the Creative Commons Attribution 4.0 License.
the Creative Commons Attribution 4.0 License.
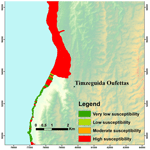
Landslide susceptibility assessment in the rocky coast subsystem of Essaouira, Morocco
Abdellah Khouz
Jorge Trindade
Sérgio C. Oliveira
Fatima El Bchari
Blaid Bougadir
Ricardo A. C. Garcia
Mourad Jadoud
In recent decades, multiple researchers have produced landslide susceptibility maps using different techniques and models, including the information value method, which is a statistical model that is widely applied to various coastal environments. This study aimed to evaluate susceptibility to landslides in the Essaouira coastal area using bivariate statistical methods. In this study, 588 distinct landslides were identified, inventoried, and mapped. Landslides are performed by means of observation and interpretation of different data sources, namely high-resolution satellite images, aerial photographs, topographic maps, and extensive field surveys. The rocky coastal system of Essaouira is located in the middle of the Atlantic coast of Morocco. The study area was split into 1534 cliff terrain units that were 50 m in width. For training and validation purposes, the landslide inventory was divided into two independent groups: 70 % for training and 30 % for validation. Twenty-two layers of landslide conditioning factors were prepared – namely, elevation, slope angle, slope aspect, plan curvature, profile curvature, cliff height, topographic wetness index, topographic position index, slope over area ratio, solar radiation, presence of faulting, lithological units, toe lithology, presence and type of cliff toe protection, layer tilt, rainfall, streams, land-use patterns, normalised difference vegetation index, lithological material grain size, and presence of springs. The statistical relationship between the conditioning factors and the different landslide types was calculated using the bivariate information value method in a pixel-based model and in the elementary terrain units-based model. Coastal landside susceptibility maps were validated using landslide training group partitions. The receiver operating characteristic curve and area under the curve were used to assess the accuracy and prediction capacity of the different coastal landslide susceptibility models. Two methodologies, considering a pixel-based approach and using coastal terrain units, were adopted to evaluate coastal landslide susceptibility. The results allowed for the classification of 38 % of the rocky coast subsystem as having high susceptibility to landslides, which were mostly located in the southern part of the Essaouira coastal area. These susceptibility maps will be useful for future planned development activities as well as for environmental protection.
- Article
(8222 KB) - Full-text XML
-
Supplement
(431 KB) - BibTeX
- EndNote
Landslides are common processes in the rocky coastal system of Essaouira. They result from the interaction of sub-aerial, marine, and anthropogenic processes (Trenhaile, 1987; Sunamura, 1992; Hampton et al., 2004; Greenwood and Orford, 2007), making this system more susceptible to anthropogenic pressure and erosional processes than any other natural system. Consequently, fast and dynamic evolution imposes restrictions on the way humans occupy coastal areas (Teixeira, 2006; Marques, 2009; Teixeira et al., 2015; Moore and Davis, 2015; Gilham, 2018).
The process of creating landslide susceptibility maps generally involves several qualitative or quantitative approaches, starting with landslide inventory as the first step for assessing landslide susceptibility, hazard, and risk (Aleotti and Chowdury, 1999; Dai and Lee, 2002; Van Westen et al., 2008; Corominas et al., 2014; Oliveira et al., 2017; Meena et al., 2019). For rocky coastal areas, landslide susceptibility and hazard assessments mainly address the evaluation of cliff retreat (Oliveira et al., 2008, 2017; Rocha et al., 2007), landslide inventorying, and susceptibility mapping (Marques et al., 2011). The identification of factors controlling the rocky coast system is a critical step for better understanding how this system is evolving and to predict its future evolution (Neves and Ramos-Pereira 1999). Landslides are responsible for significant erosion in rocky coastal systems (Andriani and Walsh, 2007; Violante, 2009; Sunamura, 2015). Therefore, by knowing the set of predisposing factors that condition landslide occurrence, it is possible to spatially predict where future landslides will occur (Varnes, 1984). Many different landslide conditioning factors play an important role in the preparation of landslide susceptibility maps (Zêzere, 2002). These factors, which are dependent of the analysis scale and landslide type, generally include elevation, slope, aspect, plan and profile curvature, topographic wetness factor index (TWI), topographic position index (TPI), slope over area ratio (SOAR), solar radiation, faulting, lithology, lithological layers tilt, precipitation, streams, land-use patterns, normalised difference vegetation index (NDVI) or vegetation density factor, grain size, and spring presence (Van Westen et al., 2008; Reichenbach et al., 2018; Pereira et al., 2020). When related to susceptibility assessments of sea cliffs, landslide conditioning factors also include cliff edge height and coastal slope toe protection (Marques et al., 2011, 2013; Marques, 2018; Gilham, 2018; Letortu et al., 2019; Queiroz and Marques, 2019). In this study, we followed the classification of Cruden and Varnes (1996), Varnes (1978), WP/WLI (1993), and Dikau et al. (1996) to differentiate the types of landslides that may occur in coastal cliffs: falls, slides, topples, lateral spreads, and flows. Identifying landslide types remains challenging, even when supported by intensive fieldwork, which often faces the lack of clear evidence associated with the degradation of landslide features or inaccessibility to cliff faces (Neves et al., 2012). Datasets of aerial photographs can be used to overcome these limitations (Oliveira et al., 2017). Multiple bivariate and multivariate statistical models are used to analyse landslide susceptibility, and most of these models require a subdivision of territory into terrain units and the selection of the appropriate type of terrain mapping units (e.g. grid cells, slope units, geo-hydrological units, unique condition units, and administrative units (Van Den Eeckhaut et al., 2009; Marques et al., 2011, 2013; Epifânio et al., 2014; Corominas et al., 2014; Zêzere et al., 2017).
Data-driven approaches are the most commonly used for landslide susceptibility and hazard zonation (Kanungo et al., 2006; Girma et al., 2015; Hamza and Raghuvanshi, 2017; Mengistu et al., 2019; Shano et al., 2020), whereas other approaches – such as bivariate, multivariate, and active learning statistical methods – are also suitable for assessing susceptibility (Corominas et al., 2014). Bivariate statistical methods use inductive logic, which assumes that the combination of conditions pertaining to various conditioning factors, analysed separately, may lead to landslide prediction in a given area. The evaluation of conditioning factors and their relationship with past landslides in the study area forms the basis for the prediction of places where future landslides may occur (Varnes, 1984 ; Van Westen et al., 1997; Dai and Lee, 2002; Lan et al., 2004; Zêzere et al., 2004; Lee and Pradhan, 2007; Girma et al., 2015; Wang et al., 2016; Chimidi et al., 2017; Shano et al., 2020).
The information value (IV) method (Yin and Yan, 1988) is considered to be appropriate for evaluating landslide susceptibility (Corominas et al., 2014). It has been widely used worldwide, with different geomorphological backgrounds (Yin and Yan, 1988; Jade and Sarkar, 1993; Lin and Tung, 2003; Yalcin, 2008; Balasubramani and Kumaraswamy, 2013; Zêzere et al., 2017; Mengistu et al., 2019). The IV model is based on the weighted presence or absence of drivers of slope instability. Thus, the landslide density for conditioning factor classes can be determined by overlaying maps of both conditioning factors and inventoried landslides (Mengistu et al., 2019; Shano et al., 2020). If the resulting IV is positive, the causative factor class represents a strong interdependence with landslides in the area (Yin and Yan, 1988; Shano et al., 2020), and the weighted value of a conditioning factor class can be represented as the natural logarithm of the landslide density in a factor class divided by the landslide density in the total map area (Van Westen et al., 1997; Shano et al., 2020).
Validation of the landslide susceptibility map is essential for evaluating the predictive capacity of the model. It can be considered as a test of the model's ability to reflect the real environment trough and to evaluate its accuracy and predictive capacity (Beguería, 2006; Frattini et al., 2010; Shano et al., 2020; Mateus et al., 2021). The receiver operator characteristic (ROC) is a recognised technique used in statistical approach validations to check the performance of the predictive ability of bivariate methods (Shano et al., 2020). This represents a plot of the probability of correctly identified landslides against the probability of incorrectly identified landslides (Gorsevski et al., 2006; Shano et al., 2020).
However, the dynamics of the Essaouira coastal area have been poorly studied. A beach-based granulometric, technical study was conducted in Essaouira Bay in 1955 by the hydraulic laboratory of Neyrpic (El mimouni and Daoudi, 2012). Other studies have focused on the general morphology of sandy dunes in the upper part of the beach and on the mainland (Gentile, 1997; Simon, 2000; Lharti et al., 2006). Alternatively, this study aims to (i) define the type and emplacement of each landslide by means of an inventory validated using a field survey, (ii) identify the most important predisposing variables that control the spatial distribution of different landslide types, (iii) set and weight the different conditioning factors by applying the IV statistical method, (iv) assess landslide susceptibility in Essaouira coastal cliffs for different landslide types and classify susceptible areas according to the occurrence of landslides, and (v) validate the susceptibility map.
The Essaouira coastal area is located along the middle of the Atlantic coast of Morocco (Fig. 1) and extends over 134 km. It has high coastal system diversity, including estuaries, bays, beaches, sandy spits, cliffs, and rocky shore platforms (Weisrock, 1980; Simon, 2000; Lharti et al., 2006). A classification was applied based on three subsystems: sandy coast, rocky coast, and anthropic coast. The study site (Fig. 1) is characterised by stretches of sandy coast (48 %), rocky coast (51 %), and anthropic coast (1 %, the port of Essaouira), delimited to the north by the Tensift estuary, to the south by Timzguida Ouftas village, to the east by Essaouira province municipalities, and to the west by the Atlantic Ocean and the island of Mogador in front of Essaouira City (Fig. 1). This coastal area has a predominantly semi-natural landscape which is locally interrupted by heavily anthropised coastal areas, particularly in Essaouira City (Fig. 1).
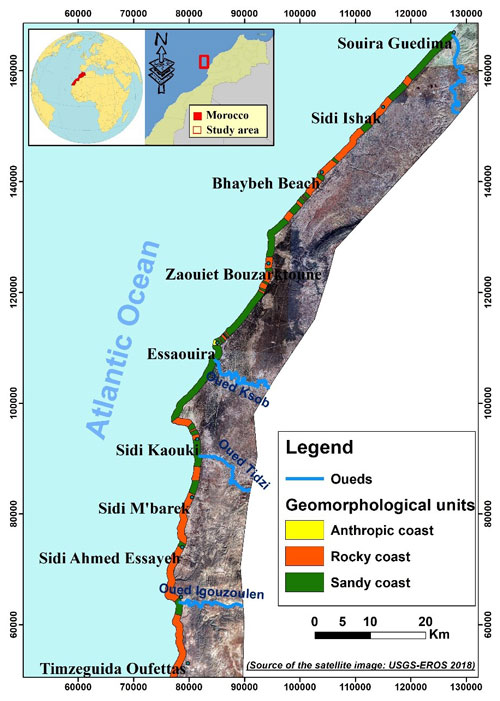
Figure 1Geographic location of Essaouira coastal area and its sandy and rocky coast subsystems (coordinate systems: Lambert Zone I projection).
Geologically, the study area is located in the Atlantic Atlas, which is considered to be the westernmost part of the High Atlas mountains (Weisrock, 1980), with its northern and largest plateau (Haha and Chiadma) dropping gently from SE to NW, in accordance with the overall structural framework. However, the landscape is varied, crossed by cuestas and vigorous crests, turned towards the SE, and associated with the frequent alternations of sandstone, dolomitic, limestone, marl, clay, and gypsum layers. The landscape is interrupted by sudden, isolated anticlinal folds, such as the Jbel Hadid (725 m high) to the north or the Jbel Ouamsitten (900 m high) to the south. Towards the west, the abundance of consolidated dunes and sandstones with oblique stratification and conglomeratic levels is relevant (Weisrock, 1980). To the south, a coastal basin with original sedimentary material known as the “Haha Basin” (Dufaud et al., 1966) is associated with the opening of the North Atlantic, which is generally consistent with the end of the Triassic (Choubert et al., 1971; Hallam, 1971; Le Pichón, 1971; Weisrock, 1980). It consists mainly of sandstones, pelites, conglomerates, and red salt clays, with essentially continental facies. Deep marine sedimentation was successful from the Lower Liassic to the Upper Cretaceous. During these long periods, the sedimentation of the coastal basin constantly oscillated between an epicontinental regime, with terrigenous deltaic or alluvial contributions and marine organogenic or evaporitic deposits, and a more open marine regime, with neritic limestones and marls. Towards the north, the coastal platform – also called the “Moghrebian platform”, from the name attributed to the sandy and sandstone deposits that cover it (Choubert and Ambrogi, 1953; Weisrock, 1980) – is largely developed and thus tapers off at the southern mountainous part.
From a structural point of view, the study area is characterised by a double structural division marked by a close adaptation to the hydrographic network (Weisrock, 1980). The first structural division is linked to the opening of the Atlantic, including the extensional faults fundamentally oriented NNE–SSW, for the entire Atlantic Atlas and its northern edge. The second direction may have the same origin as the first; these Hercynian breaks in the basement influenced sedimentation and then reappeared, affecting the cover during the Atlasic phases (Saadi, 1972). This second direction, WNW–ESE, related to the opening of the Atlantic, is increasingly evident (Oued Ksob, northern fallout anticlines, Oued Tensift). This direction is attenuated towards the S, while in the central region (Tamanar Plateau), a W–E direction appears as a result of the ancient Hercynian direction (Saadi, 1972; Michard et al., 1975). In addition to these two fundamental systems and because of the thickness of the saliferous clay layer, the Essaouira region is marked by the development of diapiric-style tectonics, particularly well represented in the SE of Essaouira (Weisrock, 1980).
From a geomorphological point of view, landform distribution in the study area is asymmetrical: all the plateaus dominate to the N and NW sectors and are almost absent to the S and SE sectors occupied by the mountain. In accordance with the general layout of the High Atlas, the altitudes increase towards the south and east. Thus, the morphogenesis of the Atlantic Atlas depends on general physical geography in addition to the structural morphology of the folded chains, the phenomena of encrustation, coastal eolian constructions, and glaciation (Weisrock, 1980). The Atlantic Atlas is open to oceanic influences. This area is particularly characterised by its dual character as a mountainous and coastal region, which makes it possible to link continental and marine morphology; the latter offers the advantage of being able to establish a solid chronological base from the Pliocene onwards by comprising a whole series of stepped fossil beaches. The coastal area has a uniform appearance from north to south. On average, the Mesozoic bedrock disappears under a sandy cover shaped into innumerable encrusted hills along the ocean (Weisrock, 1980).
From a hydrological point of view, the presence of two large watersheds, Oued Tensift and Oued Ksob, were noted, to which coastal Oueds are added: Oued Tidzi and Oued Igouzoullen. These hydrographic networks are an important source of sediment supply and are characterised by a flow which is roughly carried out from E to W, rather faithfully adapted to the topographic framework; however, the courses of the valleys, more often monoclinal or orthoclinal than cataclinal, reveal a long evolution and successive re-adaptations (Weisrock 1980).
The Essaouira cliffed coastal sector is characterised by the presence of multiple landslide types, which are the dominant hazards responsible for the constraints of human activities and safe land use (Moore and Griggs, 2002). The seismic context shows that the coast between Safi and Essaouira has landslide activity that is likely related to seismic events (Elmrabet et al., 1989). The most significant of which, capable of causing disproportionate effects on a highly unstable cliff, occurred in 1757, on 7 March 1930 (32∘ N, 11.5∘ W, M=5.1, felt in Casablanca, Safi, and Essaouira, intensity IV), and on 2 August 1963 (34.7∘ N, 8.9∘ W, M=4.1, felt in Casablanca and Mohammedia, intensity IV). In the 1757 event, the landslide could also have been conditioned by an aftershock of the earthquake on 1st November 1755 affecting the natural instability of the cliff, which had been enhanced by the effects of the tidal wave (Elmrabet et al., 1989).
Climatically, the Atlantic Atlas is located at a relatively low latitude (approximately 31∘ parallel), which places it under the predominant influence of subtropical anticyclonic cells at the limit of the great displacements of polar air masses. It is a position sensitive to the slightest deviations of these centres of action; thus, it is particularly interesting to reconstitute the possible conditions of past climatic oscillations identified by their morphogenetic marks (Weisrock, 1980).
The Essaouira province is characterised by a steppe climate of type BSh, according to the Köppen–Geiger classification, with low rainfall, an average annual temperature of 18.7 ∘C in Essaouira City , and average annual rainfall of 295 mm (https://en.climate-data.org/, last access: 14 November 2022). The dominant climate in the Essaouira region is semi-arid, with diverse temperature and precipitation values. This is because of the oceanic (Atlantic) setting on one side and the height of the mountains on the other. The Essaouira region is an area where hot summer and humid winter winds are constantly alternating between the “Chergui” (the hot wind from the Sahara) and the northeast wind that blows almost all year round. The Essaouira region is characterised by a mild climate throughout the year. The average temperatures are 16.4 ∘C in January and 22.5 ∘C in August. The annual rainfall is approximately 280 mm. Two main seasons can be distinguished: (i) the wet season that includes winter and autumn, with a monthly maximum fluctuating between December and November (precipitation peaks are clearly marked in autumn and winter before gradually decreasing from February to May), and (ii) the dry season from April to September, which is characterised by scarce rainfall; July and August are the driest months throughout the year, with almost no rainfall. Regarding the spatial distribution, both the precipitation and humidity are higher in the coastal zone, and they are always >75 %. Summer fog is particularly important in Essaouira and other sites that are exposed to maritime influences (Hander, 1993).
Using the rainfall data from stations of Adamna, Chichaoua, Talmest, Abadla, and Igrounzar, which were provided to us by the Tensift Water Basin Agency, the average monthly variability of rainfall was analysed for the period 1965–2015, and results show the existence of a rainy season between October and April, with a maximum in March for the Abadla and Chichaoua stations and a maximum in December and November for the Talmest, Igrounzar, and Adamna stations. The dry season extends between June and September, with the lowest rainfall recorded in July and August. The monthly variation in rainfall showed an average of 15.3 mm for Chichaoua and 14.4 mm for Abadla. Rainfall was similar over the same period for Chichaoua and Abadla. The values observed from October to April exceeded the average rainfall for each of these two stations, with a maximum in March (27 mm) and a minimum in July (0.5 mm) and August (1 mm). Thus, the evolution of monthly precipitation was the same at these two stations. It argues in favour of a simple hydrological regime characterised by a regular annual alternation of high and low water. The monthly rainfall at the three stations Adamna, Talmest, and Igrounzar showed that the maximum rainfall was recorded in November and December, while the average rainfall was approximately 20 mm for Igrounzar and Talmest and 26 mm for Adamna.
Regarding the annual variations in rainfall at the five stations, the Adamna, Igrounzar, and Talmest stations have mean annual rainfall of 322, 229.1, and 255.4 mm, respectively. At the Adamna station, there were several rainy years with values that greatly exceeded the interannual average – namely 1987–1988, 1988–1989, 1994–1995 to 1996–1997, 2008–2009 to 2010–2011, with a maximum rainfall of approximately 718 mm in 1995–1996 and a minimum of approximately 136 mm in 2006–2006. For the Igrounzar station, the highest rainfall was observed during 1987–1988, 1988–1989, 1994–1995 to 1996–1997, 2008–2009, and 2009–2010. However, the least rainy years were 1968–1969, 1976–1977, 1991–1992, and 2014–2015. For the Talmest station, the wettest year was 1995–1996, with 559.5 mm, and the driest year was 2014–2015. For this station, we did not have data for the years 1971–1972 until 1975–1976.
From a hydrogeological point of view, the Essaouira Basin and its coastal zone constitute a set of independent but very similar hydrogeological systems that correspond to synclinal basins. Groundwater exists only in localised areas within these systems. Water generally circulates at depth in different limestone or sandstone levels by means of karstic pathways and comes out in the form of springs at low points in contact with an impermeable clay or marl level (Cochet and Combe, 1975). The combination of the effects of tectonics and diapirism has caused the compartmentalisation of the basin into several aquifer systems.
For example, the piezometry of the Plio-Quaternary aquifer shows an overall flow direction from E–SE to W–NW, conditioned by the straightening of its bedrock to the east following the uplift of the Tidzi diapir (Mennani, 2001). There are significant fluctuations between periods of high and low water (Fekri, 1993; Mennani, 2001; Bahir et al., 2002, 2017), which are related to precipitation, which thus controls the regime of the phreatic aquifer. Several problems related to water scarcity and long, recurrent periods of drought have been observed in the Essaouira region in recent decades (Bahir et al., 2002, 2017; Chkir et al., 2008; Chamchati and Bahir, 2013). For this reason, the piezometric level in the study area tends to decline with the inability of some other wells to recover their initial water level, aggravated by the combined effect of the year 1995, the driest year that Morocco experienced during the 20th century (Bahir et al., 2002), and overexploitation (Chkir et al., 2008; Bahir et al., 2017).
The current research used different data sources for landslide susceptibility analysis, and their preparation was supported by field surveys and validation. The methodological steps considered for training and validation of the coastal landslide susceptibility models are shown in Fig. 2 and follow this sequence: (i) elaborate the landslide inventory, classifying the landslides by type and depth of the rupture surface (shallow and deep); (ii) prepare a set of 22 conditioning factors grouped into 7 categories (topographical, geomorphological, lithological, geotechnical, hydrological, climatic, and tectonic); (iii) model coastal landslide susceptibility using the IV method for the Essaouira coastal area, using pixels and elementary terrain units (ETUs); and (iv) independently validate the predictive susceptibility models using ROC curves and area under the curve (AUC).
Coastal systems were classified into sandy and rocky subsystems according to morphometric and operational criteria, and the ETU was defined based on the methodology proposed by Marques et al. (2011); the upper and lower limits of the terrain units were defined by the bottom and the top of the cliff, respectively, while the lateral limits were geometrically drawn perpendicular to the contour lines of the topography and defined by the segmentation of the ridge line into 50 m wide sections. In total, there were 1534 terrain units on the rocky coast. Each terrain unit was classified as stable or unstable based on the quantification of the percentage of unstable area of each slope unit.
3.1 Landslide inventory
The most essential part of the landslide susceptibility assessment framework is the landslide inventory, which includes the identification of the landslide location, size, type, and depth to understand the relationship between landslide occurrence and the dataset of predisposing factors (Ercanoglu and Gokceoglu, 2004; Van Westen et al., 2006; Petley, 2008; Epifânio et al., 2013). The landslide inventory was of the historical type, with no past date of occurrence limits, and was based on the interpretation of different data sources covering the entire study area (Table 1), such as historical records, 10 m-resolution Sentinel satellite imagery, high-resolution ortho-imagery analysis, and intensive field investigation.
The identification of the landslides was based on the interpretation of their specific morphological features that are noticeable in high-resolution imagery, including the crown, main scarp, flanks, body, and toe (Pawluszek, 2019). Other features include the presence of flow materials along gullies, streams with different erosional features, flow tracks, scars along the cliff face, and block deposits on the cliff base (Epifânio et al., 2013; Elkadiri et al., 2014). In addition, extensive field observations were used to validate the inventory and to add new landslides that were not observed in satellite images or identified in other data sources.
3.2 Conditioning factors
Conditioning factors describe terrain conditions that are directly or indirectly associated with landslide occurrence and are essential for landslide susceptibility mapping based on data-driven methodologies. Different types of variables (conditioning factors) were compiled and/or generated in a GIS for susceptibility analysis. According to Marques et al. (2011, 2013), all conditioning factors influencing the stability of coastal cliffs and slopes should be considered, because they may contribute to predicting the spatial occurrence of future instability. Notably, the selection of conditioning factors associated with these processes appears to be a difficult task, because these factors typically work in combination in a multivariate system (Epifânio et al., 2013; Reichenbach et al., 2018).
Based on geomorphological characteristics, bibliography, and field surveys, 22 landslide conditioning factors were selected for the study area. From these, the following 10 conditioning factors were computed from a freely available digital elevation model (ALOS PALSAR RTC DEM) with a 12.5 m resolution (source: https://search.asf.alaska.edu/, last access: July 2021): elevation, slope angle, slope aspect, slope plan curvature, slope profile curvature, cliff height (calculated by the average of top pixels in each ETU), topographic wetness index, topographic position index (classified considering the distance of SD to the mean value for both sides of the distribution), slope over area ratio (using a base 10 logarithmic progression of class limits), and solar radiation (Table 2).
Solar radiation was used as a proxy variable for slope aspect, because it enables the quantification of the weight of trivial qualitative quadrants (Epifânio et al., 2013). Slope angle is the most important predisposing factor for the occurrence of landslides (Mancini et al., 2010); however, in our study area, the slope angle does not have the same importance for all landslide types, and plan and profile curvatures can be associated with the acceleration and deceleration of the flow as well as with the convergence or divergence of the flow and can influence the local drainage systems and the kinematics of landslides (Mancini et al., 2010).
The land-use map and the NDVI were extracted from Sentinel images 2021 (10 m resolution, Table 1). The lithology, toe lithology, and faulting data were obtained from the compilation of a bibliographical review and from three digitised geological maps: Tamanar and Taghazout 1:100 000 scale in the southern section, and Marrakech 1:500 000 scale for the northern section, completed with the field survey.
Meteorological data and historical rainfall records were used for extracting the rainfall factor using the arithmetic mean method (Smaij, 2011), which consists of calculating the annual arithmetic mean of the values obtained at the weather stations and projecting them using inverse distance weighting interpolation. Field surveys, topographic maps (1:10 000), and digital elevation models (DEM) were used to identify and map the stream networks. The presence and type of cliff toe protection, lithology tilting, and the presence of springs were extracted from satellite image observations and field surveys.
Field work revealed that most landslides occur above weak or friable layers, making geotechnical properties a factor to account for. Moreover, the grain size was added to the variable list after data extraction from 16 samples using the BetterSize laser particle size analyser 9300S (Table 2). The grain sizes of clay, silt, and sand (Table 2) were spatially identified as the same predisposing factors. The sampling sites are shown in Fig. 6 (red arrows). Using the loss on ignition (LOI) method (Heiri et al., 2001), organic matter content analysis was also applied to the samples as an important factor that has a strong relationship with the presence of vegetal cover (Table 2), which indicates that the presence of water promotes the occurrence of landslides.
3.3 Susceptibility modelling and validation
The method used to evaluate the susceptibility to the occurrence of coastal landslides is the IV (Yin and Yan, 1988; Zêzere, 2002), which is a bivariate statistical method particularly suited to studying relationships between the dependent variable (landslides) and the set of independent conditioning factors. This method has been successfully applied in coastal areas worldwide (Marques et al., 2011, 2013; Epifâneo et al., 2013, 2014).
Using this bivariate statistical method, it is possible to weight each class of each predisposition factor of slope instability in an objective and quantified manner.
The IV score (Ii) for any class Xi of an independent variable (X) was determined for each landslide type Y using the following equation:
where Si is the number of cells with landslides and variable Xi in the Essaouira coastal area. Ni is the number of cells with variable Xi in the Essaouira coastal area. S is the total number of cells with landslides in the Essaouira coastal area, and N is the total number of cells in the Essaouira coastal area.
When a class of conditioning factors does not have registers of landslides (Si=0), the Ii score is not calculated because of the impossibility of logarithmic normalisation, and it is assumed that that class has an Ii score lower than the minimum registered. For example, the minimum IV index was −5.7014031 for slope aspect Class 1 (flat areas) for deep translational slides; therefore, we took −5.702 for variable classes without any landslide.
The final value of susceptibility to landslides calculated for each cell j corresponds to the sum of the Ii scores present in that unit, given by the following equation:
where m is the number of variables and Xij is equal to 1 or 0, depending on whether variable Xi is present in cell j.
To assess coastal landslide susceptibility, 15 predictive models were individually developed for each inventoried landslide type in this coastal area, considering the landslide partitions defined in Table 3 and the standard model procedures defined in Sect. 3. Table 3 shows the 15 different landslide partitions according to the landslide type used for assessing landslide susceptibility: total landslides, deep landslides, shallow landslides, deep rotational slides, shallow rotational slides, deep translational slides, shallow translational slides, rock topples, rock falls, rock slides, debris falls, debris flows, and debris slides. With these landslide dataset partitions, we expect to better understand the drivers responsible for the occurrence of different landslide types in this coastal area. Each landslide type inventory dataset was then subdivided into training and validation groups (Remondo et al., 2003). The training group, containing 70 % of the inventory, was used in the model building, and the validation group, containing 30 % of the inventory, was used to conduct an independent cross-validation process over the model's first results; the partition was selected randomly, because it agrees with the commonly used partitions used for landslide susceptibility model training and validation (Chen et al., 2020). We also adopted a sensitive approach to eliminate some landslide conditioning factors that have little or no contribution to landslide occurrence based on the IV score results.
Additionally, to assess the importance of the representativeness of the inventory, susceptibility modelling was also considered for some landslide types, splitting them into two subgroups considering the depth of the rupture surface: shallow and deep-seated for rotational and translational slide types.
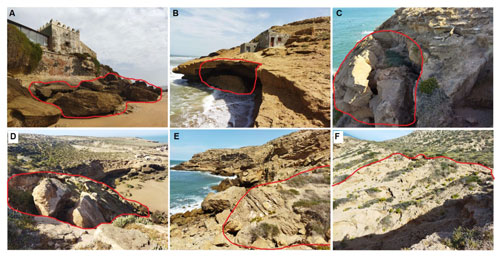
Figure 3Some landslide type examples from the study area: (A, B) rock falls, (C) rock topple, (D) translational slide, (E) rotational slide with back tilting, and (F) debris flow.
For the pixel terrain unit approach, susceptibility was assessed for the different landslide types, and all dependent and independent variables were transformed into a spatial grid database with a 12.5×12.5 m resolution following the DEM pixel size, and all the data were projected in the Lambert conformal conic Zone 1 coordinate system with Merchich datum.
For the ETU approach, to assess landslide susceptibility, the application of any statistical method requires partitioning the study area into smaller terrain units. In the present study, the main modelling was developed on a pixel-based model, and the conditioning factor layers were transformed into ETUs considering the weight of each factor in each ETU in order to apply the terrain unit method and to compare the two approaches (pixel and ETU).
However, susceptibility results are harmonised in ETUs. The ETU use is done for the following reasons: (i) they have a strong relationship with the morphology and geometry of the system that we are trying to model; (ii) they are well fitted to the most used land-use planning formats, as they are mostly vector approaches and are system based, either as a physical system or a human settlement; and (iii) they are also a factor of uniformity and help deal with heterogeneous data (Calvello et al., 2015). Additionally, for planning purposes, it is easier to clearly identify the ETU in the territory when compared to pixels.
4.1 Landslides in cliffs and coastal slopes of Essaouira
The detected landslides were assigned according to the classifications of Varnes (1978, 1996), WP/WLI (1993), Cruden and Varnes (1996), Dikau et al. (1996), and Hungr et al. (2014), and 10 landslide types were identified: debris fall, debris flow, debris slide, rock fall, rock slide, rock topple, deep rotational slide, shallow rotational slide, deep translational slide, and shallow translational slide.
Expert and fieldwork inventory validation allowed for landslide limit corrections and the identification of new landslides. Some examples of landslides are shown in Fig. 3.
The final inventory of the study area comprised 588 landslide records (Fig. 4). Rock falls were the most frequent slope instability phenomena in the study area, with 149 records, followed by rotational slides, while the least frequent landslide type was debris fall, with only 6 records. Most of the study area was occupied by translational slides (68 %) followed by rotational slides. These landslide types typically have larger areas per landslide, deeper rupture surfaces, and frequently occur along the entire cliff and coastal slope profile.
Slope instability was present along the whole study area, resulting in the identification of 974 ETUs with landslides (63.5 %) and 28 797 unstable pixels (46.5 %).
Nevertheless, the heterogeneity of the spatial distribution of landslide types (Fig. 5) over the study area was higher in the southern section because of the higher concentrations of rotational and translational slides.
4.2 Driving forces of instability in Essaouira
Lithology, structure, and landslide deposits are important conditioning factors for susceptibility analysis. These can be proxies for permeability, shear strength, and propensity for physical and chemical weathering of rock and soil materials (Varnes, 1984; Epifânio et al., 2013). Twenty main lithological units were identified in the study area: (1) calcareous crusting; (2) clay and sandstone; (3) conglomerate and dune sediments; (4) conglomerate with sandy matrix; (5) dolomitic limestones; (6) dolomitic sandstones; (7) dune sandstone with oblique stratification; (8) Essaouira sandstone calcarenite; (9) friable sandstone layer; (10) grey clays; (11) grey marls; (12) heterogeneous conglomerate; (13) limestone bar; (14) lumachelic clayey limestones; (15) marls; (16) marly limestones; (17) pudding conglomerate; (18) sandstone dolomites; (19) sequence of marls and marly limestone; and (20) terrigenous red deposit (Table 4).
The spatial distribution of the lithological units (Table 4) shows that, in general, limestone units are more frequent in the southern sector and are frequently combined with grey marls and clays of the Hauterivian and Aptian (Cretaceous). Calcareous crusting, friable sandstone layers, and terrigenous deposits are found in all coastal areas. The conglomerate and sandstone units are more concentrated in the northern sector, where consolidated dunes can also be found.
Regarding the number of ETUs per lithology type, calcareous crusting and Essaouira sandstone calcarenite are the two lithological formations most found in the majority of ETUs, present in 1216 and 1270 ETUs, respectively. This is because, in the encrustation phenomena, coastal eolian constructions become dominant in the study area, as we mentioned in geological settings.
The most lithological formations occupied by the instabilities are dune sandstone with oblique stratification, friable sandstone layers, grey marls, heterogeneous conglomerate, limestone barre, marls, sequence of marls, and marly limestone.
Stratigraphic profiles (Figs. 6 and 7) show detailed lithological changes over the study area and allow for a better understanding of cliff lithological variations and the emplacement of friable layers that have a direct influence on the occurrence of landslides.
In the southern section, a large variation in the lithological units was noted with respect to the spatial distribution; therefore, the majority of stratigraphic logs were concentrated in the southern section (from log 1 to log 13), while there was little variation in the northern section (from log 14 to log 16). Regarding the lithological materials, the presence of friable or weak layers (friable sandstone, sand, clays, and marls) was noted in all logs except logs 3, 7, 9, 13, and 14.
As tilting layers are more favourable to instabilities because of the gravitational forces, the predominant sub-horizontal layering also has a contribution, while the majority of those layers are deposited on weak or friable layers, which stimulate the instability in multiple locations in the study area referring to the field survey. These friable layers are typically placed between the impermeable or competent layers, which are the result of either the different diagenesis degrees or compaction or of the high clay content according to grain size analysis, which makes them more friable than adjacent layers. According to the field survey, these layers are generally behind the occurrence of numerous landslides, which is why they are considered to be important, particularly because some of them are in contact with springs and others are in the bottom part of the cliff, which means more lithostatic pressure and thus more susceptibility to landslide occurrence.
Elevation is another important factor in landslide susceptibility mapping. Figure 7 shows the spatial distribution of these factors, and it can be seen that the southern-section cliffs present with higher elevation, because those areas are closer to the feet of the High Atlas mountains.
Other conditioning factors are provided by the fieldwork: (i) the presence and type of cliff toe protection, as shown in Fig. 8A–C (either rock platform, slope deposit, or beach protection); (ii) lithology tilting, which has a big impact on landslide occurrence, as shown in Fig. 8D and E; (iii) the presence of stream networks and springs in the cliff face, which stimulates landslide occurrence; and (iv) the presence of springs. Nine springs were localised, four of which are concentrated around Timzeguida Oufettas village, which has a locally visible impact on landslide occurrence, particularly considering the presence of marls, which slide more when in contact with water. The other springs are in the southern section, except for one in the north between Bhaybeh beach and Sidi Ishak village. They affect the mechanical processes that lead to slope failure and to the subsequent post-failure movements considerably, particularly in the case of marls or clays.
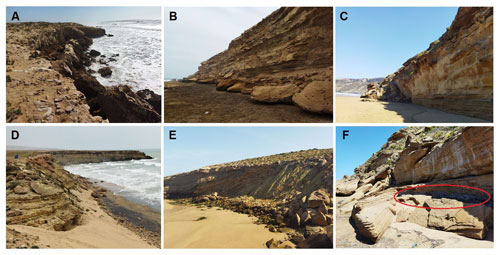
Figure 8Examples of some conditioning factors: (A) absence of toe protection, (B) rock platform protection, (C) beach protection, (D, E) tilted layers towards sea, and (F) cliff toe lithology effect.
For rainfall, the interpolation of rainfall records from 1968 to 2015 from four meteorological stations was used to assess the spatial distribution of this conditioning factor. The results showed that the maximum average of 306 mm of precipitation fell around Essaouira City, while the precipitation values decreased towards the two extremities of the study area, reaching a minimum average precipitation of 252 mm.
Finally, the NDVI and land-use map were prepared from the Sentinel satellite image analysis, and six land-use types were extracted, including bare ground, cultivated areas, light vegetation, dense vegetation, roads and habitation, and breakwater areas.
4.3 Coastal landslide susceptibility assessment
Coastal landslide susceptibility using the IV method, as mentioned in the objectives, was produced considering two different susceptibility zonation approaches: susceptibility assessed at the pixel scale and considering ETUs.
4.3.1 By pixel
Table S1 in the Supplement presents the IV scores obtained for each class of each landslide conditioning factor used in the construction of each susceptibility model for 15 landslide inventory partitions defined according to their classification into shallow and deep-seated landslides, landslide type, or type of affected material (debris or rock).
The IV scores represent a clear contrast between the most and least favourable areas for the occurrence of different landslide types, and we describe the most important conditioning factors for each landslide type:
-
For all landslide types (Model 1), the most relevant conditioning factor for the occurrence of all inventoried landslides is areas with slope angles > 45 (IV score = 1.377), followed by a solar radiation factor between 400 and 600 kWh m−2 (IV score = 1.332) and an elevation factor of 60–100 m (IV score = 1.320). The minimum value was obtained for the aspect class flat (IV score = −3.845). The results revealed, considering no differentiation in terms of landslide type or depth of the rupture surface, that slope angle and elevation are the most influential factors for landslide occurrence, particularly in dry climate areas such as the Essaouira coastal cliff area, except in the case of Model 10 (rock topple), in which the slopes > 15∘ have negative scores.
Deep-seated landslides (Model 2) in the Essaouira coastal area occurred more in areas with 400–600 kWh m−2 solar radiation (IV score = 1.536), in slope areas > 45∘ (IV score = 1.494), and in the high areas between 60 and 100 m (IV score = 1.480), where the minimum was in the same class as previous results. However, shallow mass movements occurred more in friable layers with an IV score of 3.011, in 600–700 kWh m−2 solar radiation (IV score = 2.072), and in areas with 35–45∘ slopes.
Rotational slides (Models 4, 5, and 6) generally occur in sandstone dolomites and dune sandstone with oblique stratification lithologies. For deep rotational slides, the grain size factor 38–51 (% sand) presented the highest value of 1.550, followed by slope angle factor class 30–40∘, with an IV score of 1.441. For shallow rotational slides, the grain size factor was strongly independent of the occurrence of this landslide type, with an IV score of 2.323.
-
Translational slides, deep translation slides, and shallow translational slides (Models 7, 8, and 9) in the Essaouira coastal area occur more in areas with 400–700 kWh m−2 solar radiation and in slope areas > 40∘.
-
In terms of rock topple (Model 10), the grain size factor – particularly classes 0 %–11 % silt (IV score = 2.092), 66 %–91 % sand (IV score = 2.037), and 0 %–7 % clay (IV score = 2.016) – contribute more to the occurrence of rock topples, because they typically occur next to friable layers in the Essaouira coastal cliff area.
-
Rock falls (Model 11) occur more in the “dune sandstone with oblique stratification” class of lithology factors, while the minimum IV value was −4.978 heterogeneous conglomerate, which is normal, as rock falls do not occur in this lithology type.
-
In terms of rock slides (Model 12), the lumachelic clayey limestone lithology class presented a strong dependence on rock slides, with an IV score of 3.253, while the flat (−1) areas for the aspect factor presented a minimum IV score of −3.960.
-
In terms of debris fall and flow (Models 13 and 14), the lithological material with grain size sand 51 %–66 % and silt 11 %–23 % are more favourable to the occurrence of debris fall and debris flow in the Essaouira coastal area, and the slope angle factor class 0–2∘ is less favourable, with an IV score of −4.822.
-
Debris slides (Model 15) presented a strong dependence on the terrigenous red deposit class lithology factor, while the minimum was an IV score of −3.565 for the flat (−1) class aspect factor, which is normal, because this landslide type occurs more in terrigenous lithologies and in slope areas.
To represent landslide susceptibility for each model, the final IV scores were reclassified into four classes: very low susceptibility (IV score < −1), low susceptibility (−1 < IV score < 0), moderate susceptibility (0 < IV score < 1), and high susceptibility (IV score > 1). Figure 9 presents the spatial distribution of susceptibility classes for pixel-based landslide susceptibility Model 1. It can be observed that a very low susceptibility class appeared more in the northern section of the study area, whereas the southern section presented higher susceptibility to the occurrence of landslides, particularly because of the weight of the translational and rotational slides in those areas.
The IV model allowed for the classification of 38 % of our study area as having high susceptibility to the occurrence of all landslide types, while the very-low-susceptibility class was present in 56 % of the study area (Table 5).
All other landslide type susceptibility models presented high percentages for the very-low-susceptibility class, with a maximum of 89.76 % for debris slides. The exception is for debris flow, where the highest percentage was for high susceptibility, with 53.85 % of the study area.
4.3.2 By ETUs
In general, the susceptibility assessment is conducted by classifying the ETUs into two classes: stabilised (37 % of ETUs) and non-stabilised (63 % of ETUs). The approach was performed individually for each type of landslide studied and shows that, for all landslide types, the unstable areas (classified as non-stabilised) are located more to the southern units of the study area.
To represent the ETU landslide susceptibility results, a zoomed section of the southern section of the study area next to Timzeguida Oufettas is presented (Fig. 10), from which landslide susceptibility zonation can be observed for the ETUs. This map presents the same allure or same variation as the susceptibility map produced by the pixel approach, except that, in the second ETU approach, ETU ID can be used to define the susceptible area in situ.
4.4 Validation of coastal landslide susceptibility models
All coastal landslide susceptibility models were validated by spatial confrontation, with independent landslide partitions defined as validating subsets. ROC curves (Linden, 2006; Remondo et al., 2003) (Table 6) of the predictive models were computed, and the respective AUC values were calculated. Table 6 shows the AUC values obtained in the validation process for all models. We can remark that all landslide susceptibility models presented AUC values > 0.7, and Models 1, 4, 10, 13, and 14 (0.7 to 0.8) were considered to be acceptable. Models 2, 5, 6, 7, 8, and 9 (0.8 to 0.9) were considered to be excellent, and Models 3, 11, 12, and 15 (>0.9) were considered to be outstanding.
For total landslides (Model 1) with all factors, 0.710 (Fig. 11) was obtained. Next, the topographic wetness factor and rainfall factors were eliminated due to the dry climate of the area; those factors did not present a strong dependence on the occurrence of landslides; a value of 0.798 (Fig. 11) was obtained, which means that the performance of Model 1 was improved in terms of prediction, particularly when low AUC values were obtained.
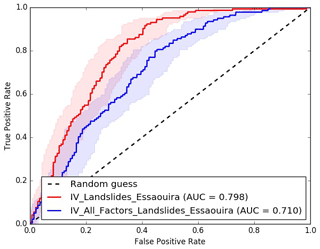
Figure 11ROC curves of the susceptibility model for all landslides with all factors (AUC = 0.710) and without TWI and rainfall factor (AUC = 0.798).
AUC graphs were plotted for translational slides (Model 7, 0.809; Fig. 12) and rotational slides (Model 4, 0.794; Fig. 13), as these two landslide types occupied approximately 85 % of the unstable area in the pixel model approach. These results show that susceptibility models have good predictive skill and highlight the higher performance of predictive models built for each type of landslide in comparison to models built for the total landslides.
The IV bivariate statistical approach to assess landslide susceptibility assessment in the 134 km of coastal area of Essaouira, based on geological and morphological analyses (interpretation of aerial photos, satellite images, and field survey), allowed for the classification of 38 % of the study area as having high susceptibility to landslide occurrence (using the pixel approach).
The translational slides followed by rotational slides occupied approximately 85 % of the landslide area, which can be explained by the fact that the conditioning factors that contribute more to the occurrence of those landslides – namely, >45∘ slope angle, 400–700 kWh m−2 solar radiation, and certain lithological formations – were all present in the study area, particularly the southern section. Another reason is that these landslide types typically occupy large areas.
Landslides are distributed along the entire study area, with a higher concentration in the southern section – mainly next to Sidi M'bark, Sidi Ahmed Essayeh, and in the northern sections of Tafedna and Timzguida Oufettas – because of its topographic characteristics, while the less susceptible areas are located in the middle and northern sections of the Essaouira coastal area.
For all landslide types, the most important explanatory drivers are slope factor (particularly >45∘), solar radiation factor class 400–600 kWh m−2, and elevation class 60–100 m. These factors have already been highlighted by multiple authors as being important conditioning factors for several landslide types. Most of the landslide susceptibility models (10 models out of 15) presented a strong interdependence with lithological factors or factors extracted from lithology, such as grain size and organic matter, which means that landslide occurrence is highly affected by lithological variations.
In the study area, precipitation was not present as a decisive conditioning factor as a consequence of the spatial distribution of rainfall, since the highest values were concentrated around Essaouira City, which is more related to sandy coast subsystems.
To define in detail the spatial distribution of the areas most susceptible to the different landslide types along the Essaouira coastal area, particularly in the southern section next to Timzeguida Oufettas village, more in-depth studies are recommended.
Both the pixel and ETU models maintain approximately the same value in all study areas. Based on these models, this study presents essential material for spatial planning and civil protection emergency actions in the Essaouira coastal area, particularly in the rocky coast subsystem.
Because ETUs are closer to the morphometry of the area, there is a more “guided” analysis in this approach compared to a pixel-based analysis that is not related to a particular morphology on the cliff area. Both approaches have advantages and are inconvenient to use. The ETU approach considers cliff morphometry more and is more useful for territorial management interventions; however, it also leads to a loss of susceptibility classification details compared to the pixel approach, which is more relevant in terms of resolution.
Universidade Aberta and Lisbon University have an institutional Esri account, and all the work done in ArcGis Pro is protected under that institutional licence.
The datasets generated during and/or analyzed during the current study are available from the corresponding author on reasonable request because the doctoral thesis is not finalized yet.
The supplement related to this article is available online at: https://doi.org/10.5194/nhess-22-3793-2022-supplement.
The main content was written by AK, who also carried out the sampling and analysis, collected the data, and prepared the figures. The landslide inventory is validated by JT, who also supervised the process and checked the modelling results. SO contributed to the conceptualization, and all authors reviewed and approved the final paper.
The contact author has declared that none of the authors has any competing interests.
Publisher's note: Copernicus Publications remains neutral with regard to jurisdictional claims in published maps and institutional affiliations.
The work of Jorge Trindade has been financed by national funds through FCT (Foundation for Science and Technology, I. P.), in the framework of the project “HighWaters – Assessing sea level rise exposure and social vulnerability scenarios for sustainable land use planning” (EXPL/GES-AMB/1246/2021). The work of Sérgio C. Oliveira has been financed by national funds through FCT (Foundation for Science and Technology, I. P.), in the framework of the project “BeSafeSlide – Landslide early warning soft technology prototype to improve community resilience and adaptation to environmental change” (PTDC/GES-AMB/30052/2017). The work of Ricardo Garcia has been funded by the Interreg Sudoe Programme through the European Regional Development Fund – RISKCoast Desarrollo de herramientas para prevenir y gestionar los riesgos en la costa ligados al cambio climático (SOE3/P4/E0868).
This paper was edited by Rachid Omira and reviewed by two anonymous referees.
Aleotti, P., Chowdhury, R.: Landslide hazard assessment: summary, review and new perspectives, Bull. Eng. Geol. Environ., 58, 21–44, 1999.
Andriani, G. F. and Walsh, N.: Rocky coast geomorphology and erosional processes: A case study along the Murgia coastline South of Bari, Apulia – SE Italy, Geomorphology, 87, 224–238, https://doi.org/10.1016/j.geomorph.2006.03.033, 2007.
Bahir, M., Mennani, A., Jalal, M., and Fakir, Y.: Impact de la sécheresse sur les potentialités hydriques de la nappe alimentant en eau potable la ville d'Essaouira (Mogador, Maroc), Sécheresse, 13, 13–9, 2002.
Bahir, M., Ouhamdouch, S., and Carreira, P. M.: Water resource in Morocco face the climatic changes; study case of the Plio-Quaternary phreatic aquifer at the synclinal basin of Essaouira, Comunicações Geológicas, 103, 35–44, 2017.
Balasubramani, K. and Kumaraswamy, K.: Application of geospatial technology and information value technique in landslide Hazard zonation mapping: a case study of Giri Valley, Himachal Pradesh, Disast. Adv., 6, 38–47, 2013.
Beguería, S.: Validation and evaluation of predictive models in hazard assessment and risk management, Nat. Hazards, 37, 315–329, 2006.
Calvello, M., Cascini, L., and Mastroianni, S.: Landslide zoning over large areas from a sample inventory by means of scale-dependent terrain units, Geomorphology, 182, 33–48, https://doi.org/10.1016/j.geomorph.2012.10.026, 2015.
Chamchati, H. and Bahir, M.: Potential Hydrogeological, Environment and Vulnerability to Pollution of the Plio-Quaternary Aquifers of the Coastal Basin of Essaouira (Morocco), J. Environ. Earth Sci., 3, 170–185, 2013.
Chen, W., Sun, Z., Zhao, X., Lei, X., Shirzadi, A., and Shahabi, H.: Performance evaluation and comparison of bivariate statistical-based artificial intelligence algorithms for spatial prediction of landslides, ISPRS Int. J. Geo-Inform., 9, 696, https://doi.org/10.3390/ijgi9120696, 2020.
Chimidi, G., Raghuvanshi, T. K., and Suryabhagavan, K. V.: Landslide hazard evaluation and zonation in and around Gimbi town, western Ethiopia – a GIS-based statistical approach, Appl. Geomat., 9, 219–236, 2017.
Chkir, N., Trabelsi, R., Bahir, M., Hadj Ammar, F., Zouari, K., Chamchati, H., and Monteiro, J. P.: Vulnérabilité des ressources en eaux des aquifères côtiers en zones semi-arides – Etude comparative entre les bassins d'Essaouira (Maroc) et de la Jeffara (Tunisie), Comunicações Geológicas, 95, 107–121, 2008.
Choubert, G. and Ambrogi, R.: Note préliminaire sur la présence de deux cycle sédimentaires dans le Pliocène marin au Maroc, N.M.S.G.M. t 7. no. 17., 3–72, 1953.
Choubert, G., Faure-Muret, A., and Hotinger, L.: La série stratigraphique de Tarfaya (Maroc sud-occidental) et le problème de la naissance de l'Océan Atlantique, N.M.S.G.M. 31, 29–40, http://pascal-francis.inist.fr/vibad/index.php?action=getRecordDetail&idt=PASCALGEODEBRGM73225666 (last access: 18 November 2022), 1971.
Cochet, A. and Combe, M.: Bassin d'Essaouira-Chichaoua et zone côtière d'Essaouira, Notes et Mémoires du Service Géologique no. 231, t. 2, 433–446, 1975.
Copernicus: Sentinel data [14/05/2021], ASF DAAC, https://scihub.copernicus.eu/, last access: 11 November 2021.
Corominas, J., Van Westen, C., Frattini, P., Cascini, L., Malet, J.-P., Fotopoulou, S., Catani, F., Van Den Eeckhaut, M., Mavrouli, O., Agliardi, F., Pitilakis, K., Winter, M. G., Pastor, M., Ferlisi, S., Tofani, V., Herva, J., and Smith, J. T.: Recommendations for the quantitative analysis of landslide risk, Bull. Eng. Geol. Environ., 73, 209–263, https://doi.org/10.1007/s10064-013-0538-8, 2014.
Cruden, D. M. and Varnes, D. J.: Landslide types and processes, in: Landslides: Investigation and Mitigation, Special Report 247, Transportation Research Board, Washington, 36–75, 1996.
Dai, A. and Lee, B.: Landslide characteristics and slope instability modeling using GIS, Lantau Island, Hong Kong, Geomorphology, 42, 213–228, 2002.
Dikau, R., Brunsden, D., Schrott, L., and Ibson, M. L.: Landslide Recognition: Identification, Movement and Causes, John Wiley & Sons, Chichester, UK, 1996.
Dufaud, F., Brun, L., and Planchut, B.: Le Basin du Sud-Ouest marocain, in Basin sédimentaires du Litoral africain, in: 1ère Partie, Littoral atlantique, Symposium de New-Delhi, 1964 de l'Asoc, des Services Géol. africains, 5–12, 1966.
Elkadiri, R., Sultan, M., Youssef, A., Elbayoumi, T., Chase, R., Bulkhi, A., and Al-Katheeri, M.: A remote sensing-based approach for debris-flow susceptibility assessment using artificial neural networks and logistic regression modeling. Selected topics in applied earth observations and remote sensing, IEEE J. Select. Top. Appl. Earth Obs. Remote Sens., 7, 4818–4835, https://doi.org/10.1109/JSTARS.2014.2337273, 2014.
El mimouni, A. and Daoudi, L.: Evolution à moyen terme du contexte hydrodynamique et morpho-sédimentaire la baie d'Essaouira (Maroc atlantique), XIIèmes Journées Nationales Génie Côtier – Génie Civil, https://doi.org/10.5150/jngcgc.2012.028-E, 2012.
Elmrabet, T., Levret, A., Ramdani, M., and Tadili, B.: Historical seismicity in Morocco: Methodological aspects and cases of multidisciplinary evaluation, Commissariat à l'Energie Atomique, Institut de protection et de sureté nucléaire, Département d'Analyse de sureté, https://inis.iaea.org/search/search.aspx?orig_q=RN:20068112 (last access: 18 November 2022), 1989.
Epifânio, B., Zêzere, J. L., and Neves, N.: Identification of hazardous zones combining cliff retreat with landslide susceptibility assessment, in: Proceedings 12th International Coastal Symposium (Plymouth, England), edited by: Conley, D. C., Masselink, G., Russel, P. E., and O'Hare, T. J., J. Coast. Res., 65, 1681–1686, 2013.
Epifânio, B., Zêzere, J. L., and Neves, M.: Susceptibility assessment to different types of landslides in the coastal cliffs of Lourinhã (Central Portugal), J. Sea Res., 93, 150–159, https://doi.org/10.1016/j.seares.2014.04.006, 2014.
Ercanoglu, M. and Gokceoglu, C.: Use of fuzzy relations to produce landslide susceptibility map of a landslide prone area (West Black Sea Region, Turkey), Eng. Geol., 75, 229–250, https://doi.org/10.1016/j.enggeo.2004.06.001, 2004.
Fekri, A.: Contribution à l'étude hydrogéologique et hydrogéochimique de la zone synclinale d'Essaouira (bassin synclinal d'Essaouira), Thèse de doctorat, Université Cadi Ayyad, Maroc, 161 pp., 1993.
Frattini, P., Crosta, G., and Carrara, A.: Techniques for evaluating the performance of landslide susceptibility models, Eng. Geol., 111, 62–72, 2010.
Gentile, W.: Caractérisation et suivi d'un champ dunaire par analyses sédimentologiques et télédétection (Essaouira-Cap Sim, Maroc Atlantique), Thèse Université de Province, Aix-Marseille I, p. 307, https://www.theses.fr/1997AIX10071 (last access: 18 November 2022), 1997.
Gilham, J. M.: Developing A Probabilistic Recession Model Through Characterisation and Quantification of the Erosion of Chalk Sea Cliffs in Brighton, Doctoral dissertation, University of Sussex, Sussex, https://core.ac.uk/download/pdf/159082376.pdf (last access: 18 November 2022), 2018.
Girma, F., Raghuvanshi, T. K., Ayenew, T., and Hailemariam, T.: Landslide hazard zonation in Ada Berga district, Central Ethiopia – a GIS based statistical approach, J. Geomat., 9, 25–38, 2015.
Gorsevski, P. V., Gessler, P. E., Foltz, R. B., and Elliot, W. J.: Spatial prediction of landslide hazard using logistic regression and ROC analysis, Trans. GIS, 10, 395–415, 2006.
Greenwood, R. O. and Orford, J. D.: Factors controlling the retreat of Drumlin coastal cliffs in a low energy marine environment – Strangford Lough, Northern Ireland, J. Coast. Res., 23, 285–297, 2007.
Halam, A.: Mesozoic geology and the opening of the North-Atlantic, J. Geol., 79, 129–157, 1971.
Hampton, M. A., Griggs, G. B., Edil, T. B., Guy, D. E., Kelley, J. T., Komar, P. D., Mickelson, D. M., and Shipman, H. M.: Processes that govern the formation and evolution of coastal cliffs, US Geological Survey professional paper 1693, US Geological Survey, 7–38, https://books.google.co.ma/books?id=UBF3EEMHhGgC (last access: 18 November 2022), 2004.
Hamza, T. and Raghuvanshi, T. K.: GIS based Landslide Hazard Evaluation and Zonation - A case from Jeldu District, Central Ethiopia, J. King Saud. Univ. Sci., 29, 151–65, 2017.
Hander, M.: Contribution à l'étude de la bioclimatologie humaine au Maroc: l'exemple d'Essaouira, Thèse de Doctorat, Paris-IV, p. 356, https://www.theses.fr/1993PA040211 (last access: 18 November 2022), 1993.
Heiri, O., Lotter, A. F., and Lemcke, G.: Loss on ignition as a method for estimating organic and carbonate content in sediments: reproducibility and comparability of results, J. Paleolimnol., 25, 101–110, 2001.
Hungr, O., Leroueil, S., and Picarelli, L.: The Varnes classification of landslide types, an update, Landslides, 11, 167–194, https://doi.org/10.1007/s10346-013-0436-y, 2014.
Jade, S. and Sarkar, S.: Statistical models for slope instability classification, Eng. Geol., 36, 91–98, 1993.
JAXA/METI: ALOS PALSAR [ALPSRP074670620 and ALPSRP074670610] [19/06/2007], ASF DAAC, https://asf.alaska.edu, last access: 20 July 2020.
Kanungo, D. P., Arora, M. K., Sarkar, S., and Gupta, R. P.: A comparative study of conventional, ANN black box, fuzzy and combined neural and fuzzy weighting procedures for landslide susceptibility zonation in Darjeeling Himalayas, Eng. Geol., 85, 347–366, 2006.
Lan, H. X., Zhou, C. H., Wang, L. J., Zhang, H. Y., and Li, R. H.: Landslide hazard spatial analysis and prediction using GIS in the Xiaojiang watershed, Yunnan, China, Eng. Geol., 76, 109–128, 2004.
Lee, S. and Pradhan, B.: Landslide hazard mapping at selangor, malaysia using frequency ratio and logistic regression models, Landslides, 4, 33–41, https://doi.org/10.1007/s10346-006-0047-y, 2007.
Le Pichon, X.: La genèse de l'Atlantique Nord, La Recherche, p. 21, 1971.
Letortu, P., Costa, S., Maquaire, O., and Davidson, R.: Marine and subaerial controls of coastal chalk cliff erosion in Normandy (France) based on a 7-year laser scanner monitoring, Geomorphology, 335, 76–91, 2019.
Lharti, S., Flor, G., Daoudi, L., Flor, G. B., El mimouni, A., and Ben Ali, A.: Morfologia y Sedimentologia del complejo playa/dunas costeras de Essaouira (Marruecos atlantico): modelo de transporte costero, in: Actas de la IX Reunión Nacional de Geomorfología, edited by: Pérez Alberti, A. and López Bedoya, J., Santiago de Compostela, 401–417, https://dialnet.unirioja.es/servlet/articulo?codigo=2193466 (last access: 18 November 2022), 2006.
Lin, M. L. and Tung, C. C.: A GIS-based potential analysis of the Landslides induced by the chi-chi earthquake, Eng. Geol., 71, 63–77, 2003.
Linden, A.: Measuring diagnostic and predictive accuracy in disease management: an introduction to receiver operating characteristic (ROC) analysis, J. Eval. Clin. Pract., 12, 132–139, 2006.
Mancini, F., Ceppi, C., and Ritrovato, G.: GIS and statistical analysis for landslide susceptibility mapping in the Daunia area, Italy, Nat. Hazards Earth Syst. Sci., 10, 1851–1864, https://doi.org/10.5194/nhess-10-1851-2010, 2010.
Marques, F.: Sea cliff instability hazard prevention and planning: examples of practice in Portugal, J. Coast. Res., 56, 856–860, 2009.
Marques, F.: Regional scale sea cliff hazard assessment at Sintra and Cascais counties, western coast of Portugal, Geosciences, 8, 80, https://doi.org/10.3390/geosciences8030080, 2018.
Marques, F., Matildes, R., and Redweik, P.: Statistically based sea cliff instability hazard assessment of Burgau-Lagos coastal section (Algarve, Portugal), Proceeding of the 11th International Coastal Symposium, J. Coast. Res., 64, 927—31, 2011.
Marques, R. T. F.: Estudo de movimentos de vertente no concelho da Povoação (ilha de São Miguel, Açores): Inventariação, caracterização e análise da susceptibilidade, PhD Thesis, Geological Risks, University of Azores, Ponta Delgada, Portugal, http://hdl.handle.net/10400.3/2958 (last access: 18 November 2022), 2013.
Mateus, L. R., Frederico, G. S., and Cesar, F., Barella: Landslide susceptibility mapping using the statistical method of Information Value: A study case in Ribeirão dos Macacos basin, Minas Gerais, Brazil, Annals of the Brazilian Academy of Sciences, An. Acad. Bras. Cienc., 93, e20180897, https://doi.org/10.1590/0001-3765202120180897, 2021.
Meena, S. R., Ghorbanzadeh, O., and Blaschke, T.: A Comparative Study of Statistics-Based Landslide Susceptibility Models: A Case Study of the Region Affected by the Gorkha Earthquake in Nepal, ISPRS Int. J. Geo-Inform., 8, 94, https://doi.org/10.3390/ijgi8020094, 2019.
Mengistu, F., Suryabhagavan, K. V., Raghuvanshi, T. K., and Lewi, E.: Landslide Hazard zonation and slope instability assessment using optical and InSAR data: a case study from Gidole town and its surrounding areas, southern Ethiopia, Remote Sens. Land., 3, :1–14, 2019.
Mennani, A.: Apports de l'hydrochimie et de l'isotopie à la connaissance du fonctionnement des aquifères de la zone côtière d'Essaouira. Thèse de doctorat, Université Cadi Ayyad, Maroc, 152 pp., 2001.
Michard, A., Westphal, M., Bosert, A., and Hamzeh, R.: Tectonique de blocs dans le socle atlaso-mésétien du Maroc: une nouvelle interprétation des données géologiques et paléomagnétiques, Earth Planet. Sc. Lett., 24, 363–368, https://doi.org/10.1016/0012-821X(75)90142-9, 1975.
Moore, L. J. and Griggs, G. B.: Long-term cliff retreat and erosion hotspots along the central shores of the monterey bay national marine sanctuary, Mar. Geol., 181, 265–283, 2002.
Moore, R. and Davis, G.: Cliff instability and erosion management in England and Wales, J. Coast. Conserv., 19, 771–784, 2015.
Neves, M. and Ramos-Pereira, A.: The interaction between marine and sub-aerial processes in the evolution of rocky coasts: the example of Castelejo (southwest Portugal), Bol. Inst. Esp. Oceanogr., 15, 251–258, 1999.
Neves, M., Zêzere, J. L., Henriques, C., Garcia, R., Oliveira, S., and Piedade, A.: Modeling the long term evolution of rocky coasts in central Portugal. Avances de la Geomorfología en España, Actas de la XII Reunión Nacional de Geomorfología, 73–76, https://www.researchgate.net/profile/Jose-Zezere/publication/285738847_Modelling_the_long_term_ (last access: 18 November 2022), 2012.
Oliveira, S. C., Catalão, J., Ferreira, Ó., and Alveirinho D. J.: Evaluation of cliff retreat and beach nourishment in southern Portugal using photogrammetric techniques, J. Coast. Res., 24, 184–193, https://doi.org/10.2112/06-0781.1, 2008.
Oliveira, S. C., Zêzere, J. L., Lajas, S., and Melo, R.: Combination of statistical and physically based methods to assess shallow slide susceptibility at the basin scale, Nat. Hazards Earth Syst. Sci., 17, 1091–1109, https://doi.org/10.5194/nhess-17-1091-2017, 2017.
Pawluszek, K.: Landslide features identifcation and morphology investigation using high-resolution DEM derivatives, Nat. Hazards, 96, 311–330, https://doi.org/10.1007/s11069-018-3543-1, 2019.
Pereira, S., Santos, P. P., Zêzere, J. L., Tavares, A. O., Garcia, R. A. C., and Oliveira, S. C.: A landslide risk index for municipal land use planning in Portugal, Sci. Total Environ., 735, 139463, https://doi.org/10.1016/j.scitotenv.2020.139463, 2020.
Petley, D. N.: The global occurrence of fatal landslides in 2007, in: Geophysical Research Abstracts, vol. 10, EGU General Assembly, p. 3, 2008.
Queiroz, S. M. and Marques, F. M.: Sea cliff instability susceptibility considering nearby human occupation and predictive capacity assessment, Eng. Geol., 253, 75–93, 2019.
Reichenbach, P., Rossi, M., Malamud, B. D., Mihir, M., and Guzzetti, F.: A review of statistically-based landslide susceptibility models, Earth-Sci. Rev., 180, 60–91, https://doi.org/10.1016/j.earscirev.2018.03.001, 2018.
Remondo, J., González, A., Díaz De Terán, J. R., Cendrero, A., Fabbri, A., and Chung, C. J. F.: Validation of Landslide Susceptibility Maps; Examples and Applications from a Case Study in Northern Spain, Nat. Hazards, 30, 437–449, 2003.
Rocha, J., Ferreira, J. C., Simões, J., and Tenedório, J. A.: Modelling coastal and land use evolution patterns through neural network and cellular automata integration, J. Coas. Res., 50, 827–831, 2007.
Saadi, M.: Relations des alignements structuraux au Maroc avec différents phénomènes géologiques et leur contribution à la compréhension de l'évolution structurale du pays, N.M.S.G.M. 236, 13–18, 1972.
Shano, L., Raghuvanshi, T. K., and Meten, M.: Landslide susceptibility evaluation and hazard zonation techniques – a review, geoenvironmental-disasters, Springer Open, https://doi.org/10.1186/s40677-020-00152-0, 2020.
Simon, C.: Le géo-système dunaire anthropisé d'Essaouira – est (Maroc Atlantique) dynamique et paléo-environnements, Thèse de doctorat, Univ. Aix Marseille I, p. 204, https://tel.archives-ouvertes.fr/tel-00171576/ (last access: 18 November 2022), 2000.
Smaij, Z.: Typologie de la qualité des ressources en eaux du bassin de Tensift Al-Haouz et cadre juridique de protection et de préservation, Université Cadi Ayyad Faculté des sciences et techniques, Marrakech, p. 96, 2011.
Sunamura, T.: Geomorphology of rocky coasts, Wiley, Chichester, 302 pp., 1992.
Sunamura, T.: Rocky coast processes: with special reference to the recession of soft rock cliffs, The Japan Academy, https://doi.org/10.2183/pjab.91.481 2015.
Teixeira, M.: Movimentos de Vertente: Factores de Ocorrência e Metodologia de Inventariação, Geonovas, 20, 12, 2006.
Teixeira, M., Bateira, C., Marques, F., and Vieira, B.: Physically based shallow translational landslide susceptibility analysis in Tibo catchment, NW of Portugal, Landslides, 12, 455–468, 2015.
Trenhaile, A. S.: The Geomorphology of Rock Coasts, Oxford University Press, Oxford, p. 384, 1987.
USGS-EROS: USGS EROS Archive – Aerial Photography – High Resolution Or thoimagery (HRO), https://earthexplorer.usgs.gov/ (last access: July 2021), 2018.
Van Den Eeckhaut, M., Reichenbach, P., Guzzetti, F., Rossi, M., and Poesen, J.: Combined landslide inventory and susceptibility assessment based on different mapping units: an example from the Flemish Ardennes, Belgium, Nat. Hazards Earth Syst. Sci., 9, 507–521, https://doi.org/10.5194/nhess-9-507-2009, 2009.
Van Westen, C. J., Rengers, N., Terlien, M. T. J., and Soeters, R.: Prediction of the occurrence of slope instability phenomenal through GIS-based hazard zonation, Geol. Rundsch., 86, 404–414, 1997.
Van Westen, C. J., van Asch, T. W. J., and Soeters, R.: Landslide hazard and risk zonation – why is it still so difficult?, Bull. Eng. Geol. Environ., 65, 167–184, 2006.
Van Westen, C. J., Castellanos, E., and Kuriakose, S. L.: Spatial data for landslide susceptibility, hazard, and vulnerability assessment: an overview, Eng. Geol., 102, 112–131, 2008.
Varnes, D. J.: Slope movement types and processes, in: Special Report 176: Landslides: Analysis and Control, edited by: Schuster, R. L. and Krizek, R. J., Transportation and Road Research Board, National Academy of Science, Washington, DC, 11–33, https://www.researchgate.net/profile/Ahmad-Solgi/post/slope_classification_by_mechanism_of_failure/attachment/5be05c69cfe4a76455ffdc61/AS:689623139381250@1541430377371/download/3.pdf (last access: 18 November 2022), 1978.
Varnes, D. J.: International Association of Engineering Geology Commission on Landslides and Other Mass Movements on Slopes, Landslide Hazard Zonation: A Review of Principles and Practice, UNESCO, Paris, 1984.
Varnes, D. J.: Landslide types and processes, in: Landslides: investigation and mitigation, special report 247, edited by: Turner, A. K. and Schuster, R. L., Transportation Research Board, National Academy Press, National Research Council, Washington, DC, 1996.
Violante, C.: Rocky coast: geological constraints for hazard assessment, Geol. Soc. Lond. Spec. Publ., 322, 1–31,, https://doi.org/10.1144/SP322.1, 2009.
Wang, L.-J., Guo, M., Sawada, K., Lin, J., and Zhang, J.: A comparative study of landslide susceptibility maps using logistic regression, frequency ratio, decision tree, weights of evidence and artificial neural network, Geosci. J., 20, 117–136, https://doi.org/10.1007/s12303-015-0026-1, 2016.
Weisrock, A.: Géomorphologie et paléo-environnements de l'Atlas atlantique (Maroc), Thèse d'état, Paris I, p. 931, 1980.
WP/WLI: International Geotechnical Societies' UNESCO (Working Party on World Landslide Inventory), Multilingual Landslide Glossary, Bitech Publisher, Richmond, British Columbia, Canada, 1993.
Yalcin, A.: GIS-based landslide susceptibility mapping using analytical hierarchy process and bivariate statistics in Ardesen (Turkey): comparisons of results and confirmations, Catena, 72, 1–12, 2008.
Yin, K. L. and Yan, T. Z.: Statistical prediction models for slope instability of metamorphosed rocks, in: Proceedings of the 5th International Symposium on Landslides, Lausanne, Switzerland, 1269–1272, http://pascal-francis.inist.fr/vibad/index.php?action=getRecordDetail&idt=7267609 (last access: 18 November 2022), 1988.
Zêzere, J. L.: Landslide susceptibility assessment considering landslide typology. A case study in the area north of Lisbon (Portugal), Nat. Hazards Earth Syst. Sci., 2, 73–82, https://doi.org/10.5194/nhess-2-73-2002, 2002.
Zêzere, J. L., Reis, E., Garcia, R., Oliveira, S., Rodrigues, M. L., Vieira, G., and Ferreira, A. B.: Integration of spatial and temporal data for the definition of different landslide hazard scenarios in the area north of Lisbon (Portugal), Nat. Hazards Earth Syst. Sci., 4, 133–146, https://doi.org/10.5194/nhess-4-133-2004, 2004.
Zêzere, J. L., Pereira, S., Melo, R., Oliveira, S. C., and Garcia, R. A. C.: Mapping landslide susceptibility using data-driven methods, Sci. Total Environ., 589, 250–267, 2017.