the Creative Commons Attribution 4.0 License.
the Creative Commons Attribution 4.0 License.
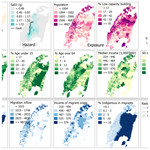
Migration as a hidden risk factor in seismic fatality: spatial modeling of the Chi-Chi earthquake and suburban syndrome
Kuan-Hui Elaine Lin
Thung-Hong Lin
Gee-Yu Liu
Chin-Hsun Yeh
Diana Maria Ceballos
Suburban areas have experienced disproportionately more fatalities during major earthquakes. Place-based models attribute this spatial disparity to hazard, exposure, and social-vulnerability factors. However, the impact of migration on seismic fatality remains underexplored, primarily due to the challenges of accessing mobility data. In this study, we apply a geospatial method, the radiation model, to estimate migration patterns as a critical component of exposure and vulnerability. Analyzing the 1999 Chi-Chi earthquake in Taiwan with Poisson regression across 4052 neighborhoods, we factor in migration inflow (i.e., population traveling from other neighborhoods), income of the migrants' origin, and the indigenous-population percentage among migrants, along with other risk factors proven in previous studies. Our findings indicate that migration inflow significantly correlates with an increased number of fatalities. Furthermore, a lower income in the neighborhood of the migrants' origin is significantly associated with more fatalities at their destination. An elevated proportion of the indigenous population in the migrants' original neighborhood also significantly correlates with an increased number of fatalities, although the impact of the Chi-Chi earthquake does not predominantly affect indigenous jurisdictions. This study underscores the seismic-fatality risk on the outskirts of megacities, where migrants from lower-income and historically marginalized groups are more likely to reside for precarious employment, emphasizing the need for affordable and safe living infrastructure for the migrating population. Addressing migrants' vulnerabilities in housing will not only reduce seismic-fatality risk but also improve preparedness against other disasters and public health emergencies.
- Article
(5173 KB) - Full-text XML
- BibTeX
- EndNote
Globally, from 1996 to 2015, earthquakes resulted in over 750 000 deaths, comprising 55.6 % of all natural hazard fatalities in this period (UNISDR, 2016). Seismic fatalities, while intuitively linked to earthquake exposure, do not always align with population density or hazard magnitude. For example, during Taiwan's 1999 Chi-Chi earthquake, most fatalities occurred not in densely populated urban centers or remote rural areas but in suburban or urban-fringe areas around the Taichung metropolitan region (Fig. 1). Similar mortality patterns were observed in subsequent significant earthquakes in Wenchuan, China (2008); Central Chile (2010); and Gorkha, Nepal (2015), predominantly affecting suburbs or neighborhoods of small to medium-sized cities (Adhikari et al., 2021; Allan et al., 2013; Panday et al., 2021; Xu et al., 2009). This pattern, which we term the “suburban syndrome”, suggests that the highest fatality rates may be more related to urban development and housing safety issues than to the earthquake's magnitude alone. Nevertheless, this socio-spatial fatality pattern in suburban belts has been underexplored in seismic-risk research.
By 2030, small and medium-sized cities and towns with populations of less than 1 million are expected to account for 54 % of the world's urban population, having experienced rapid growth and urban expansion in recent decades (UN, 2018). These cities, particularly those on the outskirts of metropolitan areas, often provide more affordable housing options for lower-income groups, including migrant workers attracted to cities for job opportunities (Taubenböck et al., 2018). During off-season periods for agriculture, rural populations wandering into urban areas frequently take on temporary jobs, such as construction work (Fan and Li, 2020; Kumar and Sati, 2023). Socioeconomically marginalized migrants from rural villages and indigenous tribes are more likely to reside in urban peripheries where lower-cost rental housing is available, though often at the expense of structure safety and maintenance (Andersen et al., 2018; Belanger et al., 2013; Shier et al., 2015). Social vulnerabilities in these areas are exacerbated by issues like unregulated development, informal settlements, overcrowded living conditions, poor construction standards, weak governance, and limited financial resources (Birkmann et al., 2016). These factors collectively heighten the social vulnerability of suburban belts to earthquakes.
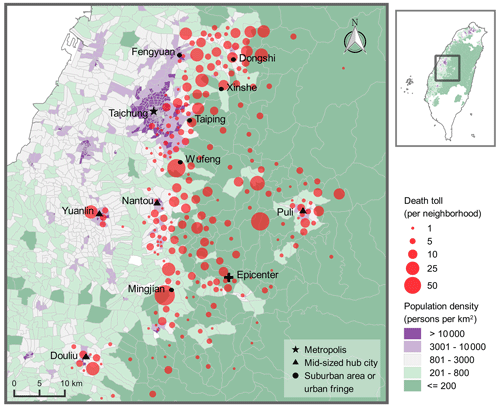
Figure 1A case of the suburban syndrome in seismic fatalities. Spatial distributions of fatalities and population density indicate that the neighborhoods in medium-sized hub cities, suburban areas, and urban fringes suffered the most fatalities during the Chi-Chi earthquake. Population data are from the Taiwan Population and Household Census of 2000, and fatality data are from Tien et al. (2002).
Seismic-risk assessment has primarily focused on individuals' places of residence to estimate exposure and other risk factors (Aldrich and Sawada, 2015; Derakhshan et al., 2020; Lin et al., 2015; Masi et al., 2021; Pavić et al., 2020; Rashed and Weeks, 2003). These measures were able to identify areas with higher exposure and social vulnerability associated with earthquake-related fatalities, thereby informing resource allocation and policy interventions. However, the residence-based approaches overlook the effect of migration patterns on daily, seasonal, and long-term scales that can have a strong impact on reshaping risks. More critically, the migration data hardly are reflected in any official population registers. We argue that migration patterns are a vital yet overlooked component in understanding the suburban syndrome in disaster-risk assessment. Ignoring population flow between places can lead to an underestimation of the exposure, root cause, and progression of vulnerability to environmental hazards (Kumar and Sati, 2023; Wang et al., 2022). For example, seasonal farm workers in North Carolina, USA, faced greater impacts from Hurricane Katrina due to inadequate housing in migrant worker camps (Montz et al., 2011). In Lisbon, Portugal, more than 50 % of the population is found in different census locations during the day compared to their residential records, resulting in a 22 % higher exposure to the 2012 Lisbon earthquake (Freire and Aubrecht, 2012). Between 1990 and 2010, the migration of the working-age population from rural to urban areas in China led to a 34 % increase in the population exposed to seismically hazardous areas, higher than the average population growth of 18 % (He et al., 2016). In the United States, exposure to toxic sites is 10 % higher when based on people's mobile signal locations than estimates based on their residence (Liu et al., 2023). These examples underscore the critical role of migration patterns in hazard exposure, necessitating a paradigm shift towards mobility-based risk assessments.
In this study, we hypothesize that incorporating the migration effect into risk models could explain the suburban syndrome, characterized by more fatalities in small and medium-sized cities and suburban areas. We also hypothesize that migrants from historically marginalized groups, especially indigenous people in colonial societies, may be exposed to higher seismic risks. Mobility data from recent technological advancements, such as social media records and mobile phone signals, were not widely available before the 2000s. Thus, we introduce the application of the radiation model (Simini et al., 2012) to estimate migration effects on seismic risks. The effect is examined through a case study of the Chi-Chi earthquake that struck Taiwan in 1999. The database for this earthquake encompasses the location of each death and high-resolution ground motion data from 650 stations. By combining earthquake hazard, socioeconomic, indigenous-population data, and migration estimates at the neighborhood level, we construct three nested multivariate models. Our analyses address three questions. (i) What are the determinant factors constituting seismic risk in terms of hazard, exposure, and vulnerability? (ii) What role do migration patterns play in seismic fatalities with respect to the size of the migrant population and the economic status of their origin? (iii) Are historically marginalized populations, especially indigenous people, more vulnerable during migration in terms of seismic risk?
Background for place-based and mobility-based effects in the suburban syndrome
When performing seismic-risk assessment, studies usually determine risk factors of a given place by measuring three distinctive components that determine fatality – hazard, exposure, and vulnerability (Bilham and Gaur, 2013; Lin et al., 2015). Hazard refers to natural or human-induced physical events that may have adverse effects on exposed elements, such as populations or buildings. Exposure refers to an inventory of elements in an area in which hazard events may occur. In seismic-risk assessments, while “vulnerability” sometimes refers to the fragility of buildings, we specifically apply this term to social vulnerability, which reflects the sociodemographic context of a neighborhood that is prone to suffering seismic fatalities (IPCC, 2012; Turner et al., 2003). Following the assumption that living in a poor neighborhood affects a wide range of individual outcomes (Wilson, 2012), the place-based effect refers to the internal physical and socioeconomic context of a neighborhood in which hazard, exposure, and vulnerability interact to shape its risk profile. In addition, the mobility-based effect, as we define it here, refers to interactions of the outer linkages of a neighborhood with its migrants from other neighborhoods that affect the risk profile, but their mobility has been underestimated. These two mechanisms increase the seismic risk in developing and densely populated neighborhoods along the expanding suburban belt – suburban areas, the urban fringe, and local hub cities and towns.
The place-based effect appears to be magnified when hazard, exposure, and vulnerability are considered to reinforce each other, and fatalities are higher in certain neighborhoods. However, investigations of these phenomena remain insufficient. Seismic-risk studies most frequently report the concentration of disadvantaged or vulnerable populations living in poorly constructed buildings (e.g., low-rent or low-price residential and commercial property or slums) in seismic-hazard-prone areas. During the past 4 decades, in particular construction booms have been witnessed in cities worldwide, resulting in an unprecedented increase in the building stock constructed using inferior materials and assembly methods (Bilham and Gaur, 2013). Most such buildings occur in developing countries or regions where building standards were poorly regulated or enforced. It has been determined that earthquake-resistant buildings are often exclusive to economically developed neighborhoods. Expensive real estate with a high standard for building codes is usually not affordable for the socially disadvantaged population (i.e., low-income, unemployed, and minority people; transient migrant or commuting workers; and people who are less educated) in earthquake zones (Önder et al., 2004). Overall, corruption in the construction industry; the absence of education about earthquakes; the prevalence of poverty; and income inequality, which relates to segregation in earthquake zones, are responsible for fatalities associated with building collapses (Anbarci et al., 2005; Escaleras et al., 2007). There are still other factors that can heighten social and geographical segregation during earthquakes. Public or social housing is often built on newly derived lands that are prone to various types of hazard threats, such as those close to transportation, hillsides, riverbanks, or coasts (Cutter et al., 2006). Proximity to industrial sites during an earthquake can increase the risk of explosions and fires, potentially leading to fatalities (Moghaddam et al., 2023). The spatial segregation of building quality and unequal exposure to hazards can be worsened under rapid and unregulated urban expansion (Brouwer et al., 2007; Lavell, 2003; McGranahan et al., 2007). Therefore, particularly during building booms, the rapidly developing yet relatively poor neighborhoods around the suburban belt could therefore face greater exposure to hazards with a higher proportion of buildings with low-quality codes that enhance seismic risks.
Another mechanism, the mobility-based effect, which focuses on human mobility across neighborhood boundaries, could profoundly influence earthquake fatalities through migration behavior. Human mobility shapes population dynamics at different timescales from a day to several seasons in a year. The simplest view of human mobility is the “push–pull” theory, which states that a pull-in force occurs in places with more favorable conditions and opportunities that attract migration, whereas a push-out force occurs in places with fewer opportunities and more constraints that force people to move out of those places (Ravenstein, 1889). These processes cause short-term population flow (e.g., commuting daily to work, weeks/months in temporary jobs, and semesters/years at school) and long-term migration; these population flows can take place in both formal and informal forms. Research indicates that the city scale (population or accumulated goods) and job opportunities are critical factors of a pull process (Jamshed et al., 2020; Simini et al., 2012). The gravity model, for example, uses two elements – population number and distance between places – to predict population flows (Simini et al., 2012; Viboud et al., 2006). Survey studies also find that low family income (Fell et al., 2004), unemployment (Böheim and Taylor, 2002), and the cost of commuting are key factors in a push process. All of this dynamism forms the prototype of the relationships in urban systems and urban–rural linkages (Dicken et al., 2001; Jessop et al., 2008). Ignoring such socio-spatial interactivities could lead to an underestimation of the population exposure, especially for hub cities with high population mobility (Lall and Deichmann, 2012). In this regard, the mobility-based effect boosts seismic risk in suburbs and medium-sized hub cities. Sharp and Clark (2008) studied commuting behaviors among urban, suburban, fringe, and rural areas and found that the fringe area is often marked with a high proportion of new buildings and high mobility. Exposure and vulnerability are two mechanisms that occur simultaneously and intensify risk for the suburban and fringe commuting population. Thus, disaster risks are likely to be underestimated in such areas.
2.1 Taiwan's Chi-Chi earthquake study area
Taiwan is located on the western edge of the Pacific Ring of Fire, a seismically active zone at the convergence of the Philippine Sea plate and the Eurasian plate. Due to complex and active tectonic settings, Taiwan experiences frequent earthquakes. On average, 18 649 earthquakes have hit Taiwan annually from 1991 to 2004, among which 1047 earthquakes could be detected (CWA, 2019). The Chi-Chi earthquake was the most lethal earthquake since the 1935 Shinchiku-Taichū earthquake. It struck at 01:47 (GMT+8) on 21 September 1999, in the township of Chi-Chi, Nantou County (23.77° N, 120.98° E; Fig. 2), southeast of the Taichung metropolis, the second largest city of Taiwan. The sequence produced a 90 km surface rupture along the Chelungpu thrust fault, stretching between foothills of the Central Mountain Range in the east to the alluvial plains and basins in the west (Fig. 2). The Mw 7.6 main shock and aftershocks resulted in either the complete destruction or the serious damage of approximately 110 000 buildings and resulted in 2444 deaths, 94 % of which were due to building collapses (Kao and Chen, 2000; Tien et al., 2002). The top three damaged structural types were reinforced concrete structures (44 %), unreinforced brick structures (22 %), and unreinforced clay block buildings (12 %) (Tsai et al., 2000). Fatalities were concentrated in Taichung County (1138 deaths) and Nantou County (928 deaths). Most fatalities occurred in suburbs or urban fringes along the periphery of the Taichung metropolis and medium-sized cities outside of the metropolis (Fig. 1). Previous studies on the Chi-Chi earthquake found known risk factors from the hazard, exposure, and vulnerability components in risks (Lin et al., 2017, 2015). Yet, migration effects and factors of the disadvantaged population remain unknown.
In this study, we adopted the neighborhood geographic unit to estimate the socio-spatial effect of the seismic fatalities of the Chi-Chi earthquake. The smallest (fourth-level) administrative division in Taiwan is the village level in rural districts, which is equivalent to a neighborhood in urban districts. As village and neighborhood refer to the same administrative level, we hereafter use neighborhood to harmonize the terminologies and use rural or urban neighborhood for specifying the context as necessary. In total, Taiwan encompassed 7558 neighborhoods with an average population of 3000 in 1999. Although the earthquake was sensible island wide, it did not yield tangible damage across the entire island. Thus, we excluded neighborhoods under the threshold of ground motion intensity, Sa03 (spectral acceleration at 0.3 s) of 0.14 g (g: the acceleration due to gravity), which suggests that no damage occurred below this intensity (Lin et al., 2015). This resulted in 4502 neighborhoods as our study area. We used the urbanization levels of districts defined by Liu et al. (2006) to determine deaths located in suburbs and the urban–rural fringe.
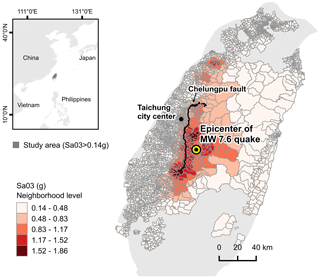
Figure 2Map of Taiwan's mainland and the study area (Sa03 ≥ 0.14 g) with gray lines showing neighborhood boundaries. The Chi-Chi earthquake resulted in a 90 km surface rupture along the Chelungpu thrust fault, stretching between the foothills of the Central Mountain Range to the plains on the western coast.
2.2 Data
We define risk according to the IPCC Fifth Assessment Report as “the potential for consequences where something of human value (i.e., fatality in our case) is at stake and where the outcome is uncertain” (IPCC, 2012; UNDRO, 1980). Thus, we collect data for our variables based on the formula of fatality risk as follows in Eq. (1; Chen et al., 2024):
2.2.1 Dependent variable: earthquake fatalities
The dependent variable in this study is the death toll in each neighborhood. We obtained the data from Tien et al. (2002), who investigated the fatalities caused by the Chi-Chi earthquake. Notably, the research team from the National Central University tracked the location of each death using the Global Positioning System (Kao and Chen, 2000; Tien et al., 2002). The death toll thus reflected the location of the death rather than their registered residence. The number of fatalities per neighborhood ranges from 0 to 87 (Table 1).
2.2.2 Independent variable: hazard
The seismic hazard dimension comprises two variables: seismic intensity and surface rupture. For seismic intensity, we measured Sa03, standing for spectral acceleration at 0.3 s. This measurement is found to be more representative than peak ground acceleration or peak ground velocity when considering damage to low- to mid-rise buildings (one to six stories), which comprised 99.8 % of the buildings damaged during the Chi-Chi earthquake (Lin et al., 2015; Wu et al., 2004, 2002). The Sa03 ground motion data were obtained from the Central Weather Administration, which had installed approximately 650 free-field strong motion stations around Taiwan before the Chi-Chi earthquake under the Taiwan Strong Motion Instrumentation Program (Shin et al., 2003). In terms of surface rupture, the Chelungpu fault surveyed by Chen et al. (2001) was applied to identify the distribution of the fault rupture. Chelungpu is a thrust fault; empirically major destruction occurred on the hanging-wall side of the depression. We thus identified a fault impact zone with a 5 km buffer (4 km on the hanging wall and 1 km on the footwall) along the fault. The ratio of the area in the fault impact zone (from 0 to 1) in each neighborhood was calculated based on the geographical information system to reflect its influence on fatalities.
2.2.3 Independent variable: exposure
The exposure variables included population size and the proportion of buildings with low seismic capacity (Lin et al., 2017, 2015). We obtained the population data from the Taiwan Population and Household Census of 2000 (Chang and Shyue, 2009). The average population per neighborhood was 2901, with a minimum of 16 people in a rural village and a maximum of 38 822 in the densest neighborhood of the urban areas. Additionally, building fragility is a crucial intermediate factor that can fundamentally influence earthquake fatalities (Bilham and Gaur, 2013; Birkmann et al., 2016; Lin et al., 2015; Yeh et al., 2006). People situated in fragile buildings with low seismic resistance would suffer from a higher degree of exposure from the doubling effect of the initial seismic hazard and the potential collapse of buildings. We obtained the building seismic-capacity data from the National Center for Research on Earthquake Engineering (Yeh et al., 2006). The building capacity classification was based on the history of seismic design codes for the buildings at four levels (high, moderate, and low code and pre-code) (Scawthorn et al., 2006). We considered pre-code and low-code buildings as low seismic capacity and calculated the percentage of the floor area with low seismic capacity per neighborhood in the year 2000. The average percentage of low-seismic-capacity buildings was 37 % among the studied neighborhoods (Table 1).
2.2.4 Independent variable: vulnerability
The vulnerability variables included the neighborhood-level sex ratio (male-to-female ratio), the percentage of population under the age of 15, the percentage of population over the age of 64, household income, income inequality, and the indigenous-population proportion. The sex ratio, the dependent population (age under 15 and over 64), median income, and income inequality are known risk factors for the Chi-Chi earthquake death toll (Li et al., 2017; Lin et al., 2015). The population dependency factor calculates the percentage of the population under the age of 15 and over the age of 64. A larger dependent population, either young or aged, indicates a higher degree of vulnerability. We obtained demographic data at the neighborhood level from the Taiwan Population and Household Census of 2000. The overall sex ratio was 1.08 on average, meaning that males outnumber females in the studied neighborhoods. There were no statistics available regarding the non-binary gender population.
Household income, measured by the median and standard deviation, reflects the average economic development and income inequities of each neighborhood, respectively, which are key factors of vulnerability (Cutter et al., 2006; Cutter and Finch, 2008; Kahn, 2005). We obtained the household income data before tax in 1999 from the Ministry of Finance (2023). Notably, the median annual household income of each neighborhood ranges from TWD 224 000 (∼ USD 7400) to TWD 1 425 000 (∼ USD 47 500), and the standard deviation of annual household income ranges from TWD 182 060 (∼ USD 6068) to TWD 23 734 840 (∼ USD 791 161); these values indicate considerable social differences between neighborhoods. We used the logarithmic transformation of the median and standard deviation of household income to deal with the skewed distributions of these variables.
We integrated the proportion of the indigenous population as a new variable to reflect the land dispossession and economic marginalization that occurred since the colonial era. The Ministry of the Interior in Taiwan defines indigenous populations as groups of people with their own distinct languages, cultures, and traditions and acknowledges the existence of 16 official indigenous groups. As of 2024, their population numbered 589 038, representing approximately 2.5 % of Taiwan's total population of 23 million (Ministry of Interior, 2024). In contrast to the majority Han population, who migrated to Taiwan in different stages in recent centuries, the indigenous peoples speak languages belonging to the Austronesian language family and have inhabited the island for millennia. The colonial history of Taiwan began in 1624 with the Dutch Republic (Dutch East India Company), followed by Spain; China (during the Ming and Qing dynasties); Japan (1895–1945); and most recently China through the Chinese Nationalist Party (also known as Kuomintang, KMT), with adherents settling in Taiwan after World War II and lifting martial law in 1987 (Nesterova and Jackson, 2018). Over the political transitions, the indigenous peoples have lost their lands, with most remaining indigenous territories being concentrating in mountainous regions. Hence, agriculture has been the primary employment sector for indigenous peoples, who comprise 20 % of the labor force, compared to total workforce participation in agriculture of 6.6 % in Taiwan in 2002 (Council of Indigenous Peoples, 2005). Manufacturing (15 %) and construction (13 %) are the next largest sectors for indigenous employment. Nevertheless, indigenous occupations often fall into the precarious category known as “3K jobs”. The term 3K jobs derives from Japanese words meaning “hard” (kitsui), “dirty” (kitanai), and “dangerous” (kiken), respectively. Typical 3K jobs are found in manufacturing and construction, usually offered on a contract basis with daily wages 2 to 3 times higher than the minimum wage, making them an attractive option for farmers during the off-season. It is worth noting that 64 % of indigenous farmers experience off-seasons ranging from 1 to 6 months, leading them to seek temporary work and housing in nearby towns (Council of Indigenous Peoples, 2005).
We obtained data regarding the proportion of the indigenous population from the Ministry of Interior (2023). The available neighborhood-level data closest to our study year was documented in 2008, and we considered the indigenous-population distribution between 1999 and 2008 to be similar.
2.2.5 Independent variable: migration pattern
In Taiwan, during the 1999 earthquake, no statistics were available on internal migration at the neighborhood level. Therefore, we utilized a radiation model to estimate population migration at this granularity (Simini et al., 2012). This model, which is widely used to determine migration flows between the origin and destination areas, utilizes population data and pairwise distances between neighborhoods to represent the push and pull factors in migration (Gibb et al., 2023; Li et al., 2017; Simini et al., 2012; Yang et al., 2014). The formula for the model uses the product of the populations of the origin and destination neighborhoods as the numerator. The denominator is an adjusted product of the populations within the vicinity of both the origin and destination areas, representing the effect of competing neighborhoods on the population flow. We applied the radiation model to quantify Tij, the proportion of the migrants from neighborhood i moving to neighborhood j, as follows in Eq. (2):
where Popi and Popj are the populations of neighborhoods i and j, respectively, and Sij is the total population within a buffer zone around the centroid of i, with the radius being the distance between the centroids of i and j, excluding the populations at both the source and destination points. Based on this origin–destination matrix, we produced three variables: estimated migration inflow, average income of the migrants' origin, and the proportion of the indigenous population of migrants.
Estimated migration inflow for neighborhood j can be represented as ∑iTiTij, where Ti is the total number of migrants originating from i. Although Ti is an unknown parameter, it is proportional to Popi based on Simini et al. (2012). Therefore, we calculated the estimated migration inflow for neighborhood j as ∑iPopiTij, which is a relative size proportional to the total number of migrants in neighborhoodj. Specifically, the estimated value represents the size of the migrant inflow assuming that every place has 100 % of its population migrating. In reality, however, the inflow is smaller and proportional to this estimate (Simini et al., 2012). Since this variable does not represent the absolute number of migrants but rather their relative size, we used the logarithm of the relative size of the migrant inflow to interpret the effect of a percent change in the number of migrants on the percent change in fatalities.
We calculated the average income of the migrants' origin by weighting the median income of the migrants' origin with the relative migrant inflow among all migrants in neighborhood j (Eq. 3). Similar to the neighborhood's income variable, we used the logarithm transformation of the variable to deal with its skewed distribution.
We calculated the proportion of the indigenous population of migrants based on the proportion of indigenous people and the relative migrant inflow. Assuming the proportion of migrants being indigenous from neighborhood i equals the proportion of indigenous population in neighborhood i, the proportion of the indigenous population of migrants in neighborhood j was calculated as in Eq. (4).
We used the library “spdep” in R to calculate distance between neighborhoods (see the “Code availability” section).
2.3 Models
We used Poisson regression and maximum likelihood estimation to predict the incidence rate ratio and significance levels for each covariate from the risk components of hazard, exposure, and vulnerability. The incidence rate ratio (IRR) of a given variable can be interpreted as the factor by which the fatality rate multiplies when that variable increases by one unit, assuming all other variables remain constant (Clayton and Hills, 2013). For example, if the IRR for the variable fault ratio is 2.65, it means that an increase in the fault ratio from 0 % to 100 % will lead to a 165 % increase in fatalities (calculated as (2.65 − 1) × 100 %). In other words, for each 10 % increase in the fault ratio, fatalities are expected to increase by 16.5 %. When a variable is in logarithmic form, the IRR can be interpreted as the factor by which the fatality rate multiplies for a 1 % increase in that variable. We modeled neighborhood-level fatalities against these variables, assuming a linear response association, across 4052 neighborhoods in Taiwan. We started with known risk factors in the basic model (Model 1; Eq. 4). Subsequently, we incorporated the proportion of the indigenous population in the model (Model 2; Eq. 5). Lastly, we included estimated characteristics of migration patterns (i.e., migrant inflow, average income of the migrants' origin, and indigenous-population proportion of migrants) in the final model (Model 3; Eq. 6). The three nested regression models can be described as follows:
The response FATi refers to the fatality count in neighborhood i. For each neighborhood i, hazard variables include Faulti (proportion of the area in the fault zone) and SA03i (the ground motion intensity measured as Sa03 (g)). Exposure variables include POPi (population) and LowCapacityBuildingi (proportion of buildings with low capacity). Vulnerability variables include SexRatioi (ratio of males to females), AgeUnder15i (percentage of the population under age 15), AgeOver64i (percentage of the population aged 65 or older), MedianIncomei (the logarithm of the median household income in neighborhood i), SDIncomei (the logarithm of the standard deviation of household income in neighborhood i), and Indigenousi (proportion of indigenous people in the population of neighborhood i). Migration pattern variables, estimated by the radiation model, included MigrationInflowi (the logarithm of the relative size of the migrant inflow to neighborhood i), MigrantIncomei (the logarithm of the average income of the migrants' origin in neighborhood i), and MigrantIndigenousi (percentage of indigenous people among migrants in neighborhood i). While we hypothesized that most variables would have a positive effect on fatality risk, some may have negative effects. For instance, median household income is associated with greater resources to cope with earthquakes. Similarly, although older groups may be more vulnerable due to physical limitations, they may also possess more experience in dealing with earthquake situations. The parameter εi represents the error term. We assessed model performance using R2 and log-likelihood values. We performed all statistical computations in R (version 4.3.1); statistical significance was evaluated at p<0.05.
3.1 Estimated migration patterns
Using the radiation model, we characterize the relative size of the migrant inflow, average income of the migrants' origin, and percentage of indigenous people among migrants (Fig. 3). Two example neighborhoods show they attracted migrants from different areas and distances based on their geography. A neighborhood in Wufeng (Fig. 1), a regional hub on the outskirts of the Taichung metropolitan area, was estimated to have migrants from other neighborhoods around 10 km away, as well as some migrants from Nantou, a mountain city about 40 km away. In contrast, the neighborhood in Puli (Fig. 1), a mountainous town, was estimated to have migrants from other mountainous villages that are up to 80 km away. This shows that the radiation model captures long-distance migration patterns between remote villages and their closest large town.
Comparing the patterns of migrants and residents, higher migration inflow, the income of the origin, and the percentage of the indigenous population among migrants were aligned with the spatial pattern of the population, population size, and percentage of the indigenous population among residents. Yet, there are some differences between the patterns of migrants and residents. Higher migration inflow was estimated in the suburbs and lower migration inflow was estimated in rural areas and city centers, as city centers have more competing destinations along the way from the rural area to the city. The radiation model also highlights a distinct zone of indigenous migrants at the foothills along the indigenous neighborhoods in mountainous areas (Fig. 3). This observation supports various case studies that highlight indigenous migrants' preference for blue-collar jobs in towns and cities while still staying close to their homeland.
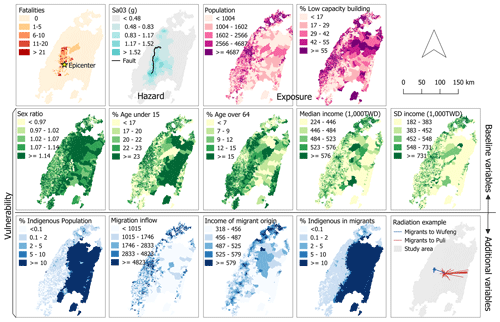
Figure 3Maps of seismic-fatality risk factors, including hazard, exposure, vulnerability, and migration pattern variables. Migrant inflow, as well as income and the proportion of the indigenous population within the migrant inflow, is estimated using the radiation model. Examples from migrant origins in Wufeng and Puli demonstrate that towns in remote areas (e.g., Puli) act as job opportunity hubs for villages far away.
3.2 Risk factors of seismic fatalities
The 1999 Chi-Chi earthquake resulted in 2444 deaths, including 1049 in suburbs and the urban–rural fringe. Model 1 confirms the findings of Lin et al. (2015), indicating that seismic intensity, the fault impact zone, population, buildings with low seismic capacity, the male-to-female ratio, lower household income, and higher income inequality are associated with an increased number of fatalities (Table 2). Seismic hazard remains for determining factors influencing the distribution of fatalities in the Chi-Chi earthquake. A 0.1 g increase in Sa03 could lead to a 173 % rise in neighborhood fatalities (mean Sa03 of 0.36), assuming other variables remain constant. The presence of a fault zone covering 10 % of a neighborhood's area resulted in a 90 % increase in fatalities. Due to the correlation between seismic intensity and the proximity to the fault, the interplay between these two variables is a primary determinant of fatalities. Exposure variables also show significant associations with fatalities. We find that an increase of 1000 residents in a neighborhood could result in a 38 % rise in fatalities, while a 10 % increase in the percentage of buildings with low seismic capacity could result in a 10 % rise in fatalities. We also find that several vulnerability variables are significant. Each 10 % increase in a neighborhood's male-to-female ratio is associated with a 3.5 % decrease in fatalities. Each 10 % increase in median household income, for example from TWD 403 000 (∼ USD 12 587) to TWD 443 300 (∼ USD 13 846), could reduce fatalities by 6 %. Each 10 % increase in the standard deviation of household income could lead to an 8 % increase in fatalities. The only variable presenting a different direction of association compared to Lin et al. (2015) is the percentage of the population aged over 64. Our findings suggest that a 10 % increase in a neighborhood's population over age 64 is associated with 10 % fewer fatalities. This discrepancy is likely due to our use of higher-resolution data at the neighborhood level, as opposed to the previous study's district-level analysis, and the observation that higher death tolls occurred in neighborhoods predominantly aged 15–64.
Model 2, which includes the proportion of the indigenous population in addition to Model 1's variables, indicates a negative association of the indigenous population with fatalities (Table 2). It suggests that every 10 % increase in a neighborhood's indigenous population is associated with a 7.5 % reduction in fatalities, demonstrating that the impact of the Chi-Chi earthquake is not primarily concentrated in indigenous jurisdictions. However, Model 3, which incorporates migration variables, reveals that a higher proportion of indigenous people among the migrants is linked to an increased number of fatalities. Specifically, a 10 % increase in the indigenous proportion among migrants correlates with a 19 % increase in fatalities. Model 3 also shows that higher migration inflow and a lower average income in migrants' places of origin are both associated with an increased number of fatalities (p<0.05).
Table 2Incidence rate ratios and standard errors estimated by Poisson models for neighborhood-level risk factors, estimated by three nested models. Model 1 includes hazard, exposure, and vulnerability variables previously found in Lin et al. (2015); Model 2 adds the indigenous-population proportion; and Model 3 includes migration pattern variables.
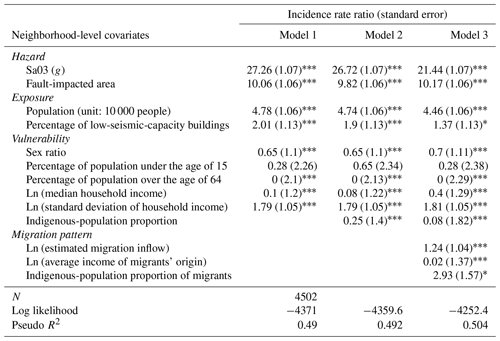
Note: p<0.001, p<0.01, and * p<0.05.
This study presents novel models to understand migration as a risk factor of seismic fatalities which has been long underestimated due to the lack of mobility data. We find that not only place-based risk factors contribute to fatality risk in the Chi-Chi earthquake. Rather, after adjusting for seismic intensity, population exposure, and residence-based socioeconomic factors, higher fatality risk was found among neighborhoods attracting migrants from an origin with a lower income or/and indigenous migrants. This finding, along with the migration patterns concentrating in the suburban areas outside large cities as well as medium-sized cities serving as regional hubs, helps explain the suburban syndrome of seismic fatalities.
Our basic model aligns with previous studies, confirming that hazard variables (fault impact and ground motion intensity), exposure factors (population and percentage of buildings with low seismic capacity), and socioeconomic-vulnerability indicators (median and standard deviation of household income) are all determinants of seismic fatality at the neighborhood level. Consistent with previous studies (Lin et al., 2015), hazard and exposure factors together constitute the most important part (R2 = 0.4) contributing to seismic fatality. Social-vulnerability variables, although playing a marginal effect (an increase of R2 < 0.1), are significantly associated with fatality disparities. That is to say, when seismic intensity and exposure are at similar levels, it is the composition of social vulnerability that could either exacerbate or mitigate the scale of seismic fatality. The basic model results explain the fatality distribution shown in Fig. 1. Most fatalities appeared in medium-sized cities in suburbs (e.g., Dali, Taiping, and Fengyuan) and urban fringes (e.g., Wufeng, Dongshi, and Daliao) of the Taichung metropolis. Those are considered satellite cities and regional hubs in the urban–rural corridor. Remarkably, the percentage of buildings with low seismic capacity is significantly correlated with fatalities and presented a predominant effect, explaining more fatalities in suburbs with lower costs of living. The analysis reflects the view of buildings becoming weapons of mass destruction. Additionally, small and medium-sized cities often suffer from the socio-political difficulties of poor governance and an insufficient capacity to cope with hazards when experiencing rapid sprawl (Bilham, 2013; Bilham and Gaur, 2013). This calls for urgent seismic-risk reduction efforts in suburban neighborhoods and hub cities or towns that could undergo intensified hazard exposure and vulnerability during an earthquake.
Our final model demonstrates that the correlation between migration patterns and fatalities is significant. Although R2 only increases 0.014 compared to the base model, the effect is statistically significant. And, most importantly, by adding the various migration variables, the role of migration patterns becomes even clearer. Not only is the estimated migrant inflow associated with more fatalities, but also the model shows that lower income at the origin and a higher proportion of indigenous people among migrants correlate with an increased number of fatalities. Thus, social vulnerability within migrant groups significantly contributes to heightened risks, beyond mere underestimated exposure. Migrant workers often face a myriad of social vulnerabilities (Ahonen et al., 2007; Flynn, 2018) which can exacerbate their risks of death during an earthquake. The vulnerability of migrant workers often leads to a high likelihood of taking risky, non-contractual, temporary employment such as that in construction or agriculture, often without sufficient benefits or safeguards (Ahonen et al., 2018; Al-Tarawneh et al., 2020; Foley, 2017; Foley et al., 2014; Howard, 2017). Precariousness in their jobs often provides economic precarity and results in many immigrant/migrant workers living in overcrowded, substandard housing arrangements, including makeshift dwellings, trailers, or employer-provided accommodations (Caxaj et al., 2024). These forms of housing often lack basic safety features, such as fire exits or structural stability, increasing their vulnerability to injury and death during an earthquake.
Migrant workers often experience what has been termed “overlapping vulnerabilities” (Ceballos et al., 2020; Flynn et al., 2015) or “cumulative precarity” (Gravel and Dubé, 2016), which creates disparities in health beyond seismic risks (Benach et al., 2011; Facey and Eakin, 2010; Krieger, 2010). For example, migrant workers experienced higher rates of illness and death during the COVID-19 pandemic, which is also associated with their commuting behavior and housing conditions (Fielding-Miller et al., 2020; Istiko et al., 2022; Reid et al., 2021). In various cultures, migratory populations are sometimes described as “wanderers”, as seen in the Taiwanese indigenous pop song Wandering and the classical German piece Zigeunerweisen, which highlight the instability experienced by migrants. In the context of the Chi-Chi earthquake, migration variables further amplify seismic risk. Indigenous hometowns in Taiwan's mountainous regions were less affected by the earthquake, while migrants who moved to small and medium-sized cities for precarious jobs faced significantly higher exposure to earthquake disasters and social vulnerability. Indigenous populations in Taiwan face an elevated risk of fatality compared to the general population (Juan et al., 2016) due to various social vulnerabilities, including low income, limited education, and inadequate access to healthcare (Liao et al., 2024). They are found to be especially vulnerable when migrating into cities for job opportunities if they experience residential segregation because only inferior rental housing is affordable (Hu and Chen, 2011; Wu et al., 2018). Therefore, our findings suggest that safety regulations for rental housing, particularly for affordable options, as well as rent subsidies for low-income, migrant, and historically marginalized groups, are imperative strategies for reducing risk.
As far as we are aware, this is the first study that incorporates migration dimensions into the hazard–exposure–vulnerability model of seismic-fatality risk. Nevertheless, this study is not without its limitations. First, given the early timeline of the case study (earthquake in 1999), we were unable to validate the migration patterns using newer technology. Previous studies that validated the model with empirical data have shown that radiation models predict commuting patterns well at the national level (Masucci et al., 2013; Simini et al., 2012), though they may underestimate long-distance and international migration (Kluge and Schewe, 2021). Future studies on recent earthquakes could employ mobile data to further distinguish between different migration behaviors (e.g., daily commuting versus seasonal migration), which would allow for disaggregating the sources of seismic risks associated with mobility. Second, we did not account for the saturation effect of Sa03 – that is, the possibility that higher Sa03 values might not cause additional damage beyond a certain threshold. Third, incorporating additional sociodemographic variables of migrant workers, such as languages spoken, occupation at the time of the earthquake, and social networks including tribal affiliations, can further elucidate the sources of vulnerability and resilience to disasters. This study reveals general relevance and opens scientific questions for future research on migrants' exposure to environmental hazards.
In this study, we integrate migration pattern variables into the traditional seismic-fatality risk model to explain the concentration of earthquake fatalities in suburban areas. While ground motion intensity and population exposure account for more seismic fatalities in suburban neighborhoods and urban–rural corridors during the Chi-Chi earthquake, the presence of migrants from low-income origins and a higher proportion of the indigenous population further amplified the fatalities in these areas. It is important to note that our findings may not extend universally to all earthquakes, given the diverse geological and tectonic structures, social contexts, institutions, and other uncertainties they involve. Nonetheless, low-cost housing options with a lack of safe infrastructure will disproportionally affect fatality during earthquakes. This illustrates a pressing necessity to enforce building code regulations, especially providing a safe housing and living standard for migrant workers without compromising affordability.
While the investigation of a single earthquake cannot be generally applied, it sheds light on pathways for improving methods, assumptions, and implications critical for advancing seismic-risk assessment studies and potentially impacting how fatalities from extreme weather events and other catastrophes are studied. Our empirical findings underscore the need to reexamine city-, region-, and nation-wide earthquake preparedness and response policies to better allocate resources to areas at greatest risk. These findings suggest that focusing seismic-risk mitigation efforts on newly developed, economically disadvantaged suburban neighborhoods and hub cities that cater to rural–urban migrations, characterized by high concentrations of migrants living in informal housing or low-cost condominiums, is important. Recognizing the suburban syndrome and implementing safety and affordability regulations in suburban housing could play a pivotal role in safeguarding human lives against future catastrophic earthquakes.
The code and data for all statistical analyses for the paper are available at https://doi.org/10.5281/zenodo.14278579 (Chen et al., 2024). All variables are aggregated to the neighborhood level. Maps presented in this paper are generated using QGIS, a freely available piece of software.
All authors designed the research. THKC performed the research. THKC, GYL, and CHY curated the data. THKC, KHEL, THL, and DMC wrote the paper.
The contact author has declared that none of the authors has any competing interests.
Publisher's note: Copernicus Publications remains neutral with regard to jurisdictional claims made in the text, published maps, institutional affiliations, or any other geographical representation in this paper. While Copernicus Publications makes every effort to include appropriate place names, the final responsibility lies with the authors.
We would like to thank the anonymous reviewers for their constructive feedback, which has helped us improve the paper.
This paper was edited by Paola Reichenbach and reviewed by two anonymous referees.
Adhikari, B., Bhandari, P. M., Neupane, D., and Mishra, S. R.: A Retrospective Analysis of Mortality From 2015 Gorkha earthquakes of Nepal: evidence and future recommendations, Disaster Med. Public, 15, 127–133, 2021.
Ahonen, E. Q., Benavides, F. G., and Benach, J.: Immigrant populations, work and health – a systematic literature review, Scandinavian journal of work, Environ. Health, 2007, 96–104, 2007.
Ahonen, E. Q., Fujishiro, K., Cunningham, T., and Flynn, M.: Work as an inclusive part of population health inequities research and prevention, Am. J. Public Health, 108, 306–311, 2018.
Aldrich, D. P. and Sawada, Y.: The physical and social determinants of mortality in the 3.11 tsunami, Soc. Sci. Med., 124, 66–75, 2015.
Allan, P., Bryant, M., Wirsching, C., Garcia, D., and Teresa Rodriguez, M.: The influence of urban morphology on the resilience of cities following an earthquake, Journal of Urban Design, 18, 242–262, 2013.
Al-Tarawneh, I. S., Wurzelbacher, S. J., and Bertke, S. J.: Comparative analyses of workers' compensation claims of injury among temporary and permanent employed workers in Ohio, Am. J. Ind. Med., 63, 3–22, 2020.
Anbarci, N., Escaleras, M., and Register, C. A.: Earthquake fatalities: the interaction of nature and political economy, J. Public Econ., 89, 1907–1933, 2005.
Andersen, M. J., Williamson, A. B., Fernando, P., Eades, S., and Redman, S.: “They took the land, now we're fighting for a house”: Aboriginal perspectives about urban housing disadvantage, Housing Stud., 33, 635–660, 2018.
Belanger, Y. D., Awosoga, O. A., and Weasel Head, G.: Homelessness, urban Aboriginal people, and the need for a national enumeration, Aboriginal Policy Studies, 2, 4–33, https://doi.org/10.5663/aps.v2i2.19006, 2013.
Benach, J., Muntaner, C., and Santana, V.: Employment conditions and health inequalities, Final report to the WHO Commission on Social Determinants of Health, Employment Conditions Knowledge Network (EMCONET), https://repository.mdx.ac.uk/download/0a79378bfa4231ed255694c87024a95e9e5021625bc62d4a26df4efcc117af13/1299821/emconet_who_report.pdf (last access: 29 November 2024), 2011.
Bilham, R.: Societal and observational problems in earthquake risk assessments and their delivery to those most at risk, Tectonophysics, 584, 166–173, 2013.
Bilham, R. and Gaur, V.: Buildings as weapons of mass destruction, Science, 341, 618–619, 2013.
Birkmann, J., Welle, T., Solecki, W., Lwasa, S., and Garschagen, M.: Boost resilience of small and mid-sized cities, Nature, 537, 605–608, 2016.
Böheim, R. and Taylor, M. P.: Tied down or room to move? Investigating the relationships between housing tenure, employment status and residential mobility in Britain, Scot. J. Polit. Econ., 49, 369–392, 2002.
Brouwer, R., Akter, S., Brander, L., and Haque, E.: Socioeconomic vulnerability and adaptation to environmental risk: a case study of climate change and flooding in Bangladesh, Risk Anal., 27, 313–326, 2007.
Caxaj, C. S., Weiler, A. M., and Martyniuk, J.: Housing conditions and health implications for migrant agricultural workers in Canada: A scoping review, Canadian Journal of Nursing Research, 56, 16–28, 2024.
Ceballos, D. M., Côté, D., Bakhiyi, B., Flynn, M. A., Zayed, J., Gravel, S., Herrick, R. F., and Labrèche, F.: Overlapping vulnerabilities in workers of the electronics recycling industry formal sector: A commentary, Am. J. Ind. Med., 63, 955–962, 2020.
Chang, C.-J. and Shyue, S.-W.: A study on the application of data mining to disadvantaged social classes in Taiwan's population census, Expert Syst. Appl., 36, 510–518, 2009.
Chen, W.-S., Chen, Y.-G., Chang, H.-C., Lee, Y.-H., and Lee, J.-C.: Paleoseismic study of the Chelungpu fault in the Wanfung area, Western Pacific Earth Sciences, 1, 499–506, 2001.
Chen, T.-H. K., Lin, K.-H. E., Lin, T.-H., Liu, G.-Y., Yeh, C.-H., and Ceballos, D. M.: Migration as a Hidden Risk Factor in Seismic Fatality: Spatial Modeling of the Chi-Chi Earthquake and Suburban Syndrome (Version 1), Zenodo [data set, code], https://doi.org/10.5281/zenodo.14278579, 2024.
Clayton, D. and Hills, M.: Statistical models in epidemiology, OUP Oxford, ISBN 9780198522218, 2013.
Council of Indigenous Peoples, Taiwan: Employment Status Survey of Taiwan Indigenous Peoples, Council of Indigenous Peoples, 2005.
Cutter, S. L. and Finch, C.: Temporal and spatial changes in social vulnerability to natural hazards, P. Natl. Acad. Sci. USA, 105, 2301–2306, 2008.
Cutter, S. L., Emrich, C. T., Mitchell, J. T., Boruff, B. J., Gall, M., Schmidtlein, M. C., Burton, C. G., and Melton, G.: The long road home: Race, class, and recovery from Hurricane Katrina, Environment, 48, 8–20, 2006.
CWA: Special issue on earthquake observations: 20 years after the Chi-Chi earthquake, Central Weather Bureau, https://scweb.cwa.gov.tw/zh-tw/page/twenty (last access: 29 November 2024), 2019.
Derakhshan, S., Hodgson, M. E., and Cutter, S. L.: Vulnerability of populations exposed to seismic risk in the state of Oklahoma, Appl. Geogr., 124, 102295, https://doi.org/10.1016/j.apgeog.2020.102295, 2020.
Dicken, P., Kelly, P. F., Olds, K., and Wai-Chung Yeung, H.: Chains and networks, territories and scales: towards a relational framework for analysing the global economy, Global Netw., 1, 89–112, 2001.
Escaleras, M., Anbarci, N., and Register, C. A.: Public sector corruption and major earthquakes: A potentially deadly interaction, Public Choice, 132, 209–230, 2007.
Facey, M. E. and Eakin, J. M.: Contingent work and ill-health: conceptualizing the links, Soc. Theor. Health, 8, 326–349, 2010.
Fan, C. C. and Li, T.: Split households, family migration and urban settlement: Findings from China's 2015 national floating population survey, Social Inclusion, 8, 252–263, 2020.
Fell, D. B., Dodds, L., and King, W. D.: Residential mobility during pregnancy, Paediatr. Perinat. Ep., 18, 408–414, 2004.
Fielding-Miller, R. K., Sundaram, M. E., and Brouwer, K.: Social determinants of COVID-19 mortality at the county level, PloS one, 15, e0240151, https://doi.org/10.1371/journal.pone.0240151, 2020.
Flynn, M., Cunningham, T., Guerin, R., Keller, B., Chapman, L., and Hudson, D.: Overlapping vulnerabilities: the occupational health and safety of young immigrant workers in small construction firms, NIOSH and ASSE Report, Centers for Disease Control and Prevention, National Institute for Occupational Safety and Health, DHHS (NIOSH), 2015.
Flynn, M. A.: Im/migration, work, and health: anthropology and the occupational health of labor im/migrants, Anthropology of work review, 39, 116, https://doi.org/10.1111/awr.12151, 2018.
Foley, M.: Factors underlying observed injury rate differences between temporary workers and permanent peers, Am. J. Ind. Med., 60, 841–851, 2017.
Foley, M., Ruser, J., Shor, G., Shuford, H., and Sygnatur, E.: Contingent workers: Workers' compensation data analysis strategies and limitations, Am. J. Ind. Med., 57, 764–775, 2014.
Freire, S. and Aubrecht, C.: Integrating population dynamics into mapping human exposure to seismic hazard, Nat. Hazards Earth Syst. Sci., 12, 3533–3543, https://doi.org/10.5194/nhess-12-3533-2012, 2012.
Gibb, R., Colón-González, F. J., Lan, P. T., Huong, P. T., Nam, V. S., Duoc, V. T., Hung, D. T., Dong, N. T., Chien, V. C., and Trang, L. T. T.: Interactions between climate change, urban infrastructure and mobility are driving dengue emergence in Vietnam, Nat. Commun., 14, 8179, https://doi.org/10.1038/s41467-023-43954-0, 2023.
Gravel, S. and Dubé, J.: Occupational health and safety for workers in precarious job situations: combating inequalities in the workplace, E-Journal of international and comparative labour studies, 5, 1–30, 2016.
He, C., Huang, Q., Dou, Y., Tu, W., and Liu, J.: The population in China's earthquake-prone areas has increased by over 32 million along with rapid urbanization, Environ. Res. Lett., 11, 074028, https://doi.org/10.1088/1748-9326/11/7/074028, 2016.
Howard, J.: Nonstandard work arrangements and worker health and safety, Am. J. Ind. Med., 60, 1–10, 2017.
Hu, C.-P. and Chen, H.-R.: A Study on Urbanization and Residential Segregation-an Example of Aborigines in Taipei County, Journal of Architecture and Planning, 12, 75–91, 2011.
IPCC: Managing the risks of extreme events and disasters to advance climate change adaptation: A special report of working groups I and II of the intergovernmental panel on climate change, edited by: Field, C. B., Barros, V., Stocker, T. F., Qin, D., Dokken, D. J., Ebi, K. L., Mastrandrea, M. D., Mach, K. J., Plattner, G.-K., Allen, S. K., Tignor, M., and Midgley, P. M., Cambridge University Press, Cambridge, UK, New York, USA, ISBN 978-1-107-60780-4, 2012.
Istiko, S. N., Durham, J., and Elliott, L.: (Not that) essential: A scoping review of migrant workers' access to health services and social protection during the COVID-19 pandemic in Australia, Canada, and New Zealand, Int. J. Env. Res. Pub. He., 19, 2981, https://doi.org/10.3390/ijerph19052981, 2022.
Jamshed, A., Birkmann, J., Rana, I. A., and McMillan, J. M.: The relevance of city size to the vulnerability of surrounding rural areas: An empirical study of flooding in Pakistan, Int. J. Disast. Risk Re., 48, 101601, https://doi.org/10.1016/j.ijdrr.2020.101601, 2020.
Jessop, B., Brenner, N., and Jones, M.: Theorizing sociospatial relations, Environ. Plann. D, 26, 389–401, 2008.
Juan, S.-C., Awerbuch-Friedlander, T., and Levins, R.: Ethnic density and mortality: aboriginal population health in Taiwan, Public Health Rev., 37, 1–8, 2016.
Kahn, M. E.: The death toll from natural disasters: the role of income, geography, and institutions, Rev. Econ. Stat., 87, 271–284, 2005.
Kao, H. and Chen, W.-P.: The Chi-Chi earthquake sequence: Active, out-of-sequence thrust faulting in Taiwan, Science, 288, 2346–2349, 2000.
Kluge, L. and Schewe, J.: Evaluation and extension of the radiation model for internal migration, Phys. Rev. E, 104, 054311, https://doi.org/10.1103/PhysRevE.104.054311, 2021.
Krieger, N.: Workers are people too: societal aspects of occupational health disparities—an ecosocial perspective, Am. J. Ind. Med., 53, 104–115, 2010.
Kumar, S. and Sati, V. P.: Patterns and determinants of rural–urban migration in the Garhwal Himalaya, GeoJournal, 88, 3679–3698, 2023.
Lall, S. V. and Deichmann, U.: Density and disasters: economics of urban hazard risk, World Bank Res. Observer., 27, 74–105, 2012.
Lavell, A.: Local level risk management-Concepts and experience in Central America, The Disaster Preparedness and Mitigation Summit, 15, 2003.
Li, F., Feng, Z., Li, P., and You, Z.: Measuring directional urban spatial interaction in China: A migration perspective, PloS one, 12, e0171107, https://doi.org/10.1371/journal.pone.0171107, 2017.
Liao, Z.-Y., Kean, S., and Haycock-Stuart, E.: Indigenous lands and health access: The influence of a sense of place on disparities in post-stroke recovery in Taiwan, Health Place, 86, 103210, https://doi.org/10.1016/j.healthplace.2024.103210, 2024.
Lin, K.-H. E., Lin, T.-H., Hu, B. W., Chang, Y.-C., Yeh, C. H., Liu, G. Y., and Chan, C. H.: Earthquake, housing damage, and fatality: A causal-effect analysis of seismic risk in Chi-Chi Earthquake, City and Planning, 44, 83–112, 2017.
Lin, K. E., Chang, Y., Liu, G., Chan, C., Lin, T., and Yeh, C.: An interdisciplinary perspective on social and physical determinants of seismic risk, Natural Hazards and Earth System Sciences, 15, 2173, 2015.
Liu, C.-Y., Hung, Y.-T., Chuang, Y.-L., Chen, Y.-J., Weng, W.-S., Liu, J.-S., and Liang, K.-Y.: Incorporating development stratification of Taiwan townships into sampling design of large scale health interview survey, J. Health Manag., 4, 1–22, 2006.
Liu, Z., Liu, C., and Mostafavi, A.: Beyond residence: A mobility-based approach for improved evaluation of human exposure to environmental hazards, Environ. Sci. Technol., 57, 15511–15522, 2023.
Masi, A., Lagomarsino, S., Dolce, M., Manfredi, V., and Ottonelli, D.: Towards the updated Italian seismic risk assessment: exposure and vulnerability modelling, B. Earthq. Eng., 19, 3253–3286, 2021.
Masucci, A. P., Serras, J., Johansson, A., and Batty, M.: Gravity versus radiation models: On the importance of scale and heterogeneity in commuting flows, Phys. Rev. E, 88, 022812, https://doi.org/10.1103/PhysRevE.88.022812, 2013.
McGranahan, G., Balk, D., and Anderson, B.: The rising tide: assessing the risks of climate change and human settlements in low elevation coastal zones, Environ. Urban., 19, 17–37, 2007.
Ministry of Finance, Taiwan: Taxable income in counties, cities, towns and villages, Ministry of Finance, 2023.
Ministry of Interior, Taiwan: Neighborhood-level statistics of indigenous population in Taiwan 2008, Ministry of Interior, 2023.
Ministry of Interior, Taiwan: Weekly Interior Statistics Bulletin, Week 6 of the Year 113, Ministry of Interior, 2024 (in Chinese).
Moghaddam, P. S., Jahangiri, K., Sohrabizadeh, S., Hassani, N., Moghaddam, M. H., and Tehrani, G. M.: Foresight of the consequences of the hazmat release from an oil refinery on the surrounding urban community following an earthquake: A Natech scenario analysis, Disaster Med. Public, 17, e79, https://doi.org/10.1017/dmp.2021.349, 2023.
Montz, B. E., Allen, T. R., and Monitz, G. I.: Systemic trends in disaster vulnerability: Migrant and seasonal farm workers in North Carolina, Risk, Hazards & Crisis in Public Policy, 2, 1–17, 2011.
Nesterova, Y. and Jackson, L.: Understanding the `local' in indigenous Taiwan, International Education Journal: Comparative Perspectives, 17, 55–66, 2018.
Önder, Z., Dökmeci, V., and Keskin, B.: The impact of public perception of earthquake risk on Istanbul's housing market, Journal of Real Estate Literature, 12, 181–194, 2004.
Panday, S., Rushton, S., Karki, J., Balen, J., and Barnes, A.: The role of social capital in disaster resilience in remote communities after the 2015 Nepal earthquake, Int. J. Disast. Risk Re., 55, 102112, https://doi.org/10.1016/j.ijdrr.2021.102112, 2021.
Pavić, G., Hadzima-Nyarko, M., Bulajić, B., and Jurković, Ž.: Development of seismic vulnerability and exposure models – A case study of Croatia, Sustainability, 12, 973, https://doi.org/10.3390/su12030973, 2020.
Rashed, T. and Weeks, J.: Assessing vulnerability to earthquake hazards through spatial multicriteria analysis of urban areas, Int. J. Geogr. Inf. Sci., 17, 547–576, 2003.
Ravenstein, E. G.: The laws of migration, J. R. Stat. Soc., 52, 241–305, 1889.
Reid, A., Ronda-Perez, E., and Schenker, M. B.: Migrant workers, essential work, and COVID-19, Am. J. Ind. Med., 64, 73–77, 2021.
Scawthorn, C., Flores, P., Blais, N., Seligson, H., Tate, E., Chang, S., Mifflin, E., Thomas, W., Murphy, J., and Jones, C.: HAZUS-MH flood loss estimation methodology. II. Damage and loss assessment, Nat. Hazards Rev., 7, 72–81, 2006.
Sharp, J. S. and Clark, J. K.: Between the country and the concrete: Rediscovering the Rural-Urban fringe, City & Community, 7, 61–79, 2008.
Shier, M. L., Graham, J. R., Fukuda, E., and Turner, A.: Risk and protective factors of precarious housing among Indigenous people living in urban centres in Alberta, Canada, Canadian Review of Social Policy, 2015, 65–94, 2015.
Shin, T., Tsai, Y., Yeh, Y., Liu, C., and Wu, Y.: Strong-motion instrumentation programs in Taiwan, International Geophysics Series, 81, 1057–1064, 2003.
Simini, F., González, M. C., Maritan, A., and Barabási, A.-L.: A universal model for mobility and migration patterns, Nature, 484, 96–100, 2012.
Taubenböck, H., Kraff, N. J., and Wurm, M.: The morphology of the Arrival City-A global categorization based on literature surveys and remotely sensed data, Appl. Geogr., 92, 150–167, 2018.
Tien, Y. M., Juang, D. S., Pai, C. H., Hisao, C. P., and Chen, C. J.: Statistical analyses of relation between mortality and building type in the 1999 chi-chi earthquake, J. Chin. Inst. Eng., 25, 577–590, 2002.
Tsai, K., Hsiao, C. P., and Bruneau, M.: Overview of building damages in 921 Chi-Chi earthquake, Earthquake Engineering and Engineering Seismology, 2, 93–108, 2000.
Turner, B. L., Kasperson, R. E., Matson, P. A., McCarthy, J. J., Corell, R. W., Christensen, L., Eckley, N., Kasperson, J. X., Luers, A., and Martello, M. L.: A framework for vulnerability analysis in sustainability science, P. Natl. Acad. Sci., 100, 8074–8079, 2003.
UN: World Urbanization Prospects 2018, Department of Economic and Social Affairs of the United Nation, ISBN 978-92-1-148319-2, 2018.
UNDRO: Natural disasters and vulnerability analysis, in: Report of Experts Group Meeting of 9–12 July 1979, UNDRO, Geneva, Switzerland, 1980.
UNISDR: Poverty & death: disaster mortality 1996–2015, United Nations Office for Disaster Risk Reduction, Geneva, Switzerland, https://www.preventionweb.net/files/50589_creddisastermortalityallfinalpdf.pdf (last access: 29 November 2024), 2016.
Viboud, C., Bjørnstad, O. N., Smith, D. L., Simonsen, L., Miller, M. A., and Grenfell, B. T.: Synchrony, waves, and spatial hierarchies in the spread of influenza, Science, 312, 447–451, 2006.
Wang, S., Zhang, M., Huang, X., Hu, T., Sun, Q. C., Corcoran, J., and Liu, Y.: Urban–rural disparity of social vulnerability to natural hazards in Australia, Sci. Rep., 12, 13665, https://doi.org/10.1038/s41598-022-17878-6, 2022.
Wilson, W. J.: The truly disadvantaged: The inner city, the underclass, and public policy, University of Chicago Press, ISBN 9780226901268, 2012.
Wu, Y.-M., Chen, Y.-C., and Chen, H.-M.: The Spatial Distribution and Center of Indigenous Population in Taiwan, Taiwan Journal of Indigenous Studies, 11, 149–189, 2018.
Wu, Y.-M., Hsiao, N.-C., and Teng, T.-L.: Relationships between strong ground motion peak values and seismic loss during the 1999 Chi-Chi, Taiwan earthquake, Nat. Hazards, 32, 357–373, 2004.
Wu, Y.-M., Hsiao, N.-C., Teng, T.-L., and Shin, T.-C.: Near real-time seismic damage assessment of the rapid reporting system, Terr. Atmos. Ocean. Sci., 13, 313–324, 2002.
Xu, X., Wen, X., Yu, G., Chen, G., Klinger, Y., Hubbard, J., and Shaw, J.: Coseismic reverse-and oblique-slip surface faulting generated by the 2008 Mw 7.9 Wenchuan earthquake, China, Geology, 37, 515–518, 2009.
Yang, Y., Herrera, C., Eagle, N., and González, M. C.: Limits of predictability in commuting flows in the absence of data for calibration, Sci. Rep., 4, 5662, https://doi.org/10.1038/srep05662, 2014.
Yeh, C.-H., Loh, C.-H., and Tsai, K.-C.: Overview of Taiwan earthquake loss estimation system, Nat. Hazards, 37, 23–37, 2006.