the Creative Commons Attribution 4.0 License.
the Creative Commons Attribution 4.0 License.
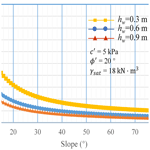
Shallow-landslide stability evaluation in loess areas according to the Revised Infinite Slope Model: a case study of the 7.25 Tianshui sliding-flow landslide events of 2013 in the southwest of the Loess Plateau, China
Jianqi Zhuang
Jianbing Peng
Chenhui Du
Yi Zhu
Jiaxu Kong
The occurrence of shallow loess landslides induced by prolonged heavy rainfall is prevalent in loess-dominated regions, often leading to property damage, human casualties, and sediment pollution. Developing an accurate prediction model for shallow landslides in loess areas is crucial for effective landslide mitigation. In 2013, prolonged heavy rains from 19–25 July triggered mass sliding-flow loess landslides in Tianshui, China. Landslide data, along with the characteristics of the sliding-flow loess landslides, were obtained through extensive field investigations and remote sensing interpretations. The sliding-flow loess landslide event demonstrated clustering, high density, small areas, and long travel distance. The depth of the sliding surface is correlated with the saturated layer resulting from rainfall infiltration; it is typically less than 2 m deep and negatively correlated with slope steepness. Based on the common characteristics of shallow loess landslides, the mechanisms involved in the sliding-flow landslide are proposed. The Revised Infinite Slope Model (RISM) was introduced using an equal differential unit method to address deficiencies when the safety factor remains constant or increases with increasing slope greater than 40°, as calculated using the Taylor slope infinite model. The relationship between the critical depth and the slope of the shallow loess landslide was determined. The intensity–duration (I–D) prediction curve of the rainfall-induced shallow loess landslides for different slopes was constructed and combined with the characteristics of rainfall infiltration for use in forecasting regional shallow loess landslides. Additionally, the influence of loess strength on the shallow loess landslide stability was analyzed. The shallow loess landslide stability responds to slope and cohesion but is not sensitive to the internal friction angle.
- Article
(12057 KB) - Full-text XML
- BibTeX
- EndNote
Loess is a porous and loose eolian deposit of silt-sized particles mainly formed during the Quaternary period, and it is widely distributed in Asia, Europe, North America, and South America (Li et al., 2019). In China, loess is widely distributed, with an area of 630 000 km2, accounting for 6.63 % of the total land area in northwest, north, and northeast China. The loess deposit reaches a depth of up to 300 m, and the integrity and continuity of the loess layers are unparalleled globally (Liu, 1985). However, environments dominated by loess are exceptionally fragile, experiencing significant soil erosion, topographical variation, and concentrated rainfall, and they have now emerged as some of the most developed geohazard areas in China (Derbyshire, 2001; Zhang and Liu, 2010; Zhuang et al., 2018). Loess is a distinctive soil characterized by its large pores, high compressibility, strong collapsibility, and high-water sensitivity, making it prone to surface failure (Xu et al., 2014; Li et al., 2007; Peng et al., 2015; Juang et al., 2019; Zhuang et al., 2022). The primary factor contributing to loess failure is the interaction between water and loess, which can disrupt the loess structure and diminish its mechanical strength. In over 85 % of loess landslides, water plays a crucial role, exhibiting characteristics such as early small-scale deformation, long runout distance, unpredictable location distribution, rapid occurrence, and liquefaction. These factors lead to severe property damage and human casualties (Dijkstra et al., 1995; Wang et al., 2014; Zhuang et al., 2018; Zhu et al., 2022).
Rainfall-induced slope failures are a common form of shallow landslides in the Chinese Loess Plateau (CLP). Field investigations have revealed that more than 50 000 landslides have occurred in the CLP in recent decades (Zhuang et al., 2018; Zhuang et al., 2022). Most of those landslides were triggered by prolonged heavy rainfall and had a slip surface depth of no more than 2 m (Zhuang et al., 2017, 2018, 2022). Precipitation infiltrates into the soil and percolates through the loess to a depth of approximately 2 m, attributed to the decreased infiltration rate with increasing depth (Tu et al., 2009; Xu et al., 2011; Zhuang et al., 2018). Shallow loess landslide events, due to prolonged heavy rainfall, mostly occur as sliding-flow landslides, occurring in the north of Shaanxi Province in 2013 and 2017 and in Tianshui, Gansu Province, in 2013 and 2015 (Peng et al., 2015; Wang et al., 2015; Zhuang et al., 2017; Zhang et al., 2020). Another impact of these landslides is the significant transportation of sediment into local rivers, resulting in river pollution, elevated riverbeds, and heightened flood risks.
Numerous researchers have investigated rainfall infiltration, landslide mechanisms, and the prediction of rainfall-induced shallow landslides. Among these studies, the most prevalent focus is on forecasting shallow loess sliding-flow landslides caused by rainfall, as this knowledge can be utilized for geohazard mitigation (Brenning, 2005; Bordoni et al., 2015, 2020; Ahmadi-adli et al., 2017; Reichenbach et al., 2018; Thomas et al., 2018; Cogan and Gratchev, 2019; Berti et al., 2020). Shallow-landslide forecasting can be divided into four categories based on the prediction method. (1) The first category is early warning of landslides through monitoring time-based deformation data (von Ruette et al., 2011; Galve et al., 2015; Roccati et al., 2018; Segoni et al., 2018; Lombardo et al., 2020; Marino et al., 2020). The failure processes of most landslides advance through stages of deformation that gradually culminate in catastrophic failure. Throughout this progression, deformation is readily observable and can be monitored, making it the most critical factor for landslide prediction. This approach is particularly pertinent for large-scale landslides with evident early deformation trends. (2) The second category is forecasting landslides through temporal and spatial rainfall monitoring (Giannecchini, 2006; Salciarini et al., 2006; De Vita et al., 2012; Giannecchini et al., 2012; Cevasco et al., 2013; Stähli et al., 2015). Rainfall is a key inducing factor of geohazards, and researchers have studied the critical rainfall values for intensity, duration, and total rainfall within specific areas for predicting landslides. (3) The third category is the utilization of statistical and qualitative methods to assess landslide susceptibility; this, in connection with the occurrence of landslides, can enable the generation of maps delineating hazard zones. These maps are valuable for land use planning and long-term forecasting (Jia et al., 2008; Zizioli et al., 2013; Cevasco et al., 2014; Goetz et al., 2015; Guzzetti et al., 2006; Di Napoli et al., 2020, 2021; Keles and Nefeslioglu, 2021). However, it is important to note that statistical method results are largely dependent on the quality of data and the specific method employed. (4) The fourth category is landslide early warning and forecasting based on physical modeling (Montgomery and Dietrich, 1994; Montrasio and Valentino, 2007; Formetta et al., 2016; Schilirò et al., 2016; Lizarraga et al., 2017; Wang et al., 2020; Leonarduzzi et al., 2021). Researchers have concentrated their efforts on elucidating the mechanisms and conditions that contribute to soil failure. The data for analysis are derived from soil tests, providing evidence of the decay of cohesiveness and angles with precipitation infiltration (Skempton, 1985; Iverson, 2000; Baum et al., 2008; Baum and Godt, 2010; Medina et al., 2021), as well as quantitative landslide assessment. Several physically based models have been proposed, including steady-state hydrology (SHALSTAB and SINMAP) (Montgomery and Dietrich, 1994; Pack et al., 1999), quasi-steady hydrology (dSLAM, IDSSM) (Dhakal and Sidle, 2003), and transient hydrology (TRIGRS) (Iverson, 2000; Baum et al., 2008).
However, due to the occurrence of shallow loess landslides on saturated or nearly saturated steep slopes that transition into loess flow, models such as TRIGRS, which is based on an infinite slope model and is primarily designed for predicting shallow landslides in areas with gentle slopes, are challenging to apply in the CLP (Wang et al., 2015; Zhuang et al., 2017). The SINMAP and SHALAD prediction models are also based on infinite slope models, with the sliding or soil depths being fixed parameters associated with landforms (Montgomery and Dietrich, 1994; Pack et al., 1999; Michel et al., 2020). According to previous studies, shallow loess landslides with sliding-flow landslide characteristics are mainly induced by prolonged heavy rainfall and the sliding surface is the saturated or nearly saturated layer (Wang and Sassa, 2001; Zhang et al., 2013; Peng et al., 2015; Wang et al., 2015; Zhuang et al., 2017; Guo et al., 2019). Due to their small scale and difficult identification, shallow landslides on the CLP are a significant safety threat to local residential areas (Peng et al., 2015; Wang et al., 2015; Guo et al., 2019; Zhang et al., 2020). Given that the majority of shallow loess landslides occur within the upper 2 m and transition into high-speed, long-runout loess flows, the depth of the saturated layer plays a critical role in the study of loess sliding-flow landslides induced by prolonged heavy rainfall. However, there is currently no quantitative assessment for the critical slip depth, making it impossible to develop a rainfall criticality model based on physical processes.
The current study provides a comprehensive evaluation of the distinct characteristics of shallow loess sliding-flow landslides induced by prolonged precipitation. After adjusting for the constant or increasing safety factor with slopes greater than 40°, calculated using the Taylor slope infinite model through the equal differential unit method, we propose the Revised Infinite Slope Model (RISM). The objectives of this study were to determine the sliding depths of the saturated layer at different slopes and to consider rainfall intensity and duration for developing a shallow loess landslide prediction model using loess infiltration characteristics. The model was verified using the 7.25 Tianshui sliding-flow landslide events of 2013 in Tianshui, Gansu Province, a sliding-flow loess landslide triggered by prolonged precipitation.
2.1 Geological and geomorphological characteristics
The study area is a hilly loess region located southwest of the CLP and is part of the transition zone between the Qinling and the Longshan (Peng et al., 2015; Zhang et al., 2020). The loess is deeply and widely distributed with vertical joints and fissures, creating a fragile geological environment where geohazards such as landslides and debris flows frequently occur (Peng et al., 2015; Zhang et al., 2020; Qi et al., 2021). The terrain of the study area is generally high in the southeast and low in the northwest, with altitudes ranging from 748 to 2120 m and a relative height difference of 100 to 1430 m. This geomorphic unit includes the loess hill area comprised of platforms (Yuan in Chinese), ridges (Liang in Chinese), domes (Mao in Chinese), and valleys. The loess hill area is dissected by the Wei River and western Han River along with their tributaries and features numerous ravines (Fig. 1). The hilltops of the loess hills form nearly horizontal surfaces at an elevation ranging from 1900 to 2000 m, with a relative height difference between the ridges of approximately 500 m. The Wei River traverses the loess hilly area from west to east.
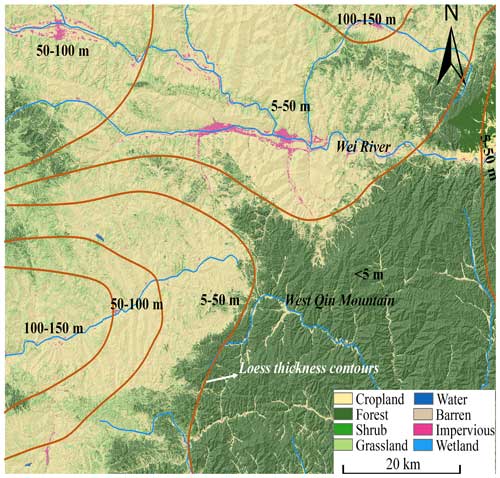
Figure 1The geological and land use of the study area. Base DEM data are from https://geocloud.cgs.gov.cn/#/home (last access: 25 July 2024); land use data are from http://www.geodata.cn (last access: 25 July 2024).
The Tianshui region is located in the western part of the Qilyu–Helan mountain zigzag structural system and the Qinling zonal structural belt. The sub-northeast-trending structural belts and Longxi structural insertions, folds, and compressive faults are highly developed in this area. The neotectonic movement is strong, and the average annual elevation of the mountains is 0.6–8 mm. Additionally, the study area is located within the north–south and Tianshui–Lanzhou seismic belts, with frequent seismic activity (Sun et al., 2017).
2.2 Climate characteristics
The study area is located in a mid-latitude inland region and experiences a cold, temperate, semi-arid, continental monsoon climate with four distinct seasons, characterized by dry winters and springs (low precipitation) and hot and humid summers (high precipitation). The annual average temperature is 10.6 °C, while the annual precipitation ranges from 400 to 700 mm.
Elevation is the primary factor affecting the precipitation amount, with high precipitation in the high-altitude area and low precipitation in the low-altitude area. According to the precipitation data in recent decades (Peng et al., 2015; Zhang et al., 2020), the greatest precipitation occurred in 1967 with 772.2 mm and the least precipitation occurred in 1939 with 316.6 mm. There are also fluctuations in the annual rainfall distribution. Most rainfall is observed from June to September, representing 70 % of the annual precipitation (Peng et al., 2015; Zhang et al., 2020). In July 2013, long-duration and large-scale heavy rainfall resulted in severe flooding and geological disasters.
Beginning on 19 June 2013, prolonged heavy precipitation occurred in the Tianshui region. The total cumulative precipitation and the maximum rainfall intensity of this event represent a 100-year return period (Peng et al., 2015; Qi et al., 2021). This heavy-rainfall event lasted for 37 d and induced a mass sliding-flow landslide disaster. During this extreme-rainfall event, there were four heavy-rainfall stages, with different rainfall periods. The first heavy-rainfall period began on 19 June at 19:00 LT and ended on 21 June at 04:00 LT, with a cumulative rainfall of 285 mm. The second period was from 03:00 to 20:00 LT on 8 July (128.9 mm), and this was followed by the third period from 16:00 LT on 21 July to 04:00 LT on 22 July (43.5 mm). The last period was from 23:00 LT on 24 July to 10:00 LT on 25 July (174.4 mm) (Table 1). Over 45 000 shallow sliding-flow landslides were triggered, characterized by shallow and small scar areas and resulted in the deaths of 25 people.
3.1 Landslide data
High-precision remote sensing imaging data (∼2 m resolution from Google Earth images) from October 2012 (before the sliding-flow landslide event) and December 2013 (after the sliding-flow landslide event), along with field observations, were used to determine that a total of 47 005 sliding-flow landslides occurred in the study area. It can be seen from Fig. 2 that the sliding-flow landslide distribution is primarily concentrated in the middle of the study area along the NNE direction and decreased gradually to the southeast and northwest.
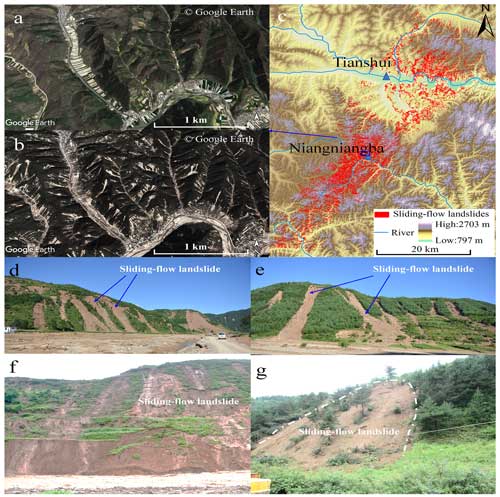
Figure 2The landslides triggered by the 7.25 Tianshui sliding-flow landslide events (a: before the 7.25 Tianshui sliding-flow landslide events in October 2012 of Niangniangba, Tianshui; b: after the 7.25 Tianshui sliding-flow landslide events in December 2013 of Niangniangba, Tianshui; c: the landslide distribution triggered by the 7.25 Tianshui sliding-flow landslide events; d–g: typical sliding-flow landslides of the 7.25 Tianshui sliding-flow landslide events). Base DEM data from https://geocloud.cgs.gov.cn/#/home (last access: 25 July 2024).
The landslides occurred in the shallow loess layer at a depth of no more than 2 m and exhibited sliding-flow landslide characteristics as observed through extensive field investigations. Shallow landslides are typically triggered by prolonged heavy-rainfall events, leading to a rapid increase in pore pressure or loss of cohesion (Iverson, 2000; Wang and Sassa, 2001; Sassa and Wang, 2005; Tu et al., 2009; Zhang et al., 2013). Consequently, a failure surface develops within the soil profile or at the depth of precipitation infiltration. This indicates that the sliding soil layer is close to its liquid-limit water content when the landslide occurs, resulting in flowing characteristics after slope failure.
3.2 Size characteristics
The sliding-flow landslide impacted area is 65.69 km2, and the density is greater than 25 sliding-flow landslides per square kilometer. As shown in Fig. 2, the area of sliding-flow landslides in the town of Niangniangba of the Tianshui region accounts for more than 35 % of the total area, and shallow-flow slips occurred on most of the slopes. The mean sliding-flow landslide area was 0.0013 km2, which is smaller than what is typical of landslides triggered by rainfall or earthquakes (Table 2). Figure 3 shows the proportion of landslides in different areas. It can be seen that most of the sliding-flow landslides were smaller than 2000 m2 and accounted for more than 80 % of the total landslides. Landslides larger than 5000 m2 accounted for only 3 % (Fig. 3), indicating that the 7.25 Tianshui sliding-flow landslide events were primarily small sliding-flow landslides occurring in groups.
Figure 4 depicts the 7.25 Tianshui sliding-flow landslide area, cumulative frequency distribution, and statistical significance of sliding-flow landslides with an area of failure ≥ 5000 m2, accounting for 90 % of the total landslides, and the largest landslide was only 0.099 km2. We examined the area–frequency distribution of the 7.25 Tianshui sliding-flow landslide events via log-binning a normalized non-cumulative size–frequency distribution to plot frequency–density (, where N refers to the number of landslides in each bin) as a function of binned landslide area (A) (Fig. 4) (Stark and Hovius, 2001; Malamud et al., 2004). The higher value a may reflect a greater ability to identify smaller landslides via high-quality imagery. The value for the 7.25 Tianshui sliding-flow landslide events () is higher than the exponents reported for other coseismic inventories. For example, for Northridge, California; for Chi-Chi (alternately Jiji), Taiwan; for Wenchuan, China; and for the average of event-based and historical inventories reported by Van den Eeckhaut et al. (2007) (Roback et al., 2018), also showing that landslides triggered by the 7.25 Tianshui sliding-flow landslide events were primarily small landslides occurring in groups.
3.3 Mobility characteristics
We examined the probability densities of equivalent friction coefficients (landslide vertical height (H) landslide travel distance (L)) for the 7.25 Tianshui sliding-flow landslide events using MATLAB (Fig. 5). The landslide vertical height (H) was based on the altitudes of the highest and the lowest points, and the movement distance was the distance between the highest point and the lowest point. The above two parameters were obtained and calculated using ArcGIS spatial analysis.
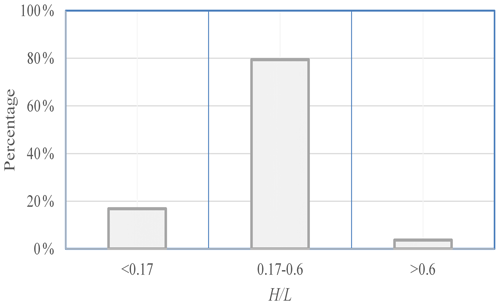
Figure 5The probability densities of equivalent coefficients of friction of the 7.25 Tianshui sliding-flow landslides.
The ratio frequency ratio of the 7.25 Tianshui sliding-flow landslide events ranged from 0.01 to 0.88 with a mean of 0.32. According to a study by Wang (2000), landslide fluidization occurs when the equivalent friction coefficient is below 0.17. In our study, 16.85 % of the loess sliding-flow landslides had equivalent friction coefficients below 0.17, indicating that the loess sliding-flow landslides moved with flow motion and resulted in longer sliding distances. For more than 96.27 % of landslides, was less than 0.6 ( indicates a long-runout landslide).
To model the empirical relationship between sliding-flow landslide height and travel length, which was later verified using images and field investigations, the aforementioned datasets were utilized to generate a plot of H and L on a single graph (Fig. 6), where L (x axis) is the landslide travel distance and H (y axis) is the landslide height.
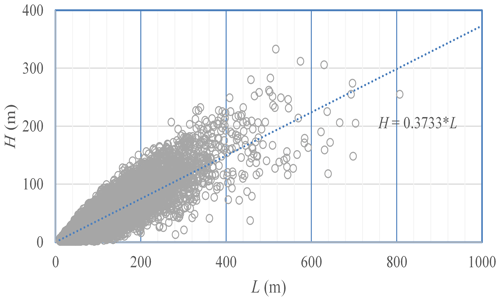
Figure 6The relationship between the height differential and travel distance of the 7.25 Tianshui sliding-flow landslides.
The relationship between height difference and travel distance is positively correlated. With an increase in the height differential, the travel distance increases and the slope of the fitting trend line between the height difference and travel distance is 0.37, indicating that the travel distance is greater than the height difference and showing evident characteristics of a long runout travel distance.
3.4 Sliding depth characteristics
To obtain sliding-flow landslide depths, we conducted a detailed investigation of sites throughout the study area, such as the town of Niangniangba of the Tianshui region, and obtained depth data for 83 landslides based on the characteristics of the sliding-flow landslide and the thickness of the loess scar at the edge of the landslide. Figure 7 displays the distribution of sliding-flow landslide depths and area; no landslide had a depth greater than 2.0 m, and over 70 % ranged from 0 to 1 m (Fig. 7). Additionally, we found that the depth of the sliding-flow landslide had no correlation with the landslide area and had a negative correlation with the slope. With increasing slope, the depth of the landslide decreases; that is, the greater the slope, the shallower the sliding surface, and the smaller the slope, the greater the depth of the sliding surface.
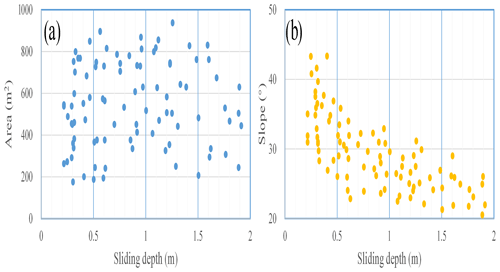
Figure 7The distribution of sliding-flow landslide depths and the area of the 7.25 Tianshui sliding-flow landslides: (a) landslide surface area vs. sliding surface depth and (b) slope vs. sliding surface depth.
Meanwhile, the distribution of the slope and sliding-flow landslides in the study area was analyzed statistically. It was observed that the majority of sliding-flow landslides had a gradient value within the range of 30–50°, while landscape areas were concentrated in the range of 10–30°. Furthermore, sliding-flow landslides with slopes greater than 50° accounted for 13.81 % in the study area. Consequently, there is a higher probability of failure for sliding-flow landslides on steep slopes (50°) in the CLP (Fig. 8).
3.5 The shallow sliding-flow landslide formation process
Force analysis shows that a slope will fail when the gravity component along the slope direction is greater than the shear strength of the soil (Sassa, 2000; Ochiai et al., 2004; Gabet and Mudd, 2006). If the stability coefficient of the slope is high and the soil reaches its liquid-limit water content before the failure, the slope will spontaneously liquefy and flow during the sliding process (Wang and Sassa, 2001; Wang et al., 2015). Usually, the ratio of pore-water pressure to the total normal stress of the soil is used to express the soil liquefaction ratio. When the liquefaction rate is 1, the pore-water pressure equals the normal stress of the soil and the failing slope is in a state of complete liquefaction (Hungr et al., 2001; Wang and Sassa, 2001; Sassa and Wang, 2005; Wang et al., 2015). Typically, the normal stress of shallow landslides is low and the slope deformation is attributed to decreased strength. The soil has an obvious volume reduction due to the large pore structure of the collapsing soil structure and is followed by a sharp increase in the pore-water pressure (Wang and Sassa, 2001; Sassa and Wang, 2005; Peng et al., 2018). Shallow sliding-flow landslides are triggered by prolonged heavy precipitation and rainfall infiltration to a certain loess depth (mostly 1–2 m) close to the liquid-limit water content, which is followed by a decrease in the soil strength (Tu et al., 2009; Xu et al., 2011; Zhuang et al., 2018). When the anti-slip force of the saturated soil is less than the sliding force, the saturated soil layer will fail. Due to the large pore structure of the soil, once soil deformation occurs, the water in the pores cannot be released, resulting in the pore-water pressure increasing sharply and causing liquefaction of the saturated soil to form a mudflow (Fig. 9).
4.1 Infinite slope model
According to field investigations and other research results, the shallow sliding-flow landslides are concentrated within 2 m of the surface and are negatively correlated with slope (Tu et al., 2009; Xu et al., 2011; Zhuang et al., 2017, 2018, 2022). Therefore, infinite slope models that take into account the soil layer thickness and maximum infiltration depth cannot be directly applied in shallow-landslide assessments. Previous studies have indicated that short-duration heavy precipitation has a lower impact on the stability of loess slopes, while prolonged heavy precipitation significantly increases water content and reduces slope stability (Wang and Sassa, 2001; Wang et al., 2015; Peng et al., 2018). Shallow-landslide transformation into mudflows occurs most often on slopes of 25 to 45°, and the sliding body is close to the liquid-limit water content before slope instability. Pore-water pressure is a key factor for soil slope failure (Iverson, 2000). Previous studies have demonstrated that pore-water pressure is the primary cause of soil landslides, and excess pore-water pressure serves as the triggering factor for fluidization (Iverson et al., 1997; Sassa and Wang, 2005; Gabet and Mudd, 2006). The failure of the sliding body is mainly attributed to a reduction in soil strength, leading to an increase in the sliding force exceeding cohesive forces following rainfall infiltration that saturates the soil (Wang et al., 2015; Peng et al., 2018).
According to the infinite slope model (Fig. 10), Taylor proposed the following safety factor equation (Taylor, 1948):
where α is the slope angle, γw is the soil floating weight, γsat is the saturation weight of the soil, hw is the depth of the sliding surface, c′ (cohesive) is the approximate depth of loess at the liquid-limit water content due to infiltration, and ϕ′ (internal friction angle) is the effective strength index of the soil at the sliding surface.
Using the mean strength of the saturated loess, the stability factor is calculated according to the infinite slope model proposed by Taylor (1948). The results are shown in Fig. 11.
Figure 11 shows that for a cohesive soil slope, the safety factor decreases with increasing slope when the slope is less than 40°. There is little difference for slopes from 40 to 50°; however, the safety factor increases when the slope is greater than 50°. The calculated results exhibit discrepancies with the actual conditions, particularly regarding the aspect of the model indicating an increase in the safety factor with increasing slope. However, most shallow-landslide physical prediction models (e.g., TRIGRS, SINMAP, SHALAD) are based on Taylor's infinite slope model. As a result, these models cannot be effectively applied to areas with high slopes (>40°), limiting their widespread applicability (Montgomery and Dietrich, 1994; Baum et al., 2008; Zhuang et al., 2017).
4.2 The Revised Infinite Slope Model
To ensure the consistency of Taylor's infinite slope model calculation results with actual conditions, this study has made modifications to the model using an equal differential unit method. As depicted in Fig. 12, by maintaining a constant depth of the saturation zone as the slope increases, the formula for calculating the self-weight of the soil strip unit has been revised as follows:
where b is the length of the sliding body and Δα is the value of the increasing slope.
In Eq. (2), the self-weight of the soil strip unit and the control condition of the soil strip area are unchanged:
Equation (2) shows that, although the change in slope does not affect the self-weight of the differential element, it leads to a change in the bottom area of the element, which causes the cohesion strength and pore-water pressure of the bottom surface of the soil strip element to change. The change in slope only influences the component values of forces in normal and tangential directions on the sliding surface. Therefore, when evaluating slope stability with different slopes, it is essential to account for changes in cohesion strength and pore-water pressure caused by an increase in slope Δα.
The change in cohesive strength (ΔC) and pore-water pressure (Δub) resulting from increasing the slope of Δα are described as
The effective normal stress (N′) and effective anti-sliding force (Tf) at the bottom of the soil strip element due to the slope increasing from α to α+Δα are expressed as
The sliding force of the soil strip unit is changed to
The revised effective anti-slip force according to Eqs. (4), (5), and (7) is
Combining Eqs. (8) and (9), the safety factor can be obtained:
Making α=α1, , and , Eq. (10) can be expressed as
Making , for a simple equation, the term c′secα1 in Eq. (11) can be defined as the reference cohesion strength. Since the value of c′secα1 varies monotonically with α1 changing, to make the reference cohesion strength corresponding to any slope angle equal, it only needs to satisfy the condition that α1 is less than or equal to α2:
Therefore, the safety factor of the RISM can be expressed as
Using the mean strength of saturated loess in the loess area, the stability is calculated according to the RISM proposed by this study, and the results are shown in Fig. 13. According to the equal differential unit method, the RISM corrects the safety factor, which increases with the slope increasing when the slope is larger than 50° (calculated using the Taylor slope infinite model). The results obtained by the RISM maintain consistency with the calculation results of the Taylor method at low angles, and the calculated results decrease with an increased slope when the slope is larger than 40°.
4.3 Critical depth of shallow loess landslides
When the stability coefficient K is 1 in Eq. (13), the critical depth of loess close to liquid-limit water content or the sliding surface of the shallow loess landslide can be obtained:
The critical depth of the liquid-limit water content or the sliding surface of the shallow loess landslide for different slopes can be determined by obtaining the soil strength of the nearly saturated state. Upon reaching the critical depth of the liquid-limit water content, the layer will experience failure. The cohesion and angle of internal friction were tested using the triaxial test method at saturation following the soil test standard GBT50123-1999. According to the loess test results, the cohesive forces of undisturbed loess at the liquid-limit water content (0–2 m) range from 3 to 9 kPa with an average of 5 kPa, and the internal friction angle ranges from 11 to 21° with an average of 15° (Fig. 14). Therefore, the relationship between the critical approximate depth of the liquid-limit water content and the slope can be calculated. With increasing slope, the critical approximate liquid-limit water content layer gradually decreases, but the rate of decrease slows, from 1.14 m at 20° to 0.47 m at 40° (Fig. 14). This relationship can be expressed via the power law as
4.4 The I–D curve of the loess shallow landslide
The critical rainfall intensity–duration (I–D) method is often used in forecasting rainfall-induced shallow landslides (Guzzetti et al., 2007, 2008; Baum and Godt, 2010; Zhuang et al., 2015). Thresholds empirically derived from rainfall intensity–duration have been widely used to identify rainfall conditions that result in the occurrence of landslides (Guzzetti et al., 2007, 2008; Baum and Godt, 2010). Inspection of the I–D thresholds reveals the following general form:
where I is the mean rainfall intensity; D is rainfall duration; and c, β, and b are other parameters. For the majority of I–D thresholds, c=0, and Eq. (16) takes the form of a simple power law.
Previous studies have created statistical I–D curves based on landslide data and rainfall data. However, these empirical models require many years of precipitation data and calibration parameters. Even so, the determination of the landslide threshold I–D curve is inaccurate due to the uncertainty in rainfall monitoring, such as the location and quantity of monitoring sites and the definition of the start and end time of rainfall events (Zhuang et al., 2015; Guo et al., 2016).
The critical I–D curve for slope instability for different slopes can be constructed using the model of the critical approximate depth of the liquid-limit water content combined with the saturated infiltration characteristics of loess in the study area. Six infiltration tests were carried out in the study area using the single-ring infiltration test to determine the infiltration coefficient (i) of loess under rainfall. The constant infiltration rates were 38.6, 33.1, 39.1, 32.2, 36.2, and 31.6 mm h−1. Additionally, it was observed that the time between initial infiltration and stable infiltration is less than 10 min. Therefore, in this study, the average stable infiltration rate of 36.0 mm h−1 was selected as the infiltration coefficient.
To model the relationship between I and D, the two variables were plotted on a single graph, where D (x axis) is the rainfall duration and I (y axis) is the rainfall intensity. According to the critical sliding surface depth with different slopes (Eq. 14), curves I and D with different slopes can be calculated using Eqs. (7)–(9). The I–D curve of the different slopes in the area can be obtained based on the infiltration coefficient and the sliding depth (Fig. 15).
5.1 Model comparison
Upon comparing the I–D curves for different slopes with existing models, it was observed that the I–D curves derived from the physical model in this study exhibit higher values compared to other statistical and probabilistic models. Additionally, while the I–D curves for other regions are obtained through statistical methods, any individual landslide occurrence within this area is considered a separate event. Meanwhile, many researchers have pointed out that antecedent rainfall plays a significant role in triggering landslides in loess areas, which is different for other regions, such as Hong Kong SAR, fire-impacted areas in the USA, and the southwest mountains in China (Cui et al., 2008; Zhuang et al., 2015). Therefore, the I–D curves of other areas will be lower than the I–D curve constructed based on physical models (Fig. 16).
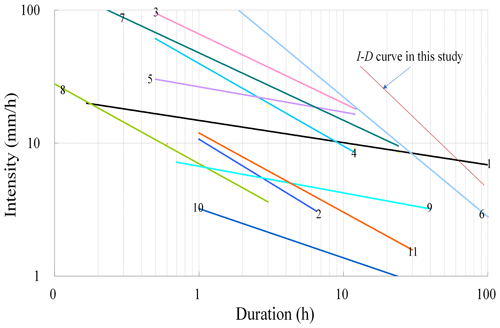
Figure 16Comparison of I–D curves from the current study and prior studies (no. 1: Caine, 1980; no. 2: Wieczorek, 1987; nos. 3, 4, 5: Jibson, 1989; no. 6: Guadagno, 1991; no. 7: Paronuzzi et al., 1998; no. 8: Crosta and Frattini, 2001; no. 9: Shieh et al., 2009; no. 10: Guo et al., 2013; no. 11: Zhuang et al., 2015).
From the distribution of shallow landslides in this area, it can be seen that these landslides mainly occur on slopes of 35 to 50°. Marking the rainfall duration and intensity of the 7.25 Tianshui sliding-flow landslide events and the “7.13”-group shallow landslide in Yan'an in 2013 (Wang et al., 2015; Zhuang et al., 2017) on the I–D line, it can be seen that the rainfall duration and intensity of both events are above the I–D curve of 35° (Fig. 15), indicating that the constructed curve is reliable and can be used to forecast shallow landslides in this area.
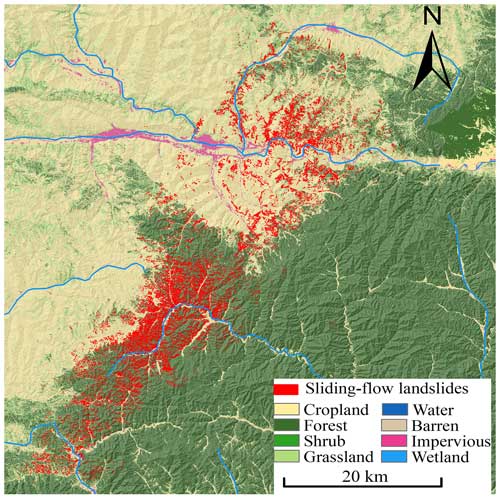
Figure 17The 7.25 Tianshui sliding-flow landslides and land use in the study area (base DEM data from https://geocloud.cgs.gov.cn/#/home, last access: 25 July 2024; land use data from http://www.geodata.cn, last access: 25 July 2024).
5.2 Model limitations
Previous studies have assumed that restoration of vegetation is beneficial for preventing landslides, mainly because of the reinforcement effect of roots on soil (Waldron, 1977; Roering et al., 2003). However, the inhibitory effect of vegetation on landslides is gradually being questioned due to research on the negative relationship between vegetation and landslides in recent mass landslides in loess and other well-vegetated areas. For example, the “9.16” mass shallow landslides in Changning, Yunnan, in 2014; the Yan'an “7.13” mass shallow-landslide event; the 2015 Tianshui mass shallow-landslide event; and the 2010 Nanping mass shallow-landslide event in Fujian Province all had mass shallow landslides induced by prolonged heavy precipitation, often occurring in well-vegetated areas (Fig. 17; Wang et al., 2015; Peng et al., 2015; Zhuang et al., 2017, 2020). Due to the complexity of the influence of vegetation on slope stability, most studies have not clarified whether the vegetation induces or inhibits landslides (Rickli and Graf, 2009; Preti, 2013; Zhuang et al., 2022). In general, plant roots can reinforce the slope soil and improve the shear strength of the soil (Waldron, 1977; Roering et al., 2003). However, the increase in vegetation can also result in greater stored precipitation and infiltration into the soil (Wang et al., 2015; Zhuang et al., 2017, 2022). Plant root systems also provide channels for preferential flow, resulting in higher soil water content, which promotes the occurrence of shallow landslides (Zhuang et al., 2017, 2022). The vegetation on the CLP is often shrubs with shallow root systems that do not exceed the sliding surface. Since most shallow landslides slide along the bottom of the root system, according to field investigations, they do not prevent these landslides (Wang et al., 2015). Slopes with good vegetation coverage increase the weight of the sliding body during precipitation. The prediction model proposed in this study does not consider the impact of vegetation on shallow landslides. According to data from monitoring numerous loess areas, increased restoration of vegetation results in more rainwater infiltrating into the loess, increasing the water content of the shallow loess, and results in an increased possibility of shallow landslides (Xu et al., 2024). There is not only downward vertical infiltration but also horizontal infiltration along the slope direction in the process of rainfall infiltration on the slope surface, resulting in the confluence of water in the slope body (Wang et al., 2015; Zhuang et al., 2022).
At the same time, according to the assumption of the infinite slope model, the model is mainly applicable to when the slope extent is much larger than the depth of potential slip surface, the slope angle is not too large, and soil characteristics are homogeneous along the slope direction, which means that the infinite slope model is only suitable for the prediction of homogeneous soil slope instability due to rainfall (Taylor, 1948). For slopes constituted of binary structures, analysis of slope failure controlled by the strength of the structure of the rock–soil mass is often not able to produce a correct factor of safety for the slope. Therefore, the RISM is also suitable for homogeneous soil slopes, and slope extent is undefined or much larger than the depth of the potential slip surface but can be applied to steeper slopes in the CLP.
5.3 Sensitivity analysis
Dry loess has high cohesive strength but loses strength significantly when wetted (Derbyshire et al., 2001; Zhuang et al., 2018), and it has water-sensitive characteristics, with the strength parameters changing rapidly with the water content increasing. According to existing research, the cohesion of loess can be reduced from greater than 50 kPa at low water content to less than 10 kPa at high water content (Zhuang et al., 2018). The internal friction angle of loess varies slightly, generally from about 25° in low water content to about 16° in a saturated state. To assess the loess strength influence on shallow loess landslides caused by prolonged heavy precipitation, the response of the slope stability to the strength change in saturated loess was calculated. Our results demonstrate that slope stability is greatly affected by cohesion, while the stability of the slope is less responsive to changes in the internal friction angle. Meanwhile, by changing the slope and fixing loess strength, the relationship between the safety factor and slope was obtained. The safety factor varies obviously with slope change, indicating that the cohesion and slope are key factors affecting soil stability and shallow landslides, whereas the internal friction angle has a minimal effect on shallow landslides in loess areas (Fig. 18).
A rainfall-induced slope failure is a common cause of shallow landslides in the CLP. This study examines the shallow loess landslide triggered by prolonged heavy rainfall on 25 July 2013, in Tianshui, China, as a case study. The following results were obtained:
-
The 7.25 Tianshui sliding-flow landslide event triggered 47 005 landslides with a total area of 65.69 km2, and the mean landslide area was 0.0013 km2, which is smaller than most landslides triggered by other rainfall or earthquakes. Most of the landslides evaluated (80 %) are smaller than 2000 m2, and landslides larger than 5000 m2 accounted for only 3 %, indicating that the 7.25 Tianshui sliding-flow landslide events are primarily small landslides with group occurrence characteristics.
-
The ratio frequency ratio of the 7.25 Tianshui sliding-flow landslide event ranged from 0.01 to 0.88 with a mean of 0.32. The equivalent coefficient of friction was below 0.17 for 16.85 % of the loess landslides, indicating that loess landslides travel via flow motion and resulting in longer sliding distances.
-
The Revised Infinite Slope Model (RISM) was proposed using an equal differential unit method that corrects for any deficiencies associated with increased safety factors on slopes that are invariable or increasing when the slope is greater than 40° according to the Taylor slope infinite model.
-
The depth of the critical approximate liquid-limit water content (also the sliding surface depth) of the shallow loess landslides with different slopes can be described as . The critical I–D curve for slope instability for different slopes was constructed using the infiltration characteristics of loess in the study area.
The data in this study were analyzed with Excel, and the figures were created with ArcView GIS and Excel. All codes and data used in this work are available upon request.
Conceptualization: JZ and JB; methodology: JZ and CH; investigation: JZ, JB, JX, and YZ; data curation: JZ and JX; writing – original draft preparation: JZ; writing – review and editing: JZ; project administration: JZ; funding acquisition: JZ. All authors have read and agreed to the published version of the paper.
The contact author has declared that none of the authors has any competing interests.
Publisher's note: Copernicus Publications remains neutral with regard to jurisdictional claims made in the text, published maps, institutional affiliations, or any other geographical representation in this paper. While Copernicus Publications makes every effort to include appropriate place names, the final responsibility lies with the authors.
The authors are very grateful to Hyuck-Jin Park, the anonymous reviewers, and the editors for their thoughtful review comments and suggestions, which have significantly improved this paper. This study was financially supported by the National Natural Science Foundation of China (grant nos. 42090053, 41922054). The authors thank AiMi Academic Services (https://www.aimieditor.com/, last access: 25 July 2024) for the English language editing and review services of an earlier version of this paper.
This research has been supported by the National Natural Science Foundation of China (grant nos. 41922054 and 42090053).
This paper was edited by Paolo Tarolli and Filippo Catani and reviewed by Hyuck-Jin Park and three anonymous referees.
Ahmadi-adli, M., Huvaj, N., and Toker, N.: Rainfall-triggered landslides in an unsaturated soil: a laboratory flume study, Environ. Earth Sci., 76, 735, https://doi.org/10.1007/s12665-017-7049-z, 2017.
Baum, R. L. and Godt, J. E.: Early warning of rainfall-induced shallow landslides and debris flows in the USA, Landslides, 7, 259–272, https://doi.org/10.1007/s10346-010-0204-1, 2010.
Baum, R. L., Savage, W. Z., and Godt, J. W.: TRIGRS-A FORTRAN program for transient rainfall infiltration and gridbased regional slope stability analysis, version 2.0, US Geological Survey Open-File Report 2008-1159, US Geological Survey, 75 pp., https://pubs.usgs.gov/of/2008/1159/ (last access: 25 July 2024), 2008.
Berti, M., Bernard, M., Gregoretti, C., and Simoni, A.: Physical interpretation of rainfall thresholds for runoff-generated debris flows, J. Geophys. Res.-Earth, 125, e2019JF005513, https://doi.org/10.1029/2019JF005513, 2020.
Bordoni, M., Meisina, C., Valentino, R., Lu, N., Bittelli, M., and Chersich, S.: Hydrological factors affecting rainfall-induced shallow landslides: From the field monitoring to a simplified slope stability analysis, Eng. Geol., 193, 19–37, https://doi.org/10.1016/j.enggeo.2015.04.006, 2015.
Bordoni, M., Galanti, Y., Bartelletti, C., Persichillo, M. G., Barsanti, M., Giannecchini, R., Avanzi, G. D., Cevasco, A., Brandolini, P., Galve, J. P., and Meisina, C.: The influence of the inventory on the determination of the rainfall-induced shallow landslides susceptibility using generalized additive models, Catena, 193, 104630, https://doi.org/10.1016/j.catena.2020.104630, 2020.
Brenning, A.: Spatial prediction models for landslide hazards: review, comparison and evaluation, Nat. Hazards Earth Syst. Sci., 5, 853–862, https://doi.org/10.5194/nhess-5-853-2005, 2005.
Bucknam, R. C., Coe, J. A., Chavarría, M. M., Godt, J. W., Tarr, A. C., Bradley, L. A., Rafferty, S., Hancock, D., Dart, R. L., and Johnson, M. L.: Landslides triggered by Hurricane Mitch in Guatemala – inventory and discussion, Open-File Rep. 2001–443, US Geol. Surv., 38 pp., https://doi.org/10.3133/ofr01443, 2001.
Caine, N.: The rainfall intensity-duration control of shallow landslides and debris flows, Geogr. Ann. A, 62, 23–27, https://doi.org/10.1007/s10346-007-0112-1, 1980.
Cevasco, A., Brandolini, P., Scopesi, C. and Rellini, I.: Relationships between geo-hydrological processes induced by heavy rainfall and land-use: The case of 25 October 2011 in the Vernazza catchment (Cinque Terre, NW Italy), J. Maps, 9, 289–298, https://doi.org/10.1080/17445647.2013.780188, 2013.
Cevasco, A., Pepe, G., and Brandolini, P.: The influences of geological and land use settings on shallow landslides triggered by an intense rainfall event in a coastal terraced environment, Bull. Eng. Geol. Environ., 73, 859–875, https://doi.org/10.1007/s10064-013-0544-x, 2014.
Cogan, J. and Gratchev, I.: A study on the effect of rainfall and slope characteristics on landslide initiation by means of flume tests, Landslides, 16, 2369–2378, https://doi.org/10.1007/s10346-019-01261-0, 2019.
Crosta, G. B. and Frattini. P.: Rainfall thresholds for triggering soil slips and debris flow, in: Proc. 2nd EGS Plinius Conf. on Mediterranean Storms, 16–18 October 2000, Siena, 463–487, https://boa.unimib.it/handle/10281/38124 (last access: 26 July 2024), 2001.
Cui, P., Zhu, Y. Y., and Chen, J.: Relationships between antecedent rainfall and debris flows in Jiangjia Ravine, China, In: Proceedings of the Fourth International Conference on Debris Flow, edited by: Chen, C. L. and Rickenmann, D., Springer Press, Wellington, 1–9, https://webofscience.clarivate.cn/wos/alldb/summary/c8412688-7797-4f83-adb1-214f3747ca8f-ed3972c7/relevance/1 (last access: 26 July 2024), 2008.
Dai, F. C., Xu, C., Yao, X., Xu, L., Tu, X. B., and Gong, Q. M.: Spatial distribution of landslides triggered by the 2008 Ms 8.0 Wenchuan earthquake, China, J. Asian Earth Sci., 40, 883-895, https://doi.org/10.1016/j.jseaes.2010.04.010, 2011.
Derbyshire, E.: Geological hazards in loess terrain, with particular reference to the loess regions of China, Earth-Sci. Rev., 54, 231–260, https://doi.org/10.1016/S0012-8252(01)00050-2, 2001.
De Vita, P., Napolitano, E., Godt, J. W., and Baum, R. L.: Deterministic estimation of hydrological thresholds for shallow landslide initiation and slope stability models: Case study from the Somma-Vesuvius area of southern Italy, Landslides, 10, 713–728, https://doi.org/10.1007/s10346-012-0348-2, 2012.
Dhakal, A. S. and Sidle, R. C.: Long-term modelling of landslides for different forest management practices, Earth Surf. Proc. Land., 28, 853–868, https://doi.org/10.1002/esp.499, 2003.
Dijkstra, T. A., Rogers, C. D. F., and van Asch, T. W. J.: Cut slope and terrace edge failures in Malan loess, Lanzhou, PR China, in: Proceedings of the XI ECSMFE conference, 28 May–1 June 1995, Copenhagen, 61–67, https://eurekamag.com/research/018/665/018665744.php (last access: 29 July 2024), 1995.
Di Napoli, M., Carotenuto, F., Cevasco, A., Confuorto, P., Di Martire, D., Firpo, M., Pepe, G., Raso, E., and Calcaterra, D.: Machine learning ensemble modelling as a tool to improve landslide susceptibility mapping reliability, Landslides, 17, 1897–1914, https://doi.org/10.1007/s10346-020-01392-9, 2020.
Di Napoli, M., Di Martire, D., Bausilio, G., Calcaterra, D., Confuorto, P., Firpo, M., Pepe, G., and Cevasco, A.: Rainfall-Induced Shallow Landslide Detachment, Transit and Runout Susceptibility Mapping by Integrating Machine Learning Techniques and GIS-Based Approaches, Water, 13, 488, https://doi.org/10.3390/w13040488, 2021.
Formetta, G., Capparelli, G., and Versace, P.: Evaluating performance of simplified physically based models for shallow landslide susceptibility, Hydrol. Earth Syst. Sci., 20, 4585–4603, https://doi.org/10.1016/j.enggeo.2009.12.004, 2016.
Gabet, E. J. and Mudd, S. M.: The mobilization of debris flows from shallow landslides, Geomorphology, 74, 207–218, https://doi.org/10.1016/j.geomorph.2005.08.013, 2006.
Galve, J. P., Cevasco, A., Brandolini, P., and Soldati, M.: Assessment of shallow landslide risk mitigation measures based on land use planning through probabilistic modelling, Landslides, 12, 101–114, https://doi.org/10.1007/s10346-014-0478-9, 2015.
Giannecchini, R.: Relationship between rainfall and shallow landslides in the southern Apuan Alps (Italy), Nat. Hazards Earth Syst. Sci., 6, 357–364, https://doi.org/10.5194/nhess-6-357-2006, 2006.
Giannecchini, R., Galanti, Y., and D'Amato, A. G.: Critical rainfall thresholds for triggering shallow landslides in the Serchio River Valley (Tuscany, Italy), Nat. Hazards Earth Syst. Sci., 12, 828–842, https://doi.org/10.5194/nhess-12-829-2012, 2012.
Goetz, J. N., Brenning, A., Petschko, H., and Leopold, P.: Evaluating machine learning and statistical prediction techniques for landslide susceptibility modeling, Comput. Geosci., 81, 1–11, https://doi.org/10.1016/j.trc.2015.03.039, 2015.
Guadagno, F. M.: Debris flows in the Campanian volcaniclastic soil (Southern Italy), in: Proc. Int. Conf. on slope stability, Isle of Wight, Thomas Telford, 125–130, https://doi.org/10.1680/ssedaa.16606.0021, 1991.
Guo, W., Luo, L., Wang, W., Liu, Z., Chen, Z., Kang, H., and Yang, B.: Sensitivity of rainstorm-triggered shallow mass movements on gully slopes to topographical factors on the Chinese Loess Plateau, Geomorphology, 337, 69–78, https://doi.org/10.1016/j.geomorph.2019.04.006, 2019.
Guo, X. J., Cui, P., and Li, Y.: Debris flow warning threshold based on antecedent rainfall: A case study in Jiangjia Ravine, Yunnan, China, J. Mount. Sci., 10, 305–314, https://doi.org/10.1007/s11629-013-2521-z, 2013.
Guo, X. J., Cui, P., Li, Y., Ma, L., Ge, Y. G., and William, B. M.: Intensity-duration threshold of rainfall-triggered debris flows in the Wenchuan Earthquake affected area, China, Geomorphology, 253, 208–216, https://doi.org/10.1016/j.geomorph.2015.10.009, 2016.
Guzzetti, F., Malamud, B. D., Turcotte, D. L., and Reichenbach, P.: Power-law correlations of landslide areas in central Italy, Earth Planet. Sc. Lett., 195, 169–183, https://doi.org/10.1016/S0012-821X(01)00589-1, 2002.
Guzzetti, F., Galli, M., Reichenbach, P., Ardizzone, F., and Cardinali, M.: Landslide hazard assessment in the Collazzone area, Umbria, Central Italy, Nat Hazards Earth Syst Sci., 6, 115–131, https://doi.org/10.5194/nhess-6-115-2006, 2006.
Guzzetti, F., Peruccacci, S., Rossi, M., and Stark, C. P.: Rainfall thresholds for the initiation of landslides in central and southern Europe, Meteorol. Atmos. Phys., 98, 239–267, https://doi.org/10.1007/s00703-007-0262-7, 2007.
Guzzetti, F., Peruccacc, S., Ross, M., and Stark, C. P.: The rainfall intensity–duration control of shallow landslides and debris flows: an update, Landslides, 5, 3–17, https://doi.org/10.1007/s10346-007-0112-1, 2008.
Harp, E. L. and Jibson, R. L.: Landslides triggered by the 1994 Northridge, California earthquake, Bull. Seismol. Soc. Am., 86, S319–S332, https://doi.org/10.1029/95JB03253, 1996.
Hungr, O., Evans, S. G., Bovis, M., and Hutchinson, J. N.: Review of the classification of landslides of the flow type, Environ. Eng. Geosci., 7, 221–238, https://doi.org/10.2113/gseegeosci.7.3.221, 2001.
Iverson, R. M.: Landslide triggering by rain infiltration, Water Resour. Res., 36, 1897–1910, https://doi.org/10.1029/2000WR900090, 2000.
Iverson, R. M., Reid, M. E., and LaHusen, R. G.: Debris-flow mobilization from landslide, Annu. Rev. Earth Planet. Sci., 25, 85–138, https://doi.org/10.1146/annurev.earth.25.1.85, 1997.
Jia, G., Yuan, T., Liu, Y. and Zhang, Y.: A static and dynamic factors-coupled forecasting model or reg. E, 51, 164–175, https://doi.org/10.1007/s11431-008-6013-2, 2008.
Jibson, R. W.: Debris flow in southern Porto Rico, special paper 236, Geological Society of America, 29–55, https://doi.org/10.1130/SPE236-p29, 1989.
Juang, C. H., Dijkstra, T., Wasowski, J., and Meng, X. M.: Loess geohazards research in china: advances and challenges for mega engineering projects, Eng. Geol., 251, 1–10, https://doi.org/10.1016/j.enggeo.2019.01.019, 2019.
Keles, F. and Nefeslioglu, H. A.: Infinite slope stability model and steady-state hydrology-based shallow landslide susceptibility evaluations: The Guneysu catchment area (Rize, Turkey), Catena, 200, 105161, https://doi.org/10.1016/j.catena.2021.105161, 2021.
Leonarduzzi, E., McArdell, B. W., and Molnar, P.: Rainfall-induced shallow landslides and soil wetness: comparison of physically based and probabilistic predictions, Hydrol. Earth Syst. Sci., 25, 5937–5950, https://doi.org/10.5194/hess-25-5937-2021, 2021.
Li, T. L., Long, J. H., and Li, X. S.: Types of loess landslides and methods for their movement forecast, J. Eng. Geol., 15, 500–506, 2007.
Li, Y. R., Shi, W., Aydin, A., Beroya-Eitnerc, M. A., and Gao, G. H.: Loess genesis and worldwide distribution, Earth-Sci. Rev., 201, 1–99, https://doi.org/10.1016/j.earscirev.2019.102947, 2019.
Lin, M. L. and Tung, C. C.: A GIS-based potential analysis of the landslides induced by the Chi-Chi earthquake, Eng. Geol., 71, 63–77, https://doi.org/10.1016/S0013-7952(03)00126-1, 2004.
Liu, T. S.: Loess and the Environment, Science Press, Beijing, China, ISBN 130312999, 1985.
Lizarraga, J. J., Frattini, P., Crosta, G. B., and Buscarnera, G.: Regional-scale modelling of shallow landslides with different initiation mechanisms: sliding versus liquefaction, Eng. Geol., 228, 346–356, https://doi.org/10.1016/j.enggeo.2017.08.023, 2017.
Lombardo, L., Opitz, T., Ardizzone, F., Guzzetti, F., and Huser, R.: Space-time landslide predictive modelling, Earth-Sci. Rev., 209, 103318, https://doi.org/10.1016/j.earscirev.2020.103318, 2020.
Malamud, B. D., Turcotte, D. L., Guzzetti, F., and Reichenbach, P.: Landslides, earthquakes, and erosion, Earth Planet. Sc. Lett., 229, 45–59, https://doi.org/10.1016/j.epsl.2004.10.018, 2004.
Marino, P., Peres, D. J., Cancelliere, A., Greco, R., and Bogaard, T. A.: Soil moisture information can improve shallow landslide forecasting using the hydrometeorological threshold approach, Landslides, 17, 2041–2054, https://doi.org/10.1007/s10346-020-01420-8, 2020.
Medina, V., Hürlimann, M., Guo, Z., Lloret, A., and Vaunat, J.: Fast physically-based model for rainfall-induced landslide susceptibility assessment at regional scale, Catena, 201, 105213, https://doi.org/10.1016/j.catena.2021.105213, 2021.
Michel, J., Dario, C., Marc-Henri, D., Thierry, O., and Bejamin, R.: A review of methods used to estimate initial landslide failure surface depths and volumes, Eng. Geol., 267, 105478, https://doi.org/10.1016/j.enggeo.2020.105478, 2020.
Montgomery, D. R. and Dietrich, W. E.: A physically based model for the topographic control on shallow landsliding, Water Resour. Res., 30, 1153–1171, https://doi.org/10.1029/93WR02979, 1994.
Montrasio, L. and Valentino, R.: Experimental analysis and modelling of shallow landslides, Landslides, 4, 291–296, https://doi.org/10.1007/s10346-007-0082-3, 2007.
Ochiai, H., Okada, Y., Furuya, G., Okura, Y., Matsui, T., Sammori, T., Terajima, T., and Sassa, K.: A fluidized landslide on a natural slope by artificial rainfall, Landslides, 1, 211–219, https://doi.org/10.1007/s10346-004-0030-4, 2004.
Owen, L. A., Kamp, U., Khattak, G. A., Harp, E. L., Keefer, D. K., and Bauer, M. A.: Landslides triggered by the 8 October 2005 Kashmir earthquake, Geomorphology, 94, 1–9, https://doi.org/10.1016/j.geomorph.2007.04.007, 2008.
Pack, R. T., Tarboton, D. G., and Goodwin, C. N.: SINMAP 2.0 – A Stability Index Approach to Terrain Stability Hazard Mapping, User's Manual, Terratech Consulting Ltd., Salmon Arm, Canada, https://digitalcommons.usu.edu/cgi/viewcontent.cgi?article=1015&context=cee_facpub (last access: 29 July 2024), 1999.
Paronuzzi, P., Coccolo, A., and Garlatti, G.: Eventi meteorici critical debris flows nei bacini montani del Friuli. L'Acqua, Sezione I=Memorie, L'Acqua, 6, 39–50, http://hdl.handle.net/11390/675800 (last access: 29 July 2024), 1998.
Peng, J. B., Fan, Z. J., Wu, D., Zhuang, J. Q., Dai, F. C., Chen, W. W., and Zhao, C.: Heavy rainfall triggered loess–mudstone landslide and subsequent debris flow in Tianshui, China, Eng. Geol., 186, 79–90, https://doi.org/10.1016/j.enggeo.2014.08.015, 2015.
Peng, J. B., Zhuang, J. Q., Wang, G. H., Dai, F. C., Zhang, F. Y., Huang, W. L., and Xu, Q.: Liquefaction of loess landslides as a consequence of irrigation, Q. J. Eng. Geol. Hydrogeol., 51, 330–337, https://doi.org/10.1144/qjegh2017-098, 2018.
Preti, F.: Forest protection and protection forest: Tree root degradation over hydrological shallow landslides triggering, Ecol. Eng., 61, 633–645, https://doi.org/10.1016/j.ecoleng.2012.11.009, 2013.
Qi, T., Zhao, Y., Meng, X., Chen, G., and Dijkstra, T.: AI-Based Susceptibility Analysis of Shallow Landslides Induced by Heavy Rainfall in Tianshui, China, Remote Sens., 13, 1819, https://doi.org/10.3390/rs13091819, 2021.
Reichenbach, P., Rossi, M., Malamud, B. D., Mihir, M., and Guzzetti, F-Sci. Rev., 180, 60–91, https://doi.org/10.1016/j.earscirev.2018.03.001, 2018.
Rickli, C. and Graf, F.: Effects of forests on shallow landslides – case studies in Switzerland, Forest. Snow Landsc. Res., 44, 33–44, 2009.
Roback, K., Clark, M. K., West, A. J., Zekkos, D., Li, G., Gallen, S. F., Chamlagain, D., and Godt, J. W.: The size, distribution, and mobility of landslides caused by the 2015 Mw 7.8 Gorkha earthquake, Nepal, Geomorphology, 301, 121–138, https://doi.org/10.1016/j.geomorph.2017.01.030, 2018.
Roccati, A., Faccini, F., Luino, F., Turconi, L., and Guzzetti, F.: Rainfall events with shallow landslides in the Entella catchment, Liguria, northern Italy, Nat. Hazards Earth Syst. Sci., 18, 2367–2386, https://doi.org/10.5194/nhess-18-2367-2018, 2018.
Roering, J. J., Schmidt, K. M., Stock, J. D., Dietrich, W. E., and Montgomery, D. R.: Shallow landsliding, root reinforcement, and the spatial distribution of trees in the Oregon Coast Range, Can. Geotech. J., 40, 237–253, 2003.
Salciarini, D., Godt, J. W., Savage, W. Z., Conversini, P., Baum, R. L., and Michael, J. A.: Modeling regional initiation of rainfall-induced shallow landslides in the eastern Umbria region of central Italy, Landslides, 3, 181–194, https://doi.org/10.1007/s10346-006-0037-0, 2006.
Sassa, K.: Mechanism of flows in granular soils, in: vol. 1, ISRM International Symposium, 19–24 November 2000, Melbourne, Australia, 1671–1702, https://onepetro.org/ISRMIS/proceedings-abstract/IS00/All-IS00/ISRM-IS-2000-043/50818 (last access: 26 July 2024), 2000.
Sassa, K. and Wang, G. H.: Mechanism of landslide-triggered debris flows: Liquefaction phenomena due to the undrained loading of torrent deposits, in: Debris-flow hazards and related phenomena, Springer, Berlin, Heidelberg, https://doi.org/10.1007/3-540-27129-5_5, 2005.
Schilirò, L., Montrasio, L., and Mugnozza, G. S.: Prediction of shallow landslide occurrence: validation of a physically-based approach through a real case study, Sci. Total Environ., 569–570, 134–144, https://doi.org/10.1016/j.scitotenv.2016.06.124, 2016.
Segoni, S., Piciullo, L., and Gariano, S. L.: A review of the recent literature on rainfall thresholds for landslide occurrence, Landslides, 15, 1483–1501, https://doi.org/10.1007/s10346-018-0966-4, 2018.
Shieh, C. L., Chen, Y. S., Tsai, Y. J., and Wu, J. H.: Variability in rainfall threshold for debris flow after the Chi-Chi earthquake in central Taiwan, China, Int. J. Sediment Res., 24, 177–188, https://doi.org/10.1016/S1001-6279(09)60025-1, 2009.
Skempton, A. W.: Residual strength of clays in landslides, folded strata and the laboratory, Geotechnique, 35, 3–18, https://doi.org/10.1680/geot.1985.35.1.3, 1985.
Stähli, M., Sättele, M., Huggel, C., McArdell, B. W., Lehmann, P., Van Herwijnen, A., Berne, A., Schleiss, M., Ferrari, A., Kos, A., Or, D., and Springman, S. M.: Monitoring and prediction in early warning systems for rapid mass movements, Nat. Hazards Earth Syst. Sci., 15, 905–917, https://doi.org/10.5194/nhess-15-905-2015, 2015.
Stark, C. P. and Hovius, N.: The characterization of landslide size distributions, Geophys. Res. Lett., 28, 1091–1094, https://doi.org/10.1029/2000GL008527, 2001.
Sun, P., Li, R. J., Jiang, H., Igwe, O., and Shi, J. S.: Earthquake-triggered landslides by the 1718 Tongwei earthquake in Gansu Province, northwest China, Bull. Eng. Geol. Environ., 76, 1281–1295, https://doi.org/10.1007/s10064-016-0949-4, 2017.
Taylor, D. W.: Fundamentals of soil mechanics, J. Wiley, New York, https://babel.hathitrust.org/cgi/pt?id=mdp.39015052877415&seq=13 (last access: 29 July 2024), 1948.
Thomas, M. A., Mirus, B. B., and Collins, B. D.: Identifying physics-based thresholds for rainfall-induced landsliding, Geophys. Res. Lett., 45, 9651–9661, https://doi.org/10.1029/2018GL079662, 2018.
Tu, X. B., Kwong, A. K. L., Dai, F. C., Tham, L. G., and Min, H.: Field monitoring of rainfall infiltration in a loess slope and analysis of failure mechanism of rainfall-induced landslides, Eng. Geol., 105, 134–150, https://doi.org/10.1016/j.enggeo.2008.11.011, 2009.
Van den Eeckhaut, M., Poesen, J., Govers, G., Verstraeten, G., and Demoulin, A.: Characteristicsf the size distribution of recent and historical landslides in a populated hilly region, Earth Planet. Sc. Lett., 256, 588–603, https://doi.org/10.1016/j.epsl.2007.01.040, 2007.
von Ruette, J., Papritz, A., Lehmann, P., Rickli, C., and Or, D.: Spatial statistical modeling of shallow landslides–validating predictions for different landslide inventories and rainfall events, Geomorphology, 133, 11–22, https://doi.org/10.1016/j.geomorph.2011.06.010, 2011.
Waldron, L. J.: The shear resistance of root-permeated homogeneous and stratified soil, J. Soil Sci. Soc. Am., 41, 843–849, https://doi.org/10.2136/sssaj1977.03615995004100050005x, 1977.
Wang, G. and Sassa, K.: Factors affecting rainfall-induced flowslides in laboratory flume tests, Geotechnique, 51, 587–599, https://doi.org/10.1680/geot.51.7.587.51386, 2001.
Wang, G. H.: An experimental study on the mechanism of fluidized landslide: with particular reference to the effect of grain size and fine-particle content on the fluidization behavior of sands, PhD thesis, Kyoto University, Kyoto, https://core.ac.uk/download/pdf/39218538.pdf (last access: 29 July 2024), 2000.
Wang, G. L., Li, T. L., Xing, X. L., and Zou, Y.: Research on loess flow-slides induced by rainfall in July 2013 in Yan'an, NW China, Environ. Earth Sci., 73, 7933–7944, https://doi.org/10.1007/s12665-014-3951-9, 2015.
Wang, J. J., Liang, Y., Zhang, H. P., Wu, Y., and Lin, X.: A loess landslide induced by excavation and rainfall, Landslides, 11, 141–152, https://doi.org/10.1007/s10346-013-0418-0, 2014.
Wang, S., Zhang, K., van Beek, L. P., Tian, X., and Bogaard, T. A.: Physically-based landslide prediction over a large region: Scaling low-resolution hydrological model results for high-resolution slope stability assessment, Environ. Model. Softw., 124, 104607, https://doi.org/10.1016/j.envsoft.2019.104607, 2020.
Wieczorek, G. F.: Effect of rainfall intensity and duration on debris flows in central Santa Cruz Mountains, in: Debris flow and avalanches: process, recognition, and mitigation, Reviews in Engineering Geology, 7, edited by: Costa, J. E. and Wieczorek, G. F., Geological Society of America, 93–104, https://doi.org/10.1130/REG7-p93, 1987.
Xu, L., Dai, F. C., Tham, L. G., Tu, X. B., Min, H., Zhou, Y. F., and Xu, K.: Field testing of irrigation effects on the stability of a cliff edge in loess, North-west China, Eng. Geol., 120, 10–17, https://doi.org/10.1016/j.enggeo.2011.03.007, 2011.
Xu, L., Dai, F. C., Tu, X. B., Tham, L. G., and Zhou, Y. F.: Landslides in a loess platform, North-west China, Landslides, 11, 993–1005, https://doi.org/10.1007/s10346-013-0445-x, 2014.
Xu, Y. G., Luo, L., Guo, W. Z., Jin, Z., Tian, P., and Wang, W. L.: Revegetation Changes Main Erosion Type on the Gully–Slope on the Chinese Loess Plateau Under Extreme Rainfall: Reducing Gully Erosion and Promoting Shallow Landslides, Water Resour. Res., 60, e2023WR036307, https://doi.org/10.1029/2023WR036307, 2024.
Zhang, F. Y., Wang, G. H., Kamai, T., Chen, W. W., Zhang, D. X., and Yang, J.: Undrained shear behavior of saturated loess at different concentrations of sodium chloride solution, Engineering Geology, 155, 69–79, https://doi.org/10.1016/j.enggeo.2012.12.018, 2013.
Zhang, M. S. and Liu, J.: Controlling factors of loess landslides in western China, Environ. Earth Sci., 59, 1671–1680, https://doi.org/10.1007/s12665-009-0149-7, 2010.
Zhang, Z. L., Wang, T., and Wu, S. R.: Distribution and features of landslides in the tianshui basin, northwest China, J. Mount. Sci., 17, 686–708, https://doi.org/10.1007/s11629-019-5595-4, 2020.
Zhu, Y., Zhuang, J. Q., and Zhao, Y.: Evaluation of loess-filled slope failure triggered by groundwater rise using a flume test, Geomat. Nat. Hazards Risk, 13, 2471–2488, https://doi.org/10.1080/19475705.2022.2122592, 2022.
Zhuang, J., Peng, C., Wang, G., Chen, X., and Guo, X.: Rainfall thresholds for the occurrence of debris flows in the Jiangjia Gully, Yunnan Province, China, Eng. Geol., 195, 335–346, https://doi.org/10.1016/j.enggeo.2015.06.006, 2015.
Zhuang, J. Q., Peng, J. B., Wang, G. H., Javed, I., Wang, Y., and Li, W.: Prediction of rainfall-induced shallow landslides in the Loess Plateau, Yan'an, China, using the TRIGRS model, Earth Surf. Proc. Land., 42, 915–927, https://doi.org/10.1002/esp.4050, 2017.
Zhuang, J. Q., Peng, J. B., Wang, G. H., Javed, I., Wang, Y., and Li, W.: Distribution and characteristics of landslide in Loess Plateau A case study in Shaanxi province, Eng. Geol., 236, 89–96, https://doi.org/10.1016/j.enggeo.2017.03.001, 2018.
Zhuang, J. Q., Ma, P. H., Zhan, J. W., Zhu, Y., Kong, J. X., Zhu, X. H., Leng, Y. Q., and Peng, J. B.: Empirical relationships of the landslides in the Chinese Loess Plateau and affect factors analysis, Geomat. Nat. Hazards Risk, 13, 250–266, https://doi.org/10.1080/19475705.2021.2020174, 2022.
Zizioli, D., Meisina, C., Valentino, R., and Montrasio, L.: Comparison between different approaches to modelling shallow landslide susceptibility: a case history in Oltrepo Pavese, Northern Italy, Nat. Hazards Earth Syst. Sci., 13, 559–573, https://doi.org/10.5194/nhess-13-559-2013, 2013.
- Abstract
- Introduction
- Study area
- The 7.25 Tianshui sliding-flow landslide event characteristics
- Forecast model of shallow loess landslide development
- Discussion
- Conclusions
- Code and data availability
- Author contributions
- Competing interests
- Disclaimer
- Acknowledgements
- Financial support
- Review statement
- References
- Abstract
- Introduction
- Study area
- The 7.25 Tianshui sliding-flow landslide event characteristics
- Forecast model of shallow loess landslide development
- Discussion
- Conclusions
- Code and data availability
- Author contributions
- Competing interests
- Disclaimer
- Acknowledgements
- Financial support
- Review statement
- References