the Creative Commons Attribution 4.0 License.
the Creative Commons Attribution 4.0 License.
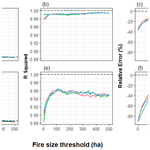
Assessing the accuracy of remotely sensed fire datasets across the southwestern Mediterranean Basin
Luiz Felipe Galizia
Thomas Curt
Renaud Barbero
Marcos Rodrigues
Recently, many remote-sensing datasets providing features of individual fire events from gridded global burned area products have been released. Although very promising, these datasets still lack a quantitative estimate of their accuracy with respect to historical ground-based fire datasets. Here, we compared three state-of-the-art remote-sensing datasets (RSDs; Fire Atlas, FRY, and GlobFire) with a harmonized ground-based dataset (GBD) compiled by fire agencies monitoring systems across the southwestern Mediterranean Basin (2005–2015). We assessed the agreement between the RSDs and the GBD with respect to both burned area (BA) and number of fires (NF). RSDs and the GBD were aggregated at monthly and 0.25∘ resolutions, considering different individual fire size thresholds ranging from 1 to 500 ha. Our results show that all datasets were highly correlated in terms of monthly BA and NF, but RSDs severely underestimated both (by 38 % and 96 %, respectively) when considering all fires > 1 ha. The agreement between RSDs and the GBD was strongly dependent on individual fire size and strengthened when increasing the fire size threshold, with fires > 100 ha denoting a higher correlation and much lower error (BA 10 %; NF 35 %). The agreement was also higher during the warm season (May to October) in particular across the regions with greater fire activity such as the northern Iberian Peninsula. The Fire Atlas displayed a slightly better performance with a lower relative error, although uncertainty in the gridded BA product largely outpaced uncertainties across the RSDs. Overall, our findings suggest a reasonable agreement between RSDs and the GBD for fires larger than 100 ha, but care is needed when examining smaller fires at regional scales.
- Article
(5967 KB) - Full-text XML
-
Supplement
(410 KB) - BibTeX
- EndNote
Vegetation fires are a common and destructive hazard in the southwestern Mediterranean Basin. Over the past 4 decades, there were, on average, 47 766 fires and 413 209 ha burned annually in this region (San-Miguel-Ayanz et al., 2017) causing extensive economic and ecological losses and even human casualties (Keeley et al., 2011; Molina-Terrén et al., 2019). Fire is a complex phenomenon due to the confluence of several factors, including climate, weather, human activities, and vegetation (Bowman et al., 2009). The Mediterranean fire regime is dominated by human-caused ignitions (Ganteaume et al., 2013), with most of the total burned area (BA) linked to a limited number of large fires during the summer (Turco et al., 2016). These large fire events are facilitated by dry conditions and high temperatures, which are both expected to increase in the future under climate change (Dupuy et al., 2020; Ruffault et al., 2020; Turco et al., 2018a). Additional factors such as landscape changes, as well as changes in forest and fire management, may also shape future fire activity (Moreira et al., 2020; Pausas and Fernández-Muñoz, 2012). Projecting future changes in fire activity requires modeling efforts across broad geographical scales to better understand processes and mechanisms conducive to fire ignition and spread. However, one of the main limitations in fire modeling lies in the lack of reliable and homogeneous information on fire activity across space (Hantson et al., 2016; Williams and Abatzoglou, 2016). This is particularly true in Europe where the lack of data sharing, as well as the lack of consistent quality-control procedures of national ground-based fire datasets, has hampered the analysis of fire regimes across broader regional or continental scales (Mouillot and Field, 2005; Turco et al., 2016). To overcome this limitation, the European Forest Fire Information System (EFFIS; San-Miguel-Ayanz et al., 2015) is increasingly using remote-sensing techniques for monitoring fire activity across Europe.
In the last decade, remote sensing has contributed to fostering fire-related products with spatial and temporal consistency and global coverage (Chuvieco et al., 2019; Mouillot et al., 2014). The MODIS sensor stands out as one of the best data providers for most burned area products such as MCD64A1 (Giglio et al., 2018) and FireCCI50 (Chuvieco et al., 2018). In particular, the latest generation of BA products, the MCD64A1v006, sets the basis for an exhaustive global estimation of fire-related carbon emissions, which is compiled in the GFED4 database (Giglio et al., 2013; Randerson et al., 2015; van der Werf et al., 2017). Although BA products typically offer information about the pixels that burned in a given day, they do not provide information such as starting/ending dates or the final extent of individual fire events (Mouillot et al., 2014). This limitation has hampered efforts to distinguish fire regimes dominated by different fire sizes as both small but frequent fires and large but rare fires may contribute equally to total burned area.
In this sense, global datasets of individual fires derived from pixel-level BA information have recently emerged as an important resource for the fire community in improving our understanding of fire regimes (Andela et al., 2019b; Artés et al., 2019; Laurent et al., 2018a). Unlike raw BA products, remote-sensing datasets of individual fires provide information beyond the BA, such as fire shape, daily rate of spread, and the number of fires (NF). The Fire Atlas (Andela et al., 2019a, b), FRY (Laurent et al., 2018a, b), and GlobFire (Artés et al., 2019; Artés Vivancos and San-Miguel-Ayanz, 2018) represent the most recent individualized fire datasets. These datasets were built from specific algorithms to reconstruct fire patches from MCD64A1 pixel-based BAs. In spite of using different methodologies and different assumptions, these datasets share a common objective: to aggregate neighboring burned pixels with sequential burn dates into individual fire patches.
Although very promising, remote-sensing datasets of individual fires have been sparingly compared to historical ground-based fire databases that are generally thought to be the most reliable source of data regarding fire occurrence and fire extent (Moreira et al., 2011; Mouillot et al., 2014). Previous studies indicated that a rigorous evaluation of satellite data with ground-based data is needed (Turco et al., 2019). Most validation procedures of these remote-sensing datasets were based on comparisons between different satellite products (Andela et al., 2019b; Laurent et al., 2018a) with, however, scarce attention paid to independent ground-based observations (Artés et al., 2019).
In this work, we compared for the first time the three most recent remote-sensing datasets of individual fires (Fire Atlas, FRY, and GlobFire) with quality-controlled fire databases compiled by regional agencies across the most active fire region in Europe (i.e., southwestern Mediterranean Basin) during the common period of observations (2005 to 2015). While most previous studies have evaluated remote-sensing data on a fire-by-fire basis, this study aggregates individual fires across months and pixels (0.25∘) and seeks to estimate to what extent the temporal variability in both fire frequency and burned area are captured by remote-sensing datasets. We sought to provide a solid answer to the following questions. (i) Are remote-sensing datasets capturing the actual pattern of fire occurrence and burned area? (ii) To what extent is their accuracy dependent on fire size? To answer these questions, we examined the agreement between remotely sensed and ground-based fire datasets aggregated at monthly and 0.25∘ resolutions across a range of individual fire size thresholds (1 to 500 ha). This study may inform end users about remote-sensing datasets' ability to proxy actual fire activity but also inform them of their limitations.
2.1 Ground-based fire data
The ground-based dataset (GBD) was built from multiple fire agency sources, including fire records from Portugal, Spain, France, and Sardinia in Italy (Table 1). All these ground monitoring systems provide high-quality datasets that have been extensively used in previous studies across France (Curt et al., 2014), Portugal (Pereira et al., 2011), Sardinia (Salis et al., 2013), and the Mediterranean basin (Rodrigues et al., 2020; Turco et al., 2016). Although not free of errors, these datasets constitute the most accurate source of historical information about fires available across the region.
Table 1Fire agencies and reference links to the data used to build the harmonized ground-based dataset (GBD) across the southwest Mediterranean Basin.
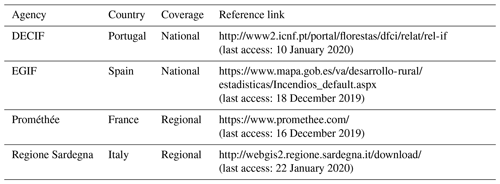
We extracted the following information from each regional dataset: the day of ignition, the fire size, and the location of each fire. To ensure consistency across regions and scales, we analyzed the overlapping recording period among the datasets, i.e., 2005–2015. Small fires (< 1 ha) were discarded to ensure the coherence of the analysis since these were not reported systematically by agencies over the studied period. The harmonized dataset contained 95 561 fire records, including only events that required a firefighting response (i.e., disregarding agricultural and prescribed fires) (see Fig. 1).
2.2 Remotely sensed fire data
We used the most recent global remote-sensing datasets (RSDs) of individual fires: Fire Atlas (Andela et al., 2019a, b), FRY (Laurent et al., 2018a, b), and GlobFire (Artés et al., 2019; Artés Vivancos and San-Miguel-Ayanz, 2018). These datasets provide the date and the spatial extent of individual fires from the pixel-based burned area MODIS product MCD64A1 Collection 6 (Table 2). The combined Terra and Aqua MCD64A1 is derived from the surface reflectance imagery and active fire observations. It provides a global coverage of burned area estimation at a resolution of 500 m (Giglio et al., 2018). Fires were individualized from different algorithms, such as a progression-based algorithm (Andela et al., 2019b), a flood-fill algorithm (Laurent et al., 2018a), and data mining (Artés et al., 2019), which share a common objective: to assemble burned pixels that were adjacent in both space and time to identify and outline individual fire events. All RSDs provide fire start and end dates, location, and the final burned area for each retrieved fire event.
Table 2Description of the remote-sensing datasets (RSDs) of individual fires, including the digital object identifier (DOI) and reference of each dataset. FA signifies Fire Atlas, FRY_M05 signifies FRY MODIS (5 d), and GF signifies GlobFire.

A key parameter of these algorithms is the cutoff value, which is defined as the maximum burn date difference allowed between two neighboring pixels to be considered as belonging to the same fire event. This cutoff influences the size, shape, and degree of clumpiness and fragmentation of individual fire events (Laurent et al., 2018a; Oom et al., 2016). Fire Atlas used spatially varying cutoff thresholds (4 to 10 d) depending on the fire frequency (Andela et al., 2019b), while the FRY algorithm processed four different cutoff scenarios (3, 5, 9, and 14 d) used in previous studies (Archibald and Roy, 2009; Hantson et al., 2015; Nogueira et al., 2017). Finally, GlobFire defined a fire event as a set of burned pixels that are connected within a 5 d window and that have not been burned over the 16 previous days (Artés et al., 2019). For simplicity, we only reported the FRY cutoff value that performed the best (5 d). The comparison with all FRY cutoff values is available in Appendix A (Fig. A1).
2.3 Methodology
We compared burned area (BA) and number of fires (NF) estimated by RSDs with the ground-based reference dataset (GBD; Fig. 2). Only the common period between RSD and GBD records (2005–2015) has been considered. We evaluated the ability of RSDs to reproduce the temporal and spatial patterns of fire activity observed in the GBD by fitting ordinary least squares (OLS) linear regressions and using different metrics (OLS slope, R2 correlation, and relative error). We calculated the relative error (ε) as follows:
where BARSD represents the BA detected by remote-sensing datasets and BAGBD represents the BA registered in the ground-based dataset over the study period. The analysis was repeated for the number of fires (NF).
We applied a land cover filter to the RSD data using CORINE Land Cover (CLC) to exclude fires located within agricultural or artificial lands that are not always reported by fire agencies. To account for the land cover changes over the study period, we used CLC 2006 as a reference to filter RSDs from the 2005 and 2009 period and CLC 2012 from 2010 and 2015. A sensitivity analysis to the land cover filter is shown in Appendix A (Fig. A2).
As RSDs are prone to omit smaller fires (< 25 ha) due to the coarse spatial resolution of the MODIS product MCD64A1 (500 m) and other limitations, we investigated different fire size thresholds increasing from 1 to 500 ha. Analyses were repeated for each size-filtered sample (i.e., excluding fires smaller than a given threshold).
2.3.1 Temporal agreement
All datasets were aggregated to a monthly scale over the whole study area. We retrieved the slope coefficient of OLS regressions and the coefficient of determination (R2) as a proxy of agreement between RSDs and the GBD. Slope values greater than 1 indicated an underestimation of fire activity as seen by the GBD and vice versa. A slope equal to 1 would imply a perfect agreement.
2.3.2 Spatial agreement
We then sought to examine how the agreement between RSDs and the GBD varies across space. There is much uncertainty in estimating the ignition point from satellite data mainly due to the spatial and temporal proximity of fire pixels and the possibility of multiple ignition points in a single fire event (Benali et al., 2016). Likewise, the GBD do not systematically provide ignition points. Thus, to overcome this limitation, we aggregated both the RSDs and the GBD onto a 0.25∘ grid (≈ 25 km), setting a common ground for both datasets.
We calculated the relative error (Eq. 1) between the RSDs and the GBD for each grid cell. Finally, we estimated the overall spatial error, computed as the ε averaged across all grid cells for each RSD.
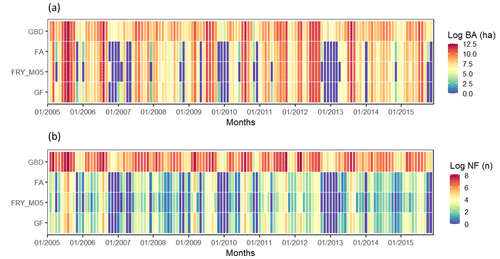
Figure 3(a) Monthly burned area and (b) number of fires (> 1 ha) in each fire dataset across the southwestern Mediterranean Basin over 2005–2015.
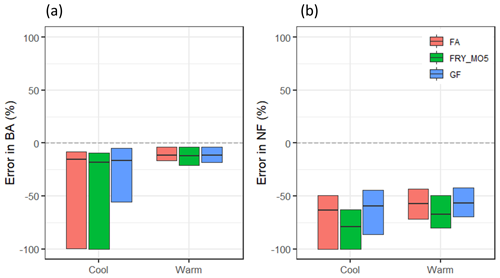
Figure 4(a) Median and interquartile range of the seasonal error (ε) observed each year for burned area and (b) number of fires in each RSD for all fires > 1 ha in the studied area. Cool season from November to April and warm season from May to October. Dashed lines represent the perfect agreement between the datasets.
3.1 Temporal agreement
We first analyzed the monthly distributions of BA and NF for all fires (> 1 ha) aggregated across the whole studied area. Figure 3 shows that RSDs follow a similar variability in terms of monthly BA but systematically underestimate BA and NF with respect to the GBD. The best agreement between RSDs and the GBD occurs mainly during the warm season (May to October; see Fig. 4). This is usually the period experiencing the largest fires which account for the bulk of the BA in the region (Turco et al., 2016). Conversely, the poorest agreement was found during the cool season (November to April), a period dominated mainly by small fires linked to agricultural activities.
Table 3Correlation between RSDs and the GBD of monthly and annual burned area and number of fires for all fires (> 1 ha) between 2005 and 2015.
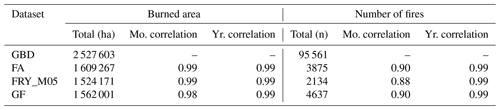
Table 3 presents the total BA and NF, as well as the monthly (i.e., including the seasonal cycle) and annual (i.e., excluding the seasonal cycle) correlation between RSDs and the GBD for all fires (> 1 ha). Monthly correlations showed a stronger agreement for BA (R2≈0.98) than for NF (R2≈0.89). Annual correlations, for which the effect of the seasonal cycle was removed, also showed very high values (R2≈0.99). Despite the fact that RSDs underestimated the total BA by 38 % and the NF by 96 % for all fires, they reproduced almost perfectly the temporal variability in both monthly and annual bases. The difference in absolute numbers thus relates to undetected small fires in RSDs.
The monthly agreement of BA and NF (Fig. 5) strongly varies with fire size thresholds (1, 50, 100, and 500 ha). The positive slope of the linear trends indicates that RSDs generally underestimate both BA and NF when accounting for all fires (> 1 ha). However, they become progressively more accurate as the fire size threshold increases, a feature that is particularly evident in NF estimates (Fig. 5e–h).
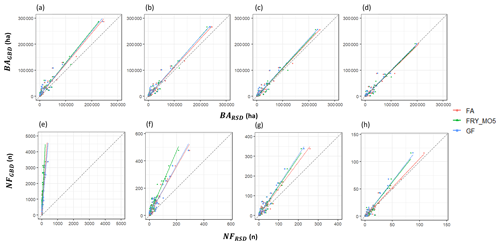
Figure 5Comparison of the GBD and RSDs with respect to monthly burned area (a–d) and the number of fires (e–h) when considering (a) all fires (> 1 ha), (b) fires > 50 ha, (c) fires > 100 ha, and (d) fires > 500 ha. (e–h) Same as (a–d) but for the number of fires. The 1:1 dashed lines represent the perfect fit between the datasets.
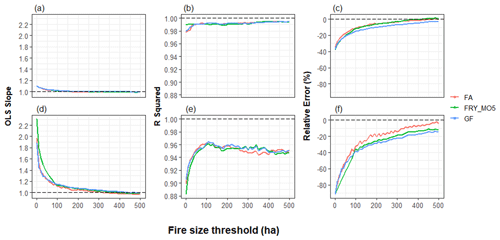
Figure 6Evaluation of RSDs through different metrics including the slope (a, d), R2 correlation (b, e), and relative error (c, f) for both burned area (a–c) and the number of fires (d–f) over a range of individual fire size thresholds (1 to 500 ha). Dashed lines indicate a perfect fit between RSDs and the GBD.
Figure 6 shows the evaluation of RSDs through different metrics over the continuum of fire size thresholds. Except for R2 (Fig. 6e) which saturates for fires > 100 ha for NF, all metrics present a similar behavior, showing better agreement when increasing the fire size threshold. Overall, BA (Fig. 6a, b, and c) is better estimated than NF (Fig. 6d, e, and f). Despite the different methodologies used to reconstruct individual fires, all datasets showed similar scores, although Fire Atlas (FA) displayed lower relative error (ε) for NF.
3.2 Spatial agreement
Figure 7 shows the spatial distribution of the relative error (ε) for BA over different individual fire size thresholds (for all fire size thresholds see Supplement). As expected from previous results, RSDs strongly underestimated BA, especially when including smaller fires. However, a few exceptions are seen for fires < 50 ha mainly over eastern Spain, suggesting that RSDs detect in that case more fires than the GBD. This may be related to a few small prescribed fires that were not reported in the GBD. Also, we found much lower ε in regions with higher fire activity, such as the northern Iberian Peninsula. This is rather expected as an absolute change in regions with a high (low) baseline will result in a small (large) percentage change.
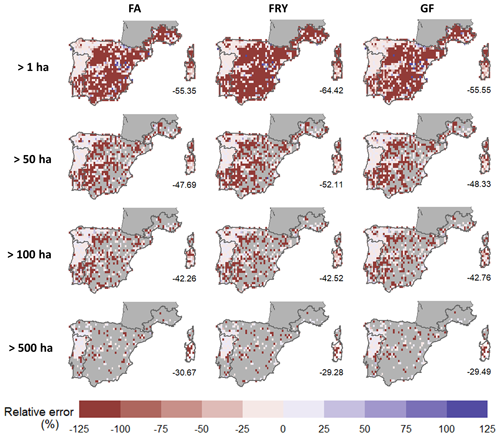
Figure 7The relative error (ε) of the total burned area computed as the relative difference between RSDs and GBD data over different individual fire size thresholds (1, 50, 100, and 500 ha). The overall ε is indicated on each map.
Likewise, RSDs strongly underestimated NF (Fig. 8), likely disregarding those smaller fires not detected by MODIS. Surprisingly, a few areas showed positive differences in NF for fires > 100 ha across parts of Spain. This overestimation of large fires may be related to the fact that RSD algorithms are likely to split larger fires into multiple events. Nevertheless, the overall relative error between RSDs and the GBD decreases when focusing on larger fires for both NF and BA.
Understanding global changes in fire activity calls for efficient and harmonized approaches to record fire activity. Satellite-borne spectral and thermal sensors offer several global fire products, evolving from BA mapping and active fire detection to novel developments postprocessing BA products into single fire datasets (Chuvieco et al., 2019). The ongoing challenge lies in determining their reliability and usefulness. Here, we compared RSDs with the GBD across the southwestern Mediterranean Basin to better understand RSD limitations and to guide end users.
Although RSDs may miss a substantial number of fires, the temporal variations in both NF and BA match very well with ground-based observations. Our results also demonstrate that the agreement between RSDs and the GBD is strongly dependent on individual fire size. Focusing on larger fires (fire typically > 100 ha), RSDs were in stronger agreement with the GBD regardless of the evaluated metrics. Fires >100 ha had a much lower error (BA 10 %; NF 35 %), especially in regions with higher fire activity such as the northwest of the Iberian Peninsula or southern Sardinia. Our findings are in agreement with previous studies which pointed at fire size as the primary limiting factor for remotely sensed fire data (Campagnolo et al., 2021; Rodrigues et al., 2019; Ying et al., 2019; Zhu et al., 2017).
The ability of RSDs to identify individual fires depends mainly on two features: the processing algorithm and the underlying reliability of the BA product. The relatively low capacity of the latter to detect small fires is related to the coarse spatial resolution (500 m) of the MODIS sensor. Several recent studies have shown that MODIS products reliably detect fires over 40–120 ha but miss a number of smaller fires (Fusco et al., 2019; Giglio et al., 2018; Rodrigues et al., 2019; Zhu et al., 2017). Although other BA products, such as FireCCI50 (Chuvieco et al., 2018), provide a finer spatial resolution (250 m), a substantial number of small and/or highly fragmented fires remain undetected, leading to a considerable underestimation of BA (Roteta et al., 2019). In addition, all space-borne BA products face many other well-documented limitations, such as the variability in orbital coverage, satellite overpass time, and satellite view obstruction (Cardoso et al., 2005; Padilla et al., 2014). In this sense, detectability may vary regionally across the globe, and without ground-based fire datasets, it may be difficult to properly validate their reliability (Turco et al., 2019). Nonetheless, the limitations of MCD64A1 are inherent to all RSDs since all of the analyzed products were built on this basis. Hence, differences among RSDs are rather expected to be associated with the underlying algorithm used to identify single fire events.
RSDs were found to better estimate BA than NF. This disparity relies on the complexity of extracting individual fires from gridded BA products. Environmental conditions (e.g., topography, cloud/smoke cover) may influence the sensor detection power, resulting in a break in BA continuity, thereby increasing the risk of artificially splitting single fires into different fire events. Likewise, if a fire lasts longer than the defined cutoff window, it will be automatically split into different events (Oom et al., 2016). By contrast, if multiple fires occur simultaneously in the same region, the parameterization of the RSD algorithms may merge multiple individual fires (Archibald et al., 2013). Lastly, regional features of the fire regime may constrain RSD accuracy. For instance, the Mediterranean fire regime is known for hosting numerous small fires which are unlikely to be detected by satellite observations (Turco et al., 2016). These fires do not contribute very much to the total annual burned area but significantly harm the performance of the RSDs in terms of NF.
The selection of an appropriate fire size threshold depends on the objectives of each analysis. However, in this study, we can generally recommend a minimum size of 100 ha, which stands out as a change point in multiple statistics (Figs. 6 to 8), with the relative error sharply decreasing in both BA and NF above this threshold. Among the analyzed RSDs, FA displayed a slightly better performance with a lower relative error. This may arise from the use of a spatially explicit cutoff threshold, taking both fire spread rate and satellite coverage into account to track the extent of individual fires (Andela et al., 2019b). However, uncertainty in MODIS largely outpaces the uncertainties across the RSDs. The low capacity of gridded BA products to detect small to mid-sized fire events (< 100 ha) can be improved by the generation of products based on higher-resolution sensors in the range of 10–30 m (Roteta et al., 2019). RSDs of individual fires derived from finer-gridded BAs would provide a better estimate of actual NF. In addition, the MCD64A1 product already incorporates the uncertainty of detection as an auxiliary variable of gridded BA data (Giglio et al., 2018). RSDs could benefit from this and report similar information at the individual fire level.
The spatiotemporal aggregation applied in our study is expected to increase the signal-to-noise ratio and thus decrease the uncertainty in RSD estimates. According to Turco (2019), the agreement between remotely sensed and ground-based fire data increases at lower resolutions, being generally best when aggregating the data onto a 1∘ grid (approximately 110 km) or beyond. Likewise, aggregating the data over time (either monthly or annually) also increases the signal-to-noise ratio by filtering out sub-monthly variations (Spadavecchia and Williams, 2009). Evaluating RSDs on shorter timescales and/or at finer spatial resolutions would likely deteriorate the agreement with the GBD. Nevertheless, the spatiotemporal aggregation, such as the one employed here, has been extensively used in previous studies analyzing fire regimes at regional (Barbero et al., 2014; Jiménez-Ruano et al., 2020; Parisien et al., 2014) and global (Bedia et al., 2015; Di Giuseppe et al., 2016; Turco et al., 2018b) scales.
Further studies are still needed to evaluate RSD spatiotemporal variability in the fire patch level (i.e., assign individual fires from RSDs to the GBD) in order to more precisely quantify the dataset accuracy at the fire scale.
In this work, we built upon previous research and investigated the reliability of three RSDs of individual fires over a range of fire size thresholds across the southwestern Mediterranean Basin. Overall, RSDs contain only a small fraction of the total number of fires documented by the GBD. However, they capture reasonably well the temporal variability in fire activity across monthly and annual scales. Despite the different methodologies used to reconstruct fire patches, all RSDs performed similarly and were increasingly accurate when focusing on larger fires. Specifically, when considering fires > 100 ha, RSDs showed reasonable agreement with the GBD.
Generally, the RSDs' underestimation of BA and NF for smaller fires is related to the coarse spatial resolution (500 m) of the pixel-based BA product and other observation limitations which prevented the detection of small fires. Features of the fire regime at regional scales may also influence the agreement between RSDs and the GBD (e.g., fire duration, density, and spread rate). In this sense, our analysis was framed in the southwestern Mediterranean region to capture homogeneous conditions in terms of fire regimes.
We found a better agreement during the warm season (May to October), the main fire season in southern Europe, especially in regions with higher fire activity (northern Iberian Peninsula and southern Sardinia). Also, RSDs were found to better estimate BA than NF. This is rather expected as numerous small fires, which are not detected by satellites, do not contribute very much to the total burned area across the study region.
In practical applications, our results may provide guidance for end users. A quantitative estimate of uncertainty is crucial for the correct interpretation of RSDs, and users should take into account their limitations. Our findings suggest that global RSDs of individual fires can be used to proxy variations in fire activity on monthly or annual timescales; however, caution is advised when drawing conclusions from smaller fires (< 100 ha) across the Mediterranean region. Fire agencies may also benefit from the spatial and temporal consistency of remote-sensing data to support their operational fire mapping system at the regional/national level. Future studies using high-quality ground-based fire data in other regions of the world featuring different fire regimes would provide further insights into RSD uncertainties.
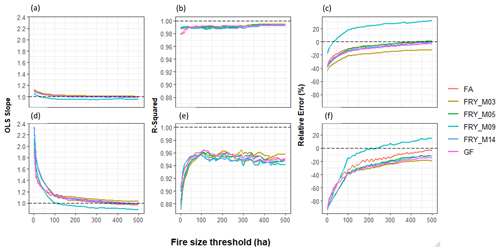
Figure A1Evaluation of RSDs including all FRY cutoff values (3 to 14 d) through different metrics including the slope (a, d), R2 correlation (b, e), and relative error (c, f) for both burned area (a–c) and the number of fires (d–f) over a range of individual fire size thresholds (1 to 500 ha). Dashed lines indicate a perfect fit between RSDs and the GBD.
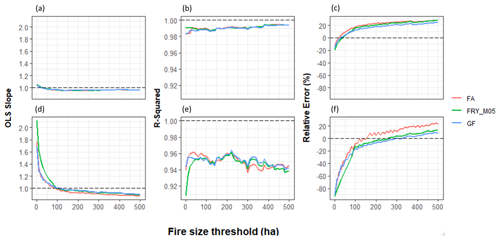
Figure A2Evaluation of “raw” RSDs (i.e., without the land cover filter) through different metrics including the slope (a, d), R2 correlation (b, e), and relative error (c, f) for both burned area (a–c) and the number of fires (d–f) over a range of individual fire size thresholds (1 to 500 ha). Dashed lines indicate a perfect fit between RSDs and the GBD.
The above-described fire datasets, their characteristics, and references to access the data can be found in Tables 1 and 2. All these fire datasets are open access except one of the ground-based datasets (EGIF) which is available upon request. The different data producers host the data in different ways, typically using websites or data repositories. The harmonized GBD used here as the ground-based reference is available at https://doi.org/10.5281/zenodo.3905040 (Galizia et al., 2020).
The supplement related to this article is available online at: https://doi.org/10.5194/nhess-21-73-2021-supplement.
LG performed the data curation, formal analysis, validation, and visualization and wrote the original paper, while all authors edited it. LG, TC, RB, and MR were responsible for the conceptualization and determined the methodology. TC and RB were responsible for supervision.
The authors declare that they have no conflict of interest.
This article is part of the special issue “Remote sensing and Earth observation data in natural hazard and risk studies”. It is not associated with a conference.
We would also like to thank Christophe Bouillon and Fabien Guerra for supporting the harmonization of regional agencies' fire datasets.
This paper was edited by Mahdi Motagh and reviewed by two anonymous referees.
Andela, N., Morton, D. C., Giglio, L., and Anderson, J. T.: Global Fire Atlas with Characteristics of Individual Fires, 2003–2016, ORNL DAAC, https://doi.org/10.3334/ORNLDAAC/1642, 2019a.
Andela, N., Morton, D. C., Giglio, L., Paugam, R., Chen, Y., Hantson, S., van der Werf, G. R., and Randerson, J. T.: The Global Fire Atlas of individual fire size, duration, speed and direction, Earth Syst. Sci. Data, 11, 529–552, https://doi.org/10.5194/essd-11-529-2019, 2019b.
Archibald, S. and Roy, D. P.: Identifying individual fires from satellite-derived burned area data, in: 2009 IEEE International Geoscience and Remote Sensing Symposium, vol. 3, III-160–III-163, 2009.
Archibald, S., Lehmann, C. E. R., Gómez-Dans, J. L., and Bradstock, R. A.: Defining pyromes and global syndromes of fire regimes, P. Natl. Acad. Sci. USA, 110, 6442–6447, https://doi.org/10.1073/pnas.1211466110, 2013.
Artés, T., Oom, D., de Rigo, D., Durrant, T. H., Maianti, P., Libertà, G., and San-Miguel-Ayanz, J.: A global wildfire dataset for the analysis of fire regimes and fire behaviour, Sci. Data, 6, 296, https://doi.org/10.1038/s41597-019-0312-2, 2019.
Artés Vivancos, T. and San-Miguel-Ayanz, J.: Global Wildfire Database for GWIS, Suppl. to Artés Vivancos, Tomàs, Oom, Duarte, Rigo, Daniele, Houst. Durrant, Tracy, Maianti, Pieralberto, Lib. Giorgio, San-Miguel-Ayanz, Jesús A Glob. wildfire dataset Anal. fire regimes fire Behav. Sci., https://doi.org/10.1594/PANGAEA.895835, 2018.
Barbero, R., Abatzoglou, J. T., Steel, E. A., and Larkin, N. K.: Modeling very large-fire occurrences over the continental United States from weather and climate forcing, Environ. Res. Lett., 9, 124009, https://doi.org/10.1088/1748-9326/9/12/124009, 2014.
Bedia, J., Herrera, S., Gutiérrez, J. M., Benali, A., Brands, S., Mota, B., and Moreno, J. M.: Global patterns in the sensitivity of burned area to fire-weather: Implications for climate change, Agr. Forest Meteorol., 214–215, 369–379, https://doi.org/10.1016/j.agrformet.2015.09.002, 2015.
Benali, A., Russo, A., Sá, A. C. L., Pinto, R. M. S., Price, O., Koutsias, N., and Pereira, J. M. C.: Determining fire dates and locating ignition points with satellite data, Remote Sens., 8, 326, https://doi.org/10.3390/rs8040326, 2016.
Bowman, D. M. J. S., Bowman, D. M. J. S., Balch, J. K., Artaxo, P., Bond, W. J., Carlson, J. M., Cochrane, M. A., Antonio, C. M. D., Defries, R. S., Doyle, J. C., Harrison, S. P., Johnston, F. H., Keeley, J. E., and Krawchuk, M. A.: Fire in the Earth System, Science, 481, 481–484, https://doi.org/10.1126/science.1163886, 2009.
Campagnolo, M. L., Libonati, R., Rodrigues, J. A., and Pereira, J. M. C.: Remote Sensing of Environment A comprehensive characterization of MODIS daily burned area mapping accuracy across fire sizes in tropical savannas, Remote Sens. Environ., 252, 112115, https://doi.org/10.1016/j.rse.2020.112115, 2021.
Cardoso, M. F., Hurtt, G. C., Moore, B., Nobre, C. A., and Bain, H.: Field work and statistical analyses for enhanced interpretation of satellite fire data, Remote Sens. Environ., 96, 212–227, https://doi.org/10.1016/j.rse.2005.02.008, 2005.
Chuvieco, E., Lizundia-Loiola, J., Pettinari, M. L., Ramo, R., Padilla, M., Tansey, K., Mouillot, F., Laurent, P., Storm, T., Heil, A., and Plummer, S.: Generation and analysis of a new global burned area product based on MODIS 250 m reflectance bands and thermal anomalies, Earth Syst. Sci. Data, 10, 2015–2031, https://doi.org/10.5194/essd-10-2015-2018, 2018.
Chuvieco, E., Mouillot, F., van der Werf, G. R., San Miguel, J., Tanasse, M., Koutsias, N., García, M., Yebra, M., Padilla, M., Gitas, I., Heil, A., Hawbaker, T. J., and Giglio, L.: Historical background and current developments for mapping burned area from satellite Earth observation, Remote Sens. Environ., 225, 45–64, https://doi.org/10.1016/j.rse.2019.02.013, 2019.
Curt, T., Fréjaville, T., and Bouillon, C.: Characterizing pyroregions in south-eastern France, Adv. For. Fire Res., 4, 1093–1101, https://doi.org/10.14195/978-989-26-0884-6_119, 2014.
Di Giuseppe, F., Pappenberger, F., Wetterhall, F., Krzeminski, B., Camia, A., Libertá, G., and Miguel, J. S.: The potential predictability of fire danger provided by numerical weather prediction, J. Appl. Meteorol. Clim., 55, 2469–2491, https://doi.org/10.1175/JAMC-D-15-0297.1, 2016.
Dupuy, J. L., Fargeon, H., Martin-StPaul, N., Pimont, F., Ruffault, J., Guijarro, M., Hernando, C., Madrigal, J., and Fernandes, P.: Climate change impact on future wildfire danger and activity in southern Europe: a review, Ann. Forest Sci., 77, 1–49, https://doi.org/10.1007/s13595-020-00933-5, 2020.
Fusco, E. J., Finn, J. T., Abatzoglou, J. T., Balch, J. K., Dadashi, S., and Bradley, B. A.: Detection rates and biases of fire observations from MODIS and agency reports in the conterminous United States, Remote Sens. Environ., 220, 30–40, https://doi.org/10.1016/j.rse.2018.10.028, 2019.
Galizia, L. F., Curt, T., Barbero, R., and Rodrigues, M.: Harmonized fire agencies dataset across the Southwestern Mediterranean basin, Zenodo, https://doi.org/10.5281/zenodo.3905040, 2020.
Ganteaume, A., Camia, A., Jappiot, M., San-Miguel-Ayanz, J., Long-Fournel, M., and Lampin, C.: A review of the main driving factors of forest fire ignition over Europe, Environ. Manage., 51, 651–662, https://doi.org/10.1007/s00267-012-9961-z, 2013.
Giglio, L., Randerson, J. T., and Van Der Werf, G. R.: Analysis of daily, monthly, and annual burned area using the fourth-generation global fire emissions database (GFED4), J. Geophys. Res.-Biogeo., 118, 317–328, https://doi.org/10.1002/jgrg.20042, 2013.
Giglio, L., Boschetti, L., Roy, D. P., Humber, M. L., and Justice, C. O.: The Collection 6 MODIS burned area mapping algorithm and product, Remote Sens. Environ., 217, 72–85, https://doi.org/10.1016/j.rse.2018.08.005, 2018.
Hantson, S., Pueyo, S., and Chuvieco, E.: Global fire size distribution is driven by human impact and climate, Global Ecol. Biogeogr., 24, 77–86, https://doi.org/10.1111/geb.12246, 2015.
Hantson, S., Arneth, A., Harrison, S. P., Kelley, D. I., Prentice, I. C., Rabin, S. S., Archibald, S., Mouillot, F., Arnold, S. R., Artaxo, P., Bachelet, D., Ciais, P., Forrest, M., Friedlingstein, P., Hickler, T., Kaplan, J. O., Kloster, S., Knorr, W., Lasslop, G., Li, F., Mangeon, S., Melton, J. R., Meyn, A., Sitch, S., Spessa, A., van der Werf, G. R., Voulgarakis, A., and Yue, C.: The status and challenge of global fire modelling, Biogeosciences, 13, 3359–3375, https://doi.org/10.5194/bg-13-3359-2016, 2016.
Jiménez-Ruano, A., de la Riva Fernández, J., and Rodrigues, M.: Fire regime dynamics in mainland Spain. Part 2: A near-future prospective of fire activity, Sci. Total Environ., 705, 135842, https://doi.org/10.1016/j.scitotenv.2019.135842, 2020.
Keeley, J. E., Bond, W. J., Bradstock, R. A., Pausas, J. G., and Rundel, P. W.: Fire in Mediterranean ecosystems: ecology, evolution and management, Cambridge University Press, New York, United States of America, 2011.
Laurent, P., Mouillot, F., Yue, C., Ciais, P., Moreno, M. V., and Nogueira, J. M. P.: Data Descriptor: FRY, a global database of fire patch functional traits derived from space-borne burned area products, Sci. Data, 5, 1–12, https://doi.org/10.1038/sdata.2018.132, 2018a.
Laurent, P., Mouillot, F., Yue, C., Ciais, P., Moreno, M. V., and Nogueira, J. M. P.: FRY: a global database of fire patch functional traits, France, https://doi.org/10.15148/0e999ffc-e220-41ac-ac85-76e92ecd0320, 2018b.
Molina-Terrén, D. M., Xanthopoulos, G., Diakakis, M., Ribeiro, L., Caballero, D., Delogu, G. M., Viegas, D. X., Silva, C. A., and Cardil, A.: Analysis of forest fire fatalities in Southern Europe: Spain, Portugal, Greece and Sardinia (Italy), Int. J. Wildland Fire, 28, 85–98, https://doi.org/10.1071/WF18004, 2019.
Moreira, F., Viedma, O., Arianoutsou, M., Curt, T., Koutsias, N., Rigolot, E., Barbati, A., Corona, P., Vaz, P., Xanthopoulos, G., Mouillot, F., and Bilgili, E.: Landscape – wildfire interactions in southern Europe: Implications for landscape management, J. Environ. Manage., 92, 2389–2402, https://doi.org/10.1016/j.jenvman.2011.06.028, 2011.
Moreira, F., Ascoli, D., Safford, H., Adams, M. A., Moreno, J. M., Pereira, J. M. C., Catry, F. X., Armesto, J., Bond, W., González, M. E., Curt, T., Koutsias, N., McCaw, L., Price, O., Pausas, J. G., Rigolot, E., Stephens, S., Tavsanoglu, C., Vallejo, V. R., Van Wilgen, B. W., Xanthopoulos, G., and Fernandes, P. M.: Wildfire management in Mediterranean-type regions: Paradigm change needed, Environ. Res. Lett., 15, 011001, https://doi.org/10.1088/1748-9326/ab541e, 2020.
Mouillot, F. and Field, C. B.: Fire history and the global carbon budget: A 1∘ × 1∘ fire history reconstruction for the 20th century, Glob. Change Biol., 11, 398–420, https://doi.org/10.1111/j.1365-2486.2005.00920.x, 2005.
Mouillot, F., Schultz, M. G., Yue, C., Cadule, P., Tansey, K., Ciais, P., and Chuvieco, E.: Ten years of global burned area products from spaceborne remote sensing-A review: Analysis of user needs and recommendations for future developments, Int. J. Appl. Earth Obs., 26, 64–79, https://doi.org/10.1016/j.jag.2013.05.014, 2014.
Nogueira, J. M. P., Ruffault, J., Chuvieco, E., and Mouillot, F.: Can we go beyond burned area in the assessment of global remote sensing products with fire patch metrics?, Remote Sens., 9, p. 7, https://doi.org/10.3390/rs9010007, 2017.
Oom, D., Silva, P. C., Bistinas, I., and Pereira, J. M. C.: Highlighting biome-specific sensitivity of fire size distributions to time-gap parameter using a new algorithm for fire event individuation, Remote Sens., 8, 1–15, https://doi.org/10.3390/rs8080663, 2016.
Padilla, M., Stehman, S. V., and Chuvieco, E.: Validation of the 2008 MODIS-MCD45 global burned area product using stratified random sampling, Remote Sens. Environ., 144, 187–196, https://doi.org/10.1016/j.rse.2014.01.008, 2014.
Parisien, M.-A., Parks, S. A., Krawchuk, M. A., Little, J. M., Flannigan, M. D., Gowman, L. M., and Moritz, M. A.: An analysis of controls on fire activity in boreal Canada: comparing models built with different temporal resolutions, Ecol. Appl., 24, 1341–1356, 2014.
Pausas, J. G. and Fernández-Muñoz, S.: Fire regime changes in the Western Mediterranean Basin: From fuel-limited to drought-driven fire regime, Clim. Change, 110, 215–226, https://doi.org/10.1007/s10584-011-0060-6, 2012.
Pereira, M. G., Malamud, B. D., Trigo, R. M., and Alves, P. I.: The history and characteristics of the 1980–2005 Portuguese rural fire database, Nat. Hazards Earth Syst. Sci., 11, 3343–3358, https://doi.org/10.5194/nhess-11-3343-2011, 2011.
Randerson, J. T., Van Der Werf, G. R., Giglio, L., Collatz, G. J., and Kasibhatla, P. S.: Global Fire Emissions Database, Version 4.1 (GFEDv4), ORNL DAAC, https://doi.org/10.3334/ORNLDAAC/1293, 2015.
Rodrigues, J. A., Libonati, R., Pereira, A. A., Nogueira, J. M. P., Santos, F. L. M., Peres, L. F., Santa Rosa, A., Schroeder, W., Pereira, J. M. C., Giglio, L., Trigo, I. F., and Setzer, A. W.: How well do global burned area products represent fire patterns in the Brazilian Savannas biome? An accuracy assessment of the MCD64 collections, Int. J. Appl. Earth Obs., 78, 318–331, https://doi.org/10.1016/j.jag.2019.02.010, 2019.
Rodrigues, M., Trigo, R. M., Vega-García, C., and Cardil, A.: Identifying large fire weather typologies in the Iberian Peninsula, Agr. Forest Meteorol., 280, 107789, https://doi.org/10.1016/j.agrformet.2019.107789, 2020.
Roteta, E., Bastarrika, A., Padilla, M., Storm, T., and Chuvieco, E.: Development of a Sentinel-2 burned area algorithm: Generation of a small fire database for sub-Saharan Africa, Remote Sens. Environ., 222, 1–17, https://doi.org/10.1016/j.rse.2018.12.011, 2019.
Ruffault, J., Curt, T., Moron, V., Trigo, R., Mouillot, F., Koutsias, N., Pimont, F., Martin-StPaul, N., Barbero, R., Dupuy, J.-L., Russo, A., and Belhadj-Kheder, C.: Increased likelihood of heat-induced large wildfires in the Mediterranean Basin, Sci. Rep.-UK, 10, 13790, https://doi.org/10.1038/s41598-020-70069-z, 2020.
Salis, M., Ager, A. A., Arca, B., Finney, M. A., Bacciu, V., Duce, P., and Spano, D.: Assessing exposure of human and ecological values to wildfire in Sardinia, Italy, Int. J. Wildland Fire, 22, 549–565, https://doi.org/10.1071/WF11060, 2013.
San-Miguel-Ayanz, J., Schmuck, G., Durrant, T., Boca, R., Libertà, G., Petroliagkis, T., Di Leo, M., Rodrigues, D., Boccacci, F., and Schulte, E.: Forest fires in Europe, Middle East and North Africa 2014, Sci. Tech. Res. Ser., 107, 0–114, https://doi.org/10.2788/1082, 2015.
San-Miguel-Ayanz, J., Durrant, T., Boca, R., Libertà, G., Branco, A., De Rigo, D., Ferrari, D., Maianti, P., Vivancos, T. A., and Costa, H.: Forest fires in Europe, Middle East North Africa, 10, Sci. Tech. Res. Ser., https://doi.org/10.2760/663443, 2017.
Spadavecchia, L. and Williams, M.: Can spatio-temporal geostatistical methods improve high resolution regionalisation of meteorological variables?, Agr. Forest Meteorol., 149, 1105–1117, https://doi.org/10.1016/j.agrformet.2009.01.008, 2009.
Turco, M., Bedia, J., Di Liberto, F., Fiorucci, P., Von Hardenberg, J., Koutsias, N., Llasat, M. C., Xystrakis, F., and Provenzale, A.: Decreasing fires in mediterranean Europe, PLoS One, 11, e0150663, https://doi.org/10.1371/journal.pone.0150663, 2016.
Turco, M., Rosa-Cánovas, J. J., Bedia, J., Jerez, S., Montávez, J. P., Llasat, M. C., and Provenzale, A.: Exacerbated fires in Mediterranean Europe due to anthropogenic warming projected with non-stationary climate-fire models, Nat. Commun., 9, 1–9, https://doi.org/10.1038/s41467-018-06358-z, 2018a.
Turco, M., Jerez, S., Doblas-Reyes, F. J., Aghakouchak, A., Llasat, M. C., and Provenzale, A.: Skilful forecasting of global fire activity using seasonal climate predictions, Nat. Commun., 9, 2718, https://doi.org/10.1038/s41467-018-05250-0, 2018b.
Turco, M., Herrera, S., Tourigny, E., Chuvieco, E., and Provenzale, A.: A comparison of remotely-sensed and inventory datasets for burned area in Mediterranean Europe, Int. J. Appl. Earth Obs., 82, 101887, https://doi.org/10.1016/j.jag.2019.05.020, 2019.
van der Werf, G. R., Randerson, J. T., Giglio, L., van Leeuwen, T. T., Chen, Y., Rogers, B. M., Mu, M., van Marle, M. J. E., Morton, D. C., Collatz, G. J., Yokelson, R. J., and Kasibhatla, P. S.: Global fire emissions estimates during 1997–2016, Earth Syst. Sci. Data, 9, 697–720, https://doi.org/10.5194/essd-9-697-2017, 2017.
Williams, A. P. and Abatzoglou, J. T.: Recent Advances and Remaining Uncertainties in Resolving Past and Future Climate Effects on Global Fire Activity, Curr. Clim. Chang. Reports, 2, 1–14, https://doi.org/10.1007/s40641-016-0031-0, 2016.
Ying, L., Shen, Z., Yang, M., and Piao, S.: Wildfire detection probability of MODIS fire products under the constraint of environmental factors: A study based on confirmed ground wildfire records, Remote Sens., 11, 3031, https://doi.org/10.3390/rs11243031, 2019.
Zhu, C., Kobayashi, H., Kanaya, Y., and Saito, M.: Size-dependent validation of MODIS MCD64A1 burned area over six vegetation types in boreal Eurasia: Large underestimation in croplands, Sci. Rep.-UK, 7, 1–9, https://doi.org/10.1038/s41598-017-03739-0, 2017.