the Creative Commons Attribution 4.0 License.
the Creative Commons Attribution 4.0 License.
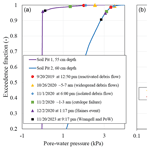
Invited perspectives: Integrating hydrologic information into the next generation of landslide early warning systems
Thom Bogaard
Roberto Greco
Manfred Stähli
Although rainfall-triggered landslides are initiated by subsurface hydro-mechanical processes related to the loading, weakening, and eventual failure of slope materials, most landslide early warning systems (LEWSs) have relied solely on rainfall event information. In previous decades, several studies demonstrated the value of integrating proxies for subsurface hydrologic information to improve rainfall-based forecasting of shallow landslides. More recently, broader access to commercial sensors and telemetry for real-time data transmission has invigorated new research into hydrometeorological thresholds for LEWSs. Given the increasing number of studies across the globe using hydrologic monitoring, mathematical modeling, or both in combination, it is now possible to make some insights into the advantages versus limitations of this approach. The extensive progress demonstrates the value of in situ hydrologic information for reducing both failed and false alarms through the ability to characterize infiltration during – as well as the drainage and drying processes between – major storm events. There are also some areas for caution surrounding the long-term sustainability of subsurface monitoring in landslide-prone terrain, as well as unresolved questions in hillslope hydrologic modeling, which relies heavily on the assumptions of diffuse flow and vertical infiltration but often ignores preferential flow and lateral drainage. Here, we share a collective perspective based on our previous collaborative work across Europe, North America, Africa, and Asia to discuss these challenges and provide some guidelines for integrating knowledge of hydrology and climate into the next generation of LEWSs. We propose that the greatest opportunity for improvement is through a measure-and-model approach to develop an understanding of landslide hydro-climatology that accounts for local controls on subsurface storage dynamics. Additionally, new efforts focused on the subsurface hydrology are complementary to existing rainfall-based methods, so leveraging these with near-term precipitation forecasts is a priority for increasing lead times.
- Article
(1154 KB) - Full-text XML
- BibTeX
- EndNote
Hydrology plays an important role in shallow-landslide initiation (Campbell, 1975; Lu and Godt, 2013; Bogaard and Greco, 2016); this has been demonstrated through many decades of monitoring hydrologic response and slope stability on individual hillslopes and zero-order basins around the world (e.g., Sidle and Swanston, 1982; Sidle and Tsuboyama, 1992; Torres et al., 1998; Godt et al., 2009; De Vita et al., 2013; Liang, 2020; Marino et al., 2020; Ashland, 2021). These observations are supported by well-established theory in soil physics and geomechanics, whereby the addition of water to porous media changes their strength and weight, contributing to a force imbalance and triggering slope failure (e.g., Terzaghi, 1943). Theoretical advances have supported the development of mathematical models to numerically simulate – with varying degrees of complexity – the conditions leading up to these critical conditions (e.g., Montgomery and Dietrich, 1994; Terlien, 1997; Van Beek, 2002; Brien and Reid, 2008; Baum et al., 2010; Lehmann and Or, 2012). Despite this conceptual understanding and advanced model development, most local and regional landslide early warning systems (LEWSs) rely on rainfall inputs alone, typically with the well-worn intensity-duration (ID) threshold approach (Cain, 1980; Saito et al., 2010; Guzzetti et al., 2008; Brunetti et al., 2010; Segoni et al., 2018) and the related event-duration (ED) threshold (Innes, 1983; Guzzetti et al., 2020). These are built upon the assumption that if it rains hard enough for long enough in a landslide-prone area, the storm event will trigger slope failures. When event-based rainfall thresholds are used alone, the hydrologic conditions preceding the triggering and the associated antecedent wetness have no bearing on predicted slope stability. The negligible role of previous rainfall, evapotranspiration, and hillslope drainage may or may not be true based on local variations (e.g., Thomas et al., 2020). Furthermore, these underlying assumptions must be questioned in the context of a changing climate and non- static (a-)biotic terrain conditions (Ehret et al., 2014), where multiple competing factors related to infiltration, drainage, and evapotranspiration interact to influence predisposing factors and triggering conditions (Gariano and Guzzetti, 2016; Jakob, 2022).
Although broadly applicable, with many centuries of rainfall data underpinning its implementation, the generalized ID approaches rely on several conceptual flaws (refer to Bogaard and Greco, 2018) and lack specificity. As the benchmark standard, these approaches have succumbed to inertia with few novel methodological advances since their early inception (e.g., Caine, 1980). Still, a handful of studies over many decades and across a variety of settings have shown that using rainfall data to develop well-informed proxies for seasonality or hillslope antecedent wetness can improve landslide prediction with ID thresholds (e.g., Campbell, 1975; Wilson and Wiezorek, 1995; Crozier, 1999; Glade, 2000; Godt et al., 2006; Napolitano et al., 2016). The calculation of these proxies often reflects the basics of infiltration and soil water storage but consistently falls short of capturing the complex wetting and drainage dynamics observed in the variably saturated near surface. Similarly, satellite and remote sensing products capture the seasonal shifts in landscape wetness that are broadly relevant for landslide potential (Felsberg et al., 2021; Zhao et al., 2021; Stanley et al., 2021; Distefano et al., 2023), but their coarse resolution and considerable latency fail to capture the rapid subsurface dynamics on hillslopes that are critical for forecasting landslide potential (Thomas et al., 2019). Electrical resistivity tomography has revealed nuances related to subsurface moisture patterns in landslide settings (e.g., Perrone et al., 2014; Uhlemann et al., 2017), but these hydrogeophysical methods remain cumbersome to implement and their sampling rates are currently too slow to capture the rapid unsaturated zone responses that trigger shallow landslides (Nimmo et al., 2021). Recent progress with automated empirical modeling shows some promise in recreating hillslope hydrologic response (Orland et al., 2020) and highlights the importance of rainfall over specific terrain attributes in predicting spatiotemporal populations of landslides (Mondini et al., 2023). However, in an uncertain future with increasing landscape disturbances, climate change, and non-stationary responses in hydrologic systems, the next generation of LEWSs can be advanced through incorporating a mechanistic understanding of the hydroclimatology of triggering conditions.
Recently, the emergence of the “internet of things” has provided further motivation for integrating hydrologic information to improve LEWSs' predictive performance because subsurface monitoring data can be accessed in real time to understand evolving hillslope wetness conditions (Mirus et al., 2018; Abraham et al., 2020; Piciullo et al., 2022). This approach has the potential to outperform rainfall-based estimates of antecedent wetness and imminent triggering conditions because in situ data can capture the true hillslope hydrologic response associated with landslide initiation. A handful of studies that integrate different types of subsurface measurements directly into landslide initiation thresholds show some promising results (Mirus et al., 2018; Zhao et al., 2019; Marino et al., 2020; Wicki et al., 2020; Abraham et al., 2021; Pecoraro and Calvello, 2021); their success reflects the understanding of the relevant hydrologic processes for their region of interest. Despite the many advances and limitations of current approaches to LEWSs (refer to reviews by Guzzetti et al., 2012, 2020; Stähli et al., 2015; Piciullo et al., 2018), new research on real-time hydrometeorological thresholds is still an emerging field (Greco et al., 2023). No guidelines have been established for developing a reliable LEWS that is informed, at least in part, by real-time hydrological information. Considering the first action in the Kyoto Landslide Commitment 2020 involves improving the precision and reliability of landslide warning (Sassa et al., 2023), we propose that integrating insights from in situ hydrologic measurements into LEWSs is essential.
Continuous field monitoring for comprehension of triggering processes
The complex interaction between hydrological and mechanical processes results in many possible ways of adding water to transition from stable to unstable conditions, leading to very different types of triggering conditions for seemingly similar settings (e.g., Fusco et al., 2022). These depend largely on how geology, climate, geomorphology, vegetation, and landscape disturbances have influenced the geometry and hydromechanical properties of soils, vegetation distribution, and the geometry of hillslope source areas where landslides initiate (Sidle et al., 2017). Every hillslope is unique, so we cannot characterize the true subsurface heterogeneity and corresponding controls on landslide triggering across a landscape, but hydrological information provides a foundation of comprehension to inform landslide forecasting across contrasting locations. For example, in areas with strong seasonality the antecedent soil moisture conditions may be critical for refining landslide initiation thresholds (e.g., Godt et al., 2006; Mirus et al., 2018; Wicki et al., 2020; Marino et al., 2021). In contrast, some regions typically remain quite wet, and prior conditions seem to add very limited value in constraining landslide potential (e.g., Thomas et al., 2020; Patton et al., 2023). During the most recent decades, soil moisture has become increasingly easy to monitor in situ, but other measured variables such as groundwater levels have also been used quite effectively (Wei et al., 2019, 2020; Illien et al., 2021; Marino et al., 2021; Uwihirwe et al., 2022; Roman Quintero et al., 2023). As a state variable, the analysis of volumetric water content profiles can reveal whether the soil is accommodating infiltration through changes in storage or if it has exceeded field capacity and is allowing more rapid vertical fluxes to the saturated zone below. In contrast, shallow-groundwater fluctuations (when they can be measured) reflect not just water added from vertical infiltration, but also the three-dimensional (3D) subsurface flow field from upslope accumulation to downslope drainage. Of course, other hydrologic state variables may be used as proxies for antecedent conditions such as snowmelt (Mostbauer et al., 2018; Wayllace et al., 2019) and catchment storage (Ciavolella et al., 2016; Marino et al., 2022).
It is difficult to know a priori which conditions and variables are important for an area of interest, but even a few years of hydrologic monitoring can improve understanding of the variably saturated hillslope responses in stormflow generation (e.g., Beven, 2012; Blume and van Meerveld, 2015) and landslide initiation or reactivation (e.g., Godt et al., 2009; Mirus et al., 2017). Accounting for regionally specific controls on infiltration and hillslope drainage dynamics could help improve hydrometeorological thresholds by reducing failed alarms, such as those produced by relatively modest storms on already very wet soils, as well as lowering the number of false alarms, such as those related to heavy precipitation on dry soils. Therefore, to develop the next generation of LEWSs, expanding hillslope hydrologic monitoring from a handful of existing networks to a wider variety of landslide prone terrain worldwide would be highly beneficial. The potential value of long-term hydrologic measurements can be inferred from recent advances in characterizing landslide triggering based on identifying rainfall anomalies or recurrence intervals (e.g., Kirschbaum and Stanley, 2018; Marc et al., 2022). A greatly expanded and openly accessible network of hydrologic observations would further supplement such approaches, using relative hillslope wetness to support new inferences about triggering potential.
However, established guidelines for landslide hydrological monitoring are lacking, and providing general advice on how to select appropriate sites and instrumentation equipment is exceptionally difficult. This challenge is compounded by subsurface heterogeneity that cannot be known a priori and logistical considerations that often influence instrument placement (e.g., site access and safety considerations on steep slopes). These nuances are not emphasized in publications or presentations, so in practice the many seemingly subjective elements in such studies reflect the crucial role of expert judgment. Theoretically, each individual hillslope has a critical pore water pressure (or saturated thickness) that triggers failure, but this critical state is difficult to identify for any given hillslope, and there are a multitude of hydrologic responses to spatially variable rainfall across a heterogeneous landscape. Additionally, the precise calibration of sensors to measure accurate volumetric water contents is important for some modeling calculations but may be of limited value for warnings compared to capturing the range of responses to multiple storms (e.g., Wicki et al., 2020). Thus, a first step for hydrologic monitoring is developing a strong conceptual model of local conditions at the site of interest, based on available observations including geologic maps, soil classifications, landscape morphology, climate, and even records of rainfall and streamflow from the region. We also stress that the measurement of the specific hydrologic state variables (i.e., soil moisture versus groundwater levels versus soil suction) or the precise values is not critical. Instead, we conclude that identifying a suitable monitoring location and selecting measured variables that capture the relative change in hillslope wetness conditions across the landscape provide the most informative variables. In particular, site installations that reflect the widest variability during and between landslide events reveal the most about hillslope storage dynamics.
The influence of spatial variability – as well as the difficulty in deciding what to measure and where – can be demonstrated anecdotally using data from the USGS landslide monitoring site in Sitka, Alaska (Smith et al., 2023). This includes volumetric soil water content and positive pore water pressures measured in two shallow soil pits that are less than 10 m apart from each other on a steep hillslope. The period shown (Fig. 1) encompasses responses to several major storm events that triggered landsliding across the region, one of which ultimately culminated in a fatal landslide in Haines, Alaska (refer to Darrow et al., 2022), roughly 200 km to the northeast of the monitoring site in Sitka. In Soil Pit 1, the matric potential and soil moisture sensors show some variations in near-surface conditions and flashy piezometer response only during peak rainfall. Less than 10 m downslope, the near surface in Soil Pit 2 is persistently wet with continuous shallow-groundwater fluctuations throughout the period. Thus, for informing LEWS development, the largely absent pore pressure measurements in Soil Pit 1 might seem of limited value compared to the soil moisture record for assessing antecedent conditions. In contrast, Soil Pit 2 clearly shows the gradual elevation in groundwater levels for successive landslide-producing storms, but consistently high soil moisture values exhibit limited dynamics with no valuable information for LEWSs. Thus, the value of soil moisture in the unsaturated zone versus pore water pressures in the shallow saturated zone would depend entirely upon the location of the soil pit within the landscape, which is difficult to assess from the landscape position alone. However, together, instrumentation in these two soil pits reveals a potential mechanistic explanation for the shallow landslides and debris flows around Sitka: well-drained hillslopes remain consistently wet and support disconnected zones of perched saturation, so landslides tend to occur once these perched saturated zones connect across broader areas of steep hillslopes and their drainage capacity is overwhelmed by consistently high rainfall input.
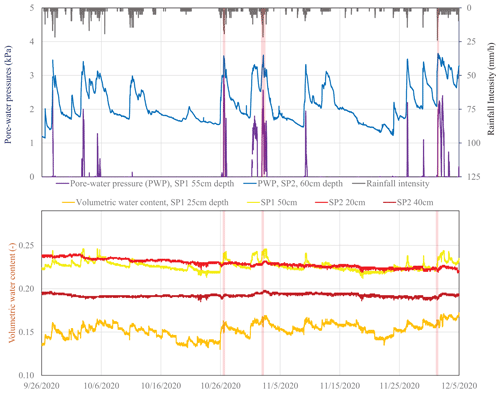
Figure 1Time series of rainfall and pore water pressure and volumetric water content in two soil pits (SP1 and SP2) recorded on a steep hillslope above Sitka, in southeast Alaska, observed during a sequence of large storm events in October–December 2020 (dates in UTC); the days on which landslides occurred throughout the region are shown by the transparent red bars. Note that data are available for download in Smith et al. (2023), and near-real-time plots of data are available for situational awareness at https://usgs.gov/programs/landslide-hazards/science/sitka-ak, last access: 11 November 2024.
From another perspective, the same hydrologic data can be plotted within a probabilistic framework to illustrate the importance of the relative wetness of selected measurement records rather than absolute values of all the different state variables. Here, we compare the landslide occurrence data relative to the cumulative frequency distributions of volumetric water content and pore water pressure values from both soil pits, as well as the established rainfall threshold for Sitka, Alaska, for a period from September 2019 through December 2023 (Fig. 2). The rainfall threshold uses the daily maximum rainfall accumulation during any 3 h period and was established with extensive calibration and validation using many decades of continuous records and landslide occurrence data (Patton et al., 2023). In contrast the hydrologic data reflect just a few years of discontinuous monitoring records (Smith et al., 2023). A comparison of the three plots underscores the value of the shallow-water-table fluctuations relative to the rainfall threshold and soil moisture for this location. In particular, the mere presence of shallow groundwater in Soil Pit 1 apparently exhibits the strongest correlation with landslide occurrence (Fig. 2), even for distal locations well beyond the area considered for the calibration of the rainfall threshold (refer to Patton et al., 2023).
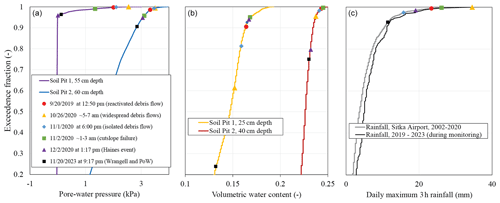
Figure 2Known landslide events across southeast Alaska (AK) with cumulative probability distribution for different observations in Sitka, AK. (a) Shallow-groundwater pressures measured every 5 min with piezometers in two different soil pits, (b) volumetric soil water content measured every 5 min by four probes in these two soil pits, and (c) daily maximum accumulation of rainfall during any 3 h period. Note that all landslide events are shown in local Alaska time: those listed from September 2019–November 2020 occurred within 2 km of Sitka, AK (Patton et al., 2023); the December 2020 event occurred 250 km to the northeast in Haines, AK, and resulted in two fatalities (Darrow et al., 2022); and the November 2023 event had a widespread impact on areas over 150 km to the southeast on Prince of Wales (PoW) Island and in Wrangell, where six people were killed (Nicolazzo et al., 2024).
The major benefits of hydrologic data for improving comprehension and capturing landslide-triggering conditions (Figs. 1 and 2) can be tempered by acknowledging several underappreciated issues with using in situ monitoring for landslide forecasting. First, the return period of relatively infrequent (but highly destructive) landslide events is often longer than the typical life expectancy of subsurface hydrologic monitoring equipment. Broad estimates of the rainfall recurrence needed to trigger widespread landsliding events across the globe range from a variable 95th percentile (Kirschbaum and Stanley, 2018) to 10-year anomalies (Marc et al., 2022), but on these timescales, instrumentation often requires substantial maintenance that is resource-intensive or may exceed their manufacturer-reported life expectancies, experiences the onset of electronic drift, or succumbs to destruction from wildlife or vandalism. Indeed, along with solid expert judgment in site selection, there are also substantial elements of chance that allowed previous researchers to capture the hydrologic conditions during a natural landslide initiation event (Montgomery et al., 2009; Godt et al., 2009; Mirus et al., 2017; Liang, 2020). Whereas instruments to measure groundwater tables, streamflow, and precipitation can potentially be replaced as needed, volumetric water content sensors must be placed in relatively undisturbed soil (Caldwell et al., 2022), which means that when sensors fail, they cannot be readily exchanged in the same place without disturbances to the porous medium itself. Installation of pore pressure sensors varies, with some favoring direct contact with the porous media (e.g., Smith et al., 2023) and others using standpipe piezometers (e.g., Montgomery et al., 2009) that potentially allow sensors to be exchanged more easily. Although interoperability across measurement types presents a potential challenge to the long-term sustainability of a global near-real-time hydrologic monitoring network to inform LEWSs, useful hydrometeorological thresholds can be developed by intelligently leveraging existing hydrologic monitoring networks. In particular, observed records can be extended with a measure-and-model approach, whereby shorter periods of data along with measured hydraulic and mechanical properties can inform robust models of the hydrologic conditions related to slope failure (e.g., Ebel et al., 2008; Wicki et al., 2021; Uwihirwe et al., 2022). Even if hillslope hydrologic modeling has its own problem areas (refer to the discussion below) it is likely the most sustainable way to synthesize hydrologic information across space and time.
Second, we accept that in situ subsurface instrumentation favors monitoring precise variations in time rather than capturing broader spatial patterns, and it therefore shares similar limitations to any other point measurements, such as rain gages. As with all measurement networks, some expert judgment is needed to design and select a site for subsurface instrumentation, which influences the data collected and corresponding conclusions that can be inferred. Numerous studies related to subsurface stormflow response used distributed measurements to identify very localized processes that govern hillslope hydrologic responses such as the role of irregular subsurface topography on “fill-and-spill” processes (Tromp-van Meerveld and McDonnell, 2011), heterogeneous soil profiles and weathering (Zimmer and Gannon, 2018), and preferential flow (Beven and Germann, 1982). However, the same is the case for rainfall measurements, which must rely on radar and satellite estimates of rainfall variability to determine spatial patterns at relatively coarse scale.
2.1 Extrapolating across spatial and temporal scales
It may be even more difficult to extrapolate subsurface hydrologic response dynamics derived from one soil profile across a heterogeneous landscape than atmospheric processes such as rainfall intensities, so some clear advances in remote sensing methods may ultimately be informative for landslide modeling. At the scale of tens to hundreds of meters, cosmic-ray neutron sensors have the capacity to estimate changes in relative hillslope wetness between storms (e.g., Francke et al., 2022), yet these methods remain largely untested for landslide studies in steep, densely vegetated terrain. At the scale of tens to hundreds of kilometers, distributed estimates of soil moisture from remote sensing provides global coverage but falls short of capturing the temporal variations observed in situ that are critical for precise landslide forecasting (e.g., Thomas et al., 2019). Novel space–time approaches leverage extensive information on timing and locations of past landslide occurrences to simulate the evolution of landslide probabilities across a given landscape during storm events (e.g., Lombardo et al., 2020; Bordoni et al., 2021). Although these data-driven approaches are inherently biased by the available data, emerging approaches for integrating the rainfall triggering with the underlying landscape characteristics seem quite promising (Steger et al., 2024) and would likely benefit further from considering local hydrologic information. Ongoing efforts to incrementally improve probabilistic thresholds for global nowcasting have leveraged satellite rainfall and soil moisture products (i.e., Stanley et al., 2021) but lack a framework for considering the inherently local controls on subsurface infiltration and drainage processes. Despite the unknowable heterogeneity in the subsurface, one of the greatest opportunities for hydrometeorological threshold improvement is to use accurate temporal dynamics from in situ observations to inform improved landslide modeling across the larger spatial footprints at hillslope, watershed, and regional scales. A mechanistic understanding of landslide-triggering conditions associated with different hydrologic responses would also help constrain the spatial extent over which different hydrometeorological thresholds apply. The most promising new approach for this leverages an intentionally oversimplified bucket model of infiltration and drainage along with spatially variable rainfall, which is able to simulate relative hillslope wetness across vast regions with remarkable accuracy (Perkins et al., 2024).
2.2 Alternative hydrometeorological threshold formulations
The selection of statistical optimization criteria can influence the balance between failed and false alarms for a given hydrometeorological threshold formulation (e.g., Conrad et al., 2021), but a thorough discussion of uncertainty and performance criteria (e.g., Piciullo et al., 2020) is outside of the scope of this perspective. Instead, we address some informative contrasts between the formats of hydrometeorological thresholds derived using contrasting methods and data inputs (Fig. 3). The deterministic threshold (Fig. 3, purple) is based on millions of simulated events from a one-dimensional (1D) infiltration model calibrated using a few positive pore water pressure measurements and evaluated with landsliding events from the San Francisco Bay Area, California (Thomas et al., 2018). This threshold lacks a functional format, but one could theoretically be developed for its convex form, with rapidly decreasing stability under higher antecedent wetness and unconditionally unstable conditions above roughly 0.6 saturation. The format of a bilinear threshold (Fig. 3, blue) was identified empirically and optimized using receiver operating characteristics with numerous landslide events and years of hydrologic monitoring in the Puget Sound area, Washington (Mirus et al., 2018; Conrad et al., 2021). It is certainly oversimplified as well, but the convenient functional format has led to its implementation in other settings such as data-sparse Rwanda (Uwihirwe et al., 2022) and the testing of satellite-based thresholds in data-rich parts of California (Thomas et al., 2019).
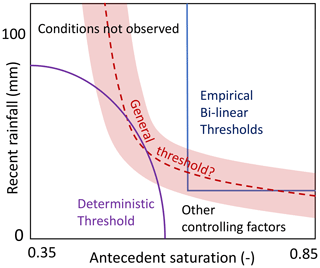
Figure 3Different formats of hydrometeorological thresholds for landslide initiation developed based on empirical interpretation (blue), shown with bilinear thresholds optimized using several years of monitoring data and landslide inventories (Mirus et al., 2018), versus theoretical understanding (purple) based on a deterministic method using infiltration modeling and millions of synthetic storm events (Thomas et al., 2018). The deterministic threshold shows unconditionally unstable conditions for excessive rainfall or high saturation levels. In contrast, empirical thresholds indicate that excessive rainfall on dry soils will not trigger failures, whereas even at the highest observed saturations moderate rainfall is still needed to trigger failures. Heuristically, a general threshold that accommodates both theoretical understanding and empirical observations seems more reasonable.
The insight that the deterministic versus empirical approaches support entirely different functional formats of hydrometeorological thresholds raises the questions of whether one or the other is more correct and why they vary so distinctly. The deterministic thresholds reflect our limited ability to quantify the complex storage dynamics during landslide events and not necessarily all the processes relevant to infiltration and drainage, which results in considerably broad conditions that are unconditionally unstable. On the other hand, the empirical threshold format was selected based only on the conditions we have observed thus far and does not necessarily represent all the relevant possibilities. In a recent paper, Palazzolo et al. (2023) propose a hydrometeorological threshold using antecedent soil moisture versus rainfall intensity that exhibits a similar format to our deterministic threshold at low antecedent wetness combined with a constant rainfall intensity cutoff for higher saturations just like the empirical bilinear threshold. Earlier studies have tested linear models, which also led to improvements relative to rainfall-only thresholds (Mirus et al., 2018; Marino et al., 2020).
Overall, these sparse examples of contrasting formats reflect subjective modeling choices and represent just some of the results emerging from recent research into hydrometeorological thresholds, but they raise two important issues: first that there are observed storage and drainage processes that our hydrological models do not adequately capture (i.e., known conceptual limitations) and second that there are conditions we have not yet observed that are difficult to predict (i.e., unknown range of responses). For example, when the vast majority of both triggering and non-triggering events exhibit a correlation between antecedent wetness and rainfall accumulation (e.g., Palazzolo et al., 2023), it is particularly difficult to project responses to unprecedented rainfall on very dry antecedent soils or rapid snowmelt events during low-intensity rainfall. Heuristically, we would expect a more generalized threshold that considers the uncertainty in triggering conditions and reflects our conceptual understanding that either more precipitation or wetter antecedent conditions should each increase the likelihood of landsliding (Fig. 2, red). However, our hypothesis of this generalized hydrometeorological threshold remains untested. Further exploration in different regions with contrasting geologic and environmental settings should help refine best practices and guidelines. Conversely, additional studies might also reveal that there is no universally superior format for hydrometeorological thresholds, and instead local practices and priorities will determine what is used operationally, depending on data availability, system expectations, and risk tolerances.
2.3 Limits of process understanding
Three-dimensional, fully coupled surface–subsurface hydrologic models emerged as a relatively effective but data-intensive method for quantifying the hydrogeomorphic processes that contribute to landslide initiation (Loague et al., 2006; Ebel et al., 2008). Now, extensive databases on soil geometry and textural classifications (e.g., SSURGO, 2024), novel pedotransfer functions (e.g., Van Looy et al., 2017; Lehmann et al., 2021), high-resolution continental-scale DEMs (e.g., 3DEP: US Geological Survey, 2019), and comprehensive precipitation databases (e.g., IMERG: Huffman et al., 2015) could, in theory, facilitate using physics-based approaches, both conceptual and deterministic, to develop hydrometeorological thresholds for settings all over the globe (e.g., Lehmann et al., 2019). However, theoretical gaps in hillslope hydrologic modeling remain a major obstacle. Well-calibrated infiltration models designed to capture observed landslide initiation processes still struggle to simulate the continuous soil moisture dynamics between events (Wicki et al., 2021; Piciullo et al., 2022) or the influence of complex soil structures (Mirus, 2015; Fatichi et al., 2020).
Regardless of their high computational expense and data demands for parameterization, the reality is we still do not fully comprehend the physics of variably saturated subsurface flow through complex landscapes. The equation used to simulate diffusive flow through variably saturated soils has been around for quite some time (Richards, 1931), but infiltration models based on Richards' equation cannot produce results that are consistent with observations of non-sequential wetting fronts (e.g., Graham and Lin, 2011) or preferential flow in the unsaturated zone (Nimmo, 2012, 2016; Beven and Germann, 1982, 2013). These localized hydrological processes combined with subsurface heterogeneity may explain why some hillslopes fail and why other adjacent slopes remain stable, and hence this may explain to some degree the variability and uncertainty in landslide-triggering conditions. Such questions remain largely academic, as this degree of detail is not necessary (or even achievable) to provide useful and actionable information for landslide loss reduction. Instead, we can focus on more practical modeling and comprehension of hydroclimatology with representative state variables to reduce failed and false alarms.
Ultimately, landslides occur at some point after the initiation zones fill up with infiltration at a rate faster than they can drain. Although the infiltration component of landslide triggering involves the largely vertical 1D percolation of precipitation through unsaturated soils, stormflow responses involve 3D processes including lateral flow diversion and drainage that can either enhance or reduce landslide potential. These processes are controlled not just within the soils where shallow landslides initiate but by complex flow paths in the underlying saprolite, weathered bedrock, and even fracture flow through intact bedrock. Although robust physically based models still struggle to fully capture those two competing processes due to both a lack of data and a lack of process understanding, further expansion of simpler conceptual models that are informed entirely by monitoring data such as a leaky bucket (e.g., Wilson and Wiezorek, 1995), wetness indices (e.g., Godt et al., 2006; Perkins et al., 2024), source-responsive methods (e.g., Nimmo, 2016), or empirical approaches (e.g., Orland et al., 2020; Bordoni et al., 2021) may be beneficial. These would have the greatest impact if they can leverage long-term monitoring data to capture the critical conditions when vertical infiltration exceeds drainage during major storm events, as well as the effect of the landscape-scale storage dynamics during and between such events. Further iterations of measurement–model comparisons would be helpful to determine how we can better represent these two competing infiltration and drainage processes in a way that is representative enough to improve landslide forecasting.
2.4 Limits of observational datasets
The wide availability of precipitation records, going back centuries in many cases, paired with the limited frequency of landslide events, might lead many to believe that there are no surprises and that rainfall thresholds are the most robust and achievable route to inform LEWSs. However, the challenges with balancing failed and false alarms in virtually all landslide forecasts indicate that there are processes we have not understood and conditions we have not yet observed. For example, the hydrometeorological threshold models discussed in Fig. 3 were developed without any observations of very large storms on very dry soils, and yet that is potentially the combination of conditions that may influence shallow landsliding in a changing climate (Gariano and Guzzetti, 2016).
Indeed, the literature includes many different assertions about what to expect in a warming world for different flavors of landslides (Cannon and DeGraff, 2009; Bennett et al., 2016; Coe et al., 2016, 2018; Parker et al., 2016; Handwerger et al., 2019; Kirschbaum et al., 2020). The degree to which drier soils from elevated evapotranspiration may balance out the effects of the increased frequency and intensity of extreme meteorological events remains unclear. Thus, an important challenge is to develop a mechanistic understanding that would be applicable in a changing climate to inform LEWSs (e.g., Ehret et al., 2014). This includes understanding how the combination of hydraulic properties such as water-retention curves, porosity, saturated hydraulic conductivity, and bedrock fractures influences hillslope storage and drainage dynamics (e.g., Ebel et al., 2008) or how soil grain size and mineralogy affect mechanical properties such as suction stress, cohesion, and internal friction angle (e.g., Lehmann et al., 2021). Such an understanding can be used to improve simplified bucket models (e.g., Perkins et al., 2024) to consider spatially variable hillslope hydrologic responses. As more studies use a combination of measurements and modeling to evaluate the local and regional controls on hillslope hydrologic conditions, it seems likely that we will identify specific threshold formulations needed for different types of hydroclimatic and environmental settings. In the same way that the rainfall intensity-duration approach does not apply universally well across the globe (Caine, 1980; Guzzetti et al., 2008; Baum and Godt, 2010), we could ultimately learn more about the variability and applicability of hydrometeorological thresholds.
Despite these notable challenges, we maintain that integrating knowledge of hillslope hydrologic processes into landslide forecasting tools is a very promising path forward. Considering that both monitoring systems and models are always imperfect and that they provide an incomplete picture of the reality, a major challenge is to find a way, with limited understanding and even more limited data availability, to reduce LEWS errors. In some cases, hydrology may not add valuable information about rainfall alone, but in many other settings, we expect that further research can identify hydrologic variables linked to landscape-scale processes that reflect the geologic, geomorphic, and climatic controls on predisposing conditions. Capturing those factors would help improve advance warning for potential landslide conditions prior to short-term forecasts of the precipitation that could ultimately trigger failures locally. With realistic expectations, careful considerations of the issues we outlined above can serve as a framework for a systematic and reliable way to integrate hydrometeorological thresholds into improved local-scale LEWSs.
In contrast to accurate hyper-localized LEWSs that have been within reach for some time (e.g., Campbell, 1975; Chleborad et al., 2008), the transition towards uniform, regional-scale systems with meaningful spatial coverage would require further testing and new methods for interpolating between, and extrapolating beyond, sparse existing observations. In this context, the following efforts provide promising paths towards improving landslide forecasting models worldwide:
-
Determine regional controls on landslide hydroclimatology. This involves assessing the potential infiltration conditions that can influence landslide triggering, including snowmelt (Hinds et al., 2019), prolonged storms (Coe et al., 2015), steady and frequent rainfall (Chleborad et al., 2008), or simply high-intensity bursts of precipitation (Caine, 1980). This also includes quantitatively characterizing landslide seasonality (e.g., Luna and Korup, 2022) and how those seasons may change in the future for different types of slope failure (Jakob, 2022).
-
Develop objective methods to identify the state variables and timescales of interest (e.g., Conrad et al., 2021). In particular, how can we most effectively separate the continuous transition from antecedent to triggering conditions to improve LEWS performance? Because major obstacles to forecasting subsurface hydrologic conditions remain, it is important to identify which hydrologic factors can be leveraged effectively with the timescales supported by quantitative precipitation forecasts (e.g., Conrad et al., 2021; Patton et al., 2023).
-
Explore what currently available hydrogeologic information can reveal about subsurface responses. This includes further investigation of important differences between flat versus steep terrain (e.g., Wicki et al., 2020, 2023) and satellite versus in situ hydrologic information (e.g., Thomas et al., 2019) to better leverage existing worldwide monitoring networks. It may be particularly important to explore hydrologic information at a spatial scale that accords with the landslide release extent (rather than relying on single sensors or remotely sensed estimates). This may involve further exploration of emerging technologies (e.g., Franke et al., 2022) or the use of multiple sensors to characterize variably saturated conditions along both vertical and longitudinal hillslope profiles (e.g., Mirus et al., 2017). It is certainly more promising to look at relative changes in hydrologic metrics than to seek absolute threshold values.
-
Establish a global repository of rainfall-triggered landslide inventories with associated hydrologic information across different hydroclimatic and environmental conditions. Open access to these data will facilitate research and synthesis with different conceptual modeling approaches, from data-driven methods (e.g., Steger et al., 2024) to simple process-based forecasts (Perkins et al., 2024). Then, through a coordinated measure-and-model approach, researchers can test generalized methods to extrapolate controls on infiltration and drainage dynamics across a range of realistic landslide-triggering conditions.
Beyond these potential research opportunities, we close with a few practical considerations. There is extensive debate about what “early” means in the context of actionable information for an operational LEWS, which is a discussion reserved for another venue. Instead, it is important to consider that any LEWS that relies only on currently observed conditions, whether precipitation, hydrology, or even detection of incipient slope movement, is largely limited to “now-casting” rather than forecasting. Hydrologic state variables reflect the subsurface response to recent water inputs, and for this reason these observations should be most accurately indicative of failure (Figs. 1 and 2). Precipitation precedes infiltration and represents the forcing conditions immediately prior to the hydrologic response when landslides are imminent. To effectively leverage these two sources of information and maximize the “early” in LEWSs, hydrometeorological thresholds ideally would rely on some modeling, whether simple or complex, that starts with recent hydrologic conditions and predicts the effects of forecasted precipitation on slope stability and that potentially assimilates monitoring data to update such forecasts in near-real time.
At this point we can definitively state that integrating hydrologic information has led to improvements in landslide forecasting over existing LEWS model formats, but are the additional investments in data and research needed for these universally justified for operational systems? Ultimately, it is unclear how well we can expect any LEWS to perform barring other scientific advances. For example, we are not aware of any operational or research-oriented landslide forecasting approach that successfully accounts for the spatially variable rainfall, triggering conditions, or inherent uncertainties in short-range quantitative precipitation forecasts. Although LEWSs may remain an imperfect tool due to the inherently stochastic and episodic nature of landslide initiation, advancing our understanding of hillslope hydrology across different climatological and geologic settings is well within reach and could soon lead to improved landslide forecasting models globally.
All data can be downloaded at https://doi.org/10.5066/P9GFA6PC (Smith et al., 2023).
BBM, TB, RG, and MS contributed to the discussion and conceptualization of the perspectives herein. BBM performed the data curation and visualization. BBM prepared the original manuscript and performed the revisions with contributions and editing from all co-authors.
The contact author has declared that none of the authors has any competing interests.
Publisher's note: Copernicus Publications remains neutral with regard to jurisdictional claims made in the text, published maps, institutional affiliations, or any other geographical representation in this paper. While Copernicus Publications makes every effort to include appropriate place names, the final responsibility lies with the authors.
The authors would like to thank USGS colleagues Dennis Staley and Brian Collins, as well as Luigi Lombardo at University of Twente and Wei-Li Liang at National Taiwan University, for their constructive reviews of earlier versions of this paper. The first author is grateful for the WSL Visiting Fellowship that helped facilitate the collaborations and discussions leading to this “Invited perspectives” paper.
This paper was edited by Paolo Tarolli and reviewed by Luigi Lombardo and Wei-Li Liang.
Abraham, M. T., Satyam, N., Pradhan, B., and Alamri, A. M.: IoT-based geotechnical monitoring of unstable slopes for landslide early warning in the Darjeeling Himalayas, Sensors, 20, 2611, https://doi.org/10.3390/s20092611, 2020.
Abraham, M. T., Satyam, N., Rosi, A., Pradhan, B., and Segoni, S.: Usage of antecedent soil moisture for improving the performance of rainfall thresholds for landslide early warning, CATENA, 200, 105147, https://doi.org/10.1016/j.catena.2021.105147, 2021.
Ashland, F. X.: Critical shallow and deep hydrologic conditions associated with widespread landslides during a series of storms between February and April 2018 in Pittsburgh and vicinity, western Pennsylvania, USA, Landslides, 18, 2159–2174, https://doi.org/10.1007/s10346-021-01665-x, 2021.
Baum, R. L. and Godt, J. W.: Early warning of rainfall-induced shallow landslides and debris flows in the USA, Landslides, 7, 259–272, https://doi.org/10.1007/s10346-009-0177-0, 2010.
Baum, R. L., Godt, J. W., and Savage, W. Z.: Estimating the timing and location of shallow rainfall-induced landslides using a model for transient, unsaturated infiltration, J. Geophys. Res., 115, 3013, https://doi.org/10.1029/2009JF001321, 2010.
Bennett, G. L., Roering, J. J., Mackey, B. H., Handwerger, A. L. Schmidt, D. A., and Guillod, B .P.: Historic drought puts the brakes on earthflows in Northern California, Geophys. Res. Lett., 43, 5725–5731, https://doi.org/10.1002/2016GL068378, 2016.
Beven, K. J.: Rainfall-runoff modeling: The primer, ISBN:9781119951001, John Wiley & Sons, Ltd., https://doi.org/10.1002/9781119951001, 2012.
Beven, K. J. and Germann, P. F.: Macropores and water flow in soils, Water Resour. Res., 18, 1311–1325, https://doi.org/10.1029/WR018i005p01311, 1982.
Beven, K. J. and P. Germann, P. F.: Macropores and water flow in soils revisited, Water Resour. Res., 49, 3071–3092, https://doi.org/10.1002/wrcr.20156, 2013.
Blume, T. and van Meerveld, I.: From hillslope to stream: Methods to investigate subsurface connectivity, WIREs Water, 2, 177–199 https://doi.org/10.1002/wat2.1071, 2015.
Bogaard, T. and Greco, R.: Landslide hydrology: from hydrology to pore pressure, WIREs Water, 3, 439–459, https://doi.org/10.1002/wat2.1126, 2016.
Bogaard, T. and Greco, R.: Invited perspectives: Hydrological perspectives on precipitation intensity-duration thresholds for landslide initiation: proposing hydro-meteorological thresholds, Nat. Hazards Earth Syst. Sci., 18, 31–39, https://doi.org/10.5194/nhess-18-31-2018, 2018.
Bordoni, M., Vivaldi, V., Lucchelli, L. Ciabatta, L., Brocca, L., Galve, J. P., Meisina, C.: Development of a data-driven model for spatial and temporal shallow landslide probability of occurrence at catchment scale, Landslides, 18, 1209–1229, https://doi.org/10.1007/s10346-020-01592-3, 2021.
Brien, D. L. and Reid, M. E.: Assessing deep-seated landslide susceptibility using 3-D groundwater and slope-stability analyses, southwestern Seattle, Washington, in: Landslides and Engineering Geology of the Seattle, edited by: Baum, R. L., Godt, J. W., and Highland, L. M., Washington, Area, Geological Society of America Reviews in Engineering Geology, Geological Society of America, https://doi.org/10.1130/2008.4020(05), 2008.
Brunetti, M. T., Peruccacci, S., Rossi, M., Luciani, S., Valigi, D., and Guzzetti, F.: Rainfall thresholds for the possible occurrence of landslides in Italy, Nat. Hazards Earth Syst. Sci., 10, 447–458, https://doi.org/10.5194/nhess-10-447-2010, 2010.
Caine, N.: The rainfall intensity-duration control of shallow landslides and debris flows, Geogr. Ann A, Phys. Geogr., 62, 23–27, https://doi.org/10.2307/520449, 1980.
Caldwell, T. G., Cosh, M. H., Evett, S. R., Edwards, N., Hofman, H., Illston, B. G., Meyers, T., Skumanich, M., and Sutcliffe, K.: In situ soil moisture sensors in undisturbed soils, J. Vis. Exp., 189, e64498, https://doi.org/10.3791/64498, 2022.
Campbell, R. H.: Soil slips, debris flows, and rainstorms in the Santa Monica Mountains and vicinity, southern California, U.S. Geological Survey Professional Paper, 851, 51 pp., https://doi.org/10.3133/pp851, 1975.
Cannon, S. H. and DeGraff, J.: The increasing wildfire and post-fire debris-flow threat in western USA, and implications for consequences of climate change, in: Landslides – Disaster risk reduction, edited by: Sassa, K. and Canuti, P., Springer Berlin Heidelberg, Berlin, Heidelberg, 177–190, https://doi.org/10.1007/978-3-540-69970-5_9, 2009.
Chleborad A. F., Baum, R. L., Godt, J. W., and Powers, P. S.: A prototype system for forecasting landslides in the Seattle, Washington, area, Rev. Eng. Geol., 20, 103–120, https://doi.org/10.1130/2008.4020(06), 2008.
Ciavolella, M., Bogaard, T., Gargano, R., and Greco, R.: Is there predictive power in hydrological catchment information for regional landslide Hazard assessment?, Proced. Earth Plan. Sc., 16, 195–203, https://doi.org/10.1016/j.proeps.2016.10.021, 2016.
Coe, J. A., Kean, J. W., Godt, J. W., Baum, R. L., Jones, E. S., Gochis, D., and Anderson, G. S.: New insights into debris-flow hazards from an extraordinary event in the Colorado Front Range, GSA Today, 29, 4–10, https://doi.org/10.1130/GSATG214A.1, 2015.
Coe, J. A.: Landslide hazards and climate change: a perspective from the United States, in: Slope safety preparedness for impact of climate change, edite by: Ho, K. K. S., Lacasse, S., and Picarelli, L., CRC, Boca Raton, 479–523, https://doi.org/10.1201/9781315387789-16, 2016.
Coe, J. A., Bessette-Kirton, E. K., and Geertsema, M.: Increasing rock-avalanche size and mobility in Glacier Bay National Park and Preserve, Alaska detected from 1984 to 2016 Landsat imagery, Landslides, 15, 393–407, https://doi.org/10.1007/s10346-017-0879-7, 2018.
Conrad, J. L., Morphew, M., Baum, R. L., and Mirus, B. B.: HydroMet: A new code for automated objective optimization of hydrometeorological thresholds for landslide initiation, Water, 13, 1752, https://doi.org/10.3390/w13131752, 2021.
Crozier, M. J.: Prediction of rainfall-triggered landslides: A test of the Antecedent Water Status Model, Earth Surf. Processes, 24, 825–833, https://doi.org/10.1002/(SICI)1096-9837(199908)24:9<825::AID-ESP14>3.0.CO;2-M, 1999.
Darrow, M. M., Nelson, V. A., Grilliot, M., Wartman, J., Jacobs, A., Baichtal, J. F., and Buxton, C.: Geomorphology and initiation mechanisms of the 2020 Haines, Alaska landslide, Landslides, 19, 2177–2188, https://doi.org/10.1007/s10346-022-01899-3, 2022.
De Vita, P., Napolitano, E., Godt, J., and Baum, R.: Deterministic estimation of hydrological thresholds for shallow landslide initiation and slope stability models: case study from the Somma-Vesuvius area of southern Italy, Landslides, 10, 713–728, https://doi.org/10.1007/s10346-012-0348-2, 2013.
Distefano, P., Peres, D. J., Piciullo, L., Palazzolo, N., Scandura, P., and Cancelliere, A.: Hydro-meteorological landslide triggering thresholds based on artificial neural networks using observed precipitation and ERA5-Land soil moisture, Landslides, 20, 2725–2739, https://doi.org/10.1007/s10346-023-02132-5, 2023.
Ebel, B. A., Loague, K., Montgomery, D. R., and Dietrich, W. E.: Physics-based continuous simulation of long-term near-surface hydrologic response for the Coos Bay experimental catchment, Water Resour. Res., 44, W07417, https://doi.org/10.1029/2007WR006442, 2008.
Ehret, U., Gupta, H. V., Sivapalan, M., Weijs, S. V., Schymanski, S. J., Blöschl, G., Gelfan, A. N., Harman, C., Kleidon, A., Bogaard, T. A., Wang, D., Wagener, T., Scherer, U., Zehe, E., Bierkens, M. F. P., Di Baldassarre, G., Parajka, J., van Beek, L. P. H., van Griensven, A., and Westhoff, M. C.: Advancing catchment hydrology to deal with predictions under change, Hydrol. Earth Syst. Sci., 18, 649–671, https://doi.org/10.5194/hess-18-649-2014, 2014.
Fatichi, S., Or, D., Walko, R., Vereecken, H., Young, M. H., Ghezzehei, T. A., Hengl, T., Kollet, S., Agam, N., and Avissar, R.: Soil structure is an important omission in Earth System Models, Nat. Commun., 11, 522, https://doi.org/10.1038/s41467-020-14411-z, 2020.
Felsberg, A., De Lannoy, G. J. M., Girotto, M., Poesen, J., Reichle, R. H., and Stanley, T.: Global soil water estimates as landslide predictor: The effectiveness of SMOS, SMAP, and GRACE observations, land surface simulations, and data assimilation, J. Hydrometeorol., 22, 1065–1084, https://doi.org/10.1175/JHM-D-20-0228.1, 2021.
Francke, T., Heistermann, M., Köhli, M., Budach, C., Schrön, M., and Oswald, S. E.: Assessing the feasibility of a directional cosmic-ray neutron sensing sensor for estimating soil moisture, Geosci. Instrum. Method. Data Syst., 11, 75–92, https://doi.org/10.5194/gi-11-75-2022, 2022.
Fusco, F., Bordoni, M., Tufano, R., Vivaldi, V., Meisina, C., Valentino, R., Bittelli, M., and De Vita, P.: Hydrological regimes in different slope environments and implications on rainfall thresholds triggering shallow landslides, Nat. Hazards, 114, 907–939, https://doi.org/10.1007/s11069-022-05417-5, 2022.
Gariano, S. L. and Guzzetti, F.: Landslides in a changing climate, Earth-Sci. Rev., 162, 227–252, https://doi.org/10.1016/J.EARSCIREV.2016.08.011, 2016.
Glade, T., Crozier, M., and Smith, P.: Applying probability determination to refine landslide-triggering rainfall thresholds using an empirical “antecedent daily rainfall model”, Pure Appl. Geophys., 157, 1059–1079, https://doi.org/10.1007/s000240050017, 2000.
Godt, J. W., Baum, R. L., and Chleborad, A. F.: Rainfall characteristics for shallow landsliding in Seattle, Washington, USA, Earth Surf. Processes, 31, 97–110, https://doi.org/10.1002/esp.1237, 2006.
Godt, J. W., Baum, R. L., and Lu, N.: Landsliding in partially saturated materials, Geophys. Res. Lett., 36, 1–5, https://doi.org/10.1029/2008GL035996, 2009.
Graham, C. B. and Lin, H. S.: Controls and frequency of preferential flow occurrence: A 175-event analysis, Vadose Zone J., 10, 816–831, https://doi.org/10.2136/vzj2010.0119, 2011
Greco, R., Marino, P., and Bogaard, T. A.: Recent advancements of landslide hydrology, WIREs Water, 10, e1675, https://doi.org/10.1002/wat2.1675, 2023.
Guzzetti, F., Gariano, S. L., Peruccacci, S., Brunetti, M. T., Marchesini, I., Rossi, M., and Melillo, M.: Geographical landslide early warning systems, Earth-Sci. Rev., 200, 102973, https://doi.org/10.1016/j.earscirev.2019.102973, 2020.
Guzzetti, F., Peruccacci, S., Rossi, M., and Stark, C. P.: The rainfall intensity-duration control of shallow landslides and debris flows: An update, Landslides, 5, 3–17, https://doi.org/10.1007/s10346-007-0112-1, 2008.
Handwerger, A. L., Fielding, E. J., Huang, M. H., Bennett, G. L., Liang, C., and Schulz, W. H.: Widespread initiation, reactivation, and acceleration of landslides in the northern California Coast Ranges due to extreme rainfall, J. Geophys. Res.-Earth, 124, 1782–1797, https://doi.org/10.1029/2019JF005035, 2019.
Hinds, E., Lu, N., Mirus, B. B., and Wayllace, A.: Effects of Infiltration Characteristics on Spatial-Temporal Evolution of Stability of an Interstate Highway Embankment, J. Geotech. Geoenviron., 145, 0501908, https://doi.org/10.1061/(ASCE)GT.1943-5606.0002127, 2019.
Huffman, G. J., Bolvin, D. T., Braithwaite, D., Hsu, K., Joyce, R., Xie, P., and Yoo, S. H.: NASA global precipitation measurement (GPM) integrated multi-satellite retrievals for GPM (IMERG), Algorithm theoretical basis document (ATBD) version, https://gpm.nasa.gov/sites/default/files/2020-05/IMERG_ATBD_V06.3.pdf (last access: 6 December 2024), 2015.
Illien, L., Andermann, C., Sens-Schönfelder, C., Cook, K. L., Baidya, K. P., Adhikari, L. B., and Hovius, N.: Subsurface moisture regulates Himalayan groundwater storage and discharge, AGU Advances, 2, e2021AV00039, https://doi.org/10.1029/2021AV000398, 2021.
Innes, J. L.: Debris flows, Prog. Phys. Geog., 7, 469–501, 1983.
Jakob, M.: Chap. 14 – Landslides in a changing climate, in: Hazards and Disasters Series, Landslide Hazards, Risks, and Disasters, 2nd Edn., edited by: Davies, T., Rosser, N., and Shroder, J. F., Elsevier, 505–579, ISBN 9780128184646, https://doi.org/10.1016/B978-0-12-818464-6.00003-2, 2022.
Kirschbaum, D. and Stanley, T.: Satellite-based assessment of rainfall-triggered landslide hazard for situational awareness, Earth's Future, 6, 505–523, https://doi.org/10.1002/2017EF000715, 2018.
Kirschbaum, D., Kapnick, S. B., Stanley, T., and Pascale, S.: Changes in extreme precipitation and landslides over High Mountain Asia, Geophys. Res. Lett., 47, e2019GL085347, https://doi.org/10.1029/2019GL085347, 2020.
Lehmann, P. and Or, D.: Hydromechanical triggering of landslides: From progressive local failures to mass release, Water Resour. Res., 48, W03535, https://doi.org/10.1029/2011WR010947, 2012.
Lehmann, P., von Ruette, J., and Or, D.: Deforestation effects on rainfall-induced shallow landslides: remote sensing and physically-based modelling, Water Resour. Res., 55, 9962–9976, https://doi.org/10.1029/2019WR025233, 2019.
Lehmann, P., Leshchinsky, B., Mirus, B. B., Lu, N., Gupta, S., and Or, D.: Clays are not created equal – effects of clay mineral type on soil parameters, Geophys. Res. Lett., 48, e2021GL095311, https://doi.org/10.1029/2021GL095311, 2021.
Liang, W.-L.: Dynamics of pore water pressure at the soil–bedrock interface recorded during a rainfall-induced shallow landslide in a steep natural forested headwater catchment, Taiwan. J. Hydrol., 587, 125003, https://doi.org/10.1016/j.jhydrol.2020.125003, 2020.
Loague, K., Heppner, C. S., Mirus, B. B., Ebel, B. A., Ran, Q., Carr, A. E., BeVille, S. H., and VanderKwaak, J. E.: Physics-based hydrologic-response simulation: Foundation for hydroecology and hydrogeomorphology, Hydrol. Process., 20, 1231–1237, https://doi.org/10.1002/hyp.6179, 2006.
Lombardo, L., Opitz, T., Ardizzone, F., Guzzetti, F., and Huser, R.: Space-time landslide predictive modelling, Earth-Sci. Rev., 209, 103318, https://doi.org/10.1016/j.earscirev.2020.103318, 2020.
Lu, N. and Godt, J. W.: Hillslope hydrology and stability, Cambridge University Press, ISBN:9781139108164, https://doi.org/10.1017/CBO9781139108164, 2013.
Luna, L. V. and Korup, O.: Seasonal landslide activity lags annual precipitation pattern in the Pacific Northwest, Geophys. Res. Lett., 49, e2022GL098506, https://doi.org/10.1029/2022GL098506, 2022.
Marc, O., Oliveira, R. A. J., Gosset, M., Emberson, R., and Malet, J. P.: Global assessment of the capability of satellite precipitation products to retrieve landslide-triggering extreme rainfall events, Earth Interact., 26, 122–138, https://doi.org/10.1175/EI-D-21-0022.1, 2022.
Marino, P., Peres, D. J., Cancelliere, A., Greco, R., and Bogaard, T. A.: Soil moisture information can improve shallow landslide forecasting using the hydrometeorological threshold approach, Landslides, 17, 2041–2054, https://doi.org/10.1007/s10346-020-01420-8, 2020.
Marino, P., Santonastaso, G. F., Fan, X., and Greco, R.: Prediction of shallow landslides in pyroclastic-covered slopes by coupled modeling of unsaturated and saturated groundwater flow, Landslides, 18, 31–41, https://doi.org/10.1007/s10346-020-01484-6, 2021.
Marino, P., Siva, S., Subramanian, S., Fan, X., and Greco, R.: Changes in debris-flow susceptibility after the Wenchuan earthquake revealed by meteorological and hydro-meteorological thresholds, Catena, 210, 105929, https://doi.org/10.1016/j.catena.2021.105929, 2022.
Mirus, B. B.: Evaluating the importance of characterizing soil structure and horizons in parameterizing a hydrologic process model, Hydrol. Process., 29, 4611–4623, https://doi.org/10.1002/hyp.10592, 2015.
Mirus, B. B., Smith, J. B., and Baum, R. L.: Hydrologic impacts of landslide disturbances: Implications for remobilization and hazard persistence, Water Resour. Res., 53, 8250–8265, https://doi.org/10.1002/2017WR020842, 2017.
Mirus, B. B., Becker, R. E., Baum, R. L., and Smith, J. B.: Integrating real-time subsurface hydrologic monitoring with empirical rainfall thresholds to improve landslide early warning, Landslides, 15, 1909–1919, https://doi.org/10.1007/s10346-018-0995-z, 2018.
Mondini, A. C., Guzzetti, F., and Melillo, M.: Deep learning forecast of rainfall-induced shallow landslides, Nat. Commun., 14, 2466, https://doi.org/10.1038/s41467-023-38135-y, 2023.
Montgomery, D. R. and Dietrich, W. E.: A physically based model for the topographic control on shallow landsliding, Water Resour. Res., 30, 1153–1171, https://doi.org/10.1029/93WR02979, 1994.
Montgomery, D. R., Schmidt, K. M., Dietrich, W. E., and McKean J.: Instrumental record of debris flow initiation during natural rainfall: Implications for modeling slope stability, J. Geophys. Res., 114, F01031, https://doi.org/10.1029/2008JF001078, 2009.
Mostbauer, K., Kaitna, R., Prenner, D., and Hrachowitz, M.: The temporally varying roles of rainfall, snowmelt and soil moisture for debris flow initiation in a snow-dominated system, Hydrol. Earth Syst. Sci., 22, 3493–3513, https://doi.org/10.5194/hess-22-3493-2018, 2018.
Napolitano, E., Fusco, F., Baum, R. L., Godt, J. W., and De Vita, P.: Effect of antecedent–hydrological conditions on rainfall triggering of debris flows in ash–fall pyroclastic mantled slopes of Campania (southern Italy), Landslides, 13, 967–983, https://doi.org/10.1007/s10346-015-0647-5, 2016.
Nicolazzo, J. A., Wikstrom Jones, K. M., Salisbury, J. B., and Horen, K. C.: Post-landslide elevation changes detected from multi-temporal lidar surveys of the November 2023 Wrangell, Alaska, landslides: Alaska Division of Geological and Geophysical Surveys Preliminary Interpretive Report 2024-2, Alaska Division of Geological & Geophysical Surveys, 22 pp., https://doi.org/10.14509/31124, 2024.
Nimmo, J. R.: Preferential flow occurs in unsaturated conditions, Hydrol. Process., 26, 786–789, https://doi.org/10.1002/hyp.8380, 2012.
Nimmo, J. R.: Quantitative Framework for Preferential Flow Initiation and Partitioning, Vadose Zone J., 15, 1–12, https://doi.org/10.2136/vzj2015.05.0079, 2016.
Nimmo, J. R., Perkins, K., Pamplin, M. R., Ebel, B. A., Walvoord, M., and Mirus, B. B.: Rapid-response unsaturated zone hydrology: Small-scale data, small-scale theory, big problems, Front. Earth Sci., 9, 613564, https://doi.org/10.3389/feart.2021.613564, 2021.
Orland, E., Roering, J. J., Thomas, M. A., and Mirus, B. B.: Deep learning as a tool to forecast hydrologic response for landslide-prone hillslopes, Geophys. Res. Lett., 47, e2020GL088731, https://doi.org/10.1029/2020GL088731, 2020.
Palazzolo, N., Peres, D. J., Creaco, E., and Cancelliere, A.: Using principal component analysis to incorporate multi-layer soil moisture information in hydrometeorological thresholds for landslide prediction: an investigation based on ERA5-Land reanalysis data, Nat. Hazards Earth Syst. Sci., 23, 279–291, https://doi.org/10.5194/nhess-23-279-2023, 2023.
Parker, R., Hales, T., Mudd, S., Grieve, S. W. D., and Constantine, J. A.: Colluvium supply in humid regions limits the frequency of storm-triggered landslides, Sci. Rep., 6, 34438, https://doi.org/10.1038/srep34438, 2016.
Patton, A. I., Luna, L. V., Roering, J. J., Jacobs, A., Korup, O., and Mirus, B. B.: Landslide initiation thresholds in data-sparse regions: application to landslide early warning criteria in Sitka, Alaska, USA, Nat. Hazards Earth Syst. Sci., 23, 3261–3284, https://doi.org/10.5194/nhess-23-3261-2023, 2023.
Pecoraro, G. and Calvello, M.: Integrating local pore water pressure monitoring in territorial early warning systems for weather-induced landslides, Landslides, 18, 1191–1207, https://doi.org/10.1007/s10346-020-01599-w, 2021.
Perkins, J. P., Oakley, N. S., Collins, B. D., Corbett, S. C., and Burgess, W. P.: Characterizing the scale of regional landslide triggering from storm hydrometeorology, EGUsphere [preprint], https://doi.org/10.5194/egusphere-2024-873, 2024.
Perrone, A., Lapenna, V., and Piscitelli, S.: Electrical resistivity tomography technique for landslide investigation: A review, Earth-Sci. Rev., 135, 65–82, https://doi.org/10.1016/j.earscirev.2014.04.002, 2014.
Piciullo, L., Calvello, M., and Cepeda, J. M.: Territorial early warning systems for rainfall-induced landslides, Earth-Sci. Rev., 179, 228–247, https://doi.org/10.1016/j.earscirev.2018.02.013, 2018.
Piciullo, L., Tiranti, D., Pecoraro, G., and Calvello, M.: Standards for the performance assessment of territorial landslide early warning systems, Landslides, 17, 2533–2546, https://doi.org/10.1007/s10346-020-01486-4, 2020.
Piciullo, L., Capobianco, V., and Heyerdahl, H.: A first step towards a IoT-based local early warning system for an unsaturated slope in Norway, Nat Hazards, 114, 3377–3407, https://doi.org/10.1007/s11069-022-05524-3, 2022.
Richards, L. A.: Capillary conduction of liquids through porous mediums, Physics, 1, 318–333, https://doi.org/10.1063/1.1745010, 1931.
Roman Quintero, D. C., Marino, P., Santonastaso, G. F., and Greco, R.: Understanding hydrologic controls of sloping soil response to precipitation through machine learning analysis applied to synthetic data, Hydrol. Earth Syst. Sci., 27, 4151–4172, https://doi.org/10.5194/hess-27-4151-2023, 2023.
Saito, H., Nakayama, D., and Matsuyama, H.: Relationship between the initiation of a shallow landslide and rainfall intensity-duration thresholds in Japan, Geomorphology, 118, 167–175, https://doi.org/10.1016/j.geomorph.2009.12.016, 2010.
Sassa, K., Canuti, P., Bobrowsky, P., and Casagli, N.: International Consortium on Landslides: From IDNDR, IGCP, UNITWIN, WCDRR 2 and 3 to Kyoto Landslide Commitment 2020, in: Progress in Landslide Research and Technology, edited by: Sassa, K., Konagai, K., Tiwari, B., Arbanas, Ž., and Sassa, S., Progress in Landslide Research and Technology, Springer, Cham, https://doi.org/10.1007/978-3-031-16898-7_2, 2023.
Segoni, S., Piciullo, L., and Gariano, S. L.: A review of the recent literature on rainfall thresholds for landslide occurrence, Landslides, 15, 1483–1501, https://doi.org/10.1007/s10346-018-0966-4, 2018.
Sidle, R. C. and Swanston, D. N.: Analysis of a small debris slide in coastal Alaska, Can. Geotech. J., 19, 167–174, https://doi.org/10.1139/t82-018, 1982.
Sidle, R. C. and Tsuboyama, Y.: A comparison of piezometric response in unchanneled hillslope hollows: Coastal Alaska and Japan, J. Jpn. Soc. Hydrol. Water Res., 5, 3–11, https://doi.org/10.3178/jjshwr.5.3_3, 1992.
Sidle, R. C., Gomi, T., Usuga, J. C. L., and Jarihani, B.: Hydrogeomorphic processes and scaling issues in the continuum from soil pedons to catchments, Earth-Sci. Rev., 175, 75–96, https://doi.org/10.1016/j.earscirev.2017.10.010, 2017.
Smith, J. B., Bedinger, E., Staley, D. M, Baum, R. L., and Mirus, B. B.: Hydrologic monitoring data in steep, landslide-prone terrain, Sitka, Alaska, USA, U.S. Geological Survey Data Release [data set], https://doi.org/10.5066/P9GFA6PC, 2023.
SSURGO: Soil Survey Staff, Natural Resources Conservation Service, United States Department of Agriculture, Soil Survey Geographic Database, https://sdmdataaccess.sc.egov.usda.gov, last access: 22 April 2024.
Stähli, M., Sättele, M., Huggel, C., McArdell, B. W., Lehmann, P., Van Herwijnen, A., Berne, A., Schleiss, M., Ferrari, A., Kos, A., Or, D., and Springman, S. M.: Monitoring and prediction in early warning systems for rapid mass movements, Nat. Hazards Earth Syst. Sci., 15, 905–917, https://doi.org/10.5194/nhess-15-905-2015, 2015.
Stanley, T. A., Kirschbaum, D. B., Benz, G., Emberson, R. A., Amatya, P. M., Medwedeff, W., and Clark, M. K.: Data-driven landslide nowcasting at the global scale, Front. Earth Sci., 9, 640043, https://doi.org/10.3389/feart.2021.640043, 2021.
Steger, S., Moreno, M., Crespi, S., Gariano, S. L., Brunetti, M. T., Massimo Melillo, M., Peruccacci, S., Francesco Marra, F., de Vugt, L., Zieher, T., Rutzinger, M., Mair, V., and Pittore, M.: Adopting the margin of stability for space–time landslide prediction – A data-driven approach for generating spatial dynamic thresholds, Geosci. Front., 15, 101822, https://doi.org/10.1016/j.gsf.2024.101822, 2024.
Terlien, M. T. J.: Hydrological landslide triggering in ash-covered slopes of Manizales (Colombia), Geomorphology, 20, 165–175, https://doi.org/10.1016/S0169-555X(97)00022-6, 1997.
Terzaghi, K.: Theoretical Soil Mechanics, Wiley, New York, https://doi.org/10.1002/9780470172766, 1943.
Thomas, M. A., Mirus, B. B., and Collins, B. D.: A physics-based approach to identify thresholds for rainfall-induced shallow landsliding, Geophys. Res. Lett., 45, 9651–9661, https://doi.org/10.1029/2018GL079662, 2018.
Thomas, M. A., Collins, B. D., and Mirus, B. B.: Assessing the feasibility of satellite-based thresholds for hydrologically driven landsliding, Water Resour. Res., 55, 9006–9023, https://doi.org/10.1029/2019WR025577, 2019.
Thomas, M. A., Mirus, B. B., and Smith, J. B.: Hillslopes in humid-tropical climates aren't always wet: Implications for hydrologic response and landslide initiation in Puerto Rico, Hydrol. Process., 34, 4307–4318, https://doi.org/10.1002/hyp.13885, 2020.
Torres, R., Dietrich, W. E., Montgomery, D. R., Anderson, S. P., and Loague, K.: Unsaturated zone processes and the hydrologic response of a steep, unchanneled catchment, Water Resour. Res., 34, 1865–1879, https://doi.org/10.1029/98WR01140, 1998.
Tromp-van Meerveld, H. J. and McDonnell, J. J.: Threshold relations in subsurface stormflow: II. The fill and spill hypothesis, Water Resour. Res., 42, 1–11, https://doi.org/10.1029/2004WR003800, 2006.
Uhlemann, S., Chambers, J., Wilkinson, P., Maurer, H., Merritt, A., Meldrum, P., Kuras, O., Gunn, D., Smith, A., and Dijkstra, T.: Four-dimensional imaging of moisture dynamics during landslide reactivation, J. Geophys. Res.-Earth, 122, 398–418, https://doi.org/10.1002/2016JF003983, 2017.
US Geological Survey: 3D Elevation Program (3DEP) USGS 1/3 arc-second DEM [Data set], https://www.usgs.gov/3d-elevation-program/about-3dep-products-services (last access: 22 April 2024), 2019.
Uwihirwe, J., Hrachowitz, M., and Bogaard, T.: Integration of observed and model-derived groundwater levels in landslide threshold models in Rwanda, Nat. Hazards Earth Syst. Sci., 22, 1723–1742, https://doi.org/10.5194/nhess-22-1723-2022, 2022.
Van Beek, L. P. H.: Assessment of the influence of changes in climate and land use on landslide activity in a Mediterranean environment, Doctoral Thesis, Netherlands Geographical Studies 294, Utrecht, https://dspace.library.uu.nl/handle/1874/560 (last access: 6 Decembe 2024), 2002.
Van Looy, K., Bouma, J., Herbst, M., Koestel, J., Minasny, B., Mishra, U., Montzka, C., Nemes, A., Pachepsky, Y. A., Padarian, J., Schaap, M. G., Tóth, B., Verhoef, A., Vanderborght, J., van der Ploeg, M. J., Weihermüller, L., Zacharias, S., Zhang, Y., and Vereecken, H.: Pedotransfer functions in Earth system science: Challenges and perspectives, Rev. Geophys., 55, 1199–1256, 2017.
Wayllace, A., Thunder, B., Lu, N., Khan, A., and Godt, J. W.: Hydrological behavior of an infiltration-induced landslide in Colorado, USA, GeoFluids, 2019, 659303, https://doi.org/10.1155/2019/1659303, 2019.
Wei, X., Fan, W., Cao, Y., Chai, X., Bordoni, M., Meisina, C., and Li, J.: Integrated experiments on field monitoring and hydro-mechanical modeling for determination of a triggering threshold of rainfall-induced shallow landslides: A case study in Ren River catchment, China, Bull. Eng. Geol. Environ., 79, 513–532, https://doi.org/10.1007/s10064-019-01570-7, 2020.
Wei, Z., Lü, Q., Sun, H., and Shang, Y.: Estimating the rainfall threshold of a deep-seated landslide by integrating models for predicting the groundwater level and stability analysis of the slope, Eng. Geol., 253, 14–26, https://doi.org/10.1016/j.enggeo.2019.02.026, 2019.
Wicki, A., Lehmann, P., Hauck, C., Seneviratne, S. I., Waldner, P., and Stähli, M.: Assessing the potential of soil moisture measurements for regional landslide early warning, Landslides, 17, 1881–1896, https://doi.org/10.1007/s10346-020-01400-y, 2020.
Wicki, A., Jansson, P.-E., Lehmann, P., Hauck, C., and Stähli, M.: Simulated or measured soil moisture: which one is adding more value to regional landslide early warning?, Hydrol. Earth Syst. Sci., 25, 4585–4610, https://doi.org/10.5194/hess-25-4585-2021, 2021.
Wicki, A., Lehmann, P., Hauck, C., and Stähli, M.: Impact of topography on in situ soil wetness measurements for regional landslide early warning – a case study from the Swiss Alpine Foreland, Nat. Hazards Earth Syst. Sci., 23, 1059–1077, https://doi.org/10.5194/nhess-23-1059-2023, 2023.
Wilson, R. C. and Wieczorek, G. F.: Rainfall thresholds for the initiation of debris flows at La Honda, California, Environ. Eng. Geosci., 1, 11–27, https://doi.org/10.2113/gseegeosci.I.1.11, 1995.
Zhao, B., Dai, Q., Han, D., Dai, H., Mao, J., and Zhuo, L.: Probabilistic thresholds for landslides warning by integrating soil moisture conditions with rainfall thresholds, J. Hydrol., 574, 276–287, https://doi.org/10.1016/j.jhydrol.2019.04.062, 2019.
Zhao, B., Dai, Q., Zhuo, L., Zhu, S, Shen, Q., and Han, D.: Assessing the potential of different satellite soil moisture products in landslide hazard assessment, Remote Sens. Environ., 264, 112583, https://doi.org/10.1016/j.rse.2021.112583, 2021.
Zimmer, M. A. and Gannon, J. P.: Run-off processes from mountains to foothills: The role of soil stratigraphy and structure in influencing run-off characteristics across high to low relief landscapes, Hydrol. Process., 32, 1546–1560, https://doi.org/10.1002/hyp.11488, 2018.
- Abstract
- Subsurface hydrologic information improves landslide forecasting
- Understanding the current limits to hydrometeorological thresholds
- Towards improved landslide forecasting models
- Data availability
- Author contributions
- Competing interests
- Disclaimer
- Acknowledgements
- Review statement
- References
- Abstract
- Subsurface hydrologic information improves landslide forecasting
- Understanding the current limits to hydrometeorological thresholds
- Towards improved landslide forecasting models
- Data availability
- Author contributions
- Competing interests
- Disclaimer
- Acknowledgements
- Review statement
- References