A. P., and Miguez, M. G.: Urban flood resilience – A multi-criteria index to integrate flood resilience into urban planning, J. Hydrol., 573, 970–982, https://doi.org/10.1016/j.jhydrol.2018.06.052, 2019.
Birkmann, J.: Indicators and criteria for measuring vulnerability: Theoretical bases and requirements, in: Measuring Vulnerability to Natural Hazards: Towards Disaster Resilient Societies, vol. 02, United Nations University Press, Tokyo, New York, Paris, 55–77, 2006.
Birkmann, J., Cardona, O. D., Carreño, M. L., Barbat, A. H., Pelling, M., Schneiderbauer, S., Kienberger, S., Keiler, M., Alexander, D., Zeil, P., and Welle, T.: Framing vulnerability, risk and societal responses: the MOVE framework, Nat. Hazards, 67, 193–211, https://doi.org/10.1007/s11069-013-0558-5, 2013.
Bründl, M., Romang, H. E., Bischof, N., and Rheinberger, C. M.: The risk concept and its application in natural hazard risk management in Switzerland, Nat. Hazards Earth Syst. Sci., 9, 801–813, https://doi.org/10.5194/nhess-9-801-2009, 2009.
Cardona, O.-D., Aalst, M. K. van, Birkmann, J., Fordham, M., McGregor, G., Perez, R., Pulwarty, R. S., Schipper, E. L. F., and Sinh, B. T.: Determinants of risk: Exposure and vulnerability, in: Managing the Risks of Extreme Events and Disasters to Advance Climate Change Adaptation, vol. 34, Cambridge University Press, Cambridge, UK, and New York, NY, USA, 65–108, 2012.
Carlier, B., Puissant, A., Dujarric, C., and Arnaud-Fassetta, G.: Upgrading of an index-oriented methodology for consequence analysis of natural hazards: application to the Upper Guil catchment (southern French Alps), Nat. Hazards Earth Syst. Sci., 18, 2221–2239, https://doi.org/10.5194/nhess-18-2221-2018, 2018.
Chaliha, S., Sengupta, A., Sharma, N., and Ravindranath, N. H.: Climate variability and farmer's vulnerability in a flood-prone district of Assam, Int. J. Clim. Chang. Str., 4, 179–200, https://doi.org/10.1108/17568691211223150, 2012.
Chiu, R., Lin, L., and Ting, S.: Evaluation of green port factors and performance: a fuzzy AHP analysis, Math. Probl. Eng., 2014, 1–12, 2014.
Ciurean, R. L., Schröter, D., and Glade, T.: Conceptual Frameworks of Vulnerability Assessments for Natural Disasters Reduction, in Approaches to Disaster Management – Examining the Implications of Hazards, Emergencies and Disasters completely, Intech., London, 31 pp., 2013.
CRED: EM-DAT: The international disasters database, available at: https://www.emdat.be/database, last access: 8 December 2019.
Cutter, S. L. and Derakhshan, S.: Temporal and spatial change in disaster resilience in US counties, 2010–2015, Environ. Hazards, 19, 10–29, https://doi.org/10.1080/17477891.2018.1511405, 2020.
Cutter, S. L., Boruff, B. J., and Shirley, W. L.: Social vulnerability to environmental hazards, Soc. Sci. Quart., 84, 242–261, https://doi.org/10.1111/1540-6237.8402002, 2003.
Daksiya, V., Su, H. T., Chang, Y. H., and Lo, E. Y. M.: Incorporating socio-economic effects and uncertain rainfall in flood mitigation decision using MCDA, Nat. Hazards, 87, 515–531, https://doi.org/10.1007/s11069-017-2774-x, 2017.
de Andrade, M. M. N. and Szlafsztein, C. F.: Vulnerability assessment including tangible and intangible components in the index composition: An Amazon case study of flooding and flash flooding, Sci. Total Environ., 630, 903–912, https://doi.org/10.1016/j.scitotenv.2018.02.271, 2018.
Debortoli, N. S., Camarinha, P. I. M., Marengo, J. A., and Rodrigues, R. R.: An index of Brazil's vulnerability to expected increases in natural flash flooding and landslide disasters in the context of climate change, Nat. Hazards, 86, 557–582, https://doi.org/10.1007/s11069-016-2705-2, 2017.
de Brito, M. M. and Evers, M.: Multi-criteria decision-making for flood risk management: a survey of the current state of the art, Nat. Hazards Earth Syst. Sci., 16, 1019–1033, https://doi.org/10.5194/nhess-16-1019-2016, 2016.
de Brito, M. M., Evers, M., and Höllermann, B.: Prioritization of flood vulnerability, coping capacity and exposure indicators through the Delphi technique: A case study in Taquari-Antas basin, Brazil, Int. J. Disast. Risk Re., 24, 119–128, https://doi.org/10.1016/j.ijdrr.2017.05.027, 2017.
de Brito, M. M., Evers, M., and Almoradie, A. D. S.: Participatory flood vulnerability assessment: a multi-criteria approach, Hydrol. Earth Syst. Sci., 22, 373–390, https://doi.org/10.5194/hess-22-373-2018, 2018.
de Brito, M. M., Almoradie, A., and Evers, M.: Spatially-explicit sensitivity and uncertainty analysis in a MCDA-based flood vulnerability model, Int. J. Geogr. Inf. Sci., 33, 1788–1806, https://doi.org/10.1080/13658816.2019.1599125, 2019.
Diaz-Sarachaga, J. M. and Jato-Espino, D.: Analysis of vulnerability assessment frameworks and methodologies in urban areas, Nat. Hazards, 100, 437–457, https://doi.org/10.1007/s11069-019-03805-y, 2020.
Dilley, M., Chen, R. S., Deichmann, U., Lerner-Lam, A., Arnold, M., Agwe, J., Buys, P., Kjekstad, O., Lyon, B., and Yetman, G.: Natural disaster hotspots: A global risk analysis, World Bank and Columbia University, Washington, D.C., 2005.
Dyer, J. and Kolic, B.: Public risk perception and emotion on Twitter during the Covid-19 pandemic, Appl. Netw. Sci., 5, 99, https://doi.org/10.1007/s41109-020-00334-7, 2020.
Evers, M., Almoradie, A., and de Brito, M. M.: Enhancing Flood Resilience Through Collaborative Modelling and Multi-criteria Decision Analysis (MCDA), in: The Urban Book Series, Springer International Publishing, New York, 221–236, 2018.
Fatemi, F., Ardalan, A., Aguirre, B., Mansouri, N., and Mohammadfam, I.: Social vulnerability indicators in disasters: Findings from a systematic review, Int. J. Disast. Risk Re., 22, 219–227, https://doi.org/10.1016/j.ijdrr.2016.09.006, 2017.
Fedeski, M. and Gwilliam, J.: Urban sustainability in the presence of flood and geological hazards: The development of a GIS-based vulnerability and risk assessment methodology, Landscape Urban Plan., 83, 50–61, https://doi.org/10.1016/j.landurbplan.2007.05.012, 2007.
Feizizadeh, B. and Kienberger, S.: Spatially explicit sensitivity and uncertainty analysis for multicriteria-based vulnerability assessment, J. Environ. Plann. Man., 60, 2013–2035, https://doi.org/10.1080/09640568.2016.1269643, 2017.
Fekete, A.: Validation of a social vulnerability index in context to river-floods in Germany, Journal of the British Academy, 9, 393–403, https://doi.org/10.5871/jba/001.151, 2009.
Fekete, A.: Spatial disaster vulnerability and risk assessments: Challenges in their quality and acceptance, Nat. Hazards, 61, 1161–1178, https://doi.org/10.1007/s11069-011-9973-7, 2011.
Fekete, A., Damm, M. and Birkmann, J.: Scales as a challenge for vulnerability assessment, Nat. Hazards, 55, 729–747, https://doi.org/10.1007/s11069-009-9445-5, 2010.
Fekete, A., Aslam, A. B., de Brito, M. M., Dominguez, I., Fernando, N., Illing, C. J., KC, A. K., Mahdavian, F., Norf, C., Platt, S., Santi, P. A., and Tempels, B.: Increasing flood risk awareness and warning readiness by participation – But who understands what under `participation'?, Int. J. Disast. Risk Re., 57, 102157, https://doi.org/10.1016/j.ijdrr.2021.102157, 2021.
Fernandez, P., Mourato, S., Moreira, M., and Pereira, L.: A new approach for computing a flood vulnerability index using cluster analysis, Phys. Chem. Earth, 94, 47–55, https://doi.org/10.1016/j.pce.2016.04.003, 2016.
Freudenberg, M.: Composite Indicators of Country Performance: A Critical Assessment, Organisation for Economic Co-operation and Development, Paris, 2003.
Fuchs, S., Kuhlicke, C. and Meyer, V.: Editorial for the special issue: Vulnerability to natural hazards-the challenge of integration, Nat. Hazards, 58, 609–619, https://doi.org/10.1007/s11069-011-9825-5, 2011.
Gan, X., Fernandez, I. C., Guo, J., Wilson, M., Zhao, Y., Zhou, B., and Wu, J.: When to use what: Methods for weighting and aggregating sustainability indicators, Ecol. Indic., 81, 491–502, https://doi.org/10.1016/j.ecolind.2017.05.068, 2017.
Garbutt, K., Ellul, C., and Fujiyama, T.: Mapping social vulnerability to flood hazard in Norfolk, England, Environ. Hazards, 14, 156–186, https://doi.org/10.1080/17477891.2015.1028018, 2015.
Garschagen, M. and Kraas, F.: Assessing Future Resilience to Natural Hazards – The Challenge of Capturing Dynamic Changes under Conditions of Transformation and Climate Change, in: International Disaster and Risk Conference, IDRC, Davos, 2010.
Gerrard, R. E. C.: Developing an index of community competence in flood response for flood-affected rural parishes on the Somerset Levels and Moors using composite and spatial datasets, Area, 50, 344–352, https://doi.org/10.1111/area.12416, 2018.
Grosso, N., Dias, L., Costa, H. P., Santos, F. D., and Garrett, P.: Continental Portuguese Territory Flood Social Susceptibility Index, Nat. Hazards Earth Syst. Sci., 15, 1921–1931, https://doi.org/10.5194/nhess-15-1921-2015, 2015.
Gu, H., Du, S., Liao, B., Wen, J., Wang, C., Chen, R., and Chen, B.: A hierarchical pattern of urban social vulnerability in Shanghai, China and its implications for risk management, Sustain. Cities Soc., 41, 170–179, https://doi.org/10.1016/j.scs.2018.05.047, 2018.
Guardiola-Albert, C., Díez-Herrero, A., Amerigo Cuervo-Arango, M., Bodoque, J. M., García, J. A., Naranjo-Fernández, N., and Aroca-Jiménez, E.: Analysing flash flood risk perception through a geostatistical approach in the village of Navaluenga, Central Spain, J. Flood Risk Manag., 13, 1–16, https://doi.org/10.1111/jfr3.12590, 2020.
Guo, E., Zhang, J., Ren, X., Zhang, Q., and Sun, Z.: Integrated risk assessment of flood disaster based on improved set pair analysis and the variable fuzzy set theory in central Liaoning Province, China, Nat. Hazards, 74, 947–965, https://doi.org/10.1007/s11069-014-1238-9, 2014.
Hernández-Uribe, R. E., Barrios-Piña, H., and Ramírez, A. I.: Análisis de riesgo por inundación: Metodología y aplicación a la cuenca Atemajac, Tecnol. Cienc. Agua, 8, 5–25, 2017.
Hirsch, R. M. and Archfield, S. A.: Not higher but more often, Nat. Clim. Change, 5, 198–199, https://doi.org/10.1038/nclimate2551, 2015.
Hoffman, J., Pelzer, P., Albert, L., Béneker, T., Hajer, M., and Mangnus, A.: A futuring approach to teaching wicked problems, J. Geogr. High. Educ., https://doi.org/10.1080/03098265.2020.1869923, in press, 2021.
IPCC: Climate Change 2001: Impacts, Adaptation, and Vulnerability, 1st edn., edited by: McCarthy, J. J., Canziani, O. F., Leary, N. A., Dokken, D. J., and White, K. S., Cambridge University Press, Cambridge, 2001.
IPCC: Managing the Risks of Extreme Events and Disasters to Advance Climate Change Adaptation. A Special Report of Working Groups I and II of the Intergovernmental Panel on Climate Change, edited by: Field, C. B., Barros, V., Stocker, T. F., Qin, D., Dokken, D. J., Ebi, K. L., Mastrandrea, M. D., Mach, K. J., Plattner, G., Allen, S. K., Tignor, M., Midgley, P. M., Cambridge University Press, New York, 2012.
IPCC: Climate change 2014: Impacts, Adaptation, and Vulnerability, Cambridge University Press, Cambridge, UK and New York, USA, 2014.
Jacobs, R., Smith, P., and Goddard, M.: Measuring performance: An examination of composite performance indicators, Centre for Health Economics University of York, York, 2004.
Jamshed, A., Rana, I. A., Mirza, U. M., and Birkmann, J.: Assessing relationship between vulnerability and capacity: An empirical study on rural flooding in Pakistan, Int. J. Disast. Risk Re., 36, 101109, https://doi.org/10.1016/j.ijdrr.2019.101109, 2019.
Jamshed, A., Birkmann, J., Feldmeyer, D., and Rana, I. A.: A Conceptual Framework to Understand the Dynamics of Rural–Urban Linkages for Rural Flood Vulnerability, Sustainability, 12, 2894, https://doi.org/10.3390/su12072894, 2020.
Jha, R. K. and Gundimeda, H.: An integrated assessment of vulnerability to floods using composite index – A district level analysis for Bihar, India, Int. J. Disast. Risk Re., 35, 101074, https://doi.org/10.1016/j.ijdrr.2019.101074, 2019.
Jurgilevich, A., Räsänen, A., Groundstroem, F., and Juhola, S.: A systematic review of dynamics in climate risk and vulnerability assessments, Environ. Res. Lett., 12, 013002, https://doi.org/10.1088/1748-9326/aa5508, 2017.
Kablan, M. K. A., Dongo, K., and Coulibaly, M.: Assessment of social vulnerability to flood in urban Côte d'Ivoire using the MOVE framework, Water, 9, 292, https://doi.org/10.3390/w9040292, 2017.
Kam, J., Stowers, K., and Kim, S.: Monitoring of Drought Awareness from Google Trends: A Case Study of the 2011–17 California Drought, Weather. Clim. Soc., 11, 419–429, https://doi.org/10.1175/WCAS-D-18-0085.1, 2019.
Kappes, M. S., Papathoma-Köhle, M., and Keiler, M.: Assessing physical vulnerability for multi-hazards using an indicator-based methodology, Appl. Geogr., 32, 577–590, https://doi.org/10.1016/j.apgeog.2011.07.002, 2012.
Kelman, I.: Lost for Words Amongst Disaster Risk Science Vocabulary?, Int. J. Disast. Risk Sc., 9, 281–291, https://doi.org/10.1007/s13753-018-0188-3, 2018.
Kobiyama, M., Mendonça, M., Moreno, D. A., Marcelino, I. P. V. de O., Marcelino, E. V., Gonçalves, E. F., Brazetti, L. L. P., Goerl, R. F., Molleri, G. S. F., and Rudorff, F. de M.: Prevenção de desastres naturais: conceitos básicos, 1st edn., Organic Trading, Florianópolis, 2006.
Koks, E. E., Jongman, B., Husby, T. G., and Botzen, W. J. W.: Combining hazard, exposure and social vulnerability to provide lessons for flood risk management, Environ. Sci. Policy, 47, 42–52, https://doi.org/10.1016/j.envsci.2014.10.013, 2015.
Kontokosta, C. E. and Malik, A.: The Resilience to Emergencies and Disasters Index: Applying big data to benchmark and validate neighborhood resilience capacity, Sustain. Cities Soc., 36, 272–285, https://doi.org/10.1016/j.scs.2017.10.025, 2018.
Kotzee, I. and Reyers, B.: Piloting a social-ecological index for measuring flood resilience: A composite index approach, Ecol. Indic., 60, 45–53, https://doi.org/10.1016/j.ecolind.2015.06.018, 2016.
Kubal, C., Haase, D., Meyer, V., and Scheuer, S.: Integrated urban flood risk assessment – adapting a multicriteria approach to a city, Nat. Hazards Earth Syst. Sci., 9, 1881–1895, https://doi.org/10.5194/nhess-9-1881-2009, 2009.
Kuhlicke, C., Scolobig, A., Tapsell, S., Steinführer, A., and De Marchi, B.: Contextualizing social vulnerability: findings from case studies across Europe, Nat. Hazards, 58, 789–810, https://doi.org/10.1007/s11069-011-9751-6, 2011.
Kuhlicke, C., Seebauer, S., Hudson, P., Begg, C., Bubeck, P., Dittmer, C., Grothmann, T., Heidenreich, A., Kreibich, H., Lorenz, D. F., Masson, T., Reiter, J., Thaler, T., Thieken, A. H., and Bamberg, S.: The behavioral turn in flood risk management, its assumptions and potential implications, WIREs Water, 7, 1–22, https://doi.org/10.1002/wat2.1418, 2020.
Leung, J. Y. S., Russell, B. D., and Connell, S. D.: Summary for Policymakers, available at: https://www.ipcc.ch/site/assets/uploads/sites/2/2019/05/SR15_SPM_version_report_LR.pdf (last access: 10 May 2021), 2019.
Lianxiao and Morimoto, T.: Spatial analysis of social vulnerability to floods based on the MOVE framework and information entropy method: Case study of Katsushika Ward, Tokyo, Sustainability, 11, 529, https://doi.org/10.3390/su11020529, 2019.
Luan, W., Lu, L., Li, X., and Ma, C.: Weight Determination of Sustainable Development Indicators Using a Global Sensitivity Analysis Method, Sustainability, 9, 303, https://doi.org/10.3390/su9020303, 2017.
Merz, V. B., Kreibich, H., and Apel, H.: Flood risk analysis: Uncertainties and validation, Österreichische Wasser- und Abfallwirtschaft, 60, 89–94, https://doi.org/10.1007/s00506-008-0001-4, 2008.
Miguez, M. G. and Veról, A. P.: A catchment scale Integrated Flood Resilience Index to support decision making in urban flood control design, Environ. Plann. B, 44, 925–946, https://doi.org/10.1177/0265813516655799, 2017.
Moreira, L. L., de Brito, M. M., and Kobiyama, M.: Effects of Different Normalization, Aggregation, and Classification Methods on the Construction of Flood Vulnerability Indexes, Water, 13, 98, https://doi.org/10.3390/w13010098, 2021.
Müller, A., Reiter, J., and Weiland, U.: Assessment of urban vulnerability towards floods using an indicator-based approach – a case study for Santiago de Chile, Nat. Hazards Earth Syst. Sci., 11, 2107–2123, https://doi.org/10.5194/nhess-11-2107-2011, 2011.
Munyai, R. B., Musyoki, A., and Nethengwe, N. S.: An assessment of flood vulnerability and adaptation: A case study of Hamutsha-Muungamunwe village, Makhado municipality, Jamba J. Disaster Risk Stud., 11, 1–8, https://doi.org/10.4102/jamba.v11i2.692, 2019.
Nardo, M., Saisana, M., Saltelli, A., and Tarantola, S.: Handbook of Contructing Compsoite Indicators: Methodology and user guide, Secretary-General of the OECD, Paris, 2008.
Nasiri, H., Mohd Yusof, M. J., and Mohammad Ali, T. A.: An overview to flood vulnerability assessment methods, Sustain. Water Resour. Manag., 2, 331–336, https://doi.org/10.1007/s40899-016-0051-x, 2016.
Nazeer, M. and Bork, H. R.: Flood vulnerability assessment through different methodological approaches in the context of North-West Khyber Pakhtunkhwa, Pakistan, Sustainability, 11, 6695, https://doi.org/10.3390/su11236695, 2019.
Okazawa, Y., Yeh, P. J.-F., Kanae, S., and Oki, T.: Development of a global flood risk index based on natural and socio-economic factors, Hydrolog. Sci. J., 56, 789–804, https://doi.org/10.1080/02626667.2011.583249, 2011.
Oulahen, G., Mortsch, L., Tang, K., and Harford, D.: Unequal Vulnerability to Flood Hazards: “Ground Truthing” a Social Vulnerability Index of Five Municipalities in Metro Vancouver, Canada, Ann. Assoc. Am. Geogr., 105, 473–495, https://doi.org/10.1080/00045608.2015.1012634, 2015.
Papathoma-Köhle, M., Keiler, M., Totschnig, R., and Glade, T.: Improvement of vulnerability curves using data from extreme events: Debris flow event in South Tyrol, Nat. Hazards, 64, 2083–2105, https://doi.org/10.1007/s11069-012-0105-9, 2012.
Papathoma-Köhle, M., Gems, B., Sturm, M., and Fuchs, S.: Matrices, curves and indicators: A review of approaches to assess physical vulnerability to debris flows, Earth-Sci. Rev., 171, 272–288, https://doi.org/10.1016/j.earscirev.2017.06.007, 2017.
Pearl, J.: Probabilistic Reasoning in Intelligent Systems: Networks of Plausible Inference, Morgan Kaufmann Publishers, United States, 1988.
Rehman, S., Sahana, M., Hong, H., Sajjad, H., and Ahmed, B. Bin: A systematic review on approaches and methods used for flood vulnerability assessment: framework for future research, Nat. Hazards, 96, 975–998, https://doi.org/10.1007/s11069-018-03567-z, 2019.
Reiter, J., Wenzel, B., Dittmer, C., Lorenz, D. F., and Voss, M.: The 2013 flood in the community of Elbe-Havel-Land in the eyes of the population, Research report of the quantitative survey, KFS Working Paper, KFS, Berlin, 2018.
Remo, J. W. F., Pinter, N., and Mahgoub, M.: Assessing Illinois's flood vulnerability using Hazus-MH, Nat. Hazards, 81, 265–287, https://doi.org/10.1007/s11069-015-2077-z, 2016.
Rezende, O. M., de Franco, A. B. R. da C., de Oliveira, A. K. B., Jacob, A. C. P., and Miguez, M. G.: A framework to introduce urban flood resilience into the design of flood control alternatives, J. Hydrol., 576, 478–493, https://doi.org/10.1016/j.jhydrol.2019.06.063, 2019.
Rogelis, M. C., Werner, M., Obregón, N., and Wright, N.: Regional prioritisation of flood risk in mountainous areas, Nat. Hazards Earth Syst. Sci., 16, 833–853, https://doi.org/10.5194/nhess-16-833-2016, 2016.
Rufat, S., Tate, E., Burton, C. G., and Maroof, A. S.: Social vulnerability to floods: Review of case studies and implications for measurement, Int. J. Disast. Risk Re., 14, 470–486, https://doi.org/10.1016/j.ijdrr.2015.09.013, 2015.
Saisana, M. and Saltelli, A.: Expert Panel Opinion and Global Sensitivity Analysis for Composite Indicators, in: Computational Methods in Transport: Verification and Validation, Lecture Notes in Computational Science and Engineering, vol. 62, edited by: Graziani, F., Springer, Berlin, Heidelberg, https://doi.org/10.1007/978-3-540-77362-7_11, 2008.
Saisana, M. and Tarantola, S.: State-of-the-art Report on Current Methodologies and Practices for Composite Indicator Development, European Commission Joint Research Centre, Ispra, 1–72, 2002.
Saisana, M., Tarantola, S., and Saltelli, A.: Uncertainty and sensitivity techniques as tools for the analysis and validation of composite indicators, J. R. Stat. Soc., 168, 307–323, 2005.
Sam, A. S., Kumar, R., Kächele, H., and Müller, K.: Vulnerabilities to flood hazards among rural households in India, Nat. Hazards, 88, 1133–1153, https://doi.org/10.1007/s11069-017-2911-6, 2017.
Schmidtlein, M. C., Deutsch, R. C., Piegorsch, W. W., and Cutter, S. L.: A Sensitivity Analysis of the Social Vulnerability Index, Risk Anal., 28, 1099–1114, https://doi.org/10.1111/j.1539-6924.2008.01072.x, 2008.
Schneiderbauer, S. and Ehrlich, D.: Social levels and hazard (in)dependence in determining vulnerability, in: Measuring Vulnerability to Natural Hazards: Towards Disaster Resilient Societies, United Nations University Press, Tokyo, New York, Paris, 78–102, 2006.
Schuster-Wallace, C. J., Murray, S. J., and McBean, E. A.: Integrating Social Dimensions into Flood Cost Forecasting, Water Resour. Manag., 32, 3175–3187, https://doi.org/10.1007/s11269-018-1983-8, 2018.
Shah, A. A., Ye, J., Abid, M., Khan, J., and Amir, S. M.: Flood hazards: household vulnerability and resilience in disaster-prone districts of Khyber Pakhtunkhwa province, Pakistan, Nat. Hazards, 93, 147–165, https://doi.org/10.1007/s11069-018-3293-0, 2018.
Tarbotton, C., Osso, F. D., Dominey-howes, D., and Goff, J.: The use of empirical vulnerability functions to assess the response of buildings to tsunami impact: Comparative review and summary of best practice, Earth-Sci. Rev., 142, 120–134, https://doi.org/10.1016/j.earscirev.2015.01.002, 2015.
Tate, E.: Social vulnerability indices: a comparative assessment using uncertainty and sensitivity analysis, Nat. Hazards, 63, 325–347, https://doi.org/10.1007/s11069-012-0152-2, 2012.
Tate, E.: Uncertainty Analysis for a Social Vulnerability Index, Ann. Assoc. Am. Geogr., 103, 526–543, https://doi.org/10.1080/00045608.2012.700616, 2013.
Török, I.: Qualitative assessment of social vulnerability to flood hazards in Romania, Sustainability, 10, 3780, https://doi.org/10.3390/su10103780, 2018.
Turner, B. L., Kasperson, R. E., Matson, P. A., Mccarthy, J. J., and Corell, R. W.: A framework for vulnerability analysis in sustainability science, P. Natl. Acad. Sci. USA, 100, 8074–8079, https://doi.org/10.1073/pnas.1231335100, 2003.
UNDP: Disaster resilience measurements: Stocktaking of ongoing efforts in developing systems for measuring resilience, available at: https://www.preventionweb.net/files/37916_disasterresiliencemeasurementsundpt.pdf (last access: 10 May 2021), 2014.
UNDRR: Terminology on Disaster Risk Reduction, UNISDR, Geneva, 2017.
UNISDR: Sendai Framework for Disaster Risk Reduction 2015–2030, Sendai, 2015.
UNISDR: Report of the open-ended intergovernmental expert working group on indicators and terminology relating to disaster risk reduction, available at: https://www.preventionweb.net/files/50683_oiewgreportenglish.pdf (last access: 10 May 2021), 2016.
Wisner, B., Gaillard, J. C., and Kelman, I.: Framing disaster: Theories and stories seeking to understand hazards, vulnerability and risk, in: Handbook of Hazards and Disaster Risk Reduction, Routledge, Abingdon, 18–34, 2012.
Wu, Y., Zhong, P. an, Zhang, Y., Xu, B., Ma, B., and Yan, K.: Integrated flood risk assessment and zonation method: A case study in Huaihe River basin, China, Nat. Hazards, 78, 635–651, https://doi.org/10.1007/s11069-015-1737-3, 2015.
Yang, W., Xu, K., Lian, J., Ma, C., and Bin, L.: Integrated flood vulnerability assessment approach based on TOPSIS and Shannon entropy methods, Ecol. Indic., 89, 269–280, https://doi.org/10.1016/j.ecolind.2018.02.015, 2018a.
Yang, W., Xu, K., Lian, J., Bin, L., and Ma, C.: Multiple flood vulnerability assessment approach based on fuzzy comprehensive evaluation method and coordinated development degree model, J. Environ. Manage., 213, 440–450, https://doi.org/10.1016/j.jenvman.2018.02.085, 2018b.
Yoon, D. K.: Assessment of social vulnerability to natural disasters: A comparative study, Nat. Hazards, 63, 823–843, https://doi.org/10.1007/s11069-012-0189-2, 2012.
Zarekarizi, M., Srikrishnan, V., and Keller, K.: Neglecting uncertainties biases house-elevation decisions to manage riverine flood risks, Nat. Commun., 11, 1–11, https://doi.org/10.1038/s41467-020-19188-9, 2020.
Zhang, G. and Zhu, A.-X.: The representativeness and spatial bias of volunteered geographic information: a review, Annales of GIS, 24, 151–162, https://doi.org/10.1080/19475683.2018.1501607, 2018.
Zhang, Y. L. and You, W. J.: Social vulnerability to floods: A case study of Huaihe River Basin, Nat. Hazards, 71, 2113–2125, https://doi.org/10.1007/s11069-013-0996-0, 2014.
Zielstra, D. and Zipf, A.: A comparative study of proprietary geodata and volunteered geographic information for Germany, in: 13th AGILE International Conference on Geographic Information Science, vol. 2010, Guimarães, 1–15, available at: http://agile2010.dsi.uminho.pt/pen/shortpapers_pdf/142_doc.pdf (last access: 3 January 2021), 2010.
Here, rural areas are defined as sparsely populated areas, whereas urban areas are considered densely populated regions.
- Article
(1061 KB) - Full-text XML