the Creative Commons Attribution 4.0 License.
the Creative Commons Attribution 4.0 License.
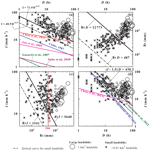
Evaluating critical rainfall conditions for large-scale landslides by detecting event times from seismic records
Hsien-Li Kuo
Chi-Wen Chen
Hitoshi Saito
Ching-Weei Lin
Hongey Chen
Wei-An Chao
One purpose of landslide research is to establish early warning thresholds for rainfall-induced landslides. Insufficient observations of past events have inhibited the analysis of critical rainfall conditions triggering landslides. This difficulty may be resolved by extracting the timing of landslide occurrences through analysis of seismic signals. In this study, seismic records of the Broadband Array in Taiwan for Seismology were examined to identify ground motion triggered by large landslides that occurred in the years 2005 to 2014. A total of 62 landslide-induced seismic signals were identified. The seismic signals were analyzed to determine the timing of landslide occurrences, and the rainfall conditions at those times – including rainfall intensity (I), duration (D), and effective rainfall (Rt) – were assessed. Three common rainfall threshold models (I–D, I–Rt, and Rt–D) were compared, and the crucial factors of a forecast warning model were found to be duration and effective rainfall. In addition, rainfall information related to the 62 landslides was analyzed to establish a critical height of water model, (. The critical height of water model was applied to data from Typhoon Soudelor of 2015, and the model issued a large landslide warning for southern Taiwan.
- Article
(12745 KB) -
Supplement
(1465 KB) - BibTeX
- EndNote
In recent years, the frequency of extreme rainfall events and the number of large-scale natural disasters have increased globally (Tu and Chou, 2013; Saito et al., 2014). These large-scale natural disasters (e.g., landslides, floods, etc.) cause huge economic losses and human casualties. In mountainous areas, large landslides can also change the landscape and erosion processes. Several previous studies have reported that the characteristics of a large landslide may include (1) extremely rapid mass movement, (2) a huge landslide volume, and (3) deep-seated excavations into rock formations (Chigira and Kiho, 1994; Lin et al., 2006). However, discriminating large landslides from small landslides still presents challenges. In practice, both the velocity of mass movement and depth of excavation are difficult to measure, so the landslide area is commonly regarded as an indicator of the scale of a landslide. Although the occurrence frequency of large landslides is lower than that of small landslides, large landslides cause rapid changes in the landscape. They also cause disasters on a far greater scale than small landslides do. In this study, a landslide that disturbed an area larger than 0.1 km2 was considered a large landslide, while one not meeting this criterion was considered a small landslide. It is well known that rainfall is a major factor in landslide occurrence, thus thorough understanding of the influences of different rainfall factors is necessary. To reduce losses, the critical rainfall conditions that trigger large landslides must be identified. These conditions can be used to determine a rainfall threshold for use as a forecast model for the prevention and mitigation of disasters.
In the past, it was difficult to estimate the threshold of precipitation convincingly due to the lack of accurate information on the timing of landslide occurrences. Recent studies in geophysics (Kanamori et al., 1984; Suriñach et al., 2005; Lin et al., 2010; Ekström and Stark, 2013; Chao et al., 2016, 2017) suggest that the mass movement of large landslides may generate ground motion. If such ground motion is recorded by seismic stations, the timing of large landslides can be extracted from the records. In general, if the exact time of a landslide is unknown, the time point with the maximum hourly rainfall will be conjectured as the time of the landslide (Chen et al., 2005; Wei et al., 2006; Staley et al., 2013; Yu et al., 2013; Xue and Huang, 2016). One case study examined the rainfall that triggered the Xiaolin landslide, a giant landslide in southern Taiwan that disturbed an area of ∼2.6 km2 and resulted in more than 400 deaths in August 2009. It was found that the difference between the conjectured and exact times was 13 h, which would result in an erroneous cumulated rainfall measurement of 513.5 mm (Fig. 1). However, seismic records can be analyzed to extract the time information for estimating critical rainfall.
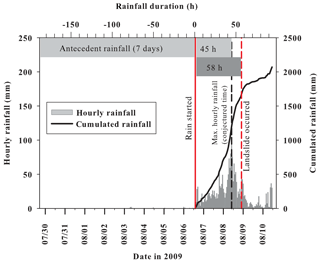
Figure 1Time series of hourly rainfall and cumulative rainfall from 29 July to 10 August 2009. Rainfall data were collected from the Central Weather Bureau (CWB) C0V250 rainfall gauge station, which is 12 km from the Xiaolin landslide. The Xiaolin landslide occurred at 22:16 UTC on 8 August 2009. The rainfall event induced by Typhoon Morakot in 2009 started at 14:00 UTC on 6 August when hourly rainfall exceeded 4 mm. The maximum hourly rainfall was at 10:00 UTC on 8 August. In general, if the exact time of landslide occurrence cannot be investigated, the time point with the maximum hourly rainfall will be conjectured as the time of the landslide (Chen et al., 2005).
In this study, landslide-generated seismic signals were analyzed to determine the times of landslides, and rainfall data corresponding to those times were used to construct rainfall thresholds. Then the thresholds suitable for triggering different warnings for small and large landslides were determined. Statistical analysis of various rainfall factors can be used to determine a statistical threshold for exploring the critical rainfall conditions of landslide occurrences, such as using rainfall intensity and duration, to define rainfall threshold curves (Caine, 1980; Guzzetti et al., 2008; Saito et al., 2010; Chen et al., 2015). Those rainfall thresholds provide valuable information for disaster prevention and mitigation. In this study, seismic data recorded by the network of the Broadband Array in Taiwan for Seismology (BATS; Fig. 2a) and landslide maps generated from satellite images were used to obtain the exact times and locations of large landslides. From these, the rainfall threshold for large landslides in Taiwan was developed.
Located at the junction of the Eurasian plate and the Philippine Sea plate, Taiwan has frequent tectonic activity (Ho, 1986; Yu et al., 1997; Willett et al., 2003). Fractured rock mass, a warm and humid climate, and an average of three to five typhoon events per year contribute to the high frequency of slope failures in mountainous areas in Taiwan (Wang and Ho, 2002; Shieh, 2000; Dadson et al., 2004; Chang and Chiang, 2009; Chen, 2011). The high coverage of the seismic network and rain gauge stations in Taiwan and the high occurrence frequency of landslides make the island a suitable area for examining the use of seismic observations to identify landslide times and thus the rainfall factors contributing to landslide events.
2.1 Large landslide mapping
To determine the locations and basic characteristics of large landslides occurring in the years 2005–2014, all landslide areas in Taiwan were identified using SPOT-4 satellite remote sensing images with a spatial resolution of 10 m in multispectral mode. Images with minimal cloud cover were selected from pre-, post-typhoon, and heavy rainfall events. All images were orthorectified to a standard base image and checked manually using fixed visible markers to ensure spatial consistency over time. Figure 2b and c show synthetic SPOT images that were used to identify landslides triggered by Typhoon Morakot in 2009. Bare areas are visibly distinguishable in the SPOT images.
The Normalized Difference Vegetation Index (NDVI) was used to conduct a preliminary classification of the bare areas (Lin et al., 2004). The exact NDVI thresholds for the bare areas differed from one image to another and were determined by tuning the cut-off value based on visible contrasts. After the image interpretation, classified areas were clustered based on slope using a digital elevation model with a resolution of 40 m to identify bare areas not associated with landslides (e.g., roads and buildings). The results of the interpretation were compared with a 1:5000 topographic map to exclude areas such as fallow farmland or alluvial fans. Landslides specifically induced by rainstorm events were distinguished by overlaying the pre- and post-event image mosaics. Based on the definition and description of deep-seated gravitational slope deformation (DSGSD) and large landslides (C. W. Lin et al., 2013; M. L. Lin et al., 2013), a large landslide should possess three characteristics: (1) a depth larger than 10 m, (2) a volume greater than 1 000 000 m3, and (3) a high velocity. In practice, it is difficult to confirm these three characteristics without in situ investigations and geodetic surveys. Therefore, a disturbed area of 100 000 m2 was employed to sort large landslides from small landslides. Landslides having an affected area of 0.1 km2 or larger were classified as large landslides, and all others, as small landslides. In this study, the types and mechanisms of individual landslides were not investigated, but landslide area was used as the main factor for investigating the rainfall conditions that trigger large and small landslides.
2.2 Interpretation of ground motions induced by large landslides
The movement of a landsliding mass has several different motion processes, such as sliding, falling, rotation, saltation, rolling, and impacting. These complex motion processes act on the ground surface and generate ground motion (Kanamori et al., 1984; Ekström and Stark, 2013). The seismic wave generated by a landslide can be attributed to the shear force and loading on the ground surface as the mass moves downslope. Many studies have shown that the source mechanism of a landslide is highly complicated, and that the seismic waves of landslides mainly consist of surface waves and shear waves. Consequently, it is difficult to distinguish P and S waves from station records (Lin et al., 2010; Suwa et al., 2010; Dammeier et al., 2011; Feng, 2011; Hibert et al., 2014). The onset of a landslide seismic signal is generally abrupt. The seismic amplitude gradually rises above the ambient noise level to the peak amplitude, exhibiting a cigar-shaped envelope. After the peak amplitude, most landslide-generated seismic signals have relatively long decay times, averaging about 70 % of the total signal duration (Norris, 1994; La Rocca et al., 2004; Suriñach et al., 2005; Deparis et al., 2008; Schneider et al., 2010; Dammeier et al., 2011; Allstadt, 2013). In the frequency domain, landslide-induced seismic energy is mainly distributed below 10 Hz, and the signature in a spectrogram is triangular due to an increase in high-frequency constituents over time (Suriñach et al., 2005; Dammeier et al., 2011). The triangular signature in the spectrogram is the distinctive property that distinguishes landslide-induced signals from those of earthquakes and other ambient noise.
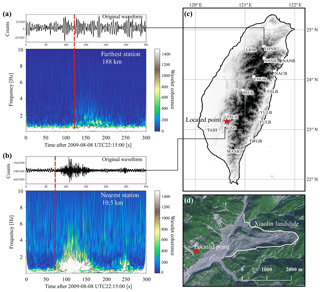
Figure 3Characteristic triangular signature visible in a spectrogram within a time window starting at 22:15 UTC and ending at 22:20 UTC on 8 August 2009. (a) Original waveform and spectrogram of the vertical component at station YHNB. (b) Original waveform and spectrogram of the vertical component at station SGSB. (c) Distribution of 15 detections of ground motion induced by the Xiaolin landslide and the location result. (d) The located point and the location of the Xiaolin landslide. The location error between the location result and the landslide site is about 1.5 km.
In this study, a total of 19 rainstorm events having cumulated rainfall exceeding 500 mm (17 typhoon-induced events and 2 heavy rainfall events) in the years 2005–2014 were selected, and the seismic records were examined (Table S1 in the Supplement). The seismic signals of local earthquakes, regional earthquakes, and teleseismic earthquakes were excluded based on the earthquake catalogues maintained by the United States Geological Survey and the Central Weather Bureau, Taiwan. After the removal of instrument response, mean, and linear trends, a multi-taper method (Percival and Walden, 1993; Burtin et al., 2009) was employed for spectral analysis of the continuous seismic records. A 5 min moving window with 50 % overlap of the seismic records provided a suitable spectrogram in the frequency range of 1–10 Hz. Landslide-related triangular signatures in the spectrograms were manually identified (Fig. 3a, b). To reduce the uncertainty caused by manual identification, only events with obvious triangular signatures in the spectrograms (e.g., Fig. S1 in the Supplement) were chosen.
A substantial key to this study was the detection of the time of landslide-induced ground motion. In seismology, many methods can be used to detect the seismic signals of earthquakes, and one widely used method is the STA/LTA ratio (short-term average to long-term average ratio; Allen, 1978). The duration of landslide-induced signals usually ranges from tens to hundreds of seconds (Helmstetter and Garambois, 2005; C. H. Chen et al., 2013). As compared with rainfall data, which are recorded once per hour, the duration of landslide-induced seismic signals is short. Thus, to avoid mis-judgements resulting from different signal-detection methods or manual interpretation, the time of the maximum amplitude of the envelope of the vertical-component signal recorded in the station closest to the landslide was employed as the time of the landslide. Considering the transmission speed of seismic waves, a time difference of several seconds to several tens of seconds was negligible with respect to the sampling rate of rainfall records.
To determine which landslides generated ground motion, it was necessary to locate the sources of the seismic signals. However, the arrival times of the P- and S-waves of landslide-induced ground motion could not be clearly distinguished. To locate the landslide-induced signals, a locating approach proposed by C. H. Chen et al. (2013) and Chao et al. (2016) was employed in this study. Locations were estimated with a cross-correlation method that could maximize tremor signal coherence among the seismic stations. The criteria for choosing the stations were their geographic locations and tremor signal-to-noise ratios. The interpreted signals were treated with an envelope function to process cross-correlations analyzed from different station pairs. Centroid location estimates were obtained by cross-correlating all station pairs and performing the Monte Carlo grid search method (Wech and Creager, 2008). Unlike traditional methods, which seek the source location that minimizes the horizontal time difference between predicted travel time and peak lag time, this method seeks to minimize the vertical correlation distance between the peak correlation value and the predicted correlation value.
Finally, the location results of landslide-induced seismic signals were compared with the exact locations of large landslides determined from the satellite images (Fig. 3c, d). If the locations matched, the times of the landslides could be obtained, and the time information could be applied to rainfall data analysis.
2.3 Analysis methods of statistically based rainfall threshold for landslides
Hourly rainfall data were collected from the records of rain gauge stations (Fig. 2a). The major rainfall events analyzed in the study were typhoon events. The distribution of precipitation during typhoon events is usually closely related to the typhoon track and the position of the windward slope, also as known as the orographic effect. In addition, the density and distribution of rainfall stations in mountainous areas directly affect the results of rainfall threshold analysis. If the landslide location and the selected rainfall station are located in different watersheds, the rainfall information is unlikely to represent the rainfall conditions for the landslide. In some cases, however, the diameter of the typhoon is so large that the orographic effects can be ignored (Chen and Chen, 2003; Sanchez-Moreno et al., 2014). Therefore, in this study, the selection criteria for a rainfall station were that the rainfall station was located within the same watershed as the landslide and at the shortest straight distance from the landslide, and that the watershed was smaller than 100 km2 in area, to ensure that the records at rain gauge stations were sufficient to represent the rainfall at the landslide locations. These criteria were established after testing the influences of distance and topographic effects on rainfall distribution (see Supplement Sect. S3). In rainfall analysis, the beginning of a rain event is defined as the time point when hourly rainfall exceeds 4 mm, and the rain event ends when the rainfall intensity has remained below 4 mm h−1 for six consecutive hours. The critical rainfall condition for a landslide was calculated from the beginning of a rain event to the time of the landslide (Jan and Lee, 2004; Lee, 2006). In this way, average rainfall intensity (mm h−1), cumulated rainfall (mm), and rainfall duration (h) for each large landslide could be used as the factors in the rainfall threshold analysis. In addition to the three factors mentioned above, the daily rainfall for the seven days preceding the rainstorm was considered as antecedent rainfall (Ra). The antecedent rainfall (Ra) was calculated with a temporal weighting coefficient of 0.7, with the weight decreasing with days before the event. The formula was Ra , where Ri is the daily rainfall of the ith day before the rainfall event. The sum of antecedent rainfall and principal event rainfall was regarded as the total effective rainfall (Rt). This definition of a rain event has been officially adopted in Taiwan (Jan and Lee, 2004). The use of different definitions of a rain event would result in differences in statistical rainfall conditions, but the statistical criteria used in this study ensured the consistency of data processing in the critical rainfall analysis.
Based on different rainfall factors, three common rainfall threshold analysis methods were used in the study. The first method was the I–D method, with the power law curve, , where a is the scaling parameter (the intercept) of the threshold curve and b is the slope (the scaling exponent) (Caine, 1980; Wieczorek, 1987; Keefer et al., 1987). In this study, the I–D rainfall threshold curve at 5 % exceedance probability was estimated by the method proposed by Brunetti et al. (2010). This threshold was expected to leave 5 % of the data points below the threshold line. The second method was the rainfall-based warning model proposed by Jan and Lee (2004), which is based on the Rt and I product values. With the I–Rt method, rainfall intensity and cumulated rainfall were plotted and used to calculate the cumulative probability of the product value of I and Rt by the Weibull distribution method (Jan and Lee, 2004). The cumulative probability of 5 % of Rt and I product values was taken as the I–Rt rainfall threshold. The third method was the Rt–D method (Aoki, 1980; Fan et al., 1999). In the Rt–D method, the 5 % cumulative probability of the product value of Rt and D by the Weibull distribution method was taken as the Rt–D rainfall threshold.
In addition to the time information of large landslides, the time information of 193 small landslides, such as shallow landslides and debris flows, from the years 2006–2014 was collected from the annual reports of debris flows investigated by the Soil and Water Conservation Bureau (SWCB) of Taiwan. For these landslides, no information was extracted from seismic records. Most of the 193 small landslides caused disasters and loss of life and property. In some cases, in situ steel cables or closed-circuit television recorded the time information. This information was applied to the rainfall data analysis and then used to compare the rainfall conditions of the large landslides.
2.4 Critical height of water model
Whether a given slope will produce a landslide depends on the balance between the shear strength of the slope material and the downslope component of the gravitational force imposed by the weight of the slope material above a potential slip surface. A critical volume of water model proposed by Keefer et al. (1987) was used in this study to construct a rainfall threshold. The model was derived from existing slope stability theory with some simplifying assumptions. The shear strength of the material at a point within a slope is expressed as
where c′ is effective cohesion of material, p is total stress perpendicular to the potential sliding surface, uw is pore-water pressure, and ϕ′ is effective friction angle of slope material. The main cause of a slope failure is the infiltration of rainfall into the slope and accumulation above the impermeable layer, which increases the pore-water pressure of the slope material. As the pore-water pressure (uw) increases, the shear strength (s) decreases, eventually leading to slope failure. A critical value of pore-water pressure uwc exists in each slope, assuming an infinite slope composed of a cohesionless sliding surface ( 0). The pore-water pressure threshold can be calculated as
where Z is the vertical depth of the sliding surface, γt is the unit weight of the slope material, and θ is the slope angle. Good development of a detachment plane (e.g., the sliding surface between sedimentary layers, connected joints, and weathered foliation) has been widely considered as the geological condition under which a large landslide occurs (Agliardi et al., 2001; Tsou et al., 2011). Therefore, in this study, the c′ of the detachment plane was simply assumed to be zero to represent the critical situation of slope stability.
As the pore-water pressure uw increases to the pore-water pressure threshold uwc, a critical height of water QC is retained above the sliding surface until the initiation of slope failure. QC is calculated as
where uwc is the critical value of pore-water pressure, γw is the unit weight of water, and nef is the effective porosity, which is the residual porosity of the slope material under free gravity drainage. The drainage rate of a saturated zone is represented by the average value I0, the unit of which is mm h−1. In a heavy rainfall event, the critical quantity of water for causing a slope failure is defined as
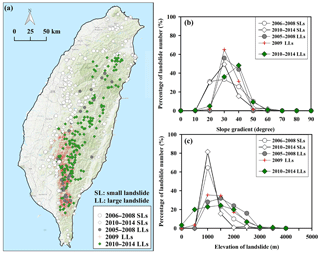
Figure 4(a) Distribution map of large landslides (LLs) from 2005 to 2014 and small landslides (SLs) from 2006 to 2014. (b) The numerical distribution of slope gradients of large and small landslides, presented in percentages. (c) The numerical distribution of elevations of large and small landslides, presented in percentages.
3.1 Topographic features of large landslides
The satellite imagery interpretation showed that, from 2005 to 2014, a total of 686 landslide events with areas greater than 0.1 km2 occurred in mountainous areas of Taiwan (Fig. 4a). Most of these large landslides had areas of 0.12 to 0.15 km2, and their slope angles before the landslides occurred were concentrated between 30 and 40∘ (Fig. 4b). The number of landslides occurring on slope angles exceeding 40∘ slightly increased after 2010. Although the increase was quite slight, it was most likely due to the fact that during the extremely heavy rainfall of Typhoon Morakot in 2009, more than 2000 mm precipitated in four days, causing a large number of landslides and exhausting many unstable slopes (Y. C. Chen et al., 2013). Consequently, landslides occurred on steeper slopes in the following years. The large landslides were primarily concentrated on slopes with elevations ranging from 500 to 2000 m (Fig. 4c), but the distributions of the highest and lowest elevations of these large landslides showed that their average vertical displacement was greater than 500 m.
The location information of the 193 small landslides investigated by the SWCB was used to obtain the topographic features of the small landslides as well. The distribution of the slope angles of these landslides was similar to that of the large landslides. However, the distribution of the elevations of the small landslides was quite different from that of the large landslides. Unlike those of the large landslides, a large portion of the elevations of small landslides was concentrated at about 1000 m. Although the difference in elevation distribution between large and small landslides seems to indicate that the topographic features of the large landslides were relatively more widespread than those of the small landslides, it should be attributed to the limited in situ investigations of the SWCB. Currently, the vast majority of landslides still cannot be investigated in the field.
3.2 The critical rainfall conditions for triggering large landslides
The location solutions of seismic signals and the landslide distribution map were compared, and it was found that the matched large landslides had deviations in distance of 0 to 20 km. In addition to distance, the resultant traces of two horizontal-component signals were plotted. The direction of the resultant trace of a given landslide-induced seismic record and the slope aspect in the vicinity of the located point were compared so as to eliminate any irrelevant landslides, those which had slope aspects different from the signal traces. The ground motion traces of the signals had to be correlated with the directions of movement of the landslides to reconfirm the matched large landslides. In total, 62 large landslides were paired successfully with seismic record locations (Fig. 2a, Table S2). These 62 large landslides were distributed in watersheds with high cumulated rainfall during heavy rainfall events. In addition, the 62 large landslides were verified by satellite images from multiple years to ensure that the shapes and positions were highly credible. Subsequently, the occurrence times of these 62 large landslides were obtained from seismic signals.
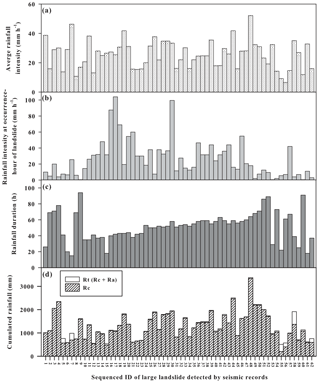
Figure 5Single-factor rainfall analysis. Each large landslide is assigned an ID number in the figure. The ID numbers of large landslides are displayed in chronological order. ID 1–4 are the large landslides occurring in 2005; ID 5 is a large landslide occurring in 2006; ID 6–9 are the large landslides occurring in 2008; ID 10–52 are the large landslides occurring in 2009; ID 53 is a large landslide occurring in 2010; ID 54–56 are the large landslides occurring in 2011; ID 57–60 are the large landslides occurring in 2012; ID 61–62 are the large landslides occurring in 2013. No large landslides occurring in 2007 or 2014 were successfully paired with seismic signal results. Most large landslides occurred when rainfall duration exceeded 24 h, cumulative rainfall exceeded 1000 mm, and rainfall intensity was less than 20 mm h−1.
The time information was used to implement rainfall analysis. About two-thirds (41) of the large landslides occurred when the total effective rainfall exceeded 1000 mm (Fig. 5). The statistical results of rainfall intensities at the times of large landslide occurrences showed that more than half of the large landslides occurred when the rainfall intensity was less than 20 mm h−1. Only seven of the large landslides occurred when the rainfall duration was less than 24 h, and the rainfall durations of these seven events all exceeded 10 h. The results of single rainfall-factor analysis indicated that the effects of rainfall duration and cumulated rainfall were much more remarkable for large landslides than for small landslides, and that the rainfall intensity at the time of landslide occurrence was not the main factor influencing large landslides. Therefore, the average rainfall intensity was adopted for the following multi-factorial analyses.
4.1 Dual rainfall-factor analysis of I–D, I–Rt, and Rt–D thresholds
The single rainfall-factor analysis indicated that there was no significant correlation between landslides and rainfall intensity at the times of large landslide occurrences. In the dual rainfall-factor analysis, the I–D rainfall threshold was assessed by using the average values of rainfall intensity and rainfall duration. The obtained I–D rainfall threshold was I= 71.9D−0.47 (D > 24 h; Fig. 6a). The rainfall information for small landslides reported by the SWCB from 2006 to 2014 was also analyzed, and the I–D rainfall threshold curve for large landslides also fit the lower boundary of the rainfall conditions of small landslides. In addition, the distribution of the rainfall durations indicated that the small landslides were distributed evenly from 3 to 70 h, while the large landslides were mostly distributed above 20 h. The rainfall intensity, however, could not be effectively used to distinguish these two kinds of slope failures. Even under the same rainfall duration, the rainfall intensities of many small landslides were higher than those of large landslides. These results sufficiently demonstrated that rainfall intensity could not be used to distinguish small landslides from large landslides. Therefore, the I–D rainfall threshold may not allow assessment of the landslide scale. It was also found that most of the large landslides affecting larger areas were concentrated in rainfall durations of more than 50 h, but average rainfall intensity was not well correlated with landslide area. The average rainfall intensity of the small landslides was very high for short durations, but the average duration of the small landslides was much lower than that of large landslides. Therefore, continuous high-intensity rainfall incurs a high likelihood of large landslide occurrence.
The I–D rainfall thresholds obtained in this study were also compared with those of previous studies that focused on shallow landslides or debris flows. This comparison revealed that the I–D threshold curve for large landslides was much higher than the threshold curves for shallow landslides or debris flows.
Based on the analysis of the relationship between total effective rainfall (Rt) and rainfall duration (D), the product of Rt and D for large landslides with a cumulative probability of 5 % was 12 773 mm h (Fig. 6b), and the rainfall threshold was also much higher than the 5 % cumulative probability of small landslides (487 mm h). The total effective rainfalls for large and small landslides differed considerably. Most small landslides had a total effective rainfall below 500 mm, and only a few occurred when total effective rainfall exceeded 1000 mm. The landslide size groups shifted from small landslides for relatively short duration and low effective rainfall to large landslides for long duration and very large effective rainfall. As a result of the disparity in the Rt–D threshold curves for large and small landslides, it was determined that Rt–D analysis could be effectively used to distinguish small landslides from large landslides.
The relationship between average rainfall intensity (I) and total effective rainfall (Rt) was analyzed, and the results indicated that the product values of both factors for 5 % cumulative probability were 5640 mm2 h−1 (Fig. 6c). The Rt–I threshold curve for large landslides was not much higher than that for small landslides (1541 mm2 h−1). The results of the three kinds of dual-factor rainfall threshold analyses were combined, and it was found that the critical rainfall conditions for small landslides included high average rainfall intensity but relatively low effective rainfall. In contrast, those for large landslides included long rainfall duration and high effective cumulated rainfall. These results corresponded well with the theoretical expectation (Van Asch et al., 1999; Iverson, 2000).
The main mechanism of shallow landslides is that heavy rainfall and rapid infiltration cause soil saturation and a temporary increase in pore-water pressure. However, prolonged rainfall allows for slow saturation, which in turn influences the groundwater level and soil moisture and can lead to large landslides. These facts have been recognized in many studies around the world (Wieczorek and Glade, 2005; Van Asch et al., 1999; Iverson, 2000), but they have been analyzed in only a few locations (e.g., a mountainous debris torrent, a shallow landslide event, and an individual rainfall event). In this study, the regional dataset of landslides and the time information were used to identify the critical rainfall conditions for large and small landslides in Taiwan.
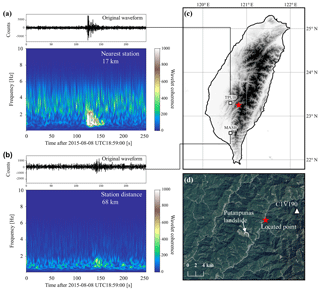
Figure 7Characteristic triangular signature visible in a spectrogram within a time window starting at 18:59 UTC and ending at 19:03 UTC on 8 August 2015. (a) Original waveform and spectrogram of the vertical component at station TPUB. (b) Original waveform and spectrogram of the vertical component at station MASB. (c) Distribution of located point (red star) and these two seismic stations. (d) The located point and the landslide site. The distance error between the location result and the landslide site is 3.7 km.
4.2 The critical height of water model for large landslides
The critical height of water, QC, on a sliding surface for each large landslide was estimated based on its slope gradient, its depth (estimated by the equation Z=26.14A0.4; Z: depth in m; A: disturbed area in m2), and the geological material parameters of the study area (Table 1). The QC value was inserted into to obtain an I0 value for each large landslide. For the 62 detected landslides, the cumulative probability of 5 % of the QC and I0 values was taken as the critical value. The critical value of I0 was 1.5, the critical QC was 430.2, which is more suitable for large than for small landslides, and the threshold curve was rewritten as (. The application of this threshold curve to average rainfall intensity and rainfall duration showed that almost all the large landslides could have been forecasted. This application demonstrated a good function as a large landslide forecasting model (Fig. 6d). In addition, the threshold curve can be used to distinguish large landslides and small landslides clearly. This advantage can prevent or reduce false forecasts. The critical height of water model combines statistical and deterministic approaches for the assessment of critical rainfall. Therefore, the parameters used to calculate QC can be adjusted based on regional geologic and topographic environments within a specific area. The QC model illustrates the importance of the cumulative volume of water and rainfall duration to large landslides and takes into account the effects of both infiltration of water and average rainfall intensity. The critical hydrological conditions for large landslides, namely a long duration and a high amount of cumulated rainfall, can be determined as well.
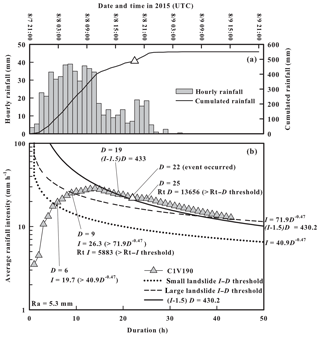
Figure 8(a) Hourly and cumulated rainfall record for rainfall station C1V190. The white triangle shows the time of the large landslide occurring in 2015. (b) The rainfall threshold of the critical height of water model issued the early warning 3 h before the landslide initiated (white triangle).
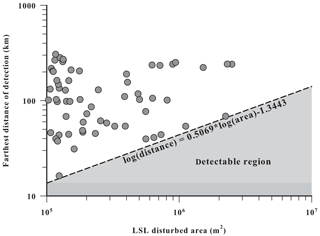
Figure 9Maximum distance of landslide signal detection as a function of landslide disturbed area. For a given large landslide, the seismic signal should be visible at all stations plotted beneath the curve.
In general, physically based models are easy to understand and have high predictive capabilities (Wilson and Wieczorek, 1995; Salciarini et al., 2013; Papa et al., 2013; Alvioli et al., 2014). However, they depend on the spatial distribution of various geotechnical data (e.g., cohesion, friction coefficient, and permeability coefficient), which are very difficult to obtain. Statistically based methods can include conditioning factors that influence slope stability, which are unsuitable for physically based models. Statistically based models rely on good landslide inventories and rainfall information. In this study, the QC threshold for a large landslide was estimated based on a mixture of physically and statistically based methods. Unlike other physically based I−D thresholds, which are commonly constructed based on artificial rainfall information for shallow landslides (Salciarini et al., 2012; Y. H. Chen et al., 2013; Napolitano et al., 2016; Table S3), the QC threshold proposed in this study seemed to be higher and more suitable for large landslides (Fig. 6d).
Although the geological and rainfall conditions in Taiwan and in other countries are not the same, seismic records can be used to obtain the timing of landslide occurrences for rainfall threshold analysis in other countries. For countries with geological and rainfall conditions similar to those of Taiwan (e.g., Japan and the Philippines; Saito and Oguchi, 2005; Yoshimatsu and Abe, 2006; Evans et al., 2007; Yumul et al., 2011), the results of this study may serve as a useful reference for the development of a forecast model for rainfall-triggered landslides.
5.1 Application of rainfall thresholds
To verify the usability of the rainfall thresholds proposed in this study, data from Typhoon Soudelor of 2015 were used to demonstrate the early warning performance. One of the most powerful storms on record, Typhoon Soudelor made landfall in Taiwan on 7 August 2015. It generated 1400 mm of rainfall in northeastern Taiwan and almost 1000 mm of rainfall in the southern mountainous area of Taiwan (Wei, 2017; Su et al., 2016). After seismic signal analysis, the time of a large landslide (named the Putanpunas landslide) in southern Taiwan, 8 August 2015 18:59:50 UTC, was obtained (Fig. 7). The seismic signals generated by the Putanpunas landslide were also detected by Chao et al. (2017). The seismic signals generated by this large landslide were identified from six BATS stations, and the distance error was less than 6 km. The rainfall records of rain gauge station C1V190, which was situated in the same watershed and 14.6 km away from the large landslide, were collected for rainfall analysis. At rain gauge station C1V190, it dropped a cumulated rainfall of 546 mm and had a maximum rainfall intensity of 39 mm h−1 on 8 August (Fig. 8). The rainfall event began at 22:00 UTC 7 August and lasted for 26 h, and the Putanpunas landslide initiated at the 22nd hour. This landslide occurred when the rainfall intensity was on the decline.
Once the rainfall conditions at a given rainfall station exceed the rainfall threshold for triggering landslides, the slopes located within the region of the rainfall station will have high potential for failure. When this threshold is reached, landslide warnings can be issued. Based on the statistically based I–D threshold for small landslides, a small-landslide warning would have been issued at the sixth hour of the rainfall event (Fig. 8), 16 hours before the Putanpunas landslide. This premature warning could have been declared a false alarm, and people might have returned to the affected area. Therefore, it is essential to establish different thresholds for landslides of different scales. The I–Rt threshold (i.e., Rt) would have led to a large-landslide warning at the ninth hour of the rainfall event (i.e., 13 hours before the Putanpunas landslide occurred), and the statistically based I–D threshold for large landslides would have yielded a landslide warning at the same time. These warnings would also have been premature. In contrast, a warning based on the Rt–D threshold (i.e., Rt) would have been issued 3 h after the time of the Putanpunas landslide. However, applying the rainfall records and the critical height of water model (i.e., () would have led to a landslide warning at 16:00 UTC on 8 August, 3 h before the time of the Putanpunas landslide. This warning would have allowed people sufficient time for evacuation and had low probability of being declared a false alarm. Compared to the statistically based I–D, the I–Rt, and the Rt–D thresholds, the critical height of water model had a better early-warning performance for the 2015 Putanpunas landslide.
5.2 Limitation of seismic detection of large landslides
The number of large landslides detected from seismic records, 62, comprised only 9 % of the total large landslides in 2005–2014 in Taiwan. This low percentage indicates that the vast majority of large landslides were not well identified from seismic records. If this limitation can be surmounted, more time information on large landslide occurrences can be used to develop rainfall thresholds. The average inter-station spacing of the Broadband Array in Taiwan for Seismology is around 30 km. A higher density of seismic stations would improve the detection function. In addition, to determine the limitation of large landslide detection distance as a function of large landslide-disturbed area, the most distant seismic station where large landslide signals were visible was selected. Some previous studies have applied similar approaches to probe the detection limit (Dammeier et al., 2011; C. H. Chen et al., 2013). The relationship between the maximum distance of detection and the large landslide-disturbed area shows a limitation of the detection distance due to the large landslide's magnitude (Fig. 9). In Fig. 9, each data point represents the distance between a landslide location and the most distant seismic station detecting it, as well as the landslide-disturbed area. In other words, when the distance between a seismic station and a landslide – which has the same given landslide-disturbed area as the data – is shorter than the value of the data, seismic signals induced by the landslide can be interpreted from the records of the seismic station. Therefore, a lower boundary of these data can be determined to demarcate an effective detectable region. As a large landslide's area increases, the maximum distance between the large landslide location and seismic detection increases. A detection limit can be described by
The boundary of detection was determined empirically based on the two lowest values of the farthest distance of detection (i.e., 31.0 and 37.6 km) of landslides having disturbed areas of 1.6×105 and 1.2×105 m2. If a station is located below the upper detection limit, the seismic signal should be detectable for a given large landslide. However, not all the stations located in detectable regions recorded clear large landslide-induced seismic signals. One possible reason for this lack of detection is that the environmental background noise affected the signal-to-noise ratio of the seismic records during heavy rainfall events. Therefore, the detection limit may also depend on the signal quality at each station.
In this study, seismic signals recorded by a broadband seismic network were used to determine the exact occurrence times of large landslides, and that time information was used to determine the rainfall threshold for large landslides. Based on the rainfall information of 62 large landslides that occurred from 2005 to 2014 in Taiwan, the rainfall conditions for triggering large landslides were found to be total effective rainfall of more than 1000 mm and rainfall duration of more than 24 h. After the rainfall thresholds were analyzed by the I–D, Rt–D, and I–Rt methods, the rainfall thresholds based on different factors for triggering large landslides were obtained. Furthermore, a critical height of water model combining statistical and deterministic approaches was developed to determine a 3-factor threshold for large landslides. The rainfall information and geologic/topographic parameters were applied to obtain the threshold curve, (, where average rainfall intensity I is in mm h−1 and rainfall duration D is in hours. This new critical height of water model can be used to improve the forecasting of large landslides and will not lead to confusion between small landslides and large landslides. The influences of extreme rainstorm events and rock types on the rainfall threshold were also investigated. However, the changes in the rainfall thresholds for large landslides either before or after an extreme event, or in different rock types, were not notable.
All data generated or analysed during this study are included in this published article (and its Supplement).
The Supplement contains five sections (S1–S5), including three supplement figures (Figs. S1–S3) and three supplement tables (Tables S1–S3). Nineteen selected rainfall events occurring in the years 2005–2014 are listed in Table S1. A sequence of spectrograms of seismic signals induced by the ID 1 landslide of 2005 is displayed in Fig. S1. The validation of the rainfall data used in the study is explained in Sect. S3, which includes Figs. S2 and S3. Detailed information on the 62 detected landslides is shown in Table S2. The equations of three physically based I–D thresholds reported in previous studies are listed in Table S3.
The supplement related to this article is available online at: https://doi.org/10.5194/nhess-18-2877-2018-supplement.
GWL devised the research, the main conceptual ideas, and proof outline. HLK and GWL performed the analytic calculations. GWL, HLK, CWC, HS, CWL, HC, and WAC contributed to the interpretation of the results. GWL took the lead in writing the manuscript. All authors provided critical feedback and helped shape the research, analysis, and manuscript.
The authors declare that they have no conflict of interest.
The authors gratefully acknowledged the financial support of the Ministry of
Science and Technology of Taiwan and the Soil and Water Conservation Bureau,
Council of Agriculture, Executive Yuan of Taiwan. The source of all seismic
and rainfall information included in this paper was the Institute of Earth
Sciences, Academia Sinica of Taiwan, and the Seismology Center, Central
Weather Bureau (CWB), Taiwan.
Edited by: Jean-Philippe Malet
Reviewed by: Odin Marc and one anonymous referee
Agliardi, F., Crosta, G., and Zanchi, A.: Structural constraints on deep-seated slope deformation kinematics, Eng. Geol., 59, 83–102, 2001.
Allen, R. V.: Automatic earthquake recognition and timing from single traces, B. Seismol. Soc. Am., 68, 1521–1532, 1978.
Allstadt, K.: Extracting source characteristics and dynamics of the August 2010 Mount Meager landslide from broadband seismograms, J. Geophys. Res.-Earth, 118, 1472–1490, https://doi.org/10.1002/jgrf.20110, 2013.
Alvioli, M., Guzzetti, F., and Rossi, M.: Scaling properties of rainfall induced landslides predicted by a physically based model, Geomorphology, 213, 38–47, https://doi.org/10.1016/j.geomorph.2013.12.039, 2014.
Aoki, S.: Critical rainfall triggering debris-flow disaster, National Research Institute for Earth Science and Disaster Prevention, 38, 22–26, 1980 (in Japanese).
Brunetti, M. T., Peruccacci, S., Rossi, M., Luciani, S., Valigi, D., and Guzzetti, F.: Rainfall thresholds for the possible occurrence of landslides in Italy, Nat. Hazards Earth Syst. Sci., 10, 447–458, https://doi.org/10.5194/nhess-10-447-2010, 2010.
Burtin, A., Bollinger, L., Cattin, R., Vergne, J., and Nabelek, J. L.: Spatiotemporal sequence of Himalayan debris flow from analysis of high-frequency seismic noise, J. Geophys. Res., 114, https://doi.org/10.1029/2008JF001198, 2009.
Caine, N.: The rainfall intensity: duration control of shallow landslides and debris flows, Geogr. Ann. A, 62, 23–27, 1980.
Chang, K. and Chiang, S.: An integrated model for predicting rainfall-induced landslides, Geomorphology, 105, 366–373, 2009.
Chao, W. A., Zhao, L., Chen, S. C., Wu, Y. M., Chen, C. H., and Huang, H. H.: Seismology-based early identification of dam-formation landquake events, Sci. Rep.-UK, 6, 19259, https://doi.org/10.1038/srep19259, 2016.
Chao, W. A., Wu, Y. M., Zhao, L., Chen, H., Chen, Y. G., Chang, J. M., and Lin, C. M.: A first near real-time seismology-based landquake monitoring system, Sci. Rep.-UK, 7, 43510, https://doi.org/10.1038/srep43510, 2017.
Chen, C. H., Chao, W. A., Wu, Y. M., Zhao, L., Chen, Y. G., Ho, W. Y., Lin, T. L., Kuo, K. H., and Chang, J. M.: A seismological study of landquakes using a real-time broad-band seismic network, Geophys. J. Int., 194, 885–898, 2013.
Chen, C. S. and Chen, Y. L.: The rainfall characteristics of Taiwan, Mon. Weather Rev., 131, 1323–1341, 2003.
Chen, C. W., Saito, H., and Oguchi, T.: Rainfall intensity–duration conditions for mass movements in Taiwan, Progress in Earth and Planetary Science, 2, 1–13, 2015.
Chen, C. Y., Chen, T. C., Yu, F. C., Yu, W. H., and Tseng, C. C.: Rainfall duration and debris-flow initiated studies for real-time monitoring, Environ. Geol., 47,5, 715–724, https://doi.org/10.1007/s00254-004-1203-0, 2005.
Chen, J. C.: Variability of impact of earthquake on debris-flow triggering conditions: case study of Chen-Yu-Lan watershed, Taiwan, Environ. Earth Sci., 64, 1787–1794, 2011.
Chen, Y. C., Chang, K. T., Chiu, Y. J., Lau, S. M., and Lee, H. Y.: Quantifying rainfall controls on catchment-scale landslide erosion in Taiwan, Earth Surf. Proc. Land., 38, 372–382, 2013.
Chen, Y. H., Tan, C. H., Chen, M. M., and Su, T. W.: Estimation of rainfall threshold for regional shallow landslides in a watershed, Journal of Chinese Soil and Water Conservation, 44, 87–96, 2013.
Chigira, M. and Kiho, K.: Deep-seated rockslide-avalanches preceded by mass rock creep of sedimentary rocks in the Akaishi Mountains, central Japan, Eng. Geol., 38, 221–230, 1994.
Dadson, S. J., Hovius, N., Chen, H., Dade, W. B., Lin, J. C., Hsu, M. L., Lin, C. W., Horng, M. J., Chen, T. C., Milliman, J., and Stark, C. P.: Earthquake triggered increase in sediment delivery from an active mountain belt, Geology, 32, 733–736, 2004.
Dammeier, F., Moore, J. R., Haslinger, F., and Loew, S.: Characterization of alpine rockslides using statistical analysis of seismic signals, J. Geophys. Res., 116, F04024, https://doi.org/10.1029/2011JF002037, 2011.
Deparis, J., Jongmans, D., Cotton, F., Baillet, L., Thouvenot, F., and Hantz, D.: Analysis of rock-fall and rock-fall avalanche seismograms in the French Alps, B. Seismol. Soc. Am., 98, 1781–1796, https://doi.org/10.1785/0120070082, 2008.
Ekström, G. and Stark, C. P.: Simple scaling of catastrophic landslide dynamics, Science, 339, 1416–1419, 2013.
Evans, S. G., Guthrie, R. H., Roberts, N. J., and Bishop, N. F.: The disastrous 17 February 2006 rockslide-debris avalanche on Leyte Island, Philippines: a catastrophic landslide in tropical mountain terrain, Nat. Hazards Earth Syst. Sci., 7, 89–101, https://doi.org/10.5194/nhess-7-89-2007, 2007.
Fan, J. C., Wu, M. F., and Peng, G. T.: The Critical Rainfall Line of Debris Flow Occurrence at Feng-Chiou, Sino-Geotechnics, 74, 39–46, 1999.
Feng, Z.: The seismic signatures of the 2009 Shiaolin landslide in Taiwan, Nat. Hazards Earth Syst. Sci., 11, 1559–1569, https://doi.org/10.5194/nhess-11-1559-2011, 2011.
Guzzetti, F., Peruccacci, S., Rossi, M., and Stark, C. P.: Rainfall thresholds for the initiation of landslides in central and southern Europe, Meteorol. Atmos. Phys., 98, 239–267, 2007.
Guzzetti, F., Peruccacci, S., Rossi, M., and Stark, C. P.: The rainfall intensity–duration control of shallow landslides and debris flows: an update, Landslides, 5, 3–17, 2008.
Handin, J. and Hager Jr., R. V.: Experimental deformation of sedimentary rocks under confining pressure: Tests at room temperature on dry samples, AAPG Bull., 41, 1–50, 1957.
Handin, J., Hager Jr., R. V., Friedman, M., and Feather, J. N.: Experimental deformation of sedimentary rocks under confining pressure: pore pressure tests, AAPG Bull., 47, 717–755, 1963.
Helmstetter, A. and Garambois, S.: Seismic monitoring of Séchilienne rockslide (French Alps): Analysis of seismic signals and their correlation with rainfalls, J. Geophys. Res.-Earth, 115, https://doi.org/10.1029/2009JF001532, 2010.
Hibert, C., Ekström, G., and Stark, C. P. : Dynamics of the Bingham Canyon Mine landslides from seismic signal analysis, Geophys. Res. Lett., 41, 4535–4541, https://doi.org/10.1002/2014gl060592, 2014.
Ho, C. S.: A synthesis of the geologic evolution of Taiwan, Tectonophysics, 125, 1–16, 1986.
Iverson, R. M.: Landslide triggering by rain infiltration, Water Resour. Res., 36, 1897–1910, 2000.
Jan, C. D. and Chen, C. L.: Debris flows caused by Typhoon Herb in Taiwan, in: Debris-Flow Hazards and Related Phenomena, Springer Berlin Heidelberg, 539–563, 2005.
Jan, C. D. and Lee, M. H.: A Debris-Flow Rainfall-Based Warning Model, Journal of Chinese Soil and Water Conservation, 35, 275–285, 2004.
Kanamori, H., Given, J. W., and Lay, T.: Analysis of seismic body waves excited by the Mount St. Helens eruption of May 18, 1980, J. Geophys. Res.-Sol. Ea., 89, 1856–1866, 1984.
Keefer, D. K., Wilson, R. C., Mark, R. K., Brabb, E. E., Brown, W. M., Ellen, S. D., and Zatkin, R. S.: Real-time landslide warning during heavy rainfall, Science, 238, 921–925, 1987.
La Rocca, M., Galluzzo, D., Saccorotti, G., Tinti, S., Cimini, G. B., and Del Pezzo, E.: Seismic signals associated with landslides and with a tsunami at Stromboli volcano, Italy, B. Seismol. Soc. Am., 94, 1850–1867, https://doi.org/10.1785/012003238, 2004.
Lee, M. H.: The Rainfall threshold and analysis of Debris flows, Doctoral dissertation, National Cheng Kung University, Taiwan, ROC, 2006 (in Chinese).
Lin, C., Kumagai, H., Ando, M., and Shin, T.: Detection of landslides and submarine slumps using broadband seismic networks, Geophys. Res. Lett., 37, L22309, https://doi.org/10.1029/2010GL044685, 2010.
Lin, C. W., Shieh, C. L., Yuan, B. D., Shieh, Y. C., Liu, S. H., and Lee, S. Y.: Impact of Chi-Chi earthquake on the occurrence of landslides and debris flows: Example from the Chenyulan River watershed, Nantou, Taiwan, Eng. Geol., 71, 49–61, 2004.
Lin, C. W., Liu, S. H., Lee, S. Y., and Liu, C. C.: Impacts of the Chi-Chi earthquake on subsequent rainfall-induced landslides in central Taiwan, Eng. Geol., 86, 87–101, 2006.
Lin, C. W., Tseng, C. M., Tseng, Y. H., Fei, L. Y., Hsieh, Y. C., and Tarolli, P.: Recognition of large scale deep-seated landslides in forest areas of Taiwan using high resolution topography, J. Asian Earth Sci., 62, 389–400, 2013.
Lin, M. L., Chen, T. W., Lin, C. W., Ho, D. J., Cheng, K. P., Yin, H. Y., and Chen, M. C.: Detecting large-scale landslides using LiDar data and aerial photos in the Namasha-Liuoguey area, Taiwan, Remote Sens., 6, 42–63, 2013.
Napolitano, E., Fusco, F., Baum, R. L., Godt, J. W., and De Vita, P.: Effect of antecedent-hydrological conditions on rainfall triggering of debris flows in ash-fall pyroclastic mantled slopes of Campania (southern Italy), Landslides, 13, 967–983, 2016.
Norris, R. D.: Seismicity of rockfalls and avalanches at 3 Cascade Range volcanos – Implications for seismic detection of hazardous mass movements, B. Seismol. Soc. Am., 84, 1925–1939, 1994.
Papa, M. N., Medina, V., Ciervo, F., and Bateman, A.: Derivation of critical rainfall thresholds for shallow landslides as a tool for debris flow early warning systems, Hydrol. Earth Syst. Sci., 17, 4095–4107, https://doi.org/10.5194/hess-17-4095-2013, 2013.
Percival, D. B. and Walden, A. T.: Spectral analysis for physical applications: Multitaper and conventional univariate techniques, Cambridge Univ. Press, Cambridge, UK, 1993.
Saito, H., Nakayama, D., and Matsuyama, H.: Relationship between the initiation of a shallow landslide and rainfall intensity–duration thresholds in Japan, Geomorphology, 118, 167–175, 2010.
Saito, H., Korup, O., Uchida, T., Hayashi, S., and Oguchi, T.: Rainfall conditions, typhoon frequency, and contemporary landslide erosion in Japan, Geology, 42, 999–1002, 2014.
Saito, K. and Oguchi, T.: Slope of alluvial fans in humid regions of Japan, Taiwan and the Philippines, Geomorphology, 70, 147–162, 2005.
Salciarini, D., Tamagnini, C., Conversini, P., and Rapinesi, S.: Spatially distributed rainfall thresholds for the initiation of shallow landslides, Nat. Hazards, 61, 229–245, 2012.
Salciarini, D., Tamagnini, C., Ponziani, F., and Berni, N.: Defining physically-based rainfall thresholds for early warning systems, in: Landslide Science and Practice, Springer, Berlin, Heidelberg, 651–657, https://doi.org/10.1007/978-3-642-31445-2_85, 2013.
Sanchez-Moreno, J. F., Mannaerts, C. M., and Jetten, V.: Influence of topography on rainfall variability in Santiago Island, Cape Verde, Int. J. Climatol., 34, 1081–1097, 2014.
Schneider, D., Bartelt, P., Caplan-Auerbach, J., Christen, M., Huggel, C., and McArdell, B. W.: Insights into rock-ice avalanche dynamics by combined analysis of seismic recordings and a numerical avalanche model, J. Geophys. Res., 115, F04026, https://doi.org/10.1029/2010jf001734, 2010.
Shieh, S. L.: User's Guide for Typhoon Forecasting in the Taiwan Area (VIII), Central Weather Bureau, Taipei Aman, 2000.
Staley, D., Kean, J. W., Cannon, S. H., Schmidt, K. M., and Laber, J. L.: Objective definition of rainfall intensity–duration thresholds for the initiation of post-fire debris flows in southern California, Landslides, 10, 547–562, 2013.
Suriñach, E., Vilajosana, I., Khazaradze, G., Biescas, B., Furdada, G., and Vilaplana, J. M.: Seismic detection and characterization of landslides and other mass movements, Nat. Hazards Earth Syst. Sci., 5, 791–798, https://doi.org/10.5194/nhess-5-791-2005, 2005.
Su, Y. F., Chen, W. B., Fu, H. S., Jang, J. H., and Chang, C. H.: Application of Rainfall Forecasting to Flood Management – A Case Study of Typhoon Soudelor, Journal of Disaster Management, 5, 1–17, 2016 (in Chinese).
Suwa, H., Mizuno, T., and Ishii, T.: Prediction of a landslide and analysis of slide motion with reference to the 2004 Ohto slide in Nara, Japan, Geomorphology, 124, 157–163, 2010.
Tsou, C. Y., Feng, Z. Y., and Chigira, M.: Catastrophic landslide induced by typhoon Morakot, Shiaolin, Taiwan, Geomorphology, 127, 166–178, 2011.
Tu, J. Y. and Chou, C.: Changes in precipitation frequency and intensity in the vicinity of Taiwan: typhoon versus non-typhoon events, Environ. Res. Lett., 8, 1–7, 2013.
Van Asch, T. W., Buma, J., and Van Beek, L. P. H.: A view on some hydrological triggering systems in landslides, Geomorphology, 30, 25–32, https://doi.org/10.1016/S0169-555X(99)00042-2, 1999.
Wang, B. and Ho, L.: Rainy season of the Asian-Pacific summer monsoon, J. Climate, 15, 386–398, 2002.
Wech, A. G. and Creager, K. C.: Automated detection and location of Cascadia tremor, Geophys. Res. Lett., 35, https://doi.org/10.1029/2008GL035458, 2008.
West, T. R.: Geology applied to engineering, Prentice Hall, Inc., Simon/Schuster Company, Englewood Cliffs, New Jersey, 07632, 541, 1995.
Wei, C. C.: Examining El Niño–Southern Oscillation effects in the subtropical zone to forecast long-distance total rainfall from typhoons: A case study in Taiwan, J. Atmos. Ocean. Technol., 34, 2141–2161, 2017.
Wei, F., Gao, K., Cui, P., Hu, K., Xu, J., Zhang, G., and Bi, B.: Method of debris flow prediction based on a numerical weather forecast and its application, WIT Transactions on Ecology and the Environment, 90, 37–46, 2006.
Wieczorek, G. and Glade, T.: Climatic Factors Influencing Occurrence of Debris Flows, in: Debris-Flow Hazards and Related Phenomena, edited by: Jakob, M. and Hungr, O., Springer, Berlin, 325–362, 2005.
Wieczorek, G. F.: Effect of rainfall intensity and duration on debris flows in central Santa Cruz Mountains, California, Rev. Eng. Geol., 7, 93–104, 1987.
Wilson, R. C. and Wieczorek, G. F.: Rainfall thresholds for the initiation of debris flows at La Honda, California, Environ. Eng. Geosci., 1, 11–27, 1995.
Willett, S. D., Fisher, D., Fuller, C., Yeh, E. C., and Lu, C. Y.: Erosion rates and orogenic wedge kinematics in Taiwan inferred from apatite fission track thermochronometry, Geology, 31, 945–948, 2003.
Xue, X. and Huang, J.: A preliminary study on the comprehensive threshold for debris-flow early warning, Nat. Hazards Earth Syst. Sci. Discuss., https://doi.org/10.5194/nhess-2016-149, 2016.
Yoshimatsu, H. and Abe, S.: A review of landslide hazards in Japan and assessment of their susceptibility using an analytical hierarchic process (AHP) method, Landslides, 3, 149–158, 2006.
Yu, B., Li, L., Wu, Y., and Chu, S.: A formation model for debris flows in the Chenyulan River Watershed, Taiwan, Nat. Hazards, 68, 745–762, 2013.
Yu, S. B., Chen, H. Y., and Kuo, L. C.: Velocity field of GPS stations in the Taiwan area, Tectonophysics, 274, 41–59, 1997.
Yumul, G. P., Cruz, N. A., Servando, N. T., and Dimalanta, C. B.: Extreme weather events and related disasters in the Philippines, 2004–08: a sign of what climate change will mean, Disasters, 35, 362–382, 2011.