the Creative Commons Attribution 4.0 License.
the Creative Commons Attribution 4.0 License.
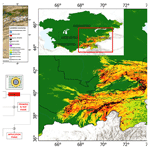
Comprehensive landslide susceptibility map of Central Asia
Ascanio Rosi
William Frodella
Nicola Nocentini
Francesco Caleca
Hans Balder Havenith
Alexander Strom
Mirzo Saidov
Gany Amirgalievich Bimurzaev
Veronica Tofani
Central Asia is an area characterized by complex tectonics and active deformation; the related seismic activity controls the earthquake hazard level that, due to the occurrence of secondary and tertiary effects, also has direct implications for the hazard related to mass movements such as landslides, which are responsible for an extensive number of casualties every year. Climatically, this region is characterized by strong rainfall gradient contrasts due to the diversity of climate and vegetation zones. The region is drained by large, partly snow- and glacier-fed rivers that cross or terminate in arid forelands; therefore, it is also affected by a significant river flood hazard, mainly in spring and summer seasons. The challenge posed by the combination of different hazards can only be tackled by considering a multi-hazard approach harmonized among the different countries, in agreement with the requirements of the Sendai Framework for Disaster Risk Reduction. This work was carried out within the framework of the Strengthening Financial Resilience and Accelerating Risk Reduction in Central Asia (SFRARR) project as part of a multi-hazard approach and is focused on the first landslide susceptibility analysis at a regional scale for Central Asia. To this aim the most detailed landslide inventories, covering both national and transboundary territories, were implemented in a random forest model, together with several independent variables. The proposed approach represents an innovation in terms of resolution (from 30 to 70 m) and extension of the analyzed area with respect to previous regional landslide susceptibility and hazard zonation models applied in Central Asia. The final aim was to provide a useful tool for land use planning and risk reduction strategies for landslide scientists, practitioners, and administrators.
- Article
(20159 KB) - Full-text XML
- Companion paper
- BibTeX
- EndNote
During the 2 decades spanning between 1988 and 2007, according to observed estimates, out of 177 reported disasters in Central Asia, 13 % were landslides, causing 700 deaths (Table 1), while in the same period economic losses have been as high as USD 150 million, including damage to infrastructures, settlings, and agricultural and pasture lands, as well as displacement of the population (GFDRR, 2009). More recent modeled estimates show that in the Central Asia states an annual average of 3 million persons are affected by earthquakes and floods, with an estimated annual average GDP of USD 9 billion (GFDRR, 2016).
Table 1Observed landslide hazard statistics (1988–2007). Source: Risk Assessment for Central Asia and Caucasus (UNISDR, 2009). NA – not available.
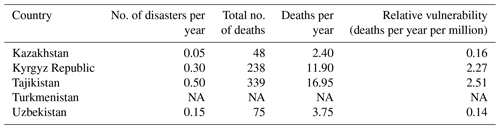
Due to their large size and impact, most of the occurring landslides have profound transboundary implications. Tajikistan and the Kyrgyz Republic are the countries that have been the most impacted by landslides: in Tajikistan around 50 000 landslides have been mapped, 1200 of which threatened settlements or facilities (Thurman, 2011), while the Kyrgyz Republic has been affected by 5000 landslides, of which 3500 at various levels of activity occurred in the southern part of the country (the Fergana Valley area) (Pusch, 2004; Li et al., 2021). Only in the Kyrgyz Republic, up to 2017, 784 landslides and 1658 mudflows (also including loess flows) and flash floods caused 352 victims (Kalmetieva et al., 2009; Havenith et al., 2015a, 2017). The Almaty Province in Kazakhstan, the cities of Tashkent and Samarkand and the Surkhandarya and Kashkadarya provinces of Uzbekistan, and the Ahal Province of Turkmenistan are also exposed to landslides (World Bank, 2006). Given the increased anthropogenic pressures and the impact of climate change, since the early 1990s several projects have tried to improve the knowledge on landslide hazards (Thurman, 2011) by providing landslide loss estimations, location, type, triggering and reactivation dates, and inventories and hazard and risk maps, as well as platforms to retrieve open disaster risk data and overviews on landslide risk reduction strategies. Among the regional studies on landslide hazards providing descriptions, statistics, and inventory maps, it is worth mentioning the following:
-
Disaster Risk Management and Climate Change Adaptation in Europe and Central Asia, developed by the World Bank – Global Facility for Disaster Reduction and Recovery (Pollner et al., 2010);
-
Disaster Risk Reduction: 20 Examples of Good Practice from Central Asia, developed by the European Union, International Strategy for Disaster Reduction (ISDR; European Commission, 2006);
-
the Science for Peace Project (983289): Prevention of Landslide Dam Disasters in the Tien Shan, Ladatsha, 2009–2012, NATO Emerging Security Challenges Division;
-
the Potsdam Research Cluster for Georisk Analysis, Environmental Change and Sustainability (PROGRESS), German Federal Ministry of Research and Technology (BMBF);
-
the Tian Shan-Pamir Monitoring Program (TIPTIMON), German Federal Ministry of Education and Research (BMBF); and
-
the M126 International Consortium on Landslides (IPL) project (funded by the International Consortium on Landslides): M2002111 detailed study of the internal structure of large rockslide dams in the Tien Shan, M2004126 compilation of landslide and rockslide inventory of the Tien Shan Mountain system.
Besides the creation of landslide inventories, a common approach to assess landslide hazard is the development of landslide susceptibility maps (LSMs), which depict the relative probability of occurrence of a given type of landslide in a given area, without considering the probability of occurrence in time (Brabb, 1984). In other words, LSMs identify those areas where landslides can occur, based on their geological, morphological, and climatic characteristics. These maps have been extensively used as useful tools for land planning (Cascini 2008; Frattini et al., 2010) and hazard assessment (Corominas et al., 2003). More recently, they have also been successfully integrated in quantitative risk assessment (Chen et al., 2016) and early warning systems (Segoni et al., 2018; Tiranti et al., 2019). LSMs have been produced by applying a wide range of mathematical techniques, from the most traditional statistic approaches like frequency ratio (Yilmaz, 2009), discriminant analysis (Carrara, 1983; Trigila et al., 2013), and logistic regression (Lee, 2005; Duman et al., 2006; Manzo et al., 2013) to more recent and more advanced techniques, like artificial neural networks (Tien Bui et al., 2016; Ermini et al., 2005), machine learning (Catani et al., 2013), and multi-criteria decision analysis (Akgun, 2012). Statistical probabilistic models for landslide susceptibility can overcome the data gaps and allow us to analyze very wide areas (from basin to national scales) by adopting a homogeneous methodology and a harmonized dataset (including global and local data sources). However, landslide hazard assessment is a complex process, since it needs accurate knowledge of the topic and appropriate input data (historical and regional inventories that mainly consist of large prehistoric events). In this work the landslide susceptibility analysis was carried out by means of the random forest (RF) machine learning algorithm, which is credited as one of the most advanced and reliable techniques in this field (Catani et al., 2013; Goetz et al., 2015). This work represents the first landslide susceptibility analysis at a regional scale for Central Asia and was carried out in the framework of the Strengthening Financial Resilience and Accelerating Risk Reduction in Central Asia (SFRARR) project as part of a multi-hazard approach (Bazzurro et al., 2023). The main challenge of this work was the creation of a unique LSM of the whole Central Asia, which involved the use of a wide range of variables to account for the features of each country and a high volume of input data and the development of new approaches to analyze these data and to take into account possible discrepancies and non-homogeneities. The proposed approach represents an innovation in terms of resolution and extension of the analyzed area with respect to previous regional landslide susceptibility and hazard zonation models applied in Central Asia (e.g., Nadim et al., 2006; Havenith et al., 2015b; Stanley and Kirschbaum, 2017; Pittore et al., 2018; World Bank, 2020).
Geographically, Central Asia is a vast and diverse region including high mountain chains, deserts, and steppes (Fig. 1). A large portion of the Central Asia countries, especially in the southern and eastern parts of the region, is occupied by the mountainous areas of the Dzungaria, Tien Shan, Pamirs, Kopet Dag, and a small part of western Altai, with peaks above 7000 m a.s.l (Strom, 2010). These intraplate mountain systems formed in the Cenozoic between the Tarim Basin and the Kazakh Shield, as a result of the India–Asia collision (Molnar and Tapponier, 1975; Abdrakhmatov et al., 1996, 2003; Zubovich et al., 2010; Ullah et al., 2015). This work is focused on the most inner part of Central Asia, represented by the territories of Turkmenistan, Kazakhstan, the Kyrgyz Republic, Uzbekistan, and Tajikistan. Active mountain building started in the Oligocene (Chedia and Lemzin, 1980) or even later (Abdrakhmatov et al., 1996), forming a complex system of basement folds disrupted by numerous thrusts and reverse faults with a significant amount of lateral offset (Delvaux et al., 2001). Several regional fault zones are aligned along large parts of the mountain belts, and others cross the orogen in a NW–SE direction, e.g., the Talas–Fergana fault, which forms a distinct boundary between the western and central Tien Shan (Trifonov et al., 1992) (Fig. 2).
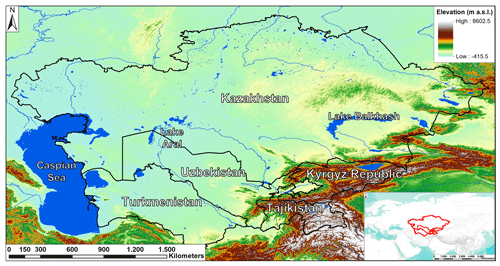
Figure 1Study area's geographical–geomorphological setting. Lakes' polygons are from Schiavina et al. (2022), while Multi-Error-Removed Improved-Terrain Digital Elevation Model (MERIT DEM; Yamazaki et al., 2017) is used as a topographic base.
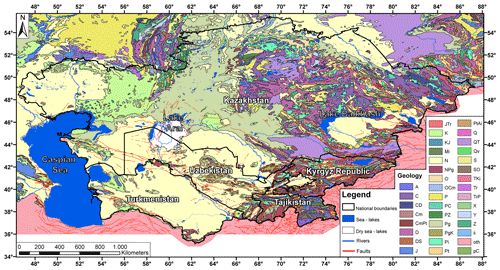
Figure 2Geological map of the study area. Geological formation data are from the United States Geological Survey (USGS) (see Persits et al., 1997, for the legend), including faults from the Active Faults of Eurasia Database (AFEAD) (Styron and Pagani, 2020).
Mountain ridges, formed mainly by Paleozoic crystalline rocks, are separated by wide lenticular or narrow, linear intermountain depressions, containing Neogene and Quaternary deposits, mainly sandstone, siltstone with gypsum interbeds, and conglomerates (Strom and Abdrakhmatov, 2017). Mesozoic and Paleogene deposits are typical of the foothill areas. Almost every ridge, especially in the Tien Shan, corresponds to a neotectonic anticline, and most of the main river valleys follow intermontane tectonic depressions, which are linked by narrow deep gorges up to 1–2 km deep (Strom and Abdrakhmatov, 2018). These mountain systems are the sources of most of Central Asia's rivers, which, being fed by glaciers, snowmelt water, and rain, have deeply incised valleys. Such extreme topography along with complex geological structure, active tectonics, and high seismicity determine important landslide predisposing factors, making landslides the third most prevalent natural hazard in Central Asia, following earthquakes and floods (CAC DRMI, 2009; Havenit et al., 2017).
2.1 Landslide types in Central Asia
According to the international Cruden and Varnes (1996) classification, landslide phenomena in Central Asia include rockslides and rock avalanches, rotational and translational slides, and mudflows and debris flows (often involving loess), which are triggered by natural events such as earthquakes, floods, rainfall, and snowmelt (Behling et al., 2014, 2016; Golovko et al., 2015; Havenith et al., 2006a, b, 2015a, b; Kalmetieva et al., 2009; Saponaro et al., 2015a, b; Strom and Abdrakhmatov, 2017, 2018). Glacial lake outburst flood phenomena, caused by the breach in natural glacial dams, often result in large-scale catastrophic mudflows and debris flows. In Central Asia, landslides more often occur in the loess zone of contact with other rocks, on clay interlayers of the Mesozoic and Cenozoic age, reaching a volume from tens of thousands up to 15–40 × 106 m3 (Juliev et al., 2017). Seismically triggered landslides are very common in tectonically active mountain regions, such as Tien Shan and Pamirs (Sternberg, 2006; Hong et al., 2007; Juliev et al., 2017). According to the literature background, most of the large mapped mass movements (especially those with a volume of more than 106 m3) were triggered generally by major (also prehistoric) earthquakes, possibly in combination with climatic factors, namely snowmelt and heavy rainfall (Havenith et al., 2003; Strom and Korup, 2006; Strom, 2010; Schlögel et al., 2011; Strom and Abdrakhmatov, 2017, 2018; Havenith et al., 2015a, 2016; Behling et al., 2014, 2016; Piroton et al., 2020). Furthermore, in the past few decades, the number and intensity of landslides have grown, owing to climate change and the increase in the anthropic pressure, due to several factors such as uncontrolled land and water use, the rising of the water tables (often induced by the increase in irrigation; Ishihara et al., 1990), mining, and excavation activities (Pollner et al., 2010; Thurman, 2011).
2.2 Large rockslides and natural dams
Numerous rockslides have occurred in the mountains, producing hazardous natural phenomena such as long runout rock avalanches (Fig. 3) and dammed lakes, more than 100 of which still store water (Strom, 2010). These mainly involve the Paleozoic magmatic and metamorphic crystalline bedrock but also the sandstone and limestone formations. Although according to Strom (2010), many of the existing dammed lakes should be considered stable, catastrophic outburst floods that occurred in the 20th century emphasize the high potential hazard of landslide natural blockages. Havenith et al. (2015a) report a catalogue of large to giant landslides (having volumes exceeding > 107 m3) in the Tien Shan area, showing information such as location, time of occurrence, volume, and thickness. Regarding the volumes of these rockslides, these range from 50 × 103 m3 to 10 km3 (Strom and Korup, 2006; Strom and Abdrakhmatov, 2018). Many of these phenomena, though not all, were triggered by earthquakes with M > 6 and have dammed a river valley (some of the dams have been naturally or artificially breached).
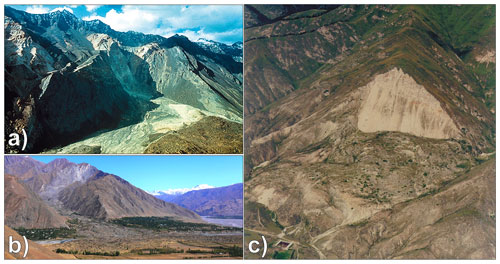
Figure 3Examples of large rockslide features in Central Asia. Helicopter view of the Usoi landslide scarp, triggered by the 1911 earthquake, Tajikistan (a) (after Strom, 2010); the Khait rock avalanche (b) (after Havenith et al., 2015a); and helicopter view of the Ananevo landslides (c) (after Havenith et al., 2015a).
2.3 Landslide in soft rocks and loose deposits
Rotational landslides mostly occur in loose unconsolidated Quaternary deposits and in soft and semi-hard rock layers in Mesozoic–Cenozoic sediments, represented mainly by layers of clays, claystones, siltstones, sandstones, marls, limestone, gypsum, and conglomerates, with intercalated clays (Roessner et al., 2004; Kalmetieva et al., 2009) (Fig. 4). These phenomena can create river dams, but they rarely are long-living dams, since usually they are small, and their bodies are eroded quickly even if they block a river channel (Strom and Korup, 2006).
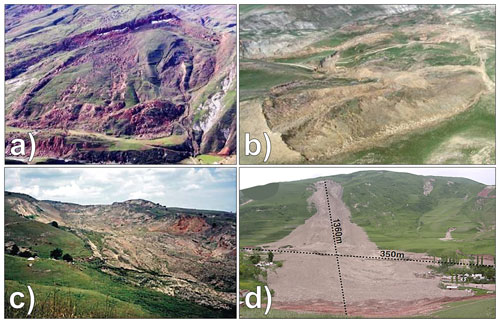
Figure 4Examples of landslides in soft rocks and loose deposits. Picture of the Kamar landslide (a) and the Beshbulak landslide (b) (after Niyazov and Nurtaev, 2013). Examples of loess slides and mixed loess–soft landslides in the NE Fergana Valley: Kochkor-Ata landslide failure in spring 1994 (c) (after Roessner et al., 2005) and field photo of the Kainama landslide (d) (after Behling et al., 2016).
The loess landslides occur quite regularly (on a yearly basis) in the regions, presenting an almost continuous and locally very thick (> 20 m) cover of this material, generally at mid-mountain altitude (900–2300 m) and mainly along the border of the Fergana Basin (the Kyrgyz Republic, Uzbekistan, and Tajikistan) and on the southern border of the Tien Shan in Tajikistan (Fig. 4).
Loess flow landslides and debris flows, involving the eluvial slope cover, represent a relevant hazardous phenomenon in the mountainous regions of Kazakhstan, in the area of Almaty, near the southern border with the Kyrgyz Republic, in the Altai area (Medeu and Blagovechshenskiy, 2016), around the Fergana Basin, all along the border between Tajikistan and the Kyrgyz Republic, and around the Tajik Depression. Landslides occurring in Quaternary loess units of up to 50 m thick are characterized by very rapid avalanche-like mass movements, which can reach several meters per second (often representing a combination of rotational slides and dry flows, resulting in long runout zones; World Bank, 2008). Typically, pure loess landslides have a volume of hundreds up to 1 million cubic meters and appear as clusters (Roessner et al., 2005). From recent history it appears that pure (or quasi-pure) loess slides and flows are particularly dangerous because of their high velocity and long runout which, in turn, can generate a great destructive power and more severe disasters than other types of mass movements of similar size (Havenith et al., 2015a; Behling et al., 2014, 2016). If failure also affects underlying materials (mostly Mesozoic and Cenozoic soft rocks), the volume of these mixed slides can exceed 10 × 106 m3.
These kinds of landslides are particularly deadly and can be triggered by a combination of long-term slope destabilization factors (e.g., rainfall and snowmelt) and short-term triggers (e.g., seismic shocks). Even though earthquake-triggered loess slides and flows are far less frequent than rainfall-triggered ones, they have caused much larger disasters in recent history, such as those triggered, respectively, by the July 1949 Khait and the January 1989 Gissar earthquakes. The number of active debris flow basins in Kazakhstan is over 300 with registered cases of more than 600 debris flows of different geneses (80 % of which are represented by heavy-rainfall-triggered debris flows, while the glacial debris flows make up about 15 % of the total).
3.1 Landslide databases
To implement the adopted susceptibility models, the largest, most accurate, and most updated landslide inventories were used (Fig. 5). These were compiled by several authors by means of decades of field surveys, remote sensing, and geophysical analysis in the study area.
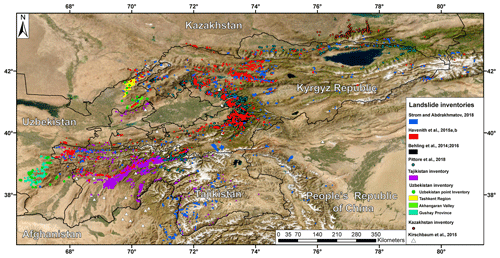
Figure 5Map of the adopted landslide inventory. Basemap source: Esri, Maxar, Earthstar Geographics, and the GIS User Community.
Hereafter we report their description in detail (Table 2):
-
The Tien Shan landslide inventory (Havenith et al., 2015a) represents the largest inventory in the study area. Compiled by means of field surveys, remote sensing data interpretation, and geophysical surveys, it comprises the rockslides of the previous inventory together with other smaller landslides in soft sediments (Havenith et al., 2006a; Schlögel et al., 2011) for a total of 3462 landslide polygons, also including information on landslide length and area.
-
The rockslides and rock avalanches of Central Asia (Strom and Abdrakhmatov, 2018) is a large inventory including 860 polygons of large-scale (≥ 1 Mm3) rockslides and rock avalanches, covering Central Asian countries (except for Turkmenistan and Altai) plus the Chinese Tien Shan and Pamirs and the Afghan Badakhshan. Compiled through decades of fieldwork and analysis of aerial and satellite imaging, it also comprises information on landslide morphometric parameters (runout, area) and 126 polygons on possible landslide bodies, dammed lakes, and head scarps. Quantitative characteristics (area, volume, runout, etc.) for about 600 cases are provided as well.
-
The multi-temporal landslide inventory for a study area in southern Kyrgyz Republic derived from RapidEye satellite time series data (2009–2013) (Behling et al., 2014, 2016; Behling and Roessner, 2020) is a semi-automated spatiotemporal landslide inventory for the period from 1986 to 2013, covering 2500 km2 in the Fergana Valley rim in the southern Kyrgyz Republic. This inventory includes 2052 landslide polygons mapped from multi-sensor optical satellite time series data, together with information on spatiotemporal landslide activity patterns (area and year of trigger).
-
The EMCA landslide catalog Central Asia (Pittore et al., 2018), which includes 3129 points, mostly covers the western and northern Kyrgyz Republic as well as Tajikistan's Region of Republican Subordination. The catalogue is a summary (point locations) of the documented landslides between 1954 and 2009 (Kalmetieva et al., 2009), which are collected by the Central Asian Institute for Applied Geosciences through geological surveys (field campaigns) on single sites close to urban areas.
-
The Tajikistan landslide database is provided by the Institute of Water Problems, Hydropower, Engineering and Ecology of Tajikistan (IWPHE), which includes 2822 landslide polygons and 114 landslide-prone areas (with information on length and area).
-
The Uzbekistan landslide inventory is provided by the Institute of Seismology of the Academy of Science of Uzbekistan (ISASUZ) and the State Monitoring Service of the Republic of Uzbekistan for hazardous geological processes, which covers the Tashkent–Kashkadarya region and the Akharangan Valley. It comprises a 49-point inventory (including location, type, volume, length, and date of triggering) and a polygon inventory digitized for this project from the maps in Juliev et al. (2017) (including a total 324 landslide polygons).
-
The Kazakhstan landslide inventory is provided by the Institute of Seismology Limited Lability Partnership (LLP) of Kazakhstan, covering mainly the Tien Shan area at the border with the Kyrgyz Republic and a small part of the western Altai, including 254 point shapefiles with information on type, area and volume, and triggering date.
-
Part of the Global Landslide Catalogue (GLC) (Kirschbaum et al., 2015), which covers the Kyrgyz Republic and Tajikistan, includes 15 landslide points with a description on landslide size and type, triggering date, and triggering and factor. The GLC has been compiled since 2007 at NASA's Goddard Space Flight Center and considers all types of mass movements triggered by rainfall, which have been reported in the media, disaster databases, scientific reports, or other sources.
3.2 Random forest (RF) model
To generate the landslide susceptibility maps in this work, the random forest model (RF) was used. RF is a nonparametric and multivariate machine learning technique, which was proposed by Breiman (2001) and first used in landslide susceptibility analysis by Brenning (2005). Since then, it has rapidly gained widespread consolidation through much research and case studies, as it is considered a relatively powerful approach in classification, regression, and unsupervised learning (Lagomarsino et al., 2017). Among the advantages of using the RF algorithm, there is the possibility of using numerical and categorical variables at the same time, without assumption about the statistical distribution of their values. Furthermore, RF is acknowledged to be capable of implicitly handling the multicollinearity of variables, identifying the uninfluential (or the detrimental) ones (Breiman, 2001; Brenning, 2005). RF also automatically performs a validation by building a receiver operating characteristic curve (ROC curve) and calculates the relative area under the curve (AUC). AUC is widely used as a quantitative indicator for the predictive effectiveness of susceptibility models: it can range from 0.5 (completely random predictions) to 1.0. This model, by means of the bootstrapping technique, also calculates the out-of-bag error (OOBE) for each variable. This parameter measures the relative error that would be committed if a given variable is excluded from the RF classifier. OOBE can be used to assess the relative importance of each independent variable, thus representing a powerful tool to interpret the results and to rank the variables according to their importance (Catani et al., 2013). RF contains a series of binary tree predictors, which are generated by using a random selection of the input data (the independent variables which in LSM studies are a set of physical parameters representing the predisposing factors), in order to split each binary node (yes/no) and to perform a classification of the target-dependent variable (in LSM studies, the presence or absence of landslides). Some of the observations are used for internal testing to evaluate the predictive capability of each predictor tree. This information is used to iterate the procedure hundreds of times by growing other random trees (hence the name random forest) and to iteratively adjust the prediction effectiveness. Once the best predictor tree is identified, it is applied to the whole study area to define the LSM. Another important key point of RF is that it has a great predictive performance and runs fast by summarizing many classification trees, and this is particularly useful when dealing with large numbers of data.
3.3 Selection of independent variables
As independent variables, 20 “basic parameters” were selected in all five countries, based on the available data and according to the ones most widely adopted in the literature (Catani et al., 2013; Reichenbach et al., 2018). Many of these are DEM-derived products (e.g., elevation, aspect, slope, slope curvature, flow accumulation, stream power index, topographic wetness index, topographic position index). It must be considered that the resolution of the susceptibility maps depends on the resolution of the input data. Therefore, it was decided to use pixels corresponding to the MERIT DEM (Yamazaki et al., 2017) resolution (about 90 m at the Equator and 70 m at 40∘ latitude). In addition, the DEM itself was used as a reference map so that the other parameters were processed to have a perfect overlapping. Therefore, the resulting landslide susceptibility maps will also be perfectly overlapping to it. The variables such as lithology and soil type were rasterized with this resolution by choosing the most frequent value in a reference window. The 20 basic parameters used are listed below, including a brief description:
-
MERIT DEM and DEM-derived products. This includes aspect, slope gradient, total curvature, profile curvature, planar curvature, flow accumulation, topographic wetness index (TWI), stream power index (SPI), and topographic position index (TPI).
-
Lithology. This is derived from the geological map of the former Soviet Union made by the USGS (Persits et al., 1997).
-
Soil-type map. This is taken from the Digital Soil Map of the World (DSMW) database (Copernicus land use; https://land.copernicus.eu/, last access: 27 July 2022).
-
Distance from faults. It is the minimum distance, in meters, between each landslide and the nearest fault. The fault database is derived from the AFEAD catalogue (Styron and Pagani, 2020) and was modified after Poggi et al. (2023a).
-
Distance from roads. It is the minimum distance, in meters, between each landslide and the nearest road. The road database is derived from Scaini et al. (2023).
-
Distance from rivers. It is the minimum distance, in meters, between each landslide and the nearest river. The river network database is derived from Coccia et al. (2023).
-
Distance from hypocenters. It is the minimum distance, in meters, between each landslide and the nearest earthquake hypocenter with a magnitude greater than 6.5 (following the methodology adopted by Havenith et al., 2015a). The hypocenter database was provided by Poggi et al. (2023a).
-
Peak ground acceleration (PGA). Four kinds of PGA maps according to different return times (475 and 1000 years) and different materials (soil layers and bedrock) to which it refers were created (Poggi et al., 2023b).
In addition to these basic parameters, in this study it was decided to use five parameters related to the propensity of the territory to be affected by precipitation (Fig. 6). These parameters were obtained from the ERA5 database (https://www.ecmwf.int/en/forecasts/datasets/reanalysis-datasets/era5, last access: 27 July 2022).
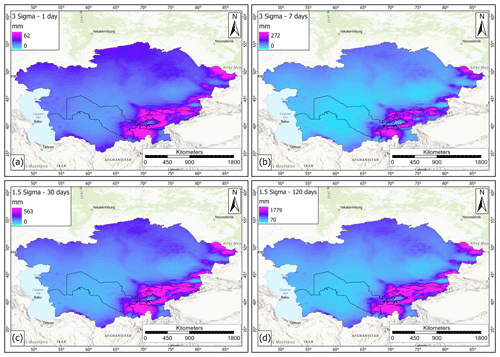
Figure 6Rainfall maps from the ERA5 database (https://www.ecmwf.int/en/forecasts/datasets/reanalysis-datasets/era5). (a) Rainfall amounts corresponding to 3 standard deviations for 1 d rainfall, (b) rainfall amounts corresponding to 3 standard deviations for 7 d rainfall, (c) rainfall amounts corresponding to 1.5 standard deviations for 30 d rainfall, and (d) rainfall amounts corresponding to 1.5 standard deviations for 120 d rainfall. Basemap source: Esri, USGS, NOAA.
Rainfall distribution maps have been used to differentiate the study area based on the rain rate and the distribution of anomalous rainfall events, since more rainy areas are more likely to experience landslide events than those that are less rainy. At the same time, a rain event with a low probability of occurrence can likely trigger a landslide even in less rainy areas, so the probability of some extreme rainfall events was calculated as well. These data span from 1981 to 2020 and have a 1 h temporal resolution (summarized to daily resolution for this work) and a spatial resolution of 0.25∘. The first parameter is the mean annual precipitation (MAP) map, where, for each pixel, the mean annual precipitation was calculated (Fig. 6). Other maps (named Sigma maps) have been calculated by the spatialization of the approach described in Martelloni et al. (2012). In detail, for each rain gauge (represented by the pixels of ERA5 maps in this work) the rain values corresponding to a given standard deviation for several cumulative intervals are defined (e.g., the rain values corresponding to 2 standard deviations of the distribution of 3 d cumulative rainfall):
-
Sigma 3–1 d. These rainfall values correspond to 3 standard deviations of daily cumulative rainfall. They range from 0 to 62.2 mm (Fig. 6a).
-
Sigma 3–7 d. These rainfall values correspond to 3 standard deviations of the 7 d cumulative rainfall. They range from 0 to 271.9 mm (Fig. 6b).
-
Sigma 1.5–30 d. These rainfall values correspond to 1.5 standard deviations of the 30 d cumulative rainfall. They range from 0 to 563.1 mm (Fig. 6c).
-
Sigma 1.5–120 d. These rainfall values correspond to 1.5 standard deviations of the 120 d cumulative rainfall. They range from 70 to 1778.8 mm (Fig. 6d).
The sigma parameters represent the probability of having a given rainfall amount over a defined time interval. In this work, four intervals were selected (1, 7, 30, and 120 d) to consider both short and long rain events that can lead to the triggering of surficial or deep-seated landslides, respectively. For 1 and 7 d the maps of the rainfall values corresponding to 3 standard deviations over the mean rainfall were selected to verify if short and very intense rainfall (with a very low probability of occurrence) could influence the slope stability in the study area. Regarding the 30 and 120 d interval, rainfall values corresponding to 1.5 standard deviation were calculated in order to assess the influence of longer and less intense rainfall on slope stability.
3.4 Model optimization
3.4.1 Independent variable optimization
The LSM was defined using the whole study area, instead of processing each country individually. This choice allowed us to overcome the boundary effects associated with the use of independent countries. In addition, a buffer of 10 km was considered around the whole area to avoid deformation due to boundary effects. These choices were helpful in reducing distortions and improving the quality of the results but also led to a huge number of data to be processed. Since the same resolution of the DEM was used for susceptibility assessment, the whole area was divided into about 1.07 × 109 cells, and for each cell 26 condition factors and 1 dependent variable were defined; this led to about 2.89 × 1010 data to be processed. In order to reduce the processing time and avoid computational problems due to the huge number of data and the width of the study area, large flat areas were filtered and not considered in the modeling process, since landslides generally take place along slopes (some exceptions to this statement in the area are represented by landslide around the flat Caspian Sea area; Pánek et al., 2016). For Turkmenistan no landslide database was available, so it was decided to train and test the model only with the other four countries to obtain the best predictor model for the available data. The trained model was then applied to the whole study area, including Turkmenistan, to define the LSM.
3.4.2 Landslide inventory harmonization
Regarding the dependent variables, the landslide inventory was created by merging the data described in Sect. 3.1. As a result, this landslide dataset was quite heterogenous; hence an initial control and homogenization phase was necessary. In this framework the landslide data were checked to verify the presence of overlapping polygons or topological errors, which were removed. Since some landslide inventories were composed solely of points, these were mapped only as “landslide points”; a 100 m buffer was created around them in order to include them in the model. However, when the points refer to large landslides, which are frequent in the study area, it is possible that a part of the body of these landslides is still outside the perimeter achieved with the buffer. To avoid classifying these areas as non-landslide points, it was decided to create an additional buffer of 1 km around points, used as a mask where the non-landslide points were not to be selected. This process reduced the probability of pixel misclassification (e.g., landslide points regarded as non-landslide points) during the training of the model. All the points inside the 1 km buffer were only considered during the model application. Some landslide-prone areas were also present in the input inventories; since these were not real landslides but landslide-prone zones, these areas were not used to train the susceptibility model but were used in the validation of the results. This optimization procedure, schematized in Fig. 7, allowed us to define an input dataset of 1.08 × 108 points (along with 27 variables for each point) to be used to define the susceptibility model.
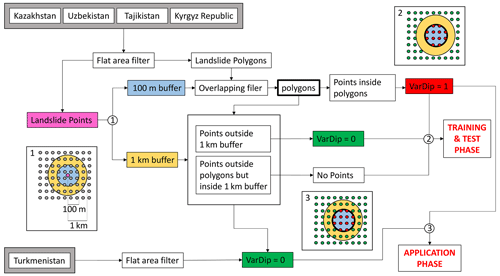
Figure 7Workflow describing the landslide database harmonization procedure. In gray: sample points for RF, pink: landslide points, blue: 100 m buffer, yellow: 1 km buffer, red: sample points identified as VarDip = 1, green: sample points identified as VarDip = 0, bold black line: landslide body, and VarDip = dependent variable.
3.4.3 Tree number optimization
A further optimization of the model was performed by the evaluation of the out-of-bag classification error, i.e., the variation in the misclassification probability with the number of grown classification trees. The classification error initially reduces with the increasing of classification trees, then it turns to be stable, so the definition of the optimal number of classification trees is required to avoid the use of an overgrown forest with an excessive number of trees (hence with high computational load and time) and without any advantage for the model (Fig. 8).
3.5 Model training
Once all the data were prepared and organized, the algorithm to create the landslide susceptibility maps was developed. A crucial step in LSM analysis is the approach used to sample the variables to train and validate the model. As in any other statistical procedure, the size of the dataset influences the results; therefore the higher the number of samples to perform the statistical calibration and validation of the model, the more reliable the obtained results are. To avoid a generalized hazard overestimation, Catani et al. (2013) demonstrated that a random sampling improves the predictive capability of the map, and the susceptibility model should also be trained and validated with respect to information about non-landslide locations. Regarding the proportion between the calibration and validation dataset samples, it is common practice to split them according to a 70 30 ratio. Therefore, using Esri ArcGIS Pro software, all the variables were sampled pixel by pixel, after which, with the MATLAB software, from the total of the sampled points, all the points within a landslide and a same amount of randomly chosen non-landslide points were extracted. This input dataset was divided into two parts: 70 % of the data (calibration dataset) were used for the training phase and the remaining 30 % (validation dataset) for the testing phase. The selection and division were randomly repeated five times in order to assess the stability of the model to the variation in the training and testing datasets, hence, to verify the absence of overfitting issues. Each one of these datasets was created to be equally composed by pixels within a known landslide and pixels outside a landslide.
All these data were then used to train and test the algorithm created to predict the landslide susceptibility of the whole area. The best predictor model identified in the training phases was then applied to all the available data (also for Turkmenistan and for the 1 km buffer area around the point-object landslides) for the development of the susceptibility map on the whole Central Asia area. The results obtained from the application of the aforementioned methodology are the susceptibility map, the receiver operating characteristic (ROC) curves with their area under the curve (AUC) values, and the histogram of the importance of variables. ROC and AUC are used to verify the quality of the landslide susceptibility model, both by a graphical and analytical approach. Due to the high volume, variety, values, and heterogeneity of the data a specific algorithm was created for this work, which was set to be able to perform several activities:
-
reading and properly formatting the input data and then dividing them between independent and dependent variables;
-
automatically and randomly selecting locations associated with landslides or outside landslides to create the training and test datasets;
-
identifying the best predictor and evaluating its performances by the calculation of the misclassification probability of the values calculated by the model;
-
evaluating the overall performances of the model by means of ROC and AUC;
-
identifying the importance of the parameters in landslide susceptibility; and
-
applying the model to the whole study area, calculating the probability of classification (landslide or non-landslide) of each pixel, and extracting of the final map in raster format.
The algorithm was set to work in classification mode; e.g., for each pixel a value (1 or 0) is assigned to identify the presence or absence of a landslide (dependent variable), along with the values of the independent variables. Using these data, the RF model identifies the best association of independent variables linked to the presence or absence of landslides (landslide susceptibility prediction model). The prediction model is then applied to all the pixels of the investigated area, and the probability of each pixel to be classified as a landslide (or non-landslide) pixel is evaluated. These probability values are those used to create the landslide susceptibility maps. It must be noted that the landslide inventories adopted to train the RF rarely reported the type of landslide, so the LSMs must be considered not related to a specific type of landslide.
3.6 Model validation
To verify the quality of the susceptibility models, besides the AUC value previously reported, a confusion matrix for the four countries where the model was trained was created (Fig. 9). In each matrix the predicted landslide classes are compared with the ground truth to verify the presence of significant misclassification error. In all the matrices the value 1 represents the presence of landslides, and the value 0 represents the absence of landslides. The numbers in each cell represent the number of pixels classified in that combination of 0 and 1, according to this scheme (the first number represents the predicted class and the second number the ground truth):
-
0–0 (true negative). Pixels outside any landslides are correctly identified as no-landslide pixels by the model.
-
1–1 (true positive). Pixels inside a landslide are correctly identified as landslide pixels by the model.
-
0–1 (false negative). Pixels inside a landslide are wrongly identified as no-landslide pixels by the model.
-
1–0 (false positive): Pixels outside any landslides are wrongly identified as landslide pixels by the model.
The 0–0 and 1–1 combinations represent well-classified pixels (blue cells in Fig. 8), while 0–1 and 1–0 represent misclassification error (light red cells in Fig. 8). Since this matrix needs some ground-truth parameters (true classes), it can be applied only where the presence or absence of landslides is known. For this reason, in this work, this matrix was calculated considering only the test dataset. A further control of the results was made using the areas prone to landslides identified in the landslide inventories used.
4.1 Susceptibility map
In the map presented in the following Figs. 10 and 11, the susceptibility values, ranging from 0 to 1, were classified into five classes (Table 3). Here the corresponding extension and percentage of the study area are also reported, showing that the most frequent susceptibility class for the whole study area is the null class (= 87.8 %; landslides generally do not occur in flat areas), followed by low and medium classes. Only the 4 % of the Central Asian territory is represented by areas with high and very high landslide susceptibility (Table 3). In Fig. 12, the susceptibility maps of five selected areas are displayed to better show the details of the susceptibility assessment and its comparison with mapped landslides in different geomorphological contexts of the study area. From these details it is possible to ascertain the high usefulness of the landslide susceptibility map realized by applying the random forest model, which, mainly based on the hydro-geomorphological properties, can establish the degree of susceptibility even in areas where there is no awareness of the predisposition to instability due to the absence of reported landslides.
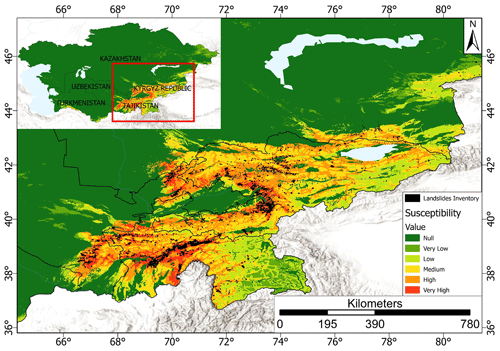
Figure 11Details of the landslide susceptibility map with the overlapping landslide polygons (in black). In the top left is the detailed area with respect to the Central Asian territory. Basemap source: Esri, USGS, NOAA.
Table 3Landslide susceptibility class intervals, corresponding area, and percentage with respect to CA.
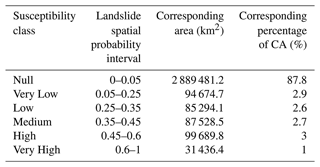
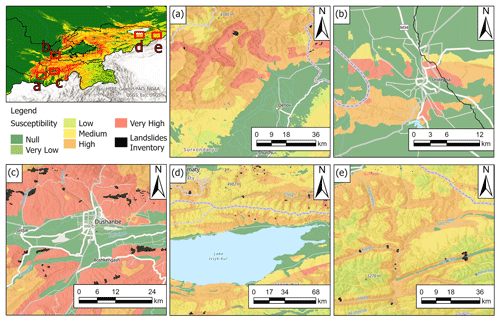
Figure 12Details of the landslide susceptibility map. (a) The city of Denau, Uzbekistan; (b) the city of Istaravshan, Tajikistan; (c) the city of Dushanbe, the Kyrgyz Republic; (d) Lake Issyk-Kul, the Kyrgyz Republic; and (e) the eastern area of the Kyrgyz Republic. Black polygons represent landslide areas from the adopted landslide inventories. Basemap source: Esri, USGS, NOAA.
In particular, the following can be observed:
-
Figure 12a shows the area north of the city of Denau, in the southeast of Uzbekistan, which is characterized by a high susceptibility, despite the almost total absence of mapped landslides.
-
Figure 12b shows the city of Istaravshan in detail, in the northwest of Tajikistan, where there are not any known landslides, but a high susceptibility has been obtained in the surrounding mountain relief.
-
In Fig. 12c there is a close-up of the city of Dushanbe, the capital of Tajikistan, where close to roads and inhabited centers a high landslide susceptibility is observed.
-
The shores of Lake Issyk-Kul in the Kyrgyz Republic, shown in Fig. 12d, are generally flat areas, with a low or null landslide susceptibility apart from in the central zone.
-
Finally, Fig. 12e shows the western area of the Kyrgyz Republic in detail, where a high landslide susceptibility is observed along the slopes adjacent to the river network.
4.2 The Fergana Valley mountainous rim
The Fergana Valley spreads across eastern Uzbekistan, the southern Kyrgyz Republic, and northern Tajikistan (Fig. 13). It is one of the largest intermountain depressions in Central Asia, located between the mountain systems of the Chatkal–Kuraminsk ranges in the north and Turkestan–Alai in the south. The two main rivers, the Naryn and the Kara Darya, flow into the valley and unite, forming the Syr Darya. In this area landslides represent one of the major natural hazards due to their frequent (seasonal) occurrence across large areas: in fact, they are particularly concentrated in a range of altitudes between 700 and 2000 m along the topographically rising rim below its transition into higher mountainous terrain (Roessner et al., 2000, 2004, 2005; Behling et al., 2014, 2016). This region is quite densely populated, and landslides lead almost every year to damage of settlements and infrastructure and loss of human life (Schloegel et al., 2011; Piroton et al., 2020). In this area landslide activity is caused by complex interactions between tectonic, geological, geomorphological, and hydrometeorological factors (Havenith et al., 2015a, b). In the Fergana Valley rim, mass movements are often characterized by deep and steep scarps, and they mobilize weakly consolidated sediments of the Tertiary or Quaternary age, including loess deposits (Piroton et al., 2020). These kinds of landslides are particularly deadly and can be triggered by a combination of long-term slope destabilization factors (e.g., rainfall and snowmelt) and short-term triggers (Danneels et al., 2008). Slope landslide susceptibility was analyzed in this area using the previously mentioned methodologies. Figure 13 shows the particulars about the landslide susceptibility map obtained for the Fergana Valley, while Fig. 14 reports the histogram of the area occupied by each susceptibility class.
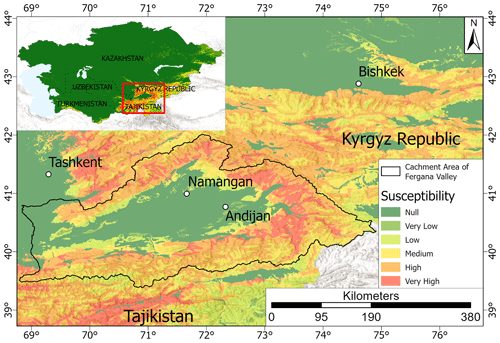
Figure 13Details of the landslide susceptibility map obtained for the Fergana Valley. Basemap source: Esri, USGS, NOAA.
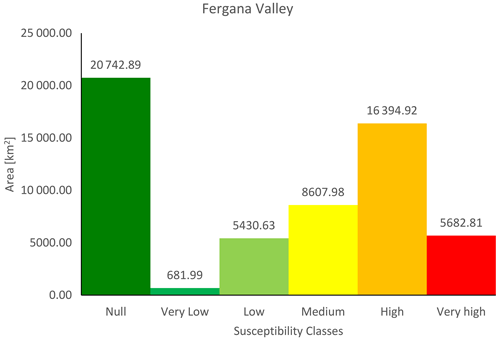
Figure 14Frequency histogram of susceptibility classes obtained for the Fergana Valley mountainous rim. On each bar the corresponding area in km2 is reported.
It can be observed that the most frequent susceptibility class in the Fergana Valley area is the null class, which covers an area of about 20 743 km2, i.e., 36 % of the valley. The very low and low classes occupy, respectively, an area of 681 km2 (1.2 %) and 5431 km2 (9.4 %). The medium class instead extends for about 8608 km2, namely 15 % of the total. The high class instead extends for about 16 395 km2, i.e., 28.5 % of the total, and finally, the remaining 9.9 % of the national territory, i.e., about 5683 km2, is classified in the very high class.
4.3 Trained model performances and conditioning factor relevance
RF was initially trained setting 1000 trees to be grown. After the first run, the analysis of the out-of-bag error revealed that the misclassification probability reduced significantly with a forest of 150 trees and then reduced slightly up to 500 trees, then it turned to be stable, so the optimal number of trees was set equal to 500 and used for all the simulations. As described above, the model was run five times to verify its stability, and the AUC values ranged from 0.93103 to 0.93144 (Fig. 15), with a mean value of 0.93122 and a standard deviation of 0.00015. The low variance of the AUC values confirmed the stability of the model and its applicability to the whole area. As we can see in the ranking of the susceptibility parameters, reported in Fig. 16, soil type, lithology, elevation, the distance from roads, and hypocenters play a crucial role in landslide susceptibility, since they are the five most influencing factors (for the four countries where the model was trained). Rainfall parameters are also important in the obtained landslide susceptibility, particularly in the 1 d rainfall value that shows the highest importance among the rainfall parameters. Also, the PGA maps are a relevant factor, while TWI and slope curvature are less important parameters. The average AUC value of the models is 0.93122, indicating their very good quality. Such high AUC values can indicate the presence of overfitting issues, but this hypothesis can be discarded, since the random variable resulted without any importance in landslide susceptibility (negative OOBE value).
The main issue affecting the utilized random forest model is the need for an adequate training dataset to properly calibrate the predictor model. The first step of the work has been the homogenization of the landslide data; the landslide inventory that was used was created starting from different sources, hence, with quite non-homogeneous data (e.g., in some cases the whole landslide perimeter was available, while in other cases only a point representing the source area of each landslide was provided, without info about the landslide dimension or propagation distance; more in general there were few or no data about the landslide type or triggering causes). The lack of some data about the landslides, or the partial or complete lack of landslides as in Kazakhstan and Turkmenistan, could lead to the underestimation of the real landslide hazard of the studied countries, since some points could have been wrongly classified (e.g., they have been regarded as no-landslide areas, but it was possible that a not-reported landslide was present). Furthermore, not all the adopted landslide inventories included information regarding the landslide types, leading to the creation of a general landslide susceptibility map, where all the types of landslides are considered. The created maps have been validated only using the available landslide dataset, providing good results and highlighting the good prediction capability of the model. In any case, an in situ validation in some sample areas can help to verify the quality of the results. As previously stated, for Turkmenistan there was no landslide inventory available to train the RF model; therefore the corresponding LSM was obtained applying the model trained for the other four countries. The lack of landslide data did not allow any validation of the result or estimation of the quality of the susceptibility map of Turkmenistan. Furthermore, applying the model developed for the other countries, the same importance of the conditioning factors (e.g., the independent variables) was assumed. For these reasons, the landslide susceptibility map for Turkmenistan is more uncertain than those evaluated for the other four countries. Among the conditioning factors used, soil type, distance from roads, and distance from hypocenters resulted in being the most influencing factors in slope stability, while planar curvature resulted in a high variability of its importance. These parameters have hence been more deeply analyzed to understand how they influence landslide susceptibility. According to the partial-dependency plots (Fig. 17), which show how the values of each conditioning factor influence the landslide susceptibility, the soil types more related to landslides are Lithosols and Cambisols, low-thickness soils limited in depth by a continuous coherent and hard rock layer, located in steeply slopes, with more than 30 % of slope gradient. While the classes that have the lowest importance score are Fluvisols (young soils in alluvial deposits), Xerosols (mainly arid clay), and Chernozems (soils rich in organic matter), each is situated in flat to hilly areas, with less than 30 % of slope gradient. Distance from roads, as expected, is important for low values, since the importance score is maximum for distances close to 0, and it decreases exponentially with the increasing of the distance. A similar behavior can be noted with the distance from hypocenters, meaning that areas close to hypocenters (within a radius of about 25 km) can more easily experience landslide phenomena in the case of future earthquakes. The partial-dependency plot of planar curvature showed that the variability highlighted in Fig. 16 is in fact not so relevant, since the range of the importance score is quite limited (values ranging from 0.4992 to 0.5008). In addition, it is possible that negative values of planar curvature have a higher importance score than 0 values or positive values, meaning that concave slopes are more prone to landslides than plain or convex surfaces.
In this work a new landslide susceptibility assessment of Central Asia was carried out as part of a multi-hazard approach in the framework of the Strengthening Financial Resilience and Accelerating Risk Reduction in Central Asia (SFRARR) project. Over 13 000 landslide elements were implemented in a random forest model to create a unique map in order to avoid boundary effects and obtain a more homogeneous and higher-resolution susceptibility map with respect to previous works. The approach used also allowed us to identify the most relevant landslide-predisposing factors: soil type and distance from roads and hypocenters. The size and heterogeneity of the study area required the use of many input variables (some of them never used before in landslide susceptibility assessment) and the elaboration of a high volume of data, as well as the adoption of specific procedures to account for the presence of heterogeneities and uncertainties in the input data (such as the presence of polygon and point landslides). The main limitation of the work is related to the absence of data about the type and geometry of several landslides; in the future a better input landslide inventory could help get to different susceptibility maps for different landslide types. Another limitation is due to the absence of any information about the presence or absence of landslides in Turkmenistan, which did not allow any clear validation of the results for this country.
The results provide a useful tool for landslide scientists, practitioners, and administrators involved in land use planning activities and risk reduction strategies in Central Asia.
The landslide susceptibility model data are not yet available, but they will be provided upon request by the World Bank. The landslide susceptibility model was implemented by using the cited landslide inventory maps, published by the following authors: Behling et al. (2014, 2016), Behling and Roessner (2020), Havenith et al. (2015a), Kirschbaum et al. (2015), Pittore et al. (2018), and Strom and Abdrakhmatov (2018). Other data implemented in the model, such as MERIT DEM, geological formations, the Active Fault Database, the soil-type map, and rainfall maps, are available from Yamazaki et al. (2017), Persits et al. (1997), Styron and Pagani (2020), and https://doi.org/10.5281/zenodo.3939050 (Buchhorn et al., 2020). The full ERA5-Land dataset is available at https://doi.org/10.24381/cds.68d2bb30 (Muñoz-Sabater, 2019b) and https://doi.org/10.24381/cds.e2161bac (Muñoz-Sabater, 2019a). The databases on infrastructures, river networks, PGA, and other landslide inventories were provided by the SFRAAR project partners: RED (Risk, Engineering + Development – Pavia, Italy), OGS (National Institute of Oceanography and Experimental Geophysics, Seismological Research Center, Trieste, Italy), IWPHE (Institute of Water Problems, Hydropower, Engineering and Ecology, Dushanbe, Republic of Tajikistan), ISASUZ (Institute of Seismology of the Academy of Science of Uzbekistan, Tashkent, Uzbekistan), the State Monitoring Service of the Republic of Uzbekistan for tracking dangerous geological processes, and LLP (Institute of Seismology of the Science Committee of the Republic of Kazakhstan, Almaty).
AR implemented the landslide susceptibility model, WF conceived with AR the article structure and collected the data, NN prepared the landslide susceptibility data and supported the model implementation, and FC prepared the infrastructure data for the model implementation and performed the statistical analysis involving the landslide susceptibility areas and the exposed elements. All the abovementioned authors contributed to the writing of the article and the figure graphics. HBH and AS provided the landslide databases from Havenith et al. (2015a) and Strom and Abdrakhmatov (2018). They also provided the landslide pictures for Figs. 3 and 4 and critically reviewed the paper. VT coordinated the work and reviewed the paper. MS and GAB provided the landslide databases of Tajikistan and a part of Uzbekistan.
The contact author has declared that none of the authors has any competing interests.
Publisher’s note: Copernicus Publications remains neutral with regard to jurisdictional claims in published maps and institutional affiliations.
This article is part of the special issue “Regionally consistent risk assessment for earthquakes and floods and selective landslide scenario analysis in Central Asia”. It is not associated with a conference.
This work was developed within the World Bank-funded project Strengthening Financial Resilience and Accelerating Risk Reduction in Central Asia (SFRARR), in collaboration with the European Union and the Global Facility for Disaster Reduction and Recovery (GFDRR), with the goal of improving financial resilience and risk-informed investment planning in the Central Asian countries Kazakhstan, the Kyrgyz Republic, Tajikistan, Turkmenistan, and Uzbekistan. This work represents the outcomes of the Task 7 Landslide Scenario Assessment, coordinated by the UNESCO Chair on Prevention and Sustainable Management of Geo-Hydrological Hazards (University of Florence). In particular, the authors would like to thank Gabriele Coccia and Paola Ceresa from Red Risk Engineering (Pavia, Italy) for providing river network data and for the valuable coordination and constant support, as well as Valerio Poggi and Chiara Scaini from OGS (National Institute of Oceanography and Experimental Geophysics, Seismological Research Center, Trieste, Italy) for providing seismic data (active faults, peak ground acceleration) and exposure data. We would also like to thank the partners from Central Asia for the landslide data and fruitful collaboration, in particular IWPHE (Tajikistan), ISASUZ and the State Monitoring Service of the Republic of Uzbekistan for tracking dangerous geological processes (Uzbekistan), the Institute of Seismology of the National Academy of Sciences of Kyrgyz Republic (ISNASKR), and the Institute of Seismology Limited Lability Partnership (LLP) of Kazakhstan.
This research has been supported by the World Bank Group (Consulting Services Contract No. 8006611 – Regionally consistent risk assessment for earthquakes and floods and selective landslide scenario analysis for strengthening financial resilience and accelerating risk reduction in Central Asia).
This paper was edited by Valerio Poggi and reviewed by Polina Lemenkova and one anonymous referee.
Abdrakhmatov, K., Havenith, H. B., Delvaux, D., Jongmans, D., and Trefois, P.: Probabilistic PGA and Arias Intensity Maps of Kyrgyz Republic (Central Asia), J. Seismol., 7, 203–220, 2003.
Abdrakhmatov, K. Y., Aldazhanov, S. A., Hager, B. H., Hamburger, M. W., Herring, T. A., Kalabaev, K. B., Makarov, P. Molnar, S. V. Panasyuk, M. T. Prilepin, R. E., Reilinger, I. S., Sadybakasov, B. J., Souter, Y. A., Trapeznikov, V. Y., and Tsurkov Zubovich, A. V.: Relatively recent construction of the Tien Shan inferred from GPS measurements of present-day crustal deformation rates, Nature, 384, 450–453, 1996.
Akgun, A. A.: comparison of landslide susceptibility maps produced by logistic regression, multi-criteria decision, and likelihood ratio methods: A case study at İzmir, Turkey, Landslides, 9, 93–106, 2012.
Bazzurro, P. et al.: Strengthening Financial Resilience and Accelerating Risk Reduction in Central Asia – the SFRARR project. The SFRARR probabilistic flood hazard assessment, in preparation, 2023.
Behling, R. and Roessner, S.: Multi-temporal landslide inventory for a study area in Southern Kyrgyz Republic derived from RapidEye satellite time series data (2009–2013), V.1.0. GFZ Data Services [data set], https://doi.org/10.5880/GFZ.1.4.2020.001, 2020.
Behling, R., Roessner, S., Kaufmann, H., and Kleinschmit, B.: Automated spatiotemporal landslide mapping over large areas using rapideye time series data, Remote Sens. 6, 8026–8055, https://doi.org/10.3390/rs6098026, 2014.
Behling, R., Roessner, S., Golovko, D., and Kleinschmit, B.: Derivation of long-term spatiotemporal landslide activity – A multi-sensor time series approach, Remote Sens. Environ., 186, 88–104, https://doi.org/10.1016/j.rse.2016.07.017, 2016.
Brabb, E. E.: Innovative approaches to landslide hazard mapping, in: Proceedings 4th International Symposium on Landslides, Toronto, Canada, 16–21 September 1984, Canadian Geotechnical Society, 1, 307–324, 1984.
Breiman, L.: Random forests, Mach. Learn. 45, 5–32, 2001.
Brenning, A.: Spatial prediction models for landslide hazards: review, comparison and evaluation, Nat. Hazards Earth Syst. Sci., 5, 853–862, https://doi.org/10.5194/nhess-5-853-2005, 2005.
Buchhorn, M., Smets, B., Bertels, L., De Roo, B., Lesiv, M., Tsendbazar, N. E., Herold, M., and Fritz, S.: Copernicus global land service: Land cover 100 m: collection 3: epoch 2019: Globe, Version V3.0.1, Zenodo [data set], https://doi.org/10.5281/zenodo.3939050, 2020.
CAC DRMI: Risk assessment for Central Asia and Caucasus: desk study review, project report, Central Asia and Caucasus Disaster Risk Management Initiative, 2009.
Carrara, A.: Multivariate models for landslide hazard evaluation, J. Int. Assoc. Math. Geol., 15, 403–426, 1983.
Cascini, L.: Applicability of landslide susceptibility and hazard zoning at different scales, Eng. Geol., 102, 164–177, 2008.
Catani, F., Lagomarsino, D., Segoni, S., and Tofani, V.: Landslide susceptibility estimation by random forests technique: sensitivity and scaling issues, Nat. Hazards Earth Syst. Sci., 13, 2815–2831, https://doi.org/10.5194/nhess-13-2815-2013, 2013.
Chedia, O. K. and Lemzin, I. N.: Seismogenerating faults of the Chatkal depression, in: Seismotectonics and seismicity of the Tien Shan, Frunze, Ilim, 18–28, 1980.
Chen, L., Van Westen, C. J., Hussin, H., Ciurean, R. L., Turkington, T., Chavarro-Rincon, D., and Shrestha, D. P.: Integrating expert opinion with modelling for quantitative multi-hazard risk assessment in the Eastern Italian Alps, Geomorphology, 273, 150–167, 2016.
Coccia, G. et al.: The SFRARR probabilistic flood hazard assessment, in preparation, 2023.
Corominas, J., Copons, R., Vilaplana, J. M., Altimir, J., and Amigó, J.: Integrated landslide susceptibility analysis and hazard assessment in the principality of Andorra, Nat. Hazards, 30, 421–435, 2003.
Cruden, D. M. and Varnes, D. J.: Landslide types and processes. Landslides: Investigation and Mitigation, Special Report 247, Transportation Research Board, Washington, 36–75, 1996.
Danneels, G., Bourdeau, C., Torgoev, I., and Havenith, H. B.: Geophysical investigation and dynamic modelling of unstable slopes: case-study of Kainama (Kyrgyzstan), Geophys. J. Int., 175, 17–34, 2008.
Delvaux, D., Abdrakhmatov, K. E., Lemzin, I. N., and Strom, A. L.: Landslides and surface breaks of the 1911 Ms 8.2 Kemin earthquake, Kyrgyzstan, Russ. Geol. Geophys.+, 42, 1667–1677, 2001.
Duman, T. Y., Can, T., Gokceoglu, C., and Sonmez, H.: Application of logistic regression for landslide susceptibility zoning of Cekmece Area, Istanbul, Turkey, Environ. Geol., 51, 241–256, 2006.
Ermini, L., Catani, F., and Casagli, N.: Artificial Neural Networks applied to landslide susceptibility assessment, Geomorphology, 66, 327–343, 2005.
European Commission: Humanitarian Aid, Civil Protection, U.N.I.S. for D.R.R.: Disaster Risk Reduction 20 Examples of Good Practice from Central Asia, European Commission, technical report, 2006.
Frattini, P., Crosta, G., and Carrara, A.: Techniques for evaluating the performance of landslide susceptibility models, Eng. Geol., 111, 62–72, 2010.
GFDRR (Global Facility for Disaster Reduction and Recovery): Disaster Risk Management Notes for Priority Countries 2009–2015, Eur. Asia, GFDRR, technical report, 48–49, 2009.
GFDRR (Global Facility for Disaster Reduction and Recovery): Europe and Central Asia-Country Risk Profiles for Floods and Earthquakes, GFDRR, technical report, 144 pp., 2016.
Goetz, J. N., Brenning, A., Petschkoc, H., and Leopold, P.: Evaluating machine learning and statistical prediction techniques for landslide susceptibility modeling, Comput Geosci., 81, 1–11, 2015.
Golovko, D., Roessner, S., Behling, R., Wetzel, H. U., and Kleinschmidt, B.: Development of multi-temporal landslide inventory information system for southern Kyrgyz Republic using GIS and satellite remote sensing, PFG 2015, 157–172, https://doi.org/10.1127/pfg/2015/0261, 2015.
Havenith, H.-B., Strom, A., Jongmans, D., Abdrakhmatov, A., Delvaux, D., and Tréfois, P.: Seismic triggering of landslides, Part A: Field evidence from the Northern Tien Shan, Nat. Hazards Earth Syst. Sci., 3, 135–149, https://doi.org/10.5194/nhess-3-135-2003, 2003.
Havenith, H. B., Strom, A., Cacerez, F., and Pirard, E.: Analysis of landslide susceptibility in the Suusamyr region, Tien Shan: statistical and geotechnical approach, Landslides, 3, 39–50, 2006a.
Havenith, H. B., Torgoev, I., Meleshko, A., Alioshin, Y., Torgoev, A., and Danneels, G.: Landslides in the Mailuu-Suu Valley, Kyrgyz Republic – hazards and impacts, Landslides, 3, 137–147, 2006b.
Havenith, H. B., Strom, A., Torgoev, I., Torgoev, A., Lamair, L., Ischuk, A., and Abdrakhmatov, K.: Tien Shan geohazards database: Earthquakes and landslides, Geomorphology, 249, 16–31, https://doi.org/10.1016/j.geomorph.2015.01.037, 2015a.
Havenith, H. B., Torgoev, A., Schlögel, R., Braun, A., Torgoev, I., and Ischuk, A.: Tien Shan geohazards database: Landslide susceptibility analysis, Geomorphology, 249, 32–43, 2015b.
Havenith, H. B., Torgoev, A., Braun, A., Schlögel, R., and Micu, M.: A new classification of earthquake-induced landslide event sizes based on seismotectonic, topographic, climatic and geologic factors, Geoenvironmental Disasters, 1, 1–24, 2016.
Havenith, H. B., Umaraliev, R., Schlögel, R., Torgoev, I., Ruslan, U., Schlogel, R., and Torgoev, I.: Past and Potential Future Socioeconomic Impacts of Environmental Hazards in Kyrgyz Republic, in: Kyrgyz Republic: Political, Economic and Social Issues, edited by: Olivier, A. P., Nova Science Publishers, Inc., Hauppauge, NY, USA, 63–113, ISBN 978-1-53612-763-8, 2017.
Hong, Y., Adler, R., and Huffman, G.: Use of satellite remote sensing data in the mapping of global landslide susceptibility, Nat. Hazards, 43, 23–44, 2007.
Ishihara, K., Okusa, S., Oyagi, N., and Ischuk, A.: Liquefaction-induced flow slide in the collapsible loess deposit in Soviet Tajik, Soils Found., 30, 73–89, 1990.
Juliev, M., Pulatov, A., and Hubl, J.: Natural hazards in mountain regions of Uzbekistan: A review of mass movement processes in Tashkent province, International Journal of Scientific & Engineering Research, 8, 1102, https://doi.org/10.14299/ijser.2017.02.013, 2017.
Kalmetieva, Z. A., Mikolaichuk, A. V, Moldobekov, B. D., Meleshko, A. V., Janaev, M. M., and Zubovich, A. V.: Atlas of earthquakes in Kyrgyz Republic, Central-Asian Institute for Applied Geosciences and United Nations International Strategy for Disaster Reduction Secretariat Office in Central Asia, Bishkek, p. 75, 2009.
Kirschbaum, D., Stanley, T., and Zhou, Y.: Spatial and temporal analysis of a global landslide catalog, Geomorphology, 249, 4–15, https://doi.org/10.1016/j.geomorph.2015.03.016, 2015.
Lagomarsino, D., Tofani, V., Segoni, S., Catani, F., and Casagli, N.: A tool for classification and regression using random forest methodology: Applications to landslide susceptibility mapping and soil thickness modeling, Environ. Model. Assess., 22, 201–214, 2017.
Lee, S.: Application of logistic regression model and its validation for landslide susceptibility mapping using GIS and remote sensing data, Int. J. Remote Sens., 26, 1477–1491, 2005.
Li, F., Torgoev, I., Zaredinov, D., Li, M., Talipov, B., Belousova, A., Kunze, C., and Schneider, P.: Influence of Earthquakes on Landslide Susceptibility in a Seismic Prone Catchment in Central Asia, Appl. Sci, 11, 3768, https://doi.org/10.3390/app11093768, 2021.
Manzo, G., Tofani, V., Segoni, S., Battistini, A., and Catani, F.: GIS techniques for regional-scale landslide susceptibility assessment: The Sicily (Italy) case study, Int. J. Geogr. Inf. Sci., 27, 1433–1452, 2013.
Martelloni, G., Segoni, S., Fanti, R., and Catani, F.: Rainfall thresholds for the forecasting of landslide occurrence at regional scale, Landslides, 9, 485–495, 2012.
Medeu, A. R. and Blagovechshenskiy, A. R.: Seismogenic Landslide risk zoning in the surrounding areas of Almaty city, Kazakhstan, Vestnick of Almaty University of Power Engineering and Telecommunications (AUPET), 6, 121–126, 2016.
Molnar, P. and Tapponnier, P.: Cenozoic Tectonics of Asia: Effects of a Continental Collision: Features of recent continental tectonics in Asia can be interpreted as results of the India-Eurasia collision, Science, 189, 419–426, 1975.
Muñoz-Sabater, J.: ERA5-Land monthly averaged data from 1981 to present, Copernicus Climate Change Service (C3S) Climate Data Store (CDS) [data set], https://doi.org/10.24381/cds.e2161bac, 2019a.
Muñoz-Sabater, J.: ERA5-Land hourly data from 1981 to present, Copernicus Climate Change Service (C3S) Climate Data Store (CDS) [data set], https://doi.org/10.24381/cds.68d2bb30, 2019b.
Nadim, F., Kjekstad, O., Peduzzi, P., Herold, C., and Jaedicke, C.: Global landslide and avalanche hotspots, Landslides, 3, 159–173, 2006.
Niyazov, R., Nurtaev, B., Bimurzaev, G., and Tashpulatov, M.: Flow Slides in Uzbekistan: Overview and Case Studies, in: Understanding and Reducing Landslide Disaster Risk, edited by: Casagli, N., Tofani, V., Sassa, K., Bobrowsky, P. T., and Takara, K., WLF 2020, ICL Contribution to Landslide Disaster Risk Reduction, Springer, Cham, https://doi.org/10.1007/978-3-030-60311-3_5, 2021.
Niyazov, R. A. and Nurtaev, B. S.: Evaluation of Landslides in Uzebekistan Caused by the Joint Impact of Precipitation and Deep-focus Pamir-Hindu Earthquakes, in: Landslides: Global Risk Preparedness, edited by: Sassa, K., Rouhban, B., Briceño, S., McSaveney, M., and He, B., Springer, Berlin, Heidelberg, 253–265, https://doi.org/10.1007/978-3-642-22087-6_17, 2013.
Pánek, T., Korup, O., Minár, J., and Hradecký J.: Giant landslides and highstands of the Caspian Sea, Geology, 44, 939–942, 2016.
Persits, F. M., Ulmishek, G. F., and Steinshouer, D. W.: Maps showing geology, oil and gas fields and geologic provinces of the Former Soviet Union, No. 97-470-E, US Geological Survey, https://doi.org/10.3133/ofr97470E, 1997.
Piroton, V., Schlögel, R., Barbier, C., and Havenith, H. B.: Monitoring the recent activity of landslides in the Mailuu-suu valley (Kyrgyz Republic) using radar and optical remote sensing techniques, Geosciences, 10, 164, https://doi.org/10.3390/geosciences10050164, 2020.
Pittore, M., Ozturk, U., Moldobekov, B., and Saponaro, A.: EMCA Landslide catalog Central Asia, V.1.0. GFZ Data Services [data set], https://doi.org/10.5880/GFZ.2.6.2018.004, 2018.
Poggi, V. et al.: Harmonising seismicity information in Central Asia: earthquake catalogue and faults. The SFRARR probabilistic flood hazard assessment, in preparation, 2023a.
Poggi, V. et al.: Development of a state of art probabilistic seismic hazard model for Central Asia countries. The SFRARR probabilistic flood hazard assessment, 2023b.
Pollner, J., Kryspin-Watson, J., and Nieuwejaar, S.: Disaster Risk Management and Climate Change Adaptation in Europe and Central Asia, World Bank, technical report, 1–53, 2010.
Pusch, C.: A comprehensive risk management framework for Europe and Central Asia, No. 9, The World Bank, Disaster Risk Management Working Paper Series, technical report, 2004.
Reichenbach, P., Rossi, M., Malamud, B. D., Mihir, M., and Guzzetti, F.: A review of statistically-based landslide susceptibility models, Earth Sci. Rev., 180, 60–91, 2018.
Roessner, S., Wetzel, H. U., Kaufmann, H., Kornus, W., Lehner, M., Reinartz, P., and Mueller, R.: Landslide Investigations in Southern Kyrgyz Republic Based on a Digital Elevation Model Derived from MOMS-2P Data, Congress “Geoinformation for all”, Amsterdam, the Netherlands, 2000, ISPRS – International Archives of Photogrammetry and Remote Sensing, Vol. 33, Part B7, 1259–1266, 2000.
Roessner, S., Wetzel, H. U., Kaufmann, H., and Sarnagoev, A.: Satellite Remote Sensing and GIS Based Analysis of Large Landslides in Southern Kyrgyz Republic, NATO Advanced Research, in: Workshop Proceedings: Security of Natural and Artificial Rockslide Dams, Bishkek, Kyrgyz Republic, 7–12 June 2004, GFZ Publication, 2004.
Roessner, S., Wetzel, H. U., Kaufmann, H., and Sarnagoev, A.: Potential of Satellite Remote Sensing and GIS for Landslide Hazard Assessment in Southern Kyrgyz Republic (Central Asia), Nat. Hazards, 35, 395–416, 2005.
Saponaro, A., Pilz, M., Bindi, D., and Parolai, S.: The contribution of EMCA to landslide susceptibility mapping in Central Asia, Ann. Geophys.-Italy, 58, S0113, https://doi.org/10.4401/ag-6668, 2015a.
Saponaro, A., Pilz, M., Wieland, M., Bindi, D., Moldobekov, B., and Parolai, S.: Landslide susceptibility analysis in data-scarce regions: the case of Kyrgyz Republic, B. Eng. Geol. Environ., 74, 1117–1136, https://doi.org/10.1007/s10064-014-0709-2, 2015b.
Scaini, C. et al.: A new regionally consistent exposure database for Central Asia: population and residential buildings, in preparation, 2023.
Schiavina, M., Melchiorri, M., Pesaresi, M., Politis, P., Freire, S., Maffenini, L., Florio, P., Ehrlich, D., Goch, K., Tommasi, P., and Kemper, T.: GHSL Data Package, Publications Office of the European Union, Luxembourg, 2022, ISBN 978-92-76-53071-8, 2022.
Schlögel, R., Torgoev, I., De Marneffe, C., and Havenith, H. B.: Evidence of a changing size-frequency distribution of landslides in the Kyrgyz Tien Shan, Central Asia, Earth Surf. Proc. Land., 36, 1658–1669, 2011.
Segoni, S., Tofani, V., Rosi, A., Catani, F., and Casagli, N.: Combination of rainfall thresholds and susceptibility maps for dynamic landslide hazardassessment at regional scale, Front Earth Sci., 6, https://doi.org/10.3389/feart.2018.00085, 2018.
Stanley, T. and Kirschbaum, D. B.: A heuristic approach to global landslide susceptibility mapping, Nat. Hazards, 87, 145–164, 2017.
Sternberg R.: Damming a river: a changing perspective on altering nature, Renew. Sust. Energ. Rev., 10, 165–197, 2006.
Strom, A.: Landslide dams in Central Asia region, Journal of the Japan Landslide Society, 47, 309–324, 2010.
Strom, A. and Abdrakhmatov, K.: Large-Scale Rockslide Inventories: From the Kokomeren River Basin to the Entire Central Asia Region, WCoE 2014–2017, IPL-106-2, in: 4th World Landslide Forum, Ljubljana, Slovenia, 29 May–2 June 2017, Springer, Cham, 339–346, https://doi.org/10.1007/978-3-319-59469-9_28, 2017.
Strom, A. and Abdrakhmatov, K.: Rockslides and rock avalanches of Central Asia: distribution, morphology, and internal structure, Elsevier, 441 pp., ISBN 978-0-12-803204-6, 2018.
Strom, A. L. and Korup, O.: Extremely large rockslides and rock avalanches in the Tien Shan, Kyrgyz Republic, Landslides 3, 125–136, 2006.
Styron, R. and Pagani, M.: The GEM Global Active Faults Database, Earthq. Spectra, 36, 160–180, 2020.
Thurman, M.: Natural Disaster Risks in Central Asia: A Synthesis, UNDP BCPR, technical report, 2011.
Tien Bui, D., Tuan, T. A., Klempe, H., Pradhan, B., and Revhaug, I.: Spatial prediction models for shallow landslide hazards: a comparative assessment of the efficacy of support vector machines, artificial neural networks, kernel logistic regression, and logistic model tree, Landslides, 13, 361–378, 2016.
Tiranti, D., Nicolò, G., and Gaeta, A. R.: Shallow landslides predisposing and triggering factors in developing a regional early warning system, Landslides, 16, 235–251, 2019.
Trifonov, V. G., Makarov, V. I., and Scobelev, S. F.: The Talas-Fergana active right-slip faults, Ann. Tectonicae, 6, 224–237, 1992.
Trigila, A., Frattini, P., Casagli, N., Catani, F., Crosta, G., Esposito, C., and Spizzichino, D.: Landslide susceptibility mapping at national scale: the Italian case study, in: Landslide Science and Practice, Springer, Berlin, Heidelberg, 287–295, https://doi.org/10.1007/978-3-642-31325-7_38, 2013.
Ullah, S., Bindi, D., Pilz, M., Danciu, L., Weatherill, G., Zuccolo, E., Anatoly Ischuk, A., Mikhailova, N. N., Abdrakhmatov, K., and Parolai, S.: Probabilistic seismic hazard assessment for Central Asia, Ann. Geophys.-Italy, 58, 1, https://doi.org/10.4401/ag-6687, 2015.
UNISDR: Risk assessment for Central Asia and Caucasus: desk study review, CAC DRMI 2009, Risk Management Working Paper Series No. 9, The World Bank, technical report, 2009.
World Bank: Natural Disaster Hotspots: Case Studies, Disaster Risk Management Series No. 6, World Bank, technical report, 2006.
World Bank: Investigation and Analysis of Natural Hazard Impacts on Linear Infrastructure in Southern Kyrgyz Republic Desk and Field Studies Report, World Bank, Report 68669, 2008.
World Bank: The Global Landslide Hazard Map: Final Project Report, World Bank, technical report, Appendix A, 2020.
Yamazaki, D., Ikeshima, D., Tawatari, R., Yamaguchi, T., O'Loughlin, F., Neal, J. C., Sampson, C. C., Kanae, S., and Bates, P. D.: A high-accuracy map of global terrain elevations, Geophys. Res. Lett., 44, 5844–5853, 2017.
Yilmaz, I.: Landslide susceptibility mapping using frequency ratio, logistic regression, artificial neural networks and their comparison: a case study from Kat landslides (Tokat–Turkey), Comput. Geosci., 35, 1125–1138, 2009.
Zubovich, A. V., Wang, X. Q., Scherba, Y. G., Schelochkov, G. G., Reilinger, R., Reigber, C., Mosienko, O., Molnar, P., Michajljow, W., Makarov, V. I., Li, J., Kuzikov, S. I., Herring, T. A., Hamburger, M. W., Hager B. H., Dang, Y., Bragin, V. D., and Beisenbaev, R.: GPS velocity field for the Tien Shan and surrounding regions, Tectonics, 29, 23, https://doi.org/10.1029/2010TC002772, 2010.