the Creative Commons Attribution 4.0 License.
the Creative Commons Attribution 4.0 License.
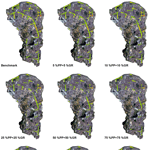
Effectiveness of low-impact development for urban inundation risk mitigation under different scenarios: a case study in Shenzhen, China
Jiansheng Wu
Rui Yang
Jing Song
The increase in impervious surfaces associated with rapid urbanization is one of the main causes of urban inundation. Low-impact development (LID) practices have been studied for mitigation of urban inundation. This study used a hydrodynamic inundation model, coupling SWMM (Storm Water Management Model) and IFMS-Urban (Integrated Flood Modelling System–Urban), to assess the effectiveness of LID under different scenarios and at different hazard levels. The results showed that LID practices can effectively reduce urban inundation. The maximum inundation depth was reduced by 3 %–29 %, average inundation areas were reduced by 7 %–55 %, and average inundation time was reduced by 0 %–43 % under the eight scenarios. The effectiveness of LID practices differed for the three hazard levels, with better mitigation of urban inundation at a low hazard level than at a high hazard level. Permeable pavement (PP) mitigated urban inundation better than green roofs (GRs) under the different scenarios and at different hazard levels. We found that more implementation area with LID was not necessarily more efficient, and the scenario of 10 % PP+10 % GR was more efficient for the study area than other scenarios. The results of this study can be used by local governments to provide suggestions for urban inundation control, disaster reduction, and urban renewal.
- Article
(3224 KB) - Full-text XML
- BibTeX
- EndNote
In recent years, urban storm water inundation hazards have occurred frequently in major cities all over the world, leading to significant property damage in local areas (Bhattarai et al., 2016). In China, according to a report by the Ministry of Housing and Urban-Rural Development (MOHURD) in 2010, 62 % of 351 cities suffered from inundation hazards, and 137 of these had negative effects from urban floods on more than three occasions from 2008 to 2010. In 2012, 2013, 2014, and 2015, the number of cities that suffered urban inundation was 184, 234, 125, and 154, respectively, including Beijing, Shanghai, Guangzhou, and Shenzhen. Urban inundation increasingly threatens the sustainable development of urban areas.
Rapid urbanization has become an important cause of frequent urban storm water inundation, in addition to extreme precipitation and low standards for urban drainage infrastructure (Arnold, 1996; Beckers et al., 2013; Claessens et al., 2006; Zahmatkesh et al., 2015b). Rapid expansion of cities generally leads to an increase in impervious surfaces, which makes the hydrological characteristics of the urban surface change significantly (Arnold, 1996; Jacobson, 2011; Rose and Peters, 2001). Impervious surfaces replace rivers, lakes, green spaces, and urban forests; weaken the flood control capability of the urban system; and change infiltration, evaporation, filtration, and storage (Hao et al., 2015; Jacobson, 2011; Meyer, 2001). The expansion of impervious areas accelerates rainwater convergence on urban surfaces, resulting in increased runoff and peak flows (Hatt et al., 2004; Leopold et al., 1995; Liu et al., 2015). The increase in runoff and peak flows taxes urban drainage facilities and exacerbates the risk of urban inundation.
To solve the problem of urban inundation, scholars in China have suggested the “sponge city” initiative, which allows cities to act as sponges to filtrate, purify, evaporate, and store rainwater (Mao et al., 2017; Sang and Yang, 2016). Low-impact development (LID), an important development concept for sponge cities, has been applied in sponge city construction (Luan et al., 2017); it is widely applied to reduce the impacts of urban inundation associated with rapid urbanization (Dietz and Clausen, 2008; Dietz, 2007; Xia et al., 2017; Zahmatkesh et al., 2015a). LID is a storm water management strategy that uses microscale and localized practices to control the runoff and pollution caused by a storm (Damodaram et al., 2010; EPA, 2000; HUD, 2003). Since the 1990s, LID practices have been widely used in countries in Europe, the United States of America, and other developed countries. LID practices include permeable pavement (PP), green roofs (GRs), bio-retention (BR), swales, infiltration wells/trenches, infiltrating wetlands, and rain barrels (Hunt et al., 2010).
The hydrological effectiveness of LID practices has been researched through field and laboratory studies (Abbot and Comino-Mateos, 2003; Berndtsson, 2010; Davis, 2008; Davis et al., 2012; Fassman and Blackbourn, 2010). For example, Hood et al. (2007) monitored low-impact residential development and traditional residential development in Waterford, Connecticut, USA, and found that LID practices helped lower runoff, peak flows, and discharge volumes. Dreelin et al. (2006) designed a test to compare the performance of asphalt and PP parking lots in Athens, Georgia, USA, and their results showed that the porous parking lot contributed 93 % less runoff than the asphalt lot during natural storm events. Bliss et al. (2009) constructed and monitored a GR in Pittsburgh, Pennsylvania, USA, and reported that the GR reduced runoff by up to 70 % and reduced peak flows by 5 %–70 %; the hydrograph was delayed by several hours more than a normal roof for the same building.
Many scholars have focused on simulations at a large scale, such as watersheds (Ahiablame et al., 2012; Dietz and Clausen, 2008; Roy et al., 2008; Salvadore et al., 2015), to explore the effectiveness of LID practices. For example, Palla and Gnecco (2015) reported that the LID combination of GR and PP decreased runoff and peak flows by 23 % and 45 %, respectively, and delayed the hydrograph by up to 19 % at the urban catchment scale. Trinh and Chui (2013) conducted a simulation and found that GR could reduce the peak flows by 50 % and delay the hydrograph by 2 h, BR systems could reduce the peak flows by 50 %, and the combined GR and BR systems could reduce the peak flows to a pre-urbanized level. Morsy et al. (2016) reported that rain gardens can mitigate runoff by approximately 15 %, 27 %, and 38 % for 2-, 5-, and 10-year storm events, respectively, which reduced the watersheds' flood risk. Ahiablame et al. (2013) assessed the effectiveness of rain barrels/cisterns and PP in two urbanized watersheds near Indianapolis, Indiana, USA; by using simulations, they found that LID practices reduced runoff and pollutant loads; they listed some LID combinations that are good retrofitting options for local areas.
Peak flow reduction, runoff reduction, and hydrograph delays are widely used indexes for evaluating the performance of LID practices (Ahiablame and Shakya, 2016; Qin et al., 2013; Zhang et al., 2016). However, these indexes are not intuitive, and the performance of LID practices for urban inundation is more useful for local residents, such as providing a guide for their travel behaviour. Some 1-D–2-D models have been applied for flood management, such as ESTRY-TUFLOW (Fewtrell et al., 2011), InfoWorks ICM (Russo et al., 2015), and MIKE FLOOD (Lowe et al., 2017). However, most of these models have a cost, which limits their application. In recent years, as an open-source and free model, SWMM (Storm Water Management Model) has been coupled with other models, such as BreZo (Burns et al., 2015) and LISFLOOD-FP (Wu et al., 2017), to simulate urban inundation, which means that the coupled models based on SWMM are needed in future research.
The goal of this study was to evaluate the effectiveness of LID practices to mitigate urban inundation in the urban watershed using a case study. The specific objectives were to establish a 1-D–2-D hydrodynamic model that coupled SWMM and IFMS-Urban (Integrated Flood Modelling System–Urban), evaluate the effectiveness of LID practices under different scenarios and hazard levels, and explore the efficiency of the LID scenarios. We intended this study to enrich LID inundation mitigation research at the urban watershed scale and to provide a reference for urban storm water management and inundation mitigation for local governments.
2.1 Study site
Shenzhen is in the coastal area of Guangdong Province in southern China (Fig. 1). It has a subtropical maritime monsoon climate; Shenzhen is hot and rainy in summer and mild in winter, and the average annual rainfall is 1837 mm. April to September is the rainy season in Shenzhen; during this period, precipitation is concentrated and storm water overflows are frequent. There were 38 rainstorm days (95 % of the year) in 2017, and the average rainfall was 170–350 mm every month during this period. Accordingly, urban inundation was particularly serious in this period; it caused loss of life and economic losses for local residents.
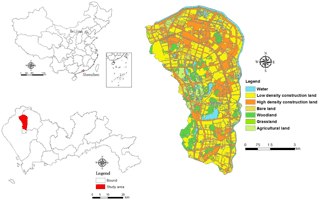
Figure 1Location and land use map of the study area in the Guangming New District of Shenzhen, China.
The study site was located in Guangming New District of Shenzhen, China, and it is in the Maozhou River basin (Fig. 1). The total area of our study site was 37.68 km2, of which 69.8 % was impervious surfaces. Guangming New District was selected as the first pilot area for LID practices in Shenzhen in October 2011 because of the intensity of its inundation disasters. There is a need to research the effectiveness of LID on urban inundation mitigation in this area.
2.2 Data
The model input data included inundation, land use, a digital elevation model (DEM), weather, and pipe network data. The land use data (2013) and pipe network data were provided by the Shenzhen government. We generalized the original data and divided the study area into water, low-density construction land, high-density construction land, bare land, woodland, grassland, and agricultural land using remote-sensing images (Fig. 1). The DEM of the study area (Fig. 2) was downloaded from the Geospatial Data Cloud (30 m resolution). The weather data were sourced from the Shenzhen Meteorological Data System (https://data.szmb.gov.cn/, last access: 5 May 2017). According to the integrity and availability of data, we chose two representative heavy-rainstorm-event datasets, 11 May 2014 and 10 May 2016 (Fig. 3), for model simulation, which included the complete volume of rainfall every hour. The corresponding inundation data were obtained from the Shenzhen SanFang (flood, drought, and wind defence) headquarters and the Guangming New District Urban Construction Bureau. We simplified the drainage data to build the model because the urban pipe network is intricate and substantial: add nodes when the pipeline is too long; keep or add the nodes that change the diameter and slope of the pipeline; keep the parallel pipelines and nodes on both sides of the roads; and delete the nodes and pipelines that are independent of this model. Finally, the 4502 pipelines and 1175 nodes in this study were generalized to 597 pipelines and 653 nodes, including 56 outlets and 597 inspection nodes (Fig. 2).
2.3 SWMM and IFMS-Urban models
Developed by the United States Environmental Protection Agency (US EPA), SWMM is an open-source model that can simulate dynamic runoff quantity and quality from urban areas, and it has been widely used to simulate the hydrologic performance of LID practices (Rossman, 2010; Wu et al., 2013). However, SWMM cannot simulate the spatial and temporal distributions of surface inundation. Recently, some scholars have conducted simulations using secondary developments of this software (Seyoum et al., 2012; Son et al., 2016; Zhu et al., 2016). We expected that this application would be difficult to use in our study area due to differences in computer programming. Coupling a model with SWMM for 2-D simulation is another way to simulate the spatial distribution of urban inundation (Huong and Pathirana, 2013; Wu et al., 2017).
IFMS-Urban was developed by the China Institute of Water Resources and Hydropower Research (IWHR) in cooperation with other institutions. Based on the simulated results from SWMM, IFMS-Urban can simulate the temporal and spatial distribution of urban inundation, and it is compatible with ArcGIS and SWMM. Data conversion and model coupling are accomplished in IFMS-Urban, and it does not require additional software programming, which is convenient for researchers and non-expert users.
Therefore, we coupled SWMM and IFMS-Urban in this study to simulate urban inundation. SWMM was applied to construct a 1-D sewer model. The study area was simplified to 577 sub-catchments, 597 pipelines, and 653 nodes. Details of model building and of SWMM's parameters can be found in many published studies (e.g. Rossman, 2010; Qin et al., 2013; Wu et al., 2017). Model coupling occurred in IFMS-Urban. First, an unstructured 2-D grid model was meshed with an average cell size of 15 m; second, ground elevations were assigned to each grid; and finally, each node was linked with a corresponding grid for water exchange, and the distribution of surface inundation was calculated with 2-D shallow-water equations. The coupled model had the advantages of SWMM and IFMS-Urban, and could be applied to simulate urban inundation and evaluate the performance of LID practices.
2.4 Scenarios of LID combinations for simulation
Considering the feasibility and representativeness of LID practices for urban inundation mitigation, we chose two types of LID practices, GR and PP, to simulate and explore their effectiveness for mitigation of urban inundation. The parameters for PP and GR are listed in Table 1, which were designed based on SWMM requirements and LID research (Ahiablame and Shakya, 2016; Chui et al., 2016; Kong et al., 2017; Qin et al., 2013). Through remote-sensing images and field investigations, we found that urban villages have diverse roof structures and shapes, which makes it difficult to implement green roofs. Therefore, we established principles for the implementation of LID practices: GR can only be built on low-density construction land, and PP can be built on low- and high-density construction land and on some streets. According to these principles, the available area for PP and GR was 5.95 and 8.92 km2, respectively. We set a series of proportions from 5 % to 100 % for the density of different types of LID combinations, and a benchmark and eight scenarios are designed below (benchmark: no LID practices):
-
Scenario 1 (S 1): 5 % GR + 5 % PP
-
Scenario 2 (S 2): 10 % GR + 10 % PP
-
Scenario 3 (S 3): 25 % GR + 25 % PP
-
Scenario 4 (S 4): 50 % GR + 50 % PP
-
Scenario 5 (S 5): 75 % GR + 75 % PP
-
Scenario 6 (S 6): 100 % GR + 100 % PP
-
Scenario 7 (S 7): 100 % PP
-
Scenario 8 (S 8): 100 % GR
3.1 Model calibration and validation
The coupled model was calibrated using rainfall and inundation data from 11 May 2014. Based on the relevant literature and the SWMM manual, we determined the final SWMM parameters (Table 2) through several calibration iterations. From the final calibration results (Table 3), we found that, except for inundation site Gm 20, the absolute value of the maximum inundation depth between the observed and simulated value was approximately 0–0.14 m and the relative error ranged from 0 %–30 %.
To further confirm the applicability of the model, the rainfall and inundation data on 10 May 2016 were chosen to validate the coupled model. Three valid datasets were simulated with the coupled model using observed urban inundation data on 10 May 2016 from the Guangming New District Urban Construction Bureau. The results showed that the absolute values of the differences between the observed and simulated maximum inundation depths were 0.04 (Gm 11), 0.05 (Gm 12), and 0.02 m (Gm 20), and the relative errors were 20 %, 7 %, and 5 %, respectively. In this study, the relative errors of calibration were a little higher, while the relative errors of validation were 5 %–20 %, which met the requirements of the Standard for Hydrologic Information and Hydrologic Forecasting in China (GBT_22482-2008).
3.2 Inundation depth under different scenarios
Figure 4 and Table 4 show the simulation results of inundation depths under different scenarios. Compared to the benchmark, the reduction rates of maximum inundation depth were 3 %, 7 %, 16 %, 22 %, 26 %, and 29 % under S 1 to S 6, respectively. The results for the 100 % PP and 100 % GR scenarios showed that PP and GR had approximately the same performance at the maximum inundation depth and that both scenarios reduced maximum inundation by 14 %.
To further explore the impacts of LID practices on inundation mitigation, on the basis of the literature (Su et al., 2016) and observed data for the study area we set three hazard levels for the depth of urban inundation: low (< 0.2 m), medium (0.2–0.4 m), and high (≥0.4 m). Compared to the benchmark, the ranges of average depth reduction rates were 15 %–80 %, 5 %–54 %, and 4 %–40 % at low, medium, and high hazard levels, respectively, for S 1 to S 6 (Fig. 5a). At different hazard levels, the average depth reduction rates increased from S 1 to S 6. The average depth reduction rates at the low level were 11 %, 26 %, 38 %, 44 %, 43 %, and 40 % higher than the high level under S 1 to S 6, respectively. These results suggest that most inundated areas could not be eliminated at the high level.
Figure 5a shows that the average depth reduction rates of 100 % PP and 100 % GR scenarios were between the 25 % GR + 25 % PP and 50 % GR + 50 % PP scenarios at different hazard levels. These results suggest that LID combinations may be more effective in reducing urban inundation than a single type of LID practice. Based on the comparison of the two LID practices, we found that the average depth reduction rates of the 100 % PP scenario were 67 %, 38 %, and 23 % at the low, medium, and high levels, respectively. These were 6 %, 7 %, and 2 % higher than the average depth reduction rates of the 100 % GR scenario. These results suggest that PP may perform better than GR for reducing the depth of inundation.
3.3 Inundation areas under different scenarios
Figure 5b shows changes in the inundation area under different scenarios and at different hazard levels. Compared to the benchmark, the ranges of average area reduction rates were 6 %–53 %, 17 %–75 %, and 24 %–90 % at low, medium, and high levels, respectively, for S 1 to S 6. The inundation areas reduced at different hazard levels after the implementation of LID practices. The average area reduction rates at the high level were up to 24 %–90 %, which were greater than those at the low level. This likely occurred because, after the implementation of LID practices, the depth of inundation decreased and most inundated areas were downgraded from a high level to a medium level or a low level.
For the 100 % PP and 100 % GR scenarios, the reduction in the inundation areas was similar to the 25 % PP + 25 % GR scenario, which also suggested that LID combinations are more effective than a single LID practice. The average area reduction rates for the 100 % PP scenario were 37 %, 65 %, and 67 % at the low, medium, and high levels, respectively, which were 5 %, 9 %, and 0 % higher than those for the 100 % GR scenario.
3.4 Inundation time under different scenarios
Inundation time is another way to represent inundation risk. Table 5 shows that the inundation time for medium and high levels was longer than the inundation time for the low level under the same scenario, which reflects increased risk of inundation at medium and high levels. As the implementation area of LID increased, the average inundation time decreased at the three hazard levels. The 100 % PP and 100 % GR scenarios had lower inundation time than the 25 % PP + 25 % GR scenario, and the inundation time for the 100 % PP scenario was 1.3 h less than the inundation time for the 100 % GR scenario.
Compared to the benchmark, the average inundation time under S 1 to S 3 changed slightly, while it decreased from S 4 to S 6. This result did not indicate that LID practices cannot decrease inundation time or that the model had errors. The inundation time decreased for all hazard levels, but for the low and medium levels some areas inundated for a short time were no longer flooded, which resulted in a different urban inundation area after the implementation of LID practices. Therefore, the average inundation time was longer than before LID practices were implemented at the low and medium levels. As LID practices were implemented, the average inundation time decreased continuously from 4.1 to 2.3 h under S 3 to S 6.
4.1 Performance of PP and GR
Researching the effectiveness of LID practices for urban inundation mitigation is important for storm water management. Our analysis showed that, although the implementation area of PP was less than that of GR, PP provided better urban inundation mitigation than GR. This result may have been due to differences in the LID parameters, but it may also have been caused by the PP's more diffuse spatial pattern. To better identify the effects of parameters, we carried out a sensitivity analysis by assuming a 50 % increase in some parameters under S 7 and S 8, and the results showed that the inundation depth has great sensitivities to some parameters (Table 6). Under the permeable-pavement scenario, the inundation decreases 15 %, 16 %, and 18 % with thickness of pavement layer, thickness of storage layer, and void ratio of storage layer, respectively. Under the rain roof scenario, the inundation decreases 17 % and 19 % with thickness and porosity of soil layer, respectively. The results indicate that LID parameters might influence the effectiveness of inundation mitigation.
Indeed, besides the LID parameters, there are some other factors – such as implementation area, spatial pattern, rainfall intensity, and rainfall frequency – that will influence the effectiveness, and these are the reasons why PP cannot always perform better and has shown varying effectiveness in different studies (Ahiablame and Shakya, 2016; Hu et al., 2017; Qin et al., 2013; Zhang et al., 2016). However, under certain scenarios of this study, PP may be a good choice for local governments because of its effectiveness for storm water management and its potential use for reconstruction in built-up areas. PP could be gradually applied to roads and parking lots, while GR is harder to implement in densely urbanized areas, especially in urban villages.
4.2 Effectiveness at different hazard levels
At the high level, the average depth reduction rates decreased from 4 % to 40 %, and the average area reduction rates decreased from 24 % to 90 % under S 1 to S 6. These results showed that the inundation hazard eased at a high level with the implementation of LID practices. However, at the high level, the average depth reduction rates were still 11 %–40 % lower and the average inundation time was 2.5–7 h longer when compared to the low level; this indicates that LID practices are more effective for urban inundation mitigation at a low hazard level. The hazard level analysis showed that, although LID practices can downgrade the inundation hazard level to medium or low, most inundated areas cannot be eliminated at a high hazard level. This means that the inundation problem could not been resolved only with LID practices, and we should recognize the insufficiencies of LID practices.
4.3 Cost-effectiveness of LID practices
Under S 1 to S 6, the effectiveness of LID practices for urban inundation mitigation increased with more area implementing LID practices. However, Table 4 and Fig. 5 showed that the reduction rates grew slowly with the increase of LID practices from 10 % to 100 %, which suggests that the efficiency of LID practices decreased from S 2 to S 6. A cost-effectiveness indicator (CEI) was used to better describe this phenomenon (Wu et al., 2017):
where R is the reduction rate of inundation depth and inundation area, and P is the proportion of LID practices, which means the cost. Table 7 showed that the CEI increased from S 1 to S 2 and decreased from S 2 to S 6, which means that the efficiency of the 10 % PP + 10 % GR scenario was higher than other scenarios. We clearly find that the reduction rates of maximum inundation depth are 7 %, 16 %, 22 %, 26 %, and 29 % from S 2 to S 6 and that the CEI has reduced continuously, especially from S 4 to S 6. This indicates that wider implementation of LID practices may not lead to higher efficiency.
One of the causes behind the phenomenon is that LID practices cannot control all the runoff of the watershed. Indeed, the runoff might come not only from sub-catchments around the inundation areas but also come from other sub-catchments through the roads and pipe networks. In this study, there are still some areas that cannot implement LID practices. Therefore, the runoff from these areas cannot be controlled by LID practices and directly influenced the effectiveness of inundation mitigation.
The phenomenon is common. In urban watershed, we could not transform all the roofs and roads to LID practices, and there are still some impervious covers that could influence the inundation that LID practices cannot control. Therefore, we should recognize the insufficiencies of LID practices and consider combining other measures such as restoring river systems, establishing urban wetlands, and improving urban drainage infrastructure to further promote the effectiveness of inundation mitigation. Furthermore, properly implementing construction intensity of LID practices to achieve optimal efficiency in urban watershed will be very important for the construction of a sponge city.
4.4 Limitations and future studies
Lacking accurate data is a common limitation for most studies. In this study, highly accurate elevation data for the study area is confidential and difficult to obtain; therefore, the ground elevation of streets was interpolated from the dense nodes of the pipe network. This method may have affected the simulation results. Moreover, the accuracy of the coupled model could be further increased with more accurate observed data and information of infrastructure, such as drainage pump station and river channel. Another limitation was that the definition of the thresholds for hazard levels was not considered sufficiently in this study. The results for the three hazard levels would be different if the thresholds changed. Therefore, research on criteria and sensitivity analysis of thresholds is needed in the future. The influences of rainfall intensity and frequency were not considered in this study, which is related to the effectiveness of LID.
In China, urban inundation appears to be increasing, and LID practices could be efficient strategies for urban inundation mitigation. At present, most research has focused on the area with LID practices and the effects on urban inundation mitigation. However, the spatial distribution and landscape patterns of LID practices also contribute to urban flooding mitigation (Giacomoni and Joseph, 2017; Kim and Park, 2016), but few studies have considered these variables. In addition, more studies should consider effective integration of LID practices into urban development (Chui et al., 2016), especially for places vulnerable to urban flooding.
This study constructed a 2-D inundation model that coupled SWMM and IFMS-Urban at the urban watershed scale; the model was used to evaluate the effectiveness of LID practices in mitigating urban inundation under different scenarios and at different hazard levels. We found that the coupled model could be applied to evaluate the effectiveness of LID for urban inundation risk mitigation. The model showed that PP was more effective for urban inundation mitigation than GR. This conclusion may be different in other regions, but it can be used by policy makers on a local basis. LID practices can only affect the inundation depth and downgrade the inundation hazard level; they cannot resolve inundation problems at a high hazard level. Therefore, other methods of storm water management should also be applied to manage severe waterlogging. In the construction of a sponge city, people paid more attention to the effectiveness, while ignoring the cost of LID. Through the analysis of cost-effectiveness, we found that wider implementation of LID practices may not lead to higher efficiency in urban watershed, and the cost and effectiveness of LID practices should be considered in the construction of a sponge city.
The DEM of the study area can be downloaded from the Geospatial Data Cloud, and the weather data can be sourced from the Shenzhen Meteorological Data System. The rest of the data are not publicly available but can be obtained by contacting the local government.
The study was conceived by JW and RY, and the methodology was designed with the contribution of all authors. RY carried out the data analysis and prepared the first version of the manuscript with the guidance of JW and JS. All authors discussed the results and contributed to editing the final version of the article.
The authors declare that they have no conflict of interest.
This research was supported by the National
Natural Science Foundation of China (grant nos. 41330747 and 41471370).
Edited by: Heidi Kreibich
Reviewed by: two anonymous referees
Abbot, C. L. and Comino-Mateos, L.: In-situ hydraulic performance of a permeable pavement sustainable urban drainage system, J. Chart. Inst. Water E., 17, 187–190, 2003.
Ahiablame, L. and Shakya, R.: Modeling flood reduction effects of low impact development at a watershed scale, J. Environ. Manage., 171, 81–91, https://doi.org/10.1016/j.jenvman.2016.01.036, 2016.
Ahiablame, L. M., Engel, B. A., and Chaubey, I.: Effectiveness of Low Impact Development Practices: Literature Review and Suggestions for Future Research, Water Air Soil Poll., 223, 4253–4273, https://doi.org/10.1007/s11270-012-1189-2, 2012.
Ahiablame, L. M., Engel, B. A., and Chaubey, I.: Effectiveness of low impact development practices in two urbanized watersheds: retrofitting with rain barrel/cistern and porous pavement, J. Environ. Manage., 119, 151–161, https://doi.org/10.1016/j.jenvman.2013.01.019, 2013.
Arnold, C. L. and Gibbons, C.J.: Impervious surface coverage – The emergence of a key environmental indicator, J. Am. Plann. Assoc., 62, 243–258, https://doi.org/10.1080/01944369608975688, 1996.
Beckers, A., Dewals, B., Erpicum, S., Dujardin, S., Detrembleur, S., Teller, J., Pirotton, M., and Archambeau, P.: Contribution of land use changes to future flood damage along the river Meuse in the Walloon region, Nat. Hazards Earth Syst. Sci., 13, 2301–2318, https://doi.org/10.5194/nhess-13-2301-2013, 2013.
Berndtsson, J. C.: Green roof performance towards management of runoff water quantity and quality: A review, Ecol. Eng., 36, 351–360, https://doi.org/10.1016/j.ecoleng.2009.12.014, 2010.
Bhattarai, R., Yoshimura, K., Seto, S., Nakamura, S., and Oki, T.: Statistical model for economic damage from pluvial floods in Japan using rainfall data and socioeconomic parameters, Nat. Hazards Earth Syst. Sci., 16, 1063–1077, https://doi.org/10.5194/nhess-16-1063-2016, 2016.
Bliss, D. J., Neufeld, R. D., and Ries, R. J.: Storm Water Runoff Mitigation Using a Green Roof, Environ. Eng. Sci., 26, 407–417, https://doi.org/10.1089/ees.2007.0186, 2009.
Burns, M. J., Schubert, J. E., Fletcher, T. D., and Sanders, B. F.: Testing the impact of at-source stormwater management on urban flooding through a coupling of network and overland flow models, Wiley Interdiscip. Rev.-Water, 2, 291–300, https://doi.org/10.1002/wat2.1078, 2015.
Chui, T. F. M., Liu, X., and Zhan, W.: Assessing cost-effectiveness of specific LID practice designs in response to large storm events, J. Hydrol., 533, 353–364, https://doi.org/10.1016/j.jhydrol.2015.12.011, 2016.
Claessens, L., Hopkinson, C., Rastetter, E., and Vallino, J.: Effect of historical changes in land use and climate on the water budget of an urbanizing watershed, Water Resour. Res., 42, 446–455, https://doi.org/10.1029/2005wr004131, 2006.
Damodaram, C., Giacomoni, M. H., Prakash Khedun, C., Holmes, H., Ryan, A., Saour, W., and Zechman, E. M.: Simulation of Combined Best Management Practices and Low Impact Development for Sustainable Stormwater Management, J. Am. Water Resour. As., 46, 907–918, https://doi.org/10.1111/j.1752-1688.2010.00462.x, 2010.
Davis, A. P.: Field performance of bioretention: Hydrology impacts, J. Hydrol. Eng., 13, 90–95, https://doi.org/10.1061/(asce)1084-0699(2008)13:2(90), 2008.
Davis, A. P., Traver, R. G., Hunt, W. F., Lee, R., Brown, R. A., and Olszewski, J. M.: Hydrologic Performance of Bioretention Storm-Water Control Measures, J. Hydrol. Eng., 17, 604–614, https://doi.org/10.1061/(asce)he.1943-5584.0000467, 2012.
Dietz, M. E.: Low Impact Development Practices: A Review of Current Research and Recommendations for Future Directions, Water Air Soil Pollut., 186, 351–363, https://doi.org/10.1007/s11270-007-9484-z, 2007.
Dietz, M. E. and Clausen, J. C.: Stormwater runoff and export changes with development in a traditional and low impact subdivision, J. Environ. Manage., 87, 560–566, https://doi.org/10.1016/j.jenvman.2007.03.026, 2008.
Dreelin, E. A., Fowler, L., and Ronald Carroll, C.: A test of porous pavement effectiveness on clay soils during natural storm events, Water Res., 40, 799–805, https://doi.org/10.1016/j.watres.2005.12.002, 2006.
EPA: Low impact development (LID), A literature review, EPA-841-B-00e005, Office of Water, Washington, DC, 2000.
Fassman, E. A. and Blackbourn, S.: Urban Runoff Mitigation by a Permeable Pavement System over Impermeable Soils, J. Hydrol. Eng., 15, 475–485, https://doi.org/10.1061/(asce)he.1943-5584.0000238, 2010.
Fewtrell, T. J., Neal, J. C., Bates, P. D., and Harrison, P. J.: Geometric and structural river channel complexity and the prediction of urban inundation, Hydrol. Process., 25, 3173–3186, https://doi.org/10.1002/hyp.8035, 2011.
Giacomoni, M. H. and Joseph, J.: Multi-Objective Evolutionary Optimization and Monte Carlo Simulation for Placement of Low Impact Development in the Catchment Scale, J. Water Resour. Plan. Manage.-ASCE, 143, 15, https://doi.org/10.1061/(asce)wr.1943-5452.0000812, 2017.
Hao, L., Sun, G., Liu, Y., Wan, J., Qin, M., Qian, H., Liu, C., Zheng, J., John, R., Fan, P., and Chen, J.: Urbanization dramatically altered the water balances of a paddy field-dominated basin in southern China, Hydrol. Earth Syst. Sci., 19, 3319–3331, https://doi.org/10.5194/hess-19-3319-2015, 2015.
Hatt, B. E., Fletcher, T. D., Walsh, C. J., and Taylor, S. L.: The influence of urban density and drainage infrastructure on the concentrations and loads of pollutants in small streams, Environ. Manage., 34, 112–124, https://doi.org/10.1007/s00267-004-0221-8, 2004.
Hood, M. J., Clausen, J. C., and Warner, G. S.: Comparison of stormwater lag times for low impact and traditional residential development, J. Am. Water Resour. As., 43, 1036–1046, https://doi.org/10.1111/j.1752-1688.2007.00085.x, 2007.
Hu, M., Sayama, T., Zhang, X., Tanaka, K., Takara, K., and Yang, H.: Evaluation of low impact development approach for mitigating flood inundation at a watershed scale in China, J. Environ. Manage., 193, 430–438, https://doi.org/10.1016/j.jenvman.2017.02.020, 2017.
Hunt, W. F., Traver, R. G., Davis, A. P., Emerson, C. H., Collins, K. A., and Stagge, J. H.: Low Impact Development Practices: Designing to Infiltrate in Urban Environments, in: Effects of Urbanization on Groundwater, edited by: Ni-Bin, C., American Society of Civil Engineers, Reston, VA, USA, 308–343, 2010.
Huong, H. T. L. and Pathirana, A.: Urbanization and climate change impacts on future urban flooding in Can Tho city, Vietnam, Hydrol. Earth Syst. Sci., 17, 379–394, https://doi.org/10.5194/hess-17-379-2013, 2013.
Jacobson, C. R.: Identification and quantification of the hydrological impacts of imperviousness in urban catchments: a review, J. Environ. Manage., 92, 1438–1448, https://doi.org/10.1016/j.jenvman.2011.01.018, 2011.
Kim, H. W. and Park, Y.: Urban green infrastructure and local flooding: The impact of landscape patterns on peak runoff in four Texas MSAs, Appl. Geogr., 77, 72–81, https://doi.org/10.1016/j.apgeog.2016.10.008, 2016.
Kong, F. H., Ban, Y. L., Yin, H. W., James, P., and Dronova, I.: Modeling stormwater management at the city district level in response to changes in land use and low impact development, Environ. Modell. Softw., 95, 132–142, https://doi.org/10.1016/j.envsoft.2017.06.021, 2017.
Leopold, L. B., Wolman, M. G., and Miller, J. P.: Fluvial Processes in Geomorphology, Geographical J., 131, 1995.
Liu, Y., Ahiablame, L. M., Bralts, V. F., and Engel, B. A.: Enhancing a rainfall-runoff model to assess the impacts of BMPs and LID practices on storm runoff, J. Environ. Manage., 147, 12–23, https://doi.org/10.1016/j.jenvman.2014.09.005, 2015.
Lowe, R., Urich, C., Domingo, N. S., Mark, O., Deletic, A., and Arnbjerg-Nielsen, K.: Assessment of urban pluvial flood risk and efficiency of adaptation options through simulations – A new generation of urban planning tools, J. Hydrol., 550, 355–367, https://doi.org/10.1016/j.jhydrol.2017.05.009, 2017.
Luan, Q., Fu, X., Song, C., Wang, H., Liu, J., and Wang, Y.: Runoff Effect Evaluation of LID through SWMM in Typical Mountainous, Low-Lying Urban Areas: A Case Study in China, Water, 9, 439, https://doi.org/10.3390/w9060439, 2017.
Mao, X., Jia, H., and Yu, S. L.: Assessing the ecological benefits of aggregate LID-BMPs through modelling, Ecol. Model., 353, 139–149, https://doi.org/10.1016/j.ecolmodel.2016.10.018, 2017.
Meyer, M. J. P. A. J. L.: Streams in the urban landscape, Annu. Rev. Ecol. Syst., 32, 333–365, https://doi.org/10.1146/annurev.ecolsys.32.081501.114040, 2001.
Morsy, M. M., Goodall, J. L., Shatnawi, F. M., and Meadows, M. E.: Distributed Stormwater Controls for Flood Mitigation within Urbanized Watersheds: Case Study of Rocky Branch Watershed in Columbia, South Carolina, J. Hydrol. Eng., 21, 05016025, https://doi.org/10.1061/(asce)he.1943-5584.0001430, 2016.
Palla, A. and Gnecco, I.: Hydrologic modeling of Low Impact Development systems at the urban catchment scale, J. Hydrol., 528, 361–368, https://doi.org/10.1016/j.jhydrol.2015.06.050, 2015.
Qin, H. P., Li, Z. X., and Fu, G.: The effects of low impact development on urban flooding under different rainfall characteristics, J. Environ. Manage., 129, 577–585, https://doi.org/10.1016/j.jenvman.2013.08.026, 2013.
Rose, S. and Peters, N. E.: Effects of urbanization on streamflow in the Atlanta area (Georgia, USA): a comparative hydrological approach, Hydrol. Process., 15, 1441–1457, https://doi.org/10.1002/hyp.218, 2001.
Rossman, L. A.: Storm water management model user's manual version 5.0, U.S. Environmental Protection Agency, Washington, DC, EPA/600/R-05040, 2010.
Roy, A. H., Wenger, S. J., Fletcher, T. D., Walsh, C. J., Ladson, A. R., Shuster, W. D., Thurston, H. W., and Brown, R. R.: Impediments and solutions to sustainable, watershed-scale urban stormwater management: lessons from Australia and the United States, Environ. Manage., 42, 344–359, https://doi.org/10.1007/s00267-008-9119-1, 2008.
Russo, B., Sunyer, D., Velasco, M., and Djordjevic, S.: Analysis of extreme flooding events through a calibrated 1D/2D coupled model: the case of Barcelona (Spain), J. Hydroinform., 17, 473–491, https://doi.org/10.2166/hydro.2014.063, 2015.
Salvadore, E., Bronders, J., and Batelaan, O.: Hydrological modelling of urbanized catchments: A review and future directions, J. Hydrol., 529, 62–81, https://doi.org/10.1016/j.jhydrol.2015.06.028, 2015.
Sang, Y.-F. and Yang, M.: Urban waterlogs control in China: more effective strategies and actions are needed, Nat. Haz., 85, 1291–1294, https://doi.org/10.1007/s11069-016-2614-4, 2016.
Seyoum, S. D., Vojinovic, Z., Price, R. K., and Weesakul, S.: Coupled 1D and Noninertia 2D Flood Inundation Model for Simulation of Urban Flooding, J. Hydraul. Eng.-Asce, 138, 23–34, https://doi.org/10.1061/(asce)hy.1943-7900.0000485, 2012.
Son, A.-L., Kim, B., and Han, K.-Y.: A Simple and Robust Method for Simultaneous Consideration of Overland and Underground Space in Urban Flood Modeling, Water, 8, 494, https://doi.org/10.3390/w8110494, 2016.
Su, B., Huang, H., and Li, Y.: Integrated simulation method for waterlogging and traffic congestion under urban rainstorms, Nat. Haz., 81, 23–40, https://doi.org/10.1007/s11069-015-2064-4, 2016.
Trinh, D. H. and Chui, T. F. M.: Assessing the hydrologic restoration of an urbanized area via an integrated distributed hydrological model, Hydrol. Earth Syst. Sci., 17, 4789–4801, https://doi.org/10.5194/hess-17-4789-2013, 2013.
U.S. Department of Housing, and Urban Development: The practice of low impact development, Contract No. H-21314CA, Office of Policy Development and Research, NAHB Research Center, Washington, DC, 2003.
Wu, J. Y., Thompson, J. R., Kolka, R. K., Franz, K. J., and Stewart, T. W.: Using the Storm Water Management Model to predict urban headwater stream hydrological response to climate and land cover change, Hydrol. Earth Syst. Sci., 17, 4743–4758, https://doi.org/10.5194/hess-17-4743-2013, 2013.
Wu, X., Wang, Z., Guo, S., Liao, W., Zeng, Z., and Chen, X.: Scenario-based projections of future urban inundation within a coupled hydrodynamic model framework: A case study in Dongguan City, China, J. Hydrology, 547, 428–442, https://doi.org/10.1016/j.jhydrol.2017.02.020, 2017.
Xia, J., Zhang, Y., Xiong, L., He, S., Wang, L., and Yu, Z.: Opportunities and challenges of the Sponge City construction related to urban water issues in China, Sci. China Earth Sci., 60, 652–658, https://doi.org/10.1007/s11430-016-0111-8, 2017.
Zahmatkesh, Z., Burian, S. J., Karamouz, M., Tavakol-Davani, H., and Goharian, E.: Low-Impact Development Practices to Mitigate Climate Change Effects on Urban Stormwater Runoff: Case Study of New York City, J. Irrig. Drain. E., 141, 04014043, https://doi.org/10.1061/(asce)ir.1943-4774.0000770, 2015a.
Zahmatkesh, Z., Karamouz, M., Goharian, E., and Burian, S. J.: Analysis of the Effects of Climate Change on Urban Storm Water Runoff Using Statistically Downscaled Precipitation Data and a Change Factor Approach, J. Hydrol. Eng., 20, 05014022, https://doi.org/10.1061/(asce)he.1943-5584.0001064, 2015b.
Zhang, X., Guo, X., and Hu, M.: Hydrological effect of typical low impact development approaches in a residential district, Nat. Haz., 80, 389–400, https://doi.org/10.1007/s11069-015-1974-5, 2016.
Zhu, Z., Chen, Z., Chen, X., and He, P.: Approach for evaluating inundation risks in urban drainage systems, Sci. Total Environ., 553, 1–12, https://doi.org/10.1016/j.scitotenv.2016.02.025, 2016.