the Creative Commons Attribution 4.0 License.
the Creative Commons Attribution 4.0 License.
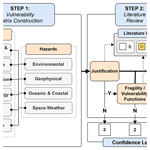
Review article: Insuring the green economy against natural hazards – charting research frontiers in vulnerability assessment
Harikesan Baskaran
Ioanna Ioannou
Tiziana Rossetto
Jonas Cels
Mathis Joffrain
Nicolas Mortegoutte
Aurelie Fallon Saint-Lo
Catalina Spataru
The insurance of green economy assets against natural hazards is a growing market. This study explores whether currently available published knowledge is adequate for the vulnerability assessment of these assets to natural hazards. A matrix is constructed to demonstrate the vulnerability to functional loss of 37 asset classes in the renewable energy, green construction, resource management, carbon capture and storage, energy storage, and sustainable transportation sectors. The 28 hazards adopted range from environmental and geophysical events to oceanic, coastal, and space weather events. A fundamental challenge in constructing the matrix was the lack of an asset–hazard taxonomy for the green economy. Each matrix cell represents the vulnerability of an asset to a specific hazard, based on a comprehensive systematic literature review. A confidence level is assigned to each vulnerability assessment based on a literature density heat map. The latter highlights specific knowledge gaps, in particular a lack of quantitative vulnerability studies that appropriately represent all functional loss mechanisms in green economy assets. Apart from charting research gaps, a main output of this study is the proposal of a representative asset–hazard taxonomy to guide future quantitative research that can be applied by the insurance industry.
- Article
(1503 KB) - Full-text XML
- BibTeX
- EndNote
Climate change is one of the most serious emerging risks faced by the world today. It is partly the consequence of the industrialization of the world economy and its heavy reliance on fossil fuels. In response, governments worldwide have increasingly shifted their focus toward building a greener economy, with many pledging to achieve net-zero greenhouse gas emissions by 2050 (e.g. King's Printer of Acts of Parliament, 2019). This transition toward net zero requires significant investments in assets supporting the green economy. FTSE Russell (2023) estimates the green economy to be the fourth largest standalone sector in the global stock markets, with a value of USD 6.5 trillion. Additionally, green buildings in emerging market cities present a USD 24.7 trillion investment opportunity (IFC, 2019), while electric vehicles are projected to account for 35 % of the global car market (IEA, 2023). According to McKinsey (2022), global investments in decarbonization (e.g. carbon capture and storage, electric vehicle charging) and renewable energy (e.g. solar power and onshore and offshore wind) technologies could reach USD 800 billion per year by 2030, which corresponds to insurance premiums of USD 10–15 billion per year on capital expenditures alone.
From an insurance sector perspective, this redirection of exposure (Nature Communications, 2023) towards the green economy offers significant opportunities for insuring new asset types (e.g. Sumaila et al., 2021). For instance, Buchana and McSharry (2019) estimated an annual probable maximum loss of EUR 1.267 billion from extratropical cyclones, for a portfolio of 38 offshore wind farms in the North Sea. However, this shift introduces challenges, notably in setting insurance premiums for the protection of green economy assets against natural hazards. This is particularly critical as insurance plays a pivotal role in enhancing resilience and the expanding green asset deployment, especially considering that they are increasingly being established in more hazard-prone regions due to land-use pressures (GCube Underwriting, 2021).
Assessing the vulnerability of green economy assets to natural hazards poses a formidable challenge. While natural catastrophes already account for a fifth of global insurance claims in the construction sector (Allianz Global Corporate & Specialty, 2023), the impact of integrating more green buildings on overall vulnerability and ensuing claims remains uncertain. Similarly, while electric vehicles may be up to 15 % more expensive to repair than conventional vehicles (Chatterjee et al., 2023), their resilience to natural hazards compared to conventional counterparts is unclear. Challenges in vulnerability evaluation arise primarily from the complexity of green economy assets, comprising intricate engineered and/or nature-based systems that are difficult to model. Being relatively new technologies/constructions, they lack historical exposure to extreme climatic or geophysical hazard events. This results in a scarcity of damage and claims data and models for insurers to base their vulnerability and risk evaluations upon. This is true even for the more established solar and wind energy sectors (Lloyd's, 2020), for which some catastrophe modelling and insurance products exist.
Given this data scarcity, insurers could rely more on published knowledge sources to justify their vulnerability models. But the following question arises: how useful is the current literature landscape for this purpose? This study aspires to highlight the limitations of existing published research in informing vulnerability assessments of key green assets to different natural hazards.
In this study, green assets encompass all insurable assets and associated activities that directly contribute to reducing carbon emissions or/and protecting nature. This study focuses on 37 key asset classes, identified across renewable energy, green construction, sustainable transportation, natural resource management, carbon capture and storage, and energy storage sectors. Regarding natural hazards, 28 environmental, geophysical, oceanic, and coastal hazards have been selected. A matrix is constructed by the defined assets and hazards, with each intersection representing the vulnerability of the asset to that hazard. Vulnerability, in this context, refers to the likelihood of functional loss and is represented by a qualitative index based on evidence from a systematic review of readily accessible literature for each intersection. Knowledge gaps are highlighted through a literature heat map, and a discussion of these research gaps and their implications for insuring green economy assets against natural hazards is presented.
The vulnerability assessment of green assets proposed in this study follows a structured approach composed of three steps, as illustrated in Fig. 1. Firstly, 37 assets and 28 hazards are defined, forming a comprehensive list of 1036 asset–hazard intersections, which serve as the basis for our vulnerability matrix. The second step involves a systematic review of global literature relevant to each intersection. This review aims to gauge the relevance and applicability of existing literature in determining vulnerability. In the third step, the results from the literature review are used to assign a qualitative vulnerability index to each asset–hazard intersection. Additionally, this step involves evaluating the confidence level associated with each vulnerability rating. This evaluation hinges on whether the reviewed literature comprises only qualitative information or includes quantitative fragility/vulnerability functions specific to the analysed asset–hazard pair. In what follows, the three steps are described in greater detail.
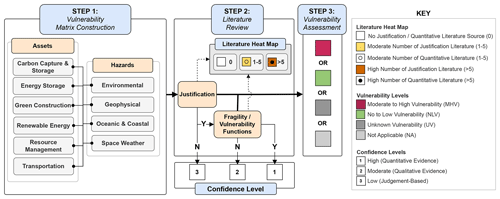
Figure 1Flowchart of developed methodology. An asset–hazard taxonomy is defined for vulnerability matrix construction in Step 1. At each matrix intersection, a systematic literature review is conducted and a heat map is developed in Step 2, enabling confidence levels to be assigned to the vulnerabilities ratings made in Step 3.
2.1 Step 1: vulnerability matrix construction
It has been observed that existing exposure taxonomy and hazard classification systems do not perfectly align with the needs of vulnerability assessments of green economy assets across various hazard types. Therefore, Step 1 focuses on adapting existing taxonomy and classification systems to allow the construction of the vulnerability matrix.
2.1.1 Defining green assets
An exposure taxonomy is essential for conducting vulnerability assessments of green assets across a spectrum of natural hazards. However, existing exposure taxonomies are often tailored to specific hazards and cover a limited range of assets. For instance, the SYNER-G (European Commission, 2019) taxonomy addresses critical infrastructure exposed to earthquakes, whilst others, like GED4GEM by Gamba et al. (2012), consider multiple hazards but overlook green economy assets such as green buildings. At present, there is no operational taxonomy available for the green economy assets that can be used by the insurance sector.
Given the lack of a comprehensive exposure taxonomy suitable for this study, we propose a broad definition of exposure based on categories of green economy assets. This approach aligns with the qualitative and macroscopic nature of vulnerability assessment conducted here. The assets are intentionally assessed as systems, without a detailed study of all their interacting components. Six primary sectors of the green economy are considered, based on the UK's net-zero policy (UK Government, 2021): renewable energy sources, green construction, transport with a focus on electric vehicles, resource management, CO2 reduction, and energy storage. Through discussions between authors at University College London and AXA Group, we identified the operational requirement of the insurance sector. This collaboration led to the development of an initial version of an operational green asset taxonomy. It includes at least two common insurable subsector assets per sector, ensuring broad coverage. These assets were chosen to reflect key areas requiring innovation and investment to meet the policy's objectives (UK Government, 2021). Each asset is defined at a level that can be priced and is practical for the insurance industry, and this includes assets currently being insured. In total, 37 assets are identified and described in detail (see Table A1 in the Appendix).
The majority of assets (20 in total) pertain to renewable energy production, including technologies such as wind, solar, marine, and geothermal energy and hydropower. This includes both established (e.g. offshore bed-fixed wind farms) and emerging infrastructure and technologies (e.g. floating offshore wind farms, floating photovoltaics, wave energy, and tidal stations). Energy distribution networks connected to renewable energy assets are excluded from the taxonomy. For energy storage infrastructure, existing assets such as electric batteries and hydrogen storage facilities are considered, as well as flywheels, which are still at the experimental stage. For biomass and biofuels, the forests and crops, respectively, which provide the raw material, as well as the industrial facilities that turn raw materials into energy, are identified as assets. Facilities for biogas production are treated separately, reflecting the distinct processes involved in anaerobic digestion and gas valorization.
It should be noted that facilities for the production of nuclear energy, although part of the net-zero policy, are not considered here as this type of energy was deemed clean but not green energy. For the transport sector, both land-based and water-based electric vehicles are included. The construction sector is represented by only two asset groups, based on green buildings, i.e. mass timber buildings and ordinary buildings retrofitted to reduce their environmental impact. For CO2 reduction, apart from the industrial facilities for carbon capture or storage, key ecosystems which capture and store carbon are considered, such as marshlands and peatlands, as well as protected and unprotected forests. In resource management, three marine ecosystems are included. Amongst them are coral reefs (The Nature Conservancy, 2022) and mangrove forests (Beck et al., 2020), which have been the recent focus of the insurance industry, with policies to protect and restore them, so they can be effective in defending coastal areas from natural hazards.
2.1.2 Defining hazards
A classification system is needed for representing key characteristics of hazards that can impact asset vulnerability. Many hazard classification systems exist (e.g. UNDRR and ISC, 2020; UNDRR, 2023). Similar to in the case of exposure (Sect. 2.1.1), existing hazard taxonomies are defined with mainly engineered assets in mind. They largely ignore hazard characteristics that can affect the vulnerability of nature-based assets. Notably, hazard duration, identified as a significant factor affecting nature-based asset vulnerability in the literature review conducted during Step 2, is frequently overlooked in existing hazard taxonomies. For example, the vulnerability of crops to a short-term heatwave versus a prolonged rise in temperature due to climate change can vary significantly. This difference arises because crop yield loss depends on the duration of exposure to temperatures above those optimal for growth (Brás et al., 2021; Zampieri et al., 2017). A new, operational hazard taxonomy for the insurance sector is therefore proposed herein that is based on the existing hazard taxonomy by UNDRR and ISC (2020), with the addition of hazard process duration in hazard descriptions. Similar to the approach used for green assets (Sect. 2.1.1), hazards were prioritized and consolidated through author discussions. This process ensured that the selected hazards were the most relevant for the chosen assets and practical for use at an operational level within the insurance industry. Unlike some risk evaluation taxonomies (e.g. RDLS, 2024), the proposed taxonomy focuses on single-hazard processes and ignores triggered, concurrent, sequential, or cascading hazards. Unlike RDLS (2024), no intensity measures are defined in the proposed taxonomy, as these are not needed for the vulnerability matrix construction herein.
The proposed hazard classification system consists of 28 natural hazard processes, categorized into four clusters: environmental (16 processes), geophysical (7 processes), oceanic and coastal (4 processes), and space weather (1 process). Table B1 provides a detailed description for each natural hazard.
2.2 Step 2: literature review
A global, systematic review of the literature was conducted for each intersection with the aim of providing justification for the vulnerability rating. A combination of asset and hazard names from the developed green economy taxonomy, alongside the terms “fragility” and “vulnerability”, were searched within easily accessible, web-based literature databases (e.g. Google Scholar). Where literature results were found to be insufficient to give a representative vulnerability rating, alternative keywords were used, before reference lists of relevant published literature were searched manually. All literature results found were included in the assessment and were only excluded when a potential or definitive report or discussion of effect, damage, vulnerability, or loss of function was not present.
The literature reviewed includes five different sources of diminishing perceived quality (Table 1). The literature regarded to be of highest quality (Tier 1) and reliability includes recent peer-reviewed articles or published research reports on the asset–hazard interaction, including fragility/vulnerability functions for key components of the asset. Tier 2 literature comprises peer-reviewed articles and scientific reports from reputable agencies but does not contain significant or useful fragility/vulnerability functions for asset components. In the absence of abundant Tier 2 literature, the review focuses on post-disaster reconnaissance reports and/or damage/loss databases from utility providers. Such sources are regarded as having lower quality albeit still very good reliability (Tier 3). These are often not peer-reviewed and present observations of asset performance specific to a particular hazard event or asset type (i.e. are less generalizable). Tier 4 sources comprise design guidelines or manuals for different asset types. Use of these assumes that assets comply with these codes and guidelines, and hence they were only considered in cases where other literature found did not suggest non-compliance as being common practice. When long-duration hazards are assessed, such as rising sea levels, these codes are assumed to be stagnant unless the trajectory of change in design is clear in other literature reviewed. If the literature sources were not accessible, a broad internet search (e.g. via Google) was conducted to identify news reports or blogs that could provide examples of catastrophic failures of a particular asset due to a given hazard (Tier 5).
Given the variability in the quality and reliability of the literature sources, confidence levels are assigned to each of the asset vulnerability assessments of Step 3, as shown in Table 1. High confidence, CL1, is assigned where the literature review yielded fragility/vulnerability functions for the asset or its key components (i.e. where Tier 1 literature was available and deemed reliable and relevant). Moderate confidence, CL2, is assigned to vulnerability assessments made based on Tier 2 to 4 literature sources. Finally, a low confidence (CL3) is assigned to vulnerability assessments made based on Tier 5 sources or where no relevant literature was found. In the latter case, the vulnerability assessment is made based on the authors' judgement and experience. It was observed by the authors that assets with a low-exposure history, including relatively new technologies/constructions, generally lacked an academic literature base. Here, the vulnerability of similar asset types was considered. In particular, where there was a lack of literature, e-fuels and hydrogen (small-scale) storage tanks were approximated to have failure mechanisms similar to steel storage tanks and silos; hydrogen (large scale) and industrial carbon storage were compared with salt caverns and depleted oil and gas wells; geothermal, concentrated solar power (CSP) plants, and biomass industrial facilities were compared with thermal power plant components, such as cooling systems, fans, and turbine generators; land and water-based electric vehicles were compared against fossil-fuel based vehicles where the failure mechanisms were similar.
In the discussion of the vulnerability matrix (see Sect. 3), the high and moderate confidence ratings are grouped together and are distinguished from the intersections based on low confidence ratings for clarity.
2.3 Step 3: vulnerability assessment
A qualitative vulnerability level is assigned to each asset–hazard pair within the matrix, using the vulnerability scale outlined in Table 2. Functional loss is a broad term covering both performance reduction and direct damage, commonly referred to as business interruption and property damage, respectively, in insurance contracts, where they are generally covered. Vulnerability to functional loss in this paper does not specifically distinguish between business interruption and property damage, as the two are closely linked, with business interruption commonly resulting from property damage and the subsequent recovery process (Rose and Huyck, 2016). Consequently, the existing literature rarely provides sufficient detail on the contribution of each to the overall losses observed. Hence, we define moderate to high vulnerability (termed hereafter MHV) as the expectation of significant asset functional loss under low to medium hazard intensities and no to low vulnerability (termed hereafter NLV) as the expectation of limited or no asset functional loss under high hazard intensity levels.
During the matrix construction, vulnerability levels are assigned to each asset–hazard pair based on findings from the literature review or through expert judgement when literature is lacking, as described in Sect. 2.2. In this assessment assets are generalized, and adaptation measures or mitigation technologies are not considered, unless literature mentions them as a minimum requirement. Therefore, the worst-case vulnerability rating is provided in the matrix. In cases where the authors are unable to reasonably assess the likelihood of an asset's functional loss for a specific hazard, a vulnerability level of “unknown” (UV) is assigned. For example, a UV level was assigned to biogas (anaerobic digester) industrial facilities under extreme cold events as literature was only found addressing their performance reduction under suboptimal temperatures (Alvarez and Lidén, 2008) and during the winter season (Pham et al., 2014). Finally, a vulnerability level of “not applicable” (NA) is applied where no literature and/or knowledge exists on the performance of an asset under a specific hazard. Commonly, this is due to the location of the asset being such that it is highly unlikely or never exposed to that specific hazard.
The only exception in applying these levels was the assessment of the green retrofitted buildings, where vulnerability was assessed relative to a building without retrofitting, as more (>V), equally (=V), or less vulnerable (<V). For the purpose of the results, >V and =V were assumed to correspond with MHV, whilst <V corresponded with NLV.
It should be noted that for assets comprising multiple components, system-wide losses were considered in the vulnerability assessment. For example, the extent of loss was assessed on the whole forest rather than on individual trees and for entire wind farms rather than individual wind turbines. However, the criticality of individual components to system functionality is also considered. For example, if a hazard event is likely to destroy only a single tree or wind turbine, the overall impact on the system is limited – hence a limited vulnerability. But if a transformer fails within a wind farm or power plant, this could lead to a system-level shutdown – hence a high vulnerability.
The vulnerability ratings of the 1064 unique intersections, conducted according to the proposed methodology, are presented in Table 3. Among these, 396 intersections received MHV ratings, while 341 intersections were classified as NLV. Additionally, 123 intersections were assigned UV ratings due to gaps in the literature.
Table 3Asset–hazard vulnerability matrix (with ratings formatted as per Table 2) with confidence levels (as per Table 1). Refer to Tables A1 and B1 for asset and hazard codes.
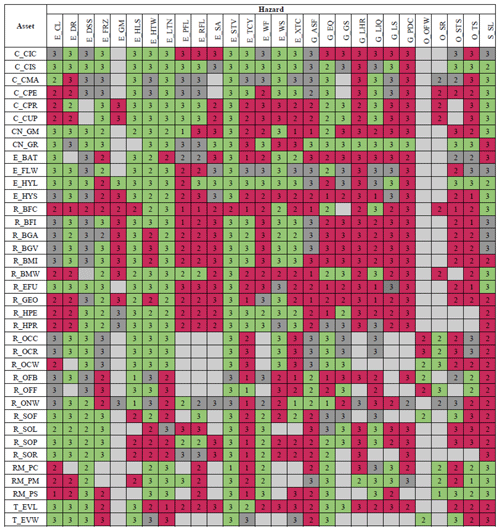
Of the total assessments, only 337 were based on relevant literature and thus associated with confidence levels (CLs) 1 or 2, indicating a higher degree of confidence. In contrast, the majority (526) of judgements were made in the absence of relevant literature (CL3). Moreover 173 intersections were categorized as NA due to improbable geographical coexistence of the asset and hazard. For example, offshore wind farms are not exposed to snow avalanches or glacial melt, warranting an NA vulnerability rating.
The presence of UV ratings highlights a significant gap in the literature. For instance, the absence of literature on the performance of flywheels during sandstorms led to a UV rating, reflecting the potential for geographical coexistence. However, for a small number of intersections a UV rating was assigned, despite the presence of literature as it was found to be insufficient, inconclusive, or under scientific debate. For example, a conclusive generic vulnerability assessment of marshlands impacted by rising sea levels cannot be made from published literature, as it is site-specific and strongly depends on the interaction between tidal imports, the species of vegetation, and depositional processes (Reed, 1995). Similarly, the net impact of storm surge on marshland is under scientific debate. Following hurricanes Katrina and Rita in 2005, there was a net deposition (5.18 cm on average) of organic and inorganic material, but storm waves can also lead to surface excavation or edge erosion (FitzGerald and Hughes, 2019). Whilst peatlands rapidly decline when impacted by ash deposits (Zhang et al., 2022), there is insufficient literature identifying the root cause of this relationship, with acidic loading considered a possible reason (Giles et al., 1999).
Table 4 presents a heat map of the literature for the intersections. In this table, the colour indicates the amount of literature found to justify the vulnerability rating for the asset–hazard intersection. The filled and empty circles represent whether literature sources containing quantitative expressions of the asset's vulnerability to the hazard exist. Quantitative studies were found for only 4 % of the total number of intersections corresponding to 18 assets, with the vast majority being fragility (or physical vulnerability) functions focusing on property damage.
Table 4Literature heat map (mapped and formatted as per Fig. 1). Refer to Tables A1 and B1 for asset and hazard codes.
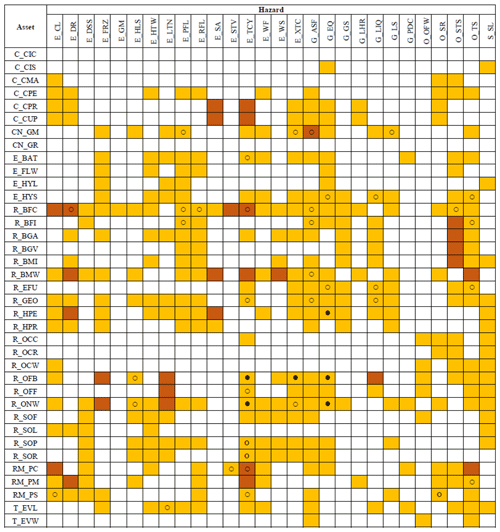
The intersections with the most significant concentration of quantitative studies are associated with the vulnerability of wind farms (both offshore and onshore) to extreme winds and earthquakes. Nonetheless, a closer look at these studies highlights a bias towards the study of specific components. Studies overwhelmingly focus on the vulnerability of the wind turbine towers (42 sources; e.g. Zhang et al., 2023), and significantly fewer studies focus on the substructure or foundations (17 sources; e.g. Ngo et al., 2023), blades (10 sources; e.g. Seo et al., 2022), or nacelles (10 sources; e.g. Rashid and Sarkar, 2022). Commonly, the fragility of the blades and nacelle is interpolated from the fragility of the tower. For the nacelle, only three sources (Cheng et al., 2023; Dueñas-Osorio and Basu, 2008; Hemmati et al., 2019) directly and empirically derive the fragility of acceleration-sensitive nacelle components, such as the generator, inverter, and control system.
The next intersection with the highest number of quantitative studies (18 sources; e.g. Schwanghart et al., 2018) is dominated by the seismic fragility assessment of concrete dams. Interestingly, this is the only intersection of hydropower assets with reservoirs where quantitative studies are present, despite the increasing exposure of these assets to other hazards. For example, the proportion of hydropower dams in basins with the highest levels of flood risk is projected to increase by nearly 20 times (e.g. from 2 % to 36 % of dams) between 2020 and 2050 (Opperman et al., 2022). However, literature for both pluvial and riverine flooding is scarce, with a single, qualitative study (Opperman et al., 2022) assessing the impact of flooding in general to dams, highlighting the importance of ageing.
Biofuel crops are the assets with the highest number of intersections with at least one quantitative study. This literature mainly focuses on the vulnerability of crops to flooding (i.e. riverine, pluvial flooding, and storm surge), drought, volcanic ash, and extreme wind. Most studies assess the vulnerability of agricultural crops, which could potentially be used for biofuel production, such as Kang et al. (2016), who estimated yield losses in rice and peanut crops at different flood depths, or Craig et al. (2021), who estimated the fragility of cereal crops to volcanic ash. Only a few studies have assessed the vulnerability of agricultural crops in general, without specifying crop type (e.g. Wang et al., 2022). Interestingly, no quantitative studies were found for the vulnerability of crops to hailstorms specifically, despite this intersection having one of the oldest insurance products (Randalls and Kneale, 2021).
As expected, natural assets and established engineered assets, which are predominant in the green economy, show a greater availability of quantitative literature. Despite this, the complexity of certain assets and the associated hazard processes can present significant barriers to research, even when a quantitative literature base exists. For example, structural components of wind turbines, such as the tower, have more fragility functions, because wind and seismic loads can be directly associated with the structure's limit states. In contrast, mechanical components within the nacelle, such as the gearbox, have limited literature on the composition of their multiple subcomponents and how these experience indirect loading (EPRI, 2021). Overall, the general lack of plentiful quantitative vulnerability studies about some or all the components of the examined assets exposed to most hazards highlights the need for expert evaluation of vulnerability from the interpretation of other available literature sources.
Given the large number of intersections, identifying trends in Tables 3 and 4 can be challenging. Therefore, assets are grouped into six subclasses of green assets, and a summary of their vulnerability ratings is provided in Table 5. Each asset is classified according to whether it is natural or engineered, with further subdivisions for natural assets into terrestrial and marine categories and engineered assets into established and emerging technologies, both onshore and offshore. In each subclass, only the intersections with hazards likely to affect its assets are considered. For example, the offshore wind farms are not exposed to snow avalanches or glacial melt.
A significant proportion (44 %) of natural asset vulnerability ratings were made with high confidence (CL1–2), compared to only 34 % for established and emerging engineered assets. With ecosystems, terrestrial assets tend to have a higher vulnerability to natural hazards (50 % assigned MHV) compared to marine-based assets (35 % assigned MHV). These evaluations are based on a significant amount of literature. For the engineered assets, the subclasses with the highest vulnerability (MHV) can be ranked as follows: onshore established (50 %), onshore emerging (33 %), offshore emerging (27 %), and offshore established (24 %). Emerging onshore engineered assets are also associated with the highest number of UV ratings (31 %).
Overall, the aggregated results highlight the general absence of relevant significant literature for the evaluation of natural hazard vulnerability of green economy assets and counter-intuitively highlight the scarcity of such literature for established (offshore and onshore) technologies.
Figures 2–4 depict the top-five hazards that dominate the MHV ratings for the assets in each subclass. The number of asset ratings is presented by subclass and differentiated by the green economy sector, with the proportion of these ratings assigned a confidence level of CL1–2 also being provided. It is highlighted that, for these dominant hazards, there is a significant amount of literature allowing high confidence in the vulnerability rating assignment.
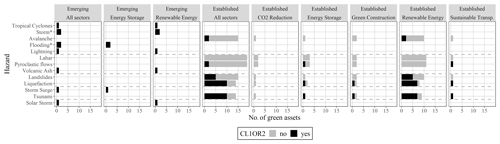
Figure 2Top hazards with MHV: engineered assets (onshore) separated by the green economy sector. * Flooding is a merger of riverine and pluvial flooding, whilst storm is a merger of ice–snow and hailstorm.
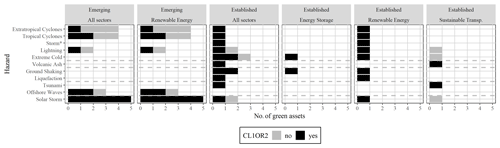
Figure 3Top hazards with MHV: engineered assets (offshore) separated by the green economy sector. * Storm is a merger of winter storm and hailstorm.
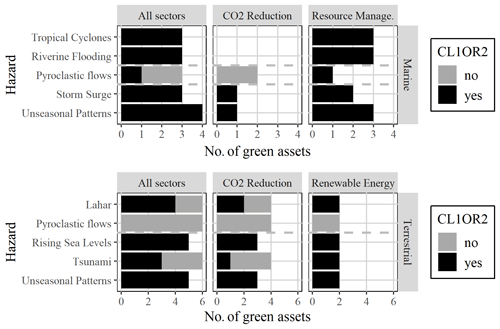
Figure 4Top hazards with MHV: natural assets (marine and terrestrial) separated by green economy sector.
It is observed that the dominant hazards vary across the considered subclasses and that there is no single hazard that is dominant for all considered engineered and natural green assets. Volcanic hazards significantly affect the vulnerability of most of the terrestrial ecosystems and engineered assets. Pyroclastic flows and lahar appear to be dominant for the vulnerability of established onshore assets and terrestrial ecosystems. By contrast, volcanic ash appears to affect the vulnerability of most emerging onshore and established offshore assets. Coastal hazards appear to affect most asset subclasses. Tsunami are found to be a major hazard for most terrestrial ecosystems and established assets. Coastal flooding also dominates the vulnerability of both marine ecosystems and onshore emerging assets. Severe storm events are shown to affect most marine ecosystems and all engineered classes except for the onshore established ones.
Some hazards appear to be significant only for assets in each class or subclass. For example, unseasonal patterns are found to affect most natural assets, while solar storms and lightning severely affect all engineered classes with the exception of the onshore established asset types. Landslides and avalanches severely affect only onshore established, engineered assets, while ground shaking and extreme cold severely affect only established offshore assets.
In what follows, a more in depth discussion is provided on the assignment of vulnerability ratings to green economy assets for four key hazard groups: volcanic hazards, severe storms, oceanic and coastal hazards, and space weather events and low-temperature hazards.
3.1 Discussion of green asset vulnerability to four significant hazard groups
3.1.1 Volcanic hazards
Among the three volcanic hazards assessed, hot, fast-moving pyroclastic flows and lahars are deemed the most mechanically destructive to infrastructure along their direct line of flow (Wardman et al., 2012b). Due to their destructive nature and geographic constraints, areas prone to these hazards generally see limited engineered or industrial construction. This is often enforced through restrictions in private and state insurance coverage, as seen by AXA Group. Consequently, this lack of exposure results in a scarcity of damage observations and research on terrestrial engineered assets in volcanic regions. Despite the limited availability of insurance products for these hazards (e.g. DCCA Hawaii Insurance Division, 2023), this study reasonably assesses vulnerability by drawing on literature related to other assets (e.g. Aspinall et al., 2011). If impacted, most terrestrial assets would be highly vulnerable to these hazards; thus they are assigned a high vulnerability rating (MHV). Field damage assessments are available for some nature-based assets, such as forests (e.g. De Guzman, 2005), mangroves (e.g. Joson et al., 2021), and crops (e.g. Dibyosaputro et al., 2015), allowing a stronger grounding for their vulnerability evaluation.
Airborne and falling ash ranks as the most geographically widespread volcanic hazard (Wardman et al., 2012b), which explains its top hazard ranking amongst two engineered asset types (established offshore and emerging onshore) supported by a robust literature base (≥ 50 % CL1–2). For example, observations of ash-induced damage on electricity infrastructure (necessary for electrified transportation) from substation flashover and on geothermal power station operation due to the abrasion of steam condenser fans have been observed in Japan (Nagai and Nakada, 2022) and Guatemala (Wardman et al., 2012a), respectively. Sea-water-based cooling systems for water-based vehicles are also susceptible to clogging by vesiculated ash, known as “pumice rafts”, which can lead to the overheating of onboard machinery (U.S. Geological Survey, 2015).
3.1.2 Severe storm events
Severe storm events are found amongst the top hazards for most engineered asset types, particularly those that are offshore and within the renewable energy sector. In most cases, the literature base for its MHV ratings is strong (≥ 50 % CL1–2).
Amongst all assets exposed to tropical and extratropical cyclones, offshore (bed-fixed) wind turbines have the highest number of quantitative studies (see Table 4). Despite this, assigning vulnerability to these assets is challenging. To ensure financial feasibility, wind turbines are designed with site-specific wind speeds and turbulence intensities, striking a balance between resistance to extreme wind conditions and optimal power output under normal operation (Larsén et al., 2022). Modern wind turbines are certified against the International Electrotechnical Commission (IEC) 61400-1 (IEC, 2019) design classes. However, existing vulnerability functions (e.g. Buchana and McSharry, 2019) are not based on turbine designs that are representative of this standard.
An interesting observation was made for floating photovoltaic (FPV) systems with regards to design standards. Hailstorms can cause the fracturing of the glass plate covering most PV modules, resulting in direct damage to the underlying PV material or long-term chemical or physical degradation of the internal components due to environmental exposure (Patt et al., 2013). According to the international qualification test IEC 61215-2:2021 (IEC, 2021), all terrestrial FPV panels operating in open-air climates must withstand a minimum of 11 impacts from a 25 mm hailstones at 23 m s−1. However, considering that the average maximum hail diameter in Europe is 50 mm (Patt et al., 2013), compliance with international standards does not necessarily imply low vulnerability.
3.1.3 Oceanic and coastal hazards
Except for the energy storage sector, at least one oceanic or coastal hazard is amongst the top hazards with MHV ratings. This is reflected across all engineered and natural asset types. Generally, the literature base for their vulnerability ratings is relevant and significant (≥ 50 % CL1–2).
The influence of extreme wave conditions produced by tropical or extratropical storms is considered in some of the literature for offshore floating and bed-fixed wind turbines (e.g. Utsunomiya et al., 2013). Floating wind turbines are seen to be more vulnerable to extreme wave conditions than bed-fixed wind turbines, as slight movements of the floating platform can lead to dramatic vibrations induced by the nacelle and moving blades (Li et al., 2022).
In terms of natural assets, Lagomasino et al. (2021) show that a combination of storm surge and poor drainage caused the highest dieback on record for mangroves in Florida, following Hurricane Irma in 2017. Meanwhile, literature at the intersection of tsunami and crops used for biofuel focuses more broadly on production constraints. For example the loss of farming equipment due to the 2009 Samoa tsunami exacerbated the loss of crops in affected areas (FAO, 2009).
3.1.4 Space weather and low-temperature hazards
Solar storms are the top MHV hazard for most engineered assets in the renewable energy sector. This hazard has the potential to disturb high-voltage electrical systems (e.g. transformers) (Radasky, 2011) and control systems (e.g. satellite communication or railway signalling) (Marusek, 2007). Despite being well supported by literature (> 50 % CL1–2), the number of literature sources for vulnerability evaluation is limited.
With a similarly strong literature base (> 50 % CL1–2), a broad range of offshore established, engineered assets (varying from energy storage to the sustainable transportation sectors) are seen to be vulnerable to extreme cold events. Li-ion batteries used in battery energy storage applications are susceptible to reduced ionic conductivity, increased lithium metal dendrite growth, and internal resistance, leading to higher temperature in the presence of a flammable electrolyte (Jeevarajan et al., 2022). Whilst water-based electric vehicles lacked a literature base, an MHV rating is still assigned considering the literature available for its land-based equivalent. A reduction in Li-ion battery performance under extreme cold conditions can reduce vehicle driving range, which is simultaneously affected by the increased battery power demands to maintain cabin temperature (Steinstraeter et al., 2021).
3.2 Research gaps
This paper highlights the need for an asset–hazard taxonomy tailored to the green economy. Adopting a standardized classification system across the insurance sector will enable the interoperability of functional loss data and analyses between researchers and insurers. In scenarios where exposure data are scarce, such a system will accelerate the development of quantitative fragility and vulnerability functions for complex green economy assets, facilitating their direct application within the insurance sector.
The taxonomy should adequately encompass both emerging and established technologies while also covering natural assets to facilitate the production of vulnerability and risk assessment products. Moreover, it is evident that duration must be explicitly considered in hazard definitions when assessing the vulnerability of natural assets. There is also a need for a species- and location-specific taxonomy for natural assets under sea level rise and flooding hazards, to ensure environmental conditions are appropriately factored into vulnerability assessments. Whilst this study assigns UV ratings to marshlands under sea level rise and storm surge, it was also noted that Simas et al. (2001) show that marshlands in the Tagus estuary (Portugal) are only susceptible to sea level rise in a worst-case scenario of 0.86 cm yr−1. Likewise, there is some evidence to suggest that more saline marshes have greater resistance to erosion under storm surge, due to deep rooting and lower soil shear strengths (FitzGerald and Hughes, 2019). The protection and restoration of forests, wetlands, and peatlands could sequester 9 Gt CO2 yr−1 by 2050 (Chaplin-Kramer et al., 2022), highlighting the urgent need for developing affordable, representative insurance products for natural assets to mitigate the impacts of climate change.
The vulnerability rating exercise conducted has highlighted a lack of literature available for the evaluation of vulnerability generally across all green economy assets and hazards. There is a clear lack of research that presents quantitatively the likely loss of function of green economy assets under natural hazards, in particular functions assessing indirect business interruption losses. The rapid growth in established, engineered assets such as utility-scale solar PV and onshore wind technologies underlines the importance addressing this gap. Both assets led the global deployment of renewable energy technologies in 2022, with nearly 90 % of them assessed to be more cost-effective than new fossil fuel alternatives (IRENA, 2023).
In the developed vulnerability matrix, there are a significant number of intersections with unknown vulnerability (UV) ratings where there is no literature available for the vulnerability evaluation of significant assets with known exposure to the considered hazards. Areas of concern include intersections between engineered (established onshore and emerging offshore), renewable energy assets with the hazards of sand storms, unseasonal weather patterns, and volcanic ash. These are seen to be significant hazards with UV ratings, with volcanic ash found consistently in the top hazard rankings of all other asset subclasses.
From an analysis of the literature for the established technologies of wind farms, it is observed that there is a need for quantitative literature on the blades and non-structural nacelle components, such as the gearbox. Failure of both of these components is currently the leading cause of wind farm insurance claims (Lloyd's, 2020). Despite offshore wind farm losses being dominated by subsea cable failures (Lloyd's, 2020; Allianz Commercial, 2023), no fragility or vulnerability functions were found for cables either. But even where literature exists, their practical applicability is limited. This study found that all 81 existing offshore (bed-fixed) wind turbine fragility functions under tropical cyclone conditions use the bespoke U.S. National Renewable Energy Laboratory (NREL) 5 MW (Jonkman et al., 2009) reference model, which does not comply with IEC 61400-1 (IEC, 2019). Moreover, the role of connecting infrastructure, such as substations, or the wider distribution and transmission system should be considered alongside these assets to understand functionality loss. Their fragilities can significantly contribute to the overall vulnerability of a wind farm. In the case of the two recorded instances of earthquake-related wind turbine damage since 1986, electricity grid failure induced the greatest losses due to downtime (DNV, 2019; Kübler et al., 2017).
As seen in the case of hydropower plants, engineered assets lack a strong literature base under flood hazards. Yet, as seen by AXA Group, there has been a significant increase in insurance claims in relation to hydropower plants impacted by flash floods, with smaller reservoir dam sizes showing a high vulnerability to these events. Assets with long expected lifetimes are in critical need of flood-related vulnerability assessments, as the design standards that they were initially designed to may not be relevant, especially in the context of climate change. Ageing hydropower assets must be prioritized amongst them, taking the highest (41 %) capacity share of global renewable energy sources (IRENA, 2023), the largest green economy sector of this study.
Whilst the quantitative literature base for crops used for biofuel was identified to be strong, there is a need for more relevant research which determines functional losses in terms of renewable energy production rather than agricultural yield loss.
To address the identified gaps, quantitative fragility and vulnerability functions can be developed using empirical models that rely on systematic observations of functional loss and its root causes, as well as a clear understanding of their link. When empirical data are limited, analytical methods may be employed as an alternative, provided numerical modelling is feasible. In cases where both empirical data and numerical modelling are unavailable, expert elicitation approaches can be used, as seen in the case of Ioannou et al. (2017), which quantified the vulnerability of reinforced concrete buildings to various fire intensities. Depending on data availability, a hybrid approach that combines empirical, analytical, and expert-based methods may also be utilized.
Assessing the vulnerability of green assets under natural hazards is a significant challenge for the insurance industry. As an emerging sector, the green economy lacks historical loss data, which traditional insurance models depend on. In such cases, insurers may use scenarios analyses based on a combination of published engineering models and internal expert judgement. In this context, the study assessed the potential of using existing published literature to support the development of these models. The limited exposure data for complex green economy assets, as seen in the insurance sector; the insufficient alignment of published vulnerability assessments with design standards and insurance needs; and the increasing intensity of hazards due to climate change have all contributed to the difficulty in establishing credible vulnerability ratings through existing research. This paper highlights the critical need for a representative green economy asset–hazard taxonomy, which is essential for guiding researchers in developing quantitative vulnerability assessments that are relevant to the insurance industry.
Future work
In this section, recommendations for research and insurance industry practitioners are made, with the intention that outputs from these stakeholders will guide policymakers in changing codes of practice for the protection of green economy assets. For the insurance sector specifically, practically applicable, quantitative vulnerability models are needed to reduce uncertainty in pricing insurance premiums. More broadly, such models will improve the industry's internal risk monitoring efforts and help confidently achieve regulatory requirements. In turn, this will help de-risk investment in the growing green economy and improve its resilience to natural hazards. To support this, the operational asset–hazard taxonomy proposed in this study is in need of expansion and standardization with inputs from the wider insurance industry.
Future vulnerability assessments conducted by researchers must consider realistic asset designs and the interaction interplay of components within green economy systems. This includes the impact of common mitigation technologies and adaptation measures on assets assessed as high vulnerability. It is also important to consider the compounding effects of multi-hazard events and the cascading impacts of dependent asset failures. In doing this, factors such as the frequency, intensity, and duration of hazard events must be accounted for. For instance, vulnerability may increase for engineered assets as fatigue accumulates over time but decrease for natural assets if the return period allows for regeneration.
In the short term, future quantitative assessments by researchers should prioritize established engineered assets that are experiencing rapid market growth, particularly solar PV and onshore wind installations. While these assets incorporate site conditions in their design standards, lower initial investment costs and the decreasing availability of optimal locations may result in reduced due diligence during the planning stage, shifting greater risk to insurers. Additionally, with the changing climate, it is crucial to prioritize ageing hydropower assets in research efforts due to their extensive global presence and the potential risks associated with their long-term operation. Simultaneously, natural assets urgently require the development of vulnerability models that are tailored to specific locations and species, ensuring their capacity to mitigate the impacts of climate change effectively.
Table A1Asset taxonomy: code and definitions. These are classified by green economy sector (SEC): CO2 reduction (C), green construction (CN), energy storage (E), renewable energy sources (R), natural resource management (RM), and sustainable transportation (ST). They are also classified by asset type (AT): natural (NAT) or engineered (ENG), established (ES) or emerging (EM), and environment (ENV), i.e. onshore (ONS), offshore (OFF), terrestrial (TER), or marine (MAR).

Tables 3, 4, and 5 were generated using Microsoft Excel and Word. Figures 2, 3, and 4 were generated using R and Microsoft Excel. The code and files used to create these figures and tables can be made available upon specific requests made to the corresponding author, with approval by AXA Group.
The list of literature sources that has been adopted for the literature heat map can be made available upon specific requests made to the corresponding author, with approval by AXA Group.
HB, II, TR, JC, and MJ conceptualized the research project and developed the methodology. HB, II, TR, and JC conducted the systematic literature review, before curating, analysing, and visualizing the literature metadata. Within this, MJ, NM, and AFS assisted with defining the green economy assets and natural hazards. HB, II, TR, JC, MJ, and CS prepared the original draft of the published work. HB, II, TR, JC, MJ, NM, AFS, and CS reviewed and provided editorial assistance on the original manuscript draft.
During development and preparation of this publication, Mathis Joffrain, Nicolas Mortegoutte, and Aurelie Fallon Saint-Lo were employed at AXA Group. Harikesan Baskaran's funding received industry sponsorship from AXA Group. The remaining authors declare that the research was conducted in the absence of any commercial or financial relationship that can be construed as a potential conflict of interest.
Publisher’s note: Copernicus Publications remains neutral with regard to jurisdictional claims made in the text, published maps, institutional affiliations, or any other geographical representation in this paper. While Copernicus Publications makes every effort to include appropriate place names, the final responsibility lies with the authors.
Harikesan Baskaran received funding from the UK Engineering and Physical Sciences Research Council (EPSRC) doctoral training partnership (DTP) (grant no. EP/W524335/1), with an industry sponsorship from AXA Group.
This research has been supported by the UK Engineering and Physical Sciences Research Council (EPSRC) (grant no. EP/W524335/1) and AXA Group.
This paper was edited by Robert Sakic Trogrlic and reviewed by two anonymous referees.
Abed, J., Rayburg, S., Rodwell, J., and Neave, M.: A Review of the Performance and Benefits of Mass Timber as an Alternative to Concrete and Steel for Improving the Sustainability of Structures, Sustainability, 14, 5570, https://doi.org/10.3390/su14095570, 2022.
Adderly, S. A., Manukian, D., Sullivan, T. D., and Son, M.: Electric vehicles and natural disaster policy implications, Energ. Policy, 112, 437–448, https://doi.org/10.1016/J.ENPOL.2017.09.030, 2018.
AIG Energy Industry Group: Lithium-ion Battery Energy Storage Systems, American International Group, 9 pp., GBL00002513 1/18, 2018.
Al-Hemoud, A., Al-Dousari, A., Misak, R., Al-Sudairawi, M., Naseeb, A., Al-Dashti, H., and Al-Dousari, N.: Economic Impact and Risk Assessment of Sand and Dust Storms (SDS) on the Oil and Gas Industry in Kuwait, Sustainability, 11, 200, https://doi.org/10.3390/SU11010200, 2019.
Allianz Commercial: A turning point for offshore wind: Global opportunities and risk trends, https://www.allianz.com/content/dam/onemarketing/azcom/Allianz_com/press/document/Allianz-Commercial_A-turning-point-for-offshore-wind.pdf, last access: 25 September 2023.
Allianz Global Corporate & Specialty: Global Industry Solutions Outlook Q&A: Construction, https://commercial.allianz.com/content/dam/onemarketing/commercial/commercial/reports/global-industry-solution-outlook-2023-construction.pdf, last access: 28 July 2023.
Alshehri, A., Dunn, C., Freeman, C., Hugron, S., Jones, T. G., and Rochefort, L.: A Potential Approach for Enhancing Carbon Sequestration During Peatland Restoration Using Low-Cost, Phenolic-Rich Biomass Supplements, Front. Environ. Sci., 8, 48, https://doi.org/10.3389/FENVS.2020.00048, 2020.
Alvarez, R. and Lidén, G.: The effect of temperature variation on biomethanation at high altitude, Bioresour. Technol., 99, 7278–7284, https://doi.org/10.1016/j.biortech.2007.12.055, 2008.
Artés, T., Oom, D., de Rigo, D., Durrant, T. H., Maianti, P., Libertà, G., and San-Miguel-Ayanz, J.: A global wildfire dataset for the analysis of fire regimes and fire behaviour, Scientific Data, 6, 296, 1–11, https://doi.org/10.1038/s41597-019-0312-2, 2019.
Aspinall, W., Auker, M., Hincks, T., Mahony, S., Nadim, F., Pooley, J., Sparks, S., and Syre, E.: GFDRR, Volcano Risk Study – Volcano Hazard and Exposure in GFDRR Priority Countries and Risk Mitigation Measures, NGI, Norway, 309 pp., 20100806-00-1-R, 2011.
Atkins: Deep Geothermal Review Study Final Report, https://assets.publishing.service.gov.uk/government/uploads/system/uploads/attachment_data/file/251943/Deep_Geothermal_Review_Study_Final_Report_Final.pdf (last access: 17 November 2022), 2013.
Australian Bureau of Meteorology: Storm Surge, http://www.bom.gov.au/cyclone/tropical-cyclone-knowledge-centre/understanding/storm-surge/, last access: 19 December 2022.
Beck, M. W., Heck, N., Narayan, S., Menéndez, P., Torres-Ortega, S., Losada, I. J., Way, M., Rogers, M., and McFarlane-Connelly, L.: Reducing Caribbean Risk: Opportunities for Cost-Effective Mangrove Restoration and Insurance, Arlington, VA, https://www.axa.com/en/press/publications/AXA-XL-Mangrove-Insurance-Report (last access: 17 July 2023), 2020.
Biskaborn, B. K., Smith, S. L., Noetzli, J., Matthes, H., Vieira, G., Streletskiy, D. A., Schoeneich, P., Romanovsky, V. E., Lewkowicz, A. G., Abramov, A., Allard, M., Boike, J., Cable, W. L., Christiansen, H. H., Delaloye, R., Diekmann, B., Drozdov, D., Etzelmüller, B., Grosse, G., Guglielmin, M., Ingeman-Nielsen, T., Isaksen, K., Ishikawa, M., Johansson, M., Johannsson, H., Joo, A., Kaverin, D., Kholodov, A., Konstantinov, P., Kröger, T., Lambiel, C., Lanckman, J. P., Luo, D., Malkova, G., Meiklejohn, I., Moskalenko, N., Oliva, M., Phillips, M., Ramos, M., Sannel, A. B. K., Sergeev, D., Seybold, C., Skryabin, P., Vasiliev, A., Wu, Q., Yoshikawa, K., Zheleznyak, M., and Lantuit, H.: Permafrost is warming at a global scale, Nat. Commun., 10, 264, https://doi.org/10.1038/s41467-018-08240-4, 2019.
Brás, T. A., Seixas, J., Carvalhais, N., and Jägermeyr, J.: Severity of drought and heatwave crop losses tripled over the last five decades in Europe, Environ. Res. Lett., 16, 065012, https://doi.org/10.1088/1748-9326/abf004, 2021.
Breeze, P.: Hydrogen Energy Storage, in: Power System Energy Storage Technologies, Elsevier, 69–77, https://doi.org/10.1016/B978-0-12-812902-9.00008-0, 2018.
Brimicombe, C., Porter, J. J., Di Napoli, C., Pappenberger, F., Cornforth, R., Petty, C., and Cloke, H. L.: Heatwaves: An invisible risk in UK policy and research, Environ. Sci. Policy, 116, 1–7, https://doi.org/10.1016/J.ENVSCI.2020.10.021, 2021.
British Geological Survey: Earthquakes, https://www.bgs.ac.uk/discovering-geology/earth-hazards/earthquakes/, last access: 19 December 2022a.
British Geological Survey: Volcanic hazards, https://www.bgs.ac.uk/discovering-geology/earth-hazards/volcanoes/volcanic-hazards/, last access: 19 December 2022b.
Brockway, A. M. and Dunn, L. N.: Weathering adaptation: Grid infrastructure planning in a changing climate, Clim. Risk Manag., 30, 100256, https://doi.org/10.1016/J.CRM.2020.100256, 2020.
Buchana, P. and McSharry, P. E.: Windstorm risk assessment for offshore wind farms in the North Sea, Wind Energy, 22, 1219–1229, https://doi.org/10.1002/WE.2351, 2019.
Bullock, J. A., Haddow, G. D., and Coppola, D. P.: Hazards, in: Introduction to Homeland Security, Butterworth-Heinemann, 57–95, https://doi.org/10.1016/B978-0-12-415802-3.00003-8, 2013
Burt, J. A.: The environmental costs of coastal urbanization in the Arabian Gulf, Analysis of Urban Change, Theory, Action, 18, 760–770, https://doi.org/10.1080/13604813.2014.962889, 2014.
Chaplin-Kramer, R., Neugarten, R. A., Sharp, R. P., Collins, P. M., Polasky, S., Hole, D., Schuster, R., Strimas-Mackey, M., Mulligan, M., Brandon, C., Diaz, S., Fluet-Chouinard, E., Gorenflo, L. J., Johnson, J. A., Kennedy, C. M., Keys, P. W., Longley-Wood, K., McIntyre, P. B., Noon, M., Pascual, U., Reidy Liermann, C., Roehrdanz, P. R., Schmidt-Traub, G., Shaw, M. R., Spalding, M., Turner, W. R., van Soesbergen, A., and Watson, R. A.: Mapping the planet's critical natural assets, Nat. Ecol. Evol., 7, 51–61, https://doi.org/10.1038/s41559-022-01934-5, 2022.
Chatterjee, M., Wörle, J., U, N. O., Zhuang, M., and Gu, R.: Gearing up for the electric vehicles ecosystem: Risks along the value chain – Part I, Swiss Re Management Ltd, Zurich, https://www.swissre.com/dam/jcr:af04aa03-0d21-4b8d-afd2-8a2f2f9f614e/2023-01-sri-electric-vehicles-ecosystem-risks-value-chain-part-1.pdf (last access: 10 December 2024), 2023.
Cheng, Y., Luo, Y., Wang, J., Dai, K., Wang, W., and El Damatty, A.: Fragility and vulnerability development of offshore wind turbines under aero-hydro loadings, Eng. Struct., 293, 116625, https://doi.org/10.1016/J.ENGSTRUCT.2023.116625, 2023.
Cloke, H., Di Baldassarre, G., Landeg, O., Pappenberger, F., and Ramos, M. H.: Hydrological risk: floods, in: Science for disaster risk management 2017: knowing better and losing less, Publications Office of the European Union, Luxembourg, 209–238, https://doi.org/10.2788/688605, 2017.
Converti, A., Oliveira, R. P. S., Torres, B. R., Lodi, A., and Zilli, M.: Biogas production and valorization by means of a two-step biological process, Bioresour. Technol., 100, 5771–5776, https://doi.org/10.1016/J.BIORTECH.2009.05.072, 2009.
Coral Reef Alliance: Types of Coral Reefs, https://coral.org/en/coral-reefs-101/types-of-coral-reef-formations/, last access: 18 March 2024.
Craig, H., Wilson, T., Magill, C., Stewart, C., and Wild, A. J.: Agriculture and forestry impact assessment for tephra fall hazard, Volcanica, 4, 345–367, https://doi.org/10.30909/vol.04.02.345367, 2021.
Danielsen, F., Sørensen, M. K., Olwig, M. F., Selvam, V., Parish, F., Burgess, N. D., Hiraishi, T., Karunagaran, V. M., Rasmussen, M. S., Hansen, L. B., Quarto, A., and Suryadiputra, N.: The Asian tsunami: A protective role for coastal vegetation, Science, 310, 643, https://doi.org/10.1126/SCIENCE.1118387, 2005.
DCCA Hawaii Insurance Division: HOMEOWNERS INSURANCE – Town Hall Meeting with Sen. San Buenaventura and Rep. Ilagan, https://cca.hawaii.gov/ins/files/2023/08/Pahoa-Community-8-23-23.pdf (last access: 31 July 2024), 2023.
De'Ath, G., Fabricius, K. E., Sweatman, H., and Puotinen, M.: The 27-year decline of coral cover on the Great Barrier Reef and its causes, P. Natl. Acad. Sci. USA, 109, 17995–17999, https://doi.org/10.1073/PNAS.1208909109, 2012.
Dibyosaputro, S., Dipayana, G. A., Nugraha, H., Pratiwi, K., and Valeda, H. P.: Lahar at Kali Konto after the 2014 Eruption of Kelud Volcano, East Java: Impacts and Risk, Forum Geografi, 29, 59–72, https://doi.org/10.23917/forgeo.v29i1.793, 2015
DNV: How do recent earthquakes events impact wind turbines?, https://www.dnv.com/article/how-do-recent-earthquakes-events-impact-wind-turbines--179350# (last access: 19 July 2023), 2019.
Dueñas-Osorio, L. and Basu, B.: Unavailability of wind turbines due to wind-induced accelerations, Eng. Struct., 30, 885–893, https://doi.org/10.1016/j.engstruct.2007.05.015, 2008.
EPRI: Wind Turbine Gearbox Reliability Assessment: Value of Increased Reliability and Reduced Operations and Maintenance Costs, https://www.epri.com/research/products/000000003002021422 (last access: 1 August 2024), 2021.
European Commission: Systemic Seismic Vulnerability and Risk Analysis for Buildings, Lifeline Networks and Infrastructures Safety Gain, https://cordis.europa.eu/project/id/244061 (last access: 6 November 2024), 2019.
European Marine Energy Centre Ltd: Wave devices, https://www.emec.org.uk/marine-energy/wave-devices/, last access: 4 November 2022.
FAO: After the tsunami, family food security in Samoa extremely fragile, https://reliefweb.int/report/samoa/after-tsunami-family-food-security-samoa-extremely-fragile (last access: 10 December 2024), 2009.
FitzGerald, D. M. and Hughes, Z.: Marsh Processes and Their Response to Climate Change and Sea-Level Rise, Annu. Rev. Earth Pl. Sc., 47, 481–517, https://doi.org/10.1146/annurev-earth-082517-010255, 2019.
FitzGerald, D. M., Fenster, M. S., Argow, B. A., and Buynevich, I. V.: Coastal Impacts Due to Sea-Level Rise, Annu. Rev. Earth Pl. Sc., 36, 601–647, https://doi.org/10.1146/annurev.earth.35.031306.140139, 2008.
Fragoso Ados Santos, H., Duarte, G. A. S., Rachid, C. T. D. C., Chaloub, R. M., Calderon, E. N., Marangoni, L. F. D. B., Bianchini, A., Nudi, A. H., Do Carmo, F. L., Van Elsas, J. D., Rosado, A. S., Castro, C. B. E., and Peixoto, R. S.: Impact of oil spills on coral reefs can be reduced by bioremediation using probiotic microbiota, Sci. Rep., 5, 18268, https://doi.org/10.1038/srep18268, 2015.
Frame, T., Harrison, G., Hewson, T., and Roberts, N.: Meteorological risk: extra-tropical cyclones, tropical cyclones and convective storms, in: Science for disaster risk management 2017: knowing better and losing less, Publications Office of the European Union, Luxembourg, 246–335, https://doi.org/10.2788/688605, 2017.
Friess, D. A., Rogers, K., Lovelock, C. E., Krauss, K. W., Hamilton, S. E., Lee, S. Y., Lucas, R., Primavera, J., Rajkaran, A., and Shi, S.: The State of the World's Mangrove Forests: Past, Present, and Future, 44, 89–115, https://doi.org/10.1146/annurev-environ-101718-033302, 2019.
FTSE Russell: Investing in the green economy 2023, https://www.lseg.com/content/dam/ftse-russell/en_us/documents/research/investing-in-the-green-economy-2023.pdf (last access: 1 August 2024), 2023.
Gamba, P., Cavalca, D., Jaiswal, K. S., Huyck, C., and Crowley, H.: The GED4GEM Project: Development of a Global Database for the Global Earthquake Model Initiative, in: Proceedings of the 15th World Conference on Earthquake Engineering, Lisbon, Portugal, 24–29 September 2012, https://www.iitk.ac.in/nicee/wcee/article/WCEE2012_3240.pdf (last access: 21 December 2024), 2012.
Gariano, S. L. and Guzzetti, F.: Landslides in a changing climate, Earth Sci. Rev., 162, 227–252, https://doi.org/10.1016/J.EARSCIREV.2016.08.011, 2016.
GCube Underwriting: Hail or High Water: The rising scale of Extreme Weather and Natural Catastrophe losses in Renewable Energy, London, United Kingdom, 46 pp., Q1 2021 Report, https://gcube-insurance.com/Insights/Reports/HAIL-OR-HIGH-WATER (last access: 21 December 2024), 2021.
Giles, T. M., Newnham, R. M., Lowe, D. J., and Munro, A. J.: Impact of tephra fall and environmental change: a 1000 year record from Matakana Island, Bay of Plenty, North Island, New Zealand, Geological Society, London, Special Publications, 161, 11–26, https://doi.org/10.1144/GSL.SP.1999.161.01.03, 1999.
Giri, C., Ochieng, E., Tieszen, L. L., Zhu, Z., Singh, A., Loveland, T., Masek, J., and Duke, N.: Status and distribution of mangrove forests of the world using earth observation satellite data, Global Ecol. Biogeogr., 20, 154–159, https://doi.org/10.1111/J.1466-8238.2010.00584.X, 2011.
De Guzman, E. M.: Eruption of Mount Pinatubo in the Philippines in June 1991, https://www.adrc.asia/publications/recovery_reports/pdf/Pinatubo.pdf (last access: 14 August 2023), 2005.
Hajtmanek, R., Morgenstein, P., Hubinský, T., Legény, J., and Špaček, R.: Determination of Solar-Surface-Area-to-Volume Ratio: Early Design Stage Solar Performance Assessment of Buildings, Buildings, 13, 296, https://doi.org/10.3390/buildings13020296, 2023.
Hall, T. and Booth, J. F.: SynthETC: A Statistical Model for Severe Winter Storm Hazard on Eastern North America, J. Climate, 30, 5329–5343, https://doi.org/10.1175/JCLI-D-16-0711.1, 2017.
Hänggi, S., Elbert, P., Bütler, T., Cabalzar, U., Teske, S., Bach, C., and Onder, C.: A review of synthetic fuels for passenger vehicles, Energy Reports, 5, 555–569, https://doi.org/10.1016/J.EGYR.2019.04.007, 2019.
Hemmati, A., Oterkus, E., and Barltrop, N.: Fragility reduction of offshore wind turbines using tuned liquid column dampers, Soil Dyn. Earthqu. Eng., 125, 105705, https://doi.org/10.1016/j.soildyn.2019.105705, 2019.
Hjort, J., Karjalainen, O., Aalto, J., Westermann, S., Romanovsky, V. E., Nelson, F. E., Etzelmüller, B., and Luoto, M.: Degrading permafrost puts Arctic infrastructure at risk by mid-century, Nat. Commun., 9, 5147, https://doi.org/10.1038/s41467-018-07557-4, 2018.
Huang, Y. and Yu, M.: Review of soil liquefaction characteristics during major earthquakes of the twenty-first century, Nat. Hazards, 65, 2375–2384, https://doi.org/10.1007/S11069-012-0433-9, 2013.
IEA: Global EV Outlook, Paris, https://iea.blob.core.windows.net/assets/dacf14d2-eabc-498a-8263-9f97fd5dc327/GEVO2023.pdf, last access: 28 July 2023.
IEC: Wind energy generation systems – Part 1: Design requirements, 4th edn., IEC 61400-1:2019, https://webstore.iec.ch/en/publication/26423 (last access: 10 December 2024), 2019.
IEC: Terrestrial photovoltaic (PV) modules – Design qualification and type approval – Part 2: Test procedures, 2nd edn., IEC 61215-2:2021, https://webstore.iec.ch/en/publication/61350 (last access: 10 December 2024), 2021.
IFC: Green Buildings: A Financial and Policy Blueprint for Emerging Markets, Washington, D.C., https://documents1.worldbank.org/curated/fr/58684157652333 0833/pdf/Green-Buildings-A-Finance-and-Policy-Blueprint-for-Emerging-Markets.pdf (last access: 10 December 2024), 2019.
Ioannou, I., Aspinall, W., Rush, D., Bisby, L., and Rossetto, T.: Expert judgment-based fragility assessment of reinforced concrete buildings exposed to fire, Reliab. Eng. Syst. Saf., 167, 105–127, https://doi.org/10.1016/j.ress.2017.05.011, 2017.
IRENA: Renewable power generation costs in 2022, Abu Dhabi, https://www.irena.org/Publications/2023/Aug/Renewable-Power-Generation-Costs-in-2022, last access: 1 December 2023.
International Federation of Red Cross and Red Crescent Societies (IFRC): Cold waves, https://www.ifrc.org/our-work/disasters-climate-and-crises/what-disaster/cold-waves, last access: 19 December 2022.
Jeevarajan, J. A., Joshi, T., Parhizi, M., Rauhala, T., and Juarez-Robles, D.: Battery Hazards for Large Energy Storage Systems, ACS Energy Lett., 7, 2725–2733, https://doi.org/10.1021/acsenergylett.2c01400, 2022.
Jeong, B., Jang, H., Lee, W., Park, C., Ha, S., Kim, D. K., and Cho, N. K.: Is electric battery propulsion for ships truly the lifecycle energy solution for marine environmental protection as a whole?, J. Clean. Prod., 355, 131756, https://doi.org/10.1016/J.JCLEPRO.2022.131756, 2022.
Jiménez, P. A., De Arellano, J. V. G., Gonzá Lez-Rouco, J. F., Navarro, J., Montá Vez, J. P., García-Bustamante, E., and Dudhia, J.: The Effect of Heat Waves and Drought on Surface Wind Circulations in the Northeast of the Iberian Peninsula during the Summer of 2003, J. Climate, 24, 5416–5422, https://doi.org/10.1175/2011JCLI4061.1, 2011.
Jonkman, J., Butterfield, S., Musial, W., and Scott, G.: Definition of a 5-MW Reference Wind Turbine for Offshore System Development, National Renewable Energy Laboratory, Golden, CO, https://doi.org/10.2172/947422, 2009.
Joson, M. P., Comia, M. J., Klarice Cureg, M., Navarrete, I. A., and Salmo III, S. G.: Comparative Assessment of Natural, Colonized and Planted Mangroves Disturbed by Lahar Deposition in Sasmuan, Pampanga, Philippines, Science & Engineering Journal (SciEnggJ), 14, 200–211, https://scienggj.org/2021-200/ (last access: 10 December 2024), 2021.
Kang, L., Ma, L., and Liu, Y.: Evaluation of farmland losses from sea level rise and storm surges in the Pearl River Delta region under global climate change, J. Geogr. Sci., 26, 439–456, https://doi.org/10.1007/s11442-016-1278-z, 2016.
Kapoor, R., Ghosh, P., Tyagi, B., Vijay, V. K., Vijay, V., Thakur, I. S., Kamyab, H., Nguyen, D. D., and Kumar, A.: Advances in biogas valorization and utilization systems: A comprehensive review, J. Clean. Prod., 273, 123052, https://doi.org/10.1016/J.JCLEPRO.2020.123052, 2020.
Kellner, E.: Wetlands – different types, their properties and functions, https://skb.se/ (last access: 4 November 2022), 2003.
Khan, N., Kalair, A., Abas, N., and Haider, A.: Review of ocean tidal, wave and thermal energy technologies, Renew. Sust. Energ. Rev., 72, 590–604, https://doi.org/10.1016/J.RSER.2017.01.079, 2017.
King's Printer of Acts of Parliament: The Climate Change Act 2008 (2050 Target Amendment) Order 2019, UK Statutory Instrument: 2019 No. 1056, https://www.legislation.gov.uk/uksi/2019/1056/contents/made (last access: 7 May 2024), 2019.
Kübler, O., Renggli, D., Meyer, S., and Benz, G.: Wind farms: harvesting energy on shaky grounds and in stormy seas, Swiss Re Management Ltd, Zurich, https://www.swissre.com/dam/jcr:dd24316a-aab5-446a-9758-7d58215e24df/Windfarms+Harvesting+energy+on+shaky+grounds+EN.pdf (last access: 10 December 2024), 2017.
Lagomasino, D., Fatoyinbo, T., Castañeda-Moya, E., Cook, B. D., Montesano, P. M., Neigh, C. S. R., Corp, L. A., Ott, L. E., Chavez, S., and Morton, D. C.: Storm surge and ponding explain mangrove dieback in southwest Florida following Hurricane Irma, Nat. Commun., 12, 4003, https://doi.org/10.1038/s41467-021-24253-y, 2021.
Larsén, X. G., Davis, N., Hannesdóttir, Á., Kelly, M., Svenningsen, L., Slot, R., Imberger, M., Olsen, B. T., and Floors, R.: The Global Atlas for Siting Parameters project: Extreme wind, turbulence, and turbine classes, Wind Energy, 25, 1841–1859, https://doi.org/10.1002/we.2771, 2022.
Li, J., Li, Z., Jiang, Y., and Tang, Y.: Typhoon Resistance Analysis of Offshore Wind Turbines: A Review, Atmosphere-Basel, 13, 451, https://doi.org/10.3390/atmos13030451, 2022.
Li, X. and Palazzolo, A.: A review of flywheel energy storage systems: state of the art and opportunities, J. Energy Storage, 46, 103576, https://doi.org/10.1016/j.est.2021.103576, 2022.
Lin, L. and Adams, J.: LESSONS FOR THE FRAGILITY OF CANADIAN HYDROPOWER COMPONENTS UNDER SEISMIC LOADING, Ninth Canadian Conference on Earthquake Engineering, Ottawa, Ontario, Canada, 26–29 June 2007, 1762–1771, https://www.earthquakescanada.nrcan.gc.ca/hazard-alea/2007/9CCEE/9CCEE_Lin_Adams_p1186.pdf (last access: 6 December 2024), 2007.
Lloyd's: Renewable energy risk and reward: Risks and technologies, https://assets.lloyds.com/assets/pdf-renewable-energy-risk-and-reward-renenergy-risksandtechnologies/1/pdf-renewable-energy-risk-and-reward-RenEnergy_RisksandTechnologies.pdf (last access: 4 July 2023), 2020.
London Energy Transformation Initiative (LETI): LETI Climate Emergency Retrofit Guide, https://www.leti.uk/_files/ugd/252d09_c71428bafc3d42fbac34f9ad0cd6262b.pdf (last access: 18 March 2024), 2021.
Louchet, F.: Snow Avalanches: Beliefs, Facts, and Science, Oxford University Press, https://doi.org/10.1093/OSO/9780198866930.003.0001, 2020.
Måren, I. E. and Sharma, L. N.: Seeing the wood for the trees: Carbon storage and conservation in temperate forests of the Himalayas, Forest Ecol. Manage., 487, 119010, https://doi.org/10.1016/J.FORECO.2021.119010, 2021.
Marker, B. R.: Land subsidence, Encyclopedia of Earth Sciences Series, 583–590, https://doi.org/10.1007/978-1-4020-4399-4_208, 2013.
Marusek, J. A.: Solar Storm Threat Analysis, Impact, Bloomfield, Indiana, 29 pp., http://www.breadandbutterscience.com/SSTA.pdf (last access: 21 December 2024), 2007.
Marzouk, O. A.: Land-Use competitiveness of photovoltaic and concentrated solar power technologies near the Tropic of Cancer, Sol. Energy, 243, 103–119, https://doi.org/10.1016/j.solener.2022.07.051, 2022.
Mathew, S. and Philip, G. S.: Wind Turbines: Evolution, Basic Principles, and Classifications, in: Comprehensive Renewable Energy, Elsevier, 104–123, https://doi.org/10.1016/B978-0-12-819727-1.00187-4, 2012.
Mazda, Y., Kobashi, D., and Okada, S.: Tidal-Scale Hydrodynamics within Mangrove Swamps, Wetl. Ecol. Manag., 13, 647–655, https://doi.org/10.1007/S11273-005-0613-4, 2005.
McKinsey: Capturing the climate opportunity in insurance, https://www.mckinsey.com/industries/financial-services/our-insights/capturing-the-climate-opportunity-in-insurance (last access: 14 July 2023), 2022.
Met Office: Tropical cyclone facts, https://www.metoffice.gov.uk/research/weather/tropical-cyclones/facts, last access: 19 December 2022.
Moelven: Mjøstårnet, https://www.moelven.com/mjostarnet/, last access: 18 March 2024.
Moyo, I. and Xulu, S.: Public Knowledge, Perceptions and Practices in the High-Risk Lightning Zone of South Africa, Int. J. Env. Res. Pub. He., 18, 7448, https://doi.org/10.3390/IJERPH18147448, 2021.
Nagai, M. and Nakada, S.: Impact on the Electric Infrastructure Due to Volcanic Ash from a Hydrovolcanic Eruption of Aso Volcano in 2016, Journal of Disaster Research, 17, 829–838, https://doi.org/10.20965/JDR.2022.P0829, 2022.
NASA: Sea Level, https://climate.nasa.gov/vital-signs/sea-level/, last access: 19 December 2022.
Nature Communications: Financing a greener future, Nat. Commun., 14, 533, https://doi.org/10.1038/s41467-023-36036-8, 2023.
New Zealand National Institute of Water and Atmospheric Research: Waves, https://niwa.co.nz/natural-hazards/hazards/waves, last access: 19 December 2022.
Ngo, D.-V., Kim, Y.-J., and Kim, D.-H.: Risk Assessment of Offshore Wind Turbines Suction Bucket Foundation Subject to Multi-Hazard Events, Energies-Basel, 16, 2184, https://doi.org/10.3390/en16052184, 2023.
Opperman, J. J., Camargo, R. R., Laporte-Bisquit, A., Zarfl, C., and Morgan, A. J.: Using the WWF Water Risk Filter to Screen Existing and Projected Hydropower Projects for Climate and Biodiversity Risks, Water-Basel, 14, 721, https://doi.org/10.3390/w14050721, 2022.
Patt, A., Pfenninger, S., and Lilliestam, J.: Vulnerability of solar energy infrastructure and output to climate change, Climatic Change, 121, 93–102, https://doi.org/10.1007/s10584-013-0887-0, 2013.
Pham, C. H., Vu, C. C., Sommer, S. G., and Bruun, S.: Factors Affecting Process Temperature and Biogas Production in Small-scale Rural Biogas Digesters in Winter in Northern Vietnam, Asian-Australas J. Anim. Sci., 27, 1050–1056, https://doi.org/10.5713/ajas.2013.13534, 2014.
Punge, H. J. and Kunz, M.: Hail observations and hailstorm characteristics in Europe: A review, Atmos. Res., 176–177, 159–184, https://doi.org/10.1016/J.ATMOSRES.2016.02.012, 2016.
Radasky, W. A.: Overview of the impact of intense geomagnetic storms on the U.S. high voltage power grid, in: 2011 IEEE International Symposium on Electromagnetic Compatibility, Long Beach, CA, USA, 14–19 August 2011, 300–305, https://doi.org/10.1109/ISEMC.2011.6038326, 2011.
Randalls, S. and Kneale, J.: A Fragile Network: Effecting Hail Insurance in Britain, 1840–1900, Enterp. Soc., 22, 739–769, https://doi.org/10.1017/eso.2020.19, 2021.
Rashid, H. and Sarkar, R.: Site-specific response of a 5 MW offshore wind turbine for Gujarat Coast of India, Mar. Georesour. Geotec., 40, 1119–1138, https://doi.org/10.1080/1064119X.2021.1972062, 2022.
RDLS: Schema Reference, https://docs.riskdatalibrary.org/en/latest/reference/schema/, last access: 10 December 2024.
Reed, D. J.: The response of coastal marshes to sea-level rise: Survival or submergence?, Earth Surf. Proc. Land., 20, 39–48, https://doi.org/10.1002/esp.3290200105, 1995.
Richardson, S. D. and Reynolds, J. M.: An overview of glacial hazards in the Himalayas, Quatern. Int., 65–66, 31–47, https://doi.org/10.1016/S1040-6182(99)00035-X, 2000.
Rose, A. and Huyck, C. K.: Improving Catastrophe Modeling for Business Interruption Insurance Needs, Risk Anal., 36, 1896–1915, https://doi.org/10.1111/risa.12550, 2016.
Sale, P. F., Feary, D. A., Burt, J. A., Bauman, A. G., Cavalcante, G. H., Drouillard, K. G., Kjerfve, B., Marquis, E., Trick, C. G., Usseglio, P., and Van Lavieren, H.: The growing need for sustainable ecological management of marine communities of the Persian Gulf, Ambio, 40, 4–17, https://doi.org/10.1007/S13280-010-0092-6, 2011.
Santos, R. M. and Bakhshoodeh, R.: Climate change/global warming/climate emergency versus general climate research: comparative bibliometric trends of publications, Heliyon, 7, e08219, https://doi.org/10.1016/J.HELIYON.2021.E08219, 2021.
Schwanghart, W., Ryan, M., and Korup, O.: Topographic and Seismic Constraints on the Vulnerability of Himalayan Hydropower, Geophys. Res. Lett., 45, 8985–8992, https://doi.org/10.1029/2018GL079173, 2018.
Selkoe, K. A., Halpern, B. S., Ebert, C. M., Franklin, E. C., Selig, E. R., Casey, K. S., Bruno, J., and Toonen, R. J.: A map of human impacts to a “pristine” coral reef ecosystem, the Papahānaumokuākea Marine National Monument, Coral Reefs, 28, 635–650, https://doi.org/10.1007/S00338-009-0490-Z, 2009.
Seo, J., Pokhrel, J., and Hu, J. W.: Multi-Hazard Fragility Analysis of Offshore Wind Turbine Portfolios using Surrogate Models, Renew. Sust. Energ. Rev., 165, 112552, https://doi.org/10.1016/J.RSER.2022.112552, 2022.
Shultz, J. M., Shepherd, J. M., Bagrodia, R., and Espinel, Z.: Tropical cyclones in a year of rising global temperatures and a strengthening El Niño, Disaster Health, 2, 151, https://doi.org/10.1080/21665044.2014.1111722, 2014.
Simas, T., Nunes, J. P., and Ferreira, J. G.: Effects of global climate change on coastal salt marshes, Ecol. Model., 139, 1–15, https://doi.org/10.1016/S0304-3800(01)00226-5, 2001.
Steinstraeter, M., Heinrich, T., and Lienkamp, M.: Effect of Low Temperature on Electric Vehicle Range, World Electric Vehicle Journal, 12, 115, https://doi.org/10.3390/wevj12030115, 2021.
Sumaila, U. R., Walsh, M., Hoareau, K., Cox, A., Teh, L., Abdallah, P., Akpalu, W., Anna, Z., Benzaken, D., Crona, B., Fitzgerald, T., Heaps, L., Issifu, I., Karousakis, K., Lange, G. M., Leland, A., Miller, D., Sack, K., Shahnaz, D., Thiele, T., Vestergaard, N., Yagi, N., and Zhang, J.: Financing a sustainable ocean economy, Nat. Commun., 12, 3259, https://doi.org/10.1038/s41467-021-23168-y, 2021.
Takagi, H., Mikami, T., Fujii, D., Esteban, M., and Kurobe, S.: Mangrove forest against dyke-break-induced tsunami on rapidly subsiding coasts, Nat. Hazards Earth Syst. Sci., 16, 1629–1638, https://doi.org/10.5194/nhess-16-1629-2016, 2016.
Tedim, F. and Leone, V.: The Dilemma of Wildfire Definition: What It Reveals and What It Implies, Frontiers in Forests and Global Change, 3, 134, https://doi.org/10.3389/FFGC.2020.553116, 2020.
The Nature Conservancy: Insuring Nature to Ensure a Resilient Future, https://www.nature.org/en-us/what-we-do/our-insights/perspectives/insuring-nature-to-ensure-a-resilient-future/ (last access: 17 July 2023), 2022.
The Royal Society: Sustainable synthetic carbon based fuels for transport: Policy briefing, London, https://royalsociety.org/-/media/policy/projects/synthetic-fuels/synthetic-fuels-briefing.pdf (last access: 17 November 2022), 2019.
Topor, Z. M., Rasher, D. B., Duffy, J. E., and Brandl, S. J.: Marine protected areas enhance coral reef functioning by promoting fish biodiversity, Conserv. Lett., 12, e12638, https://doi.org/10.1111/CONL.12638, 2019.
UK Centre for Ecology & Hydrology: Peatlands factsheet, https://www.ceh.ac.uk/sites/default/files/Peatland factsheet.pdf, last access: 4 November 2022.
UK Government: Net Zero Strategy: Build Back Greener, https://assets.publishing.service.gov.uk/government/uploads/system/uploads/attachment_data/file/1033990/net-zero-strategy-beis.pdf (last access: 14 July 2023), 2021.
UK Health and Safety Agency: About Carbon Capture and Storage, https://www.hse.gov.uk/carboncapture/about-carbon.htm, last access: 1 November 2022.
UN Climate Technology Centre & Network: Run-of-river hydropower, https://www.ctc-n.org/technologies/run-river-hydropower, last access: 18 March 2024.
UNDRR: Hazards, https://www.preventionweb.net/knowledge-base/hazards, last access: 4 August 2023.
UNDRR and ISC: Hazard definition and classification review (Technical Report), https://www.undrr.org/publication/hazard-definition-and-classification-review-technical-report (last access: 4 August 2023), 2020.
U.S. Geological Survey: Impacts & Mitigation – Marine Transportation, https://volcanoes.usgs.gov/volcanic_ash/marine_transportation.html (last access: 14 July 2023), 2015.
U.S. National Drought Mitigation Center: Types of Drought, https://drought.unl.edu/Education/DroughtIn-depth/TypesofDrought.aspx, last access: 19 December 2022.
U.S. National Oceanic and Atmospheric Administration: Climate Change: Global Sea Level, https://www.climate.gov/news-features/understanding-climate/climate-change-global-sea-level, last access: 19 December 2022a.
U.S. National Oceanic and Atmospheric Administration: Solar Radiation Storm, https://www.swpc.noaa.gov/phenomena/solar-radiation-storm, last access: 19 December 2022b,
U.S. National Park Service: Extratropical Storms, https://www.nps.gov/articles/extratropical-storms.htm, last access: 5 February 2023.
Utsunomiya, T., Sato, I., Yoshida, S., Ookubo, H., and Ishida, S.: Dynamic Response Analysis of a Floating Offshore Wind Turbine During Severe Typhoon Event, in: Proceedings of the ASME 2013 32nd International Conference on Ocean, Offshore and Arctic Engineering, Nantes, France, 9–14 June 2013, https://doi.org/10.1115/OMAE2013-10618, 2013
Wang, X., Liu, Z., and Chen, H.: Investigating Flood Impact on Crop Production under a Comprehensive and Spatially Explicit Risk Evaluation Framework, Agriculture, 12, 484, https://doi.org/10.3390/agriculture12040484, 2022.
Wardman, J., Sword-Daniels, V., Stewart, C., and Wilson, T.: Impact assessment of the May 2010 eruption of Pacaya volcano, Guatemala, Canterbury, New Zealand, https://ir.canterbury.ac.nz/bitstream/handle/10092/10563/12655195_SR 2012-09 - Pacaya Guatamala Impact 2010.pdf?sequence=1&isAllowed=y (last access: 14 July 2023), 2012a.
Wardman, J., Wilson, T., Bodger, P., Cole, J., and Stewart, C.: Potential impacts from tephra fall to electric power systems: A review and mitigation strategies, B. Volcanol., 74, 2221–2241, doi.org/10.1007/s00445-012-0664-3, 2012b.
Waring, B., Neumann, M., Prentice, I. C., Adams, M., Smith, P., and Siegert, M.: Forests and Decarbonization – Roles of Natural and Planted Forests, Frontiers in Forests and Global Change, 3, 58, https://doi.org/10.3389/FFGC.2020.00058/BIBTEX, 2020.
Waycott, M., Duarte, C. M., Carruthers, T. J. B., Orth, R. J., Dennison, W. C., Olyarnik, S., Calladine, A., Fourqurean, J. W., Heck, K. L., Hughes, A. R., Kendrick, G. A., Kenworthy, W. J., Short, F. T., and Williams, S. L.: Accelerating loss of seagrasses across the globe threatens coastal ecosystems, P. Natl. Acad. Sci. USA, 106, 12377–12381, https://doi.org/10.1073/pnas.0905620106, 2009.
Wicki, S. and Hansen, E. G.: Clean energy storage technology in the making: An innovation systems perspective on flywheel energy storage, J. Clean. Prod., 162, 1118–1134, https://doi.org/10.1016/j.jclepro.2017.05.132, 2017.
Wilkinson, C.: Status of Coral Reefs of the World: 2000, https://www.aims.gov.au/ (last access: 24 December 2022), 2000.
Wilson, T. M., Stewart, C., Sword-Daniels, V., Leonard, G. S., Johnston, D. M., Cole, J. W., Wardman, J., Wilson, G., and Barnard, S. T.: Volcanic ash impacts on critical infrastructure, Phys. Chem. Earth, Parts A/B/C, 45–46, 5–23, https://doi.org/10.1016/j.pce.2011.06.006, 2012.
Wollenberg, J. T., Ollerhead, J., and Chmura, G. L.: Rapid carbon accumulation following managed realignment on the Bay of Fundy, PLoS One, 13, e0193930, https://doi.org/10.1371/JOURNAL.PONE.0193930, 2018.
World Bank: Concentrating Solar Power: Clean Power on Demand 24/7, Washington, DC, https://pubdocs.worldbank.org/en/849341611761898393/WorldBank-CSP-Report-Concentrating-Solar-Power-Clean-Power-on-Demand-24-7-FINAL.pdf (last access: 31 October 2022), 2021.
World Bank Group, ESMAP, and SERIS: Where Sun Meets Water: Floating Solar Market, Washington, DC, https://openknowledge.worldbank.org/bitstream/handle/10986/31880/Floating-Solar-Market-Report.pdf?sequence=1&isAllowed=y (last access: 31 October 2022), 2019.
WWT: Coral reefs, https://www.wwt.org.uk/discover-wetlands/wetlands/coral-reefs/, last access: 18 March 2024,
Yair, Y.: Lightning hazards to human societies in a changing climate, Environ. Res. Lett., 13, 123002, https://doi.org/10.1088/1748-9326/aaea86, 2018.
Yu, K. F.: Coral reefs in the South China Sea: Their response to and records on past environmental changes, Sci. China Earth Sci., 55, 1217–1229, https://doi.org/10.1007/S11430-012-4449-5, 2012.
Zampieri, M., Ceglar, A., Dentener, F., and Toreti, A.: Wheat yield loss attributable to heat waves, drought and water excess at the global, national and subnational scales, Environ. Res. Lett., 12, 064008, https://doi.org/10.1088/1748-9326/aa723b, 2017.
Zhang, M., Smol, J. P., Bu, Z., Guo, X., and Li, B.: The influences of volcanic eruptions on the development of peatland: A case study from the Changbai Mountains, Northeast Asia, Catena (Amst.), 213, 106209, https://doi.org/10.1016/j.catena.2022.106209, 2022.
Zhang, Z., De Risi, R., and Sextos, A.: Multi-hazard fragility assessment of monopile offshore wind turbines under earthquake, wind and wave loads, Earthq. Eng. Struct. D., 52, 2658–2681, https://doi.org/10.1002/eqe.3888, 2023.
Zuzak, C., Goodenough, E., Stanton, C., Mowrer, M., Ranalli, N., Kealey, D., and Rozelle, J.: National Risk Index Technical Documentation, https://www.fema.gov/sites/default/files/documents/fema_national-risk-index_technical-documentation.pdf (last access: 19 December 2022), 2021.