the Creative Commons Attribution 4.0 License.
the Creative Commons Attribution 4.0 License.
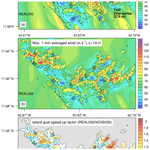
A 30 m scale modeling of extreme gusts during Hurricane Irma (2017) landfall on very small mountainous islands in the Lesser Antilles
Raphaël Cécé
Didier Bernard
Yann Krien
Frédéric Leone
Thomas Candela
Matthieu Péroche
Emmanuel Biabiany
Gael Arnaud
Ali Belmadani
Philippe Palany
Narcisse Zahibo
In view of the high vulnerability of the small islands of the Lesser Antilles to cyclonic hazards, realistic very fine scale numerical simulation of hurricane-induced winds is essential to prevent and manage risks. The present innovative modeling aims at combining the most realistically simulated strongest gusts driven by tornado-scale vortices within the eyewall and the most realistic complex terrain effects. The Weather Research and Forecasting (WRF) model with the nonlinear backscatter and anisotropy (NBA) large eddy simulation (LES) configuration was used to reconstruct the devastating landfall of category 5 Hurricane Irma (2017) on Saint Barthélemy and Saint Martin. The results pointed out that the 30 m scale seems necessary to simulate structures of multiple subtornadic-scale vortices leading to extreme peak gusts of 132 m s−1 over the sea. Based on the literature, such extreme gust values have already been observed and are expected for category 5 hurricanes like Irma. Risk areas associated with terrain gust speed-up factors greater than 1 have been identified for the two islands. The comparison between the simulated gusts and the remote sensing building damage highlighted the major role of structure strength linked with the socio-economic development of the territory. The present modeling method could be easily extended to other small mountainous islands to improve the understanding of observed past damage and to develop safer urban management and appropriate building standards.
- Article
(4450 KB) - Full-text XML
- BibTeX
- EndNote
As described by Cécé et al. (2016), the Lesser Antilles Arc includes small tropical islands (width lower than 50 km) where a total of 1.8 million people live, from Tobago (11.23∘ N, 60.67∘ W) to the Virgin Islands (18.34∘ N, 64.93∘ W). The complex topography of these islands separating the Caribbean Sea from the Atlantic Ocean reflects their volcanic origin.
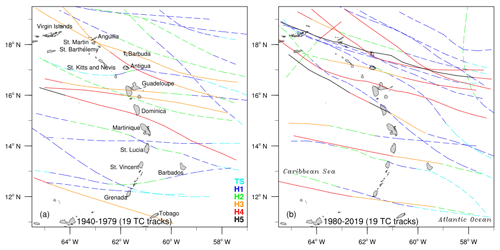
Figure 1Historical tracks of hurricanes that struck the Lesser Antilles in 1940–1979 (a) and in 1980–2019 (b). Colors indicate the hurricane intensity along the track. H1, H2, H3, H4, and H5 stand for category 1, 2, 3, 4, and 5, respectively, on the Saffir–Simpson scale. TS (tropical storm) corresponds to wind speeds lower than 64 kn. Dashed lines indicate the track sections with an intensity weaker than category 3 on the Saffir–Simpson scale. Only hurricane-force events are considered.
The Lesser Antilles are on the path of hurricanes formed over the warm waters off the coasts of West Africa and the Cape Verde islands (at 10–15∘ N latitudes), between the months of July and November. On rare occasions, they can also be exposed to cyclonic storms generated in the Caribbean Sea and taking unusual west-to-east tracks as with Hurricane Omar (2008). According to the analysis of IBTrACS (Knapp et al., 2010, 2018), the Lesser Antilles islands are hit by a hurricane approximately every 2 years. As shown by Fig. 1, the frequency of hurricanes in the region has not changed since the second half of the 20th century, with 19 events reaching or exceeding category 1 on the Saffir–Simpson scale in 1940–1979 and in 1980–2019. However, a significant increase in extreme (category 4–5) hurricanes over recent decades is observed. The number of cat 4–5 hurricanes crossing closer than 50 km from the islands has doubled in the 1980–2019 period. This finding is consistent with the observations of Bhatia et al. (2019) for the Atlantic Ocean and could suggest that the Lesser Antilles will be increasingly exposed to cyclonic risks in the future.
Tropical cyclones have killed 4700 people in the Lesser Antilles since 1900 (EM-DAT, 2019). These deaths as well as the material losses are mainly explained by the intensity of the hazards (wind, flooding, surge, landslide), but also by the high human exposure and unequal socio-economic vulnerability. A total of 13 % of the population live in coastal hazardous areas in these small mountainous islands. The mean gross domestic product (GDP) per capita is about USD 16 000 in the region. While the GDP per capita reaches values like USD 26 000 in the Guadeloupe and Martinique islands, or USD 44 000 in Saint Barthélemy, its value is below USD 8000 in Dominica. In the least developed islands, most residential buildings are small houses with vulnerable sheet-metal roofs which do not have cyclone-resistant standards. Considering the vulnerability of these islands to cyclonic hazards, realistic very fine scale numerical simulation of hurricane-induced winds, rain, and surges is essential to prevent and manage risks. For cases of extreme wind gusts, numerical modeling may help to identify areas with local wind speed-up effects and their factors in order to define appropriate new housing and building standards.
For 10 years, the development of computing resources has allowed more use of the large eddy simulation (LES) technique (i.e. 100 m scale) in numerical weather models like the Weather Research and Forecasting Model (WRF, Skamarock et al., 2008). This very fine scale modeling type has also been applied to study physical processes driving hazardous hurricane-induced gusts (Rotunno et al., 2009; Green and Zhang, 2015; Ito et al., 2017; Worsnop et al., 2017; Stern and Bryan, 2018; Wu et al., 2019). Based on unprecedented Doppler on Wheels (DOW) radar observations during the Hurricane Harvey (2017) landfall, Wurman and Kosiba (2018) showed that local strongest gusts needed to be linked with mesoscale vortices (i.e. several kilometers in size) or tornado-scale vortices (i.e. subkilometer size) occurring within the eyewall. Wu et al. (2019) used a 37 m scale WRF–LES framework to successfully reproduce the tornado-scale vortices characterized by a low-level vertical velocity and a vertical relative vorticity above 20 m s−1 and 0.2 s−1, respectively. However, most recent numerical realistic models of hurricane-induced surface gusts were performed over the sea, without taking into account the effects of lands on the extreme surface winds. Miller et al. (2013) used a linearized model to examine the topography and surface roughness effects of the Bermuda island on Hurricane Fabian (2003) winds. While open-water wind speeds were of category 2 on the Saffir–Simpson scale, the effects of the topography led to maximum modeled wind speeds of category 4 with a clear correlation with the observed damage (Miller et al., 2013). Done et al. (2020) presented a new modeling system to simulate the evolution of the low-level wind fields during tropical cyclone landfall, taking into account topography and surface roughness effects. For the study case of the category 5 Hurricane Maria (2017) landfall in Puerto Rico, the simulated wind reduction factor ranged from 0.5 to 1.0 depending on the spatial surface roughness and spatial terrain height (Done et al., 2020). But this numerical approach seems limited for realistic landfall reconstruction: only the maximum sustained wind may be estimated and not the turbulent peak gusts induced in rain bands or by tornado-scale vortices; the land radiative effects that can result in surface wind enhancement or reduction are not taken into account.
With peak maximum sustained winds of 80 m s−1 and a minimum pressure of 914 hPa, Hurricane Irma (2017) was the strongest Atlantic hurricane ever recorded outside the Caribbean Sea and Gulf of Mexico (Cangialosi et al., 2018; Rey et al., 2019). On 6 September 2017, this category 5 hurricane hit the Lesser Antilles islands, reaching land with this maximum wind speed on Barbuda, Saint Barthélemy, and Saint Martin, at 05:45, 09:30, and 10:30 UTC, respectively. Irma caused 15 deaths and damaged most of the urban structures on the island of Saint Martin. The total cost of the insured damage was estimated at EUR 1.17 billion for the French part (FR) of Saint Martin and EUR 823 million for Saint Barthélemy (Rey et al., 2019). The extreme gusts that occurred during the Irma landfall over Saint Barthélemy and Saint Martin islands were examined with numerical simulations reaching the maximum resolution of 280 m (Duvat et al., 2019; Pillet et al., 2019; Rey et al., 2019). But this subkilometer scale corresponding to the numerical region gap in turbulence modeling, usually called the “turbulence gray zone” or “terra incognita” (Wyngaard, 2004), may lead to erroneous simulated winds. This subkilometer scale also seems insufficient to represent the terrain effects of these two very small mountainous islands (width lower than 15 km).
In the present study, a 30 m scale WRF–LES framework is used to reconstruct the devastating surface peak gusts generated by Hurricane Irma during landfall on Saint Barthélemy and Saint Martin islands. The innovative originality of this new modeling approach aims at combining the most realistic simulated strongest gusts driven by tornado-scale vortices within the eyewall and the most realistic effects of the small mountainous island complex terrain. Two LES turbulence parametrizations are compared at the “terra incognita” scale of 280 m without land interaction. The effects of the resolutions (i.e. 280, 90, and 30 m) on the simulation of the hazardous small-scale vortices are also analyzed in open-water surface conditions. Model outputs allowed island terrain gust speed-up factors to be computed at the 30 m scale for the two islands. The extreme simulated instantaneous surface gusts above 170 m s−1 occurring at the Saint Barthélemy hilltop are examined with 10 Hz numerical time series. For the more populated island of Saint Martin including more coastal urban lowland areas, topography factors and land use factors are also computed separately. The simulated peak gusts are compared to the remote sensing building damage (Copernicus EMSN049, 2018a) estimated in Saint Barthélemy and Saint Martin (FR).
Saint Martin and Saint Barthélemy are two small mountainous islands located in the northern part of the Lesser Antilles around 17.97∘ N and 62.97∘ W (Fig. 2). The two islands are separated by a distance of 20 km. Saint Martin island is divided into two political entities: on the south side, the Netherlands territory called Sint Maarten; on the north side, the French territory called Saint Martin (Rey at al., 2019). The entire island covers an area of 90 km2 with a maximum width of 15 km. Saint Martin island had a population of about 74 000 in 2017 (822 inhabitants per km2). Most urban areas are located in coastal flat lowlands with elevation lower than 25 m a.s.l. (above sea level), and the inland mountain top reaches 424 m a.s.l. (Fig. 2c). Saint Barthélemy is a French island about 4 times smaller than Saint Martin with a surface area of 25 km2 and a maximum length of 9 km (Fig. 2d). This very small island had a population of about 9800 in 2016 (392 inhabitants per km2). In contrast to Saint Martin island, the coastal topography of Saint Barthélemy is mainly characterized by steep cliffs. The mountain top of 286 m is located on the east side of the island. As argued by Cécé et al. (2014), the mechanical effects of mountainous islands on steady winds may be characterized by the local Froude number, which is defined by (U∕Nh), where U is the wind speed, h is the height of the mountain, and N is the buoyancy frequency. When the Froude number is well below unity, the flow can be blocked on the windward side of the mountain inducing wind speed slowdown. In contrast when the Froude number is well above unity the flow passes over the obstacle creating a local wind speed-up at the hilltop. During Hurricane Irma, local Froude numbers at the top of Saint Martin and Saint Barthélemy were 19 and 28, respectively (with U=80 m s−1; N=0.01 s−1; and h=421 and 284 m). As described by Done et al. (2020), the high Froude number induces the flow to pass directly over the hill crest. Under mass continuity, this flow is accelerated at the hilltop due to the local constriction of the air column. These orographic wind speed-up effects have been found during Hurricane Fabian (2003) over the low hill crest of Bermuda (i.e. 86 m) (Miller et al., 2013).
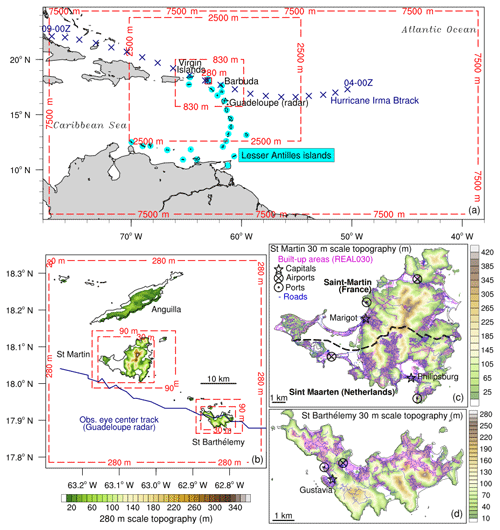
Figure 2Nested domains map: (a) from 7.5 km scale to 280 m scale and (b) from 280 m scale to 30 m scale. Terrain height (m): at 280 m scale (b) and at 30 m scale for St Martin island (c) and St Barthélemy island (d).
These high Froude number values suggest large speed-up factors and surface gusts on the mountain crests for the two islands.
On 6 September 2017, Irma made landfall with these maximum sustained winds of 80 m s−1 successively on Saint Barthélemy at 09:30 UTC and on Saint Martin at 10:30 UTC. With 15 deaths and most of the urban structures damaged, Saint Martin island was more impacted than Saint Barthélemy. According to the remote sensing damage assessment analysis (Copernicus EMSN049, 2018a), more than 95 % of the buildings were damaged on the two islands, with 30 % and 5 % being seriously damaged in Saint Martin (FR) and Saint Barthélemy, respectively. These wide disparities in building damage between these two close small islands could probably be linked with the inequalities in their economic development. While Saint Martin, with a GDP per capita of EUR 16 600, is generally associated with small houses and buildings with vulnerable sheet-metal roofs, Saint Barthélemy, with a GDP per capita of EUR 39 000, has stronger buildings with solid roofs.
All numerical experiments are focused on the landfall of Hurricane Irma on Saint Barthélemy and Saint Martin islands. The simulations cover a period of 6 h between 06:00 and 12:00 UTC on 6 September 2017. The Weather Research and Forecasting model (WRF ARW 3.8.1, Skamarock et al., 2008) is used to perform the simulations. A two-way nested framework with a maximum number of six domains is used to reproduce multi-scale patterns of the hurricane. These six nested domains have resolutions of 7.5, 2.5 km, 833.333 m (approx. 830 m), 277.778 m (approx. 280 m), 92.592 m (approx. 90 m), and 30.864 m (approx. 30 m), respectively (Fig. 2a and b). For simplicity, in the following, we will use the approximate values (i.e. 830, 280, 90, 30 m) to describe the grid scales of the four innermost domains. The fourth nested domain (280 m scale) covers the focus area including Saint Martin island and Saint Barthélemy island. Two pairs of sub-100 m scale inner domains are centered on Saint Martin island and Saint Barthélemy island, respectively (Fig. 2b). The model has 99 terrain-following vertical levels in a logarithmic resolution that is finer in lower levels, and the top is at 30 hPa (Jury et al., 2019). Near the surface, below 1 km altitude, 16 vertical levels are used with the first level at 13
The simulations are initialized with the hybrid ETKF-3DVAR assimilation (Wang et al., 2008) in the outermost domain (7.5 km scale), in the same way as in Jury et al. (2019) and Rey et al. (2019). A parametric Holland vortex (Holland, 1980, Krien et al., 2018) is assimilated using equal weights for the static covariance and the ensemble covariance. An ensemble of 50 perturbed members based on the 0.1∘ scale 6-hourly ECMWF operational analyses is run during 6 h before the initialization time. This method allows a “warm start” of the simulations with a reduced spinup period which is typically equal to 6 h. The 0.1∘ scale 6-hourly ECMWF operational analyses are also used for boundary conditions. Sea surface temperature input fields are provided by NCEP RTG 0.08∘ analyses.
To realistically reproduce the complex terrain of the two islands, 1 s (approx. 30 m) SRTM topography and a custom 30 m scale land-use map have been included in the three innermost domains. The 30 m scale land-use map was established with IGBP MODIS 20-category classification, combining the 2.5 m scale Copernicus EMSN049 land-use maps (Copernicus EMSN049, 2018b), OpenStreetMap data, and the 300 m scale ESA CCI land cover (ESA, 2019).
The main physics parametrizations used here are the rapid radiative transfer model (RRTMG) scheme (Iacono et al., 2008), the WSM6 microphysics scheme (Hong and Lim, 2006), the Noah land surface scheme, and the Monin–Obukhov similarity scheme with a strong wind Donelan–Garratt surface flux option (Green and Zang, 2013). The Kain–Fritsh convective parametrization (Kain, 2004) is added in the outermost domain. All domains include at the top a Rayleigh damping layer of 5 km. As for turbulence parametrization, the 1D YSU PBL scheme (Hong et al., 2006) is turned on in the three outermost domains and turned off in the very fine scale grids (280, 90, and 30 m). These domains are run with a 3D large eddy simulation configuration allowing the most energetic scales of the three-dimensional atmospheric turbulence to be explicitly resolved while the smaller-scale portion of the turbulence spectrum is modeled with a subfilter-scale (SFS) stress model (Mirocha et al., 2010). As explained by Green and Zhang (2015), while the mesoscale 1D PBL turbulence scheme begins to fail for Dx<1 km, LES SFS models are not appropriate when the grid spacing is outside the inertial subrange (when Dx>100 m). This numerical region gap between mesoscale and LES is usually called the “turbulence gray zone” or “terra incognita” (Wyngaard, 2004).
Green and Zhang (2015) showed that the nonlinear backscatter and anisotropy (NBA) SFS stress model (Kosovic, 1997; Mirocha et al., 2010) allows the turbulent structures of the inner core of a real tropical cyclone to be reproduced at grayscale (e.g. 333 m). According to Rotunno et al. (2009), these turbulent structures would be only exhibited at sub-100 m scales with the 1.5-order turbulence kinetic energy (TKE) linear eddy-viscosity SFS stress model (Lilly, 1967). In the present study, the two SFS (TKE and NBA) surface simulated gusts are compared in the 280 m resolution domain.
The results presented here correspond to the numerical experiments described in Table 1. Three experiment types are run: REAL, NOIS, and NOTP, corresponding to real island terrain (i.e. with real topography and real land use), removed island terrain (i.e. with topography set to constant zero value and land use set to constant water category), and removed topography (i.e. with topography set to constant zero value and real land use), respectively. REAL simulations highlight the realistic reconstruction of the Hurricane Irma landfall on Saint Martin and Saint Barthélemy islands. NOIS simulations focus on the sea surface gusts only driven by hurricane eyewall processes. NOTP experiments point out the dynamical and thermal effects of the land-use types over the hurricane winds. These three surface condition experiments are also used to compute surface speed-up factors induced by the real islands, the topography, and the land-use categories. To examine resolution effects avoiding two-way child domain perturbations, all presented model outputs correspond to the innermost domain of the numerical experiments. For example, while the REAL280 experiment includes four nested domains with the innermost domain resolution of 280 m, the REAL090 experiment includes five nested domains with the innermost domain resolution of 90 m.
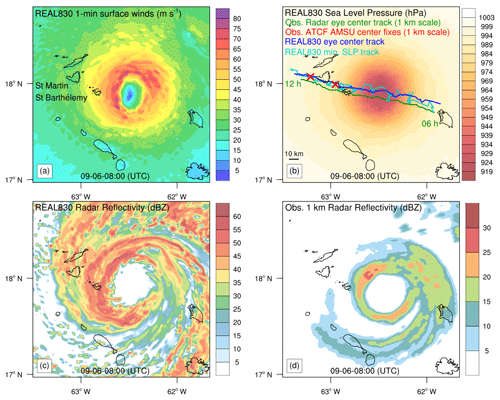
Figure 3Simulated Irma eye at 830 m scale and at 08:00 UTC (REAL830). (a) Sustained surface winds (m s−1). (b) Sea level pressure (hPa) with simulated tracks and observed radar eye center track. The time interval for all tracks is 5 min. (c) Simulated radar reflectivity (dBZ). (d) Observed radar reflectivity (dBZ).
4.1 Mesoscale reconstruction of Hurricane Irma
The intensity and the track of the Hurricane Irma vortex are successfully simulated in the 830 m scale domain (Fig. 3). At 08:00 UTC, 1 h before landfall on Saint Barthélemy, the simulated maximum sustained winds reach 81 m s−1 and the model minimum central pressure of 919 hPa. Based on available observational data, these parameters were officially estimated at 80 m s−1 and 914 hPa between 06:00 and 11:15 UTC (Cangialosi et al., 2018). The model 5 min vortex track shows good agreement with the observed 5 min radar eye center track (Fig. 3b). While the simulated minimum pressure track swirls with the main mesovortex looping the eye center, the simulated eye center track is quite parallel to the radar track with a northward 6 h averaged bias of 10 km. However, this small northward bias needs to be balanced with the uncertainties linked with the 200 km distance of the vortex from the radar located in Guadeloupe. Moreover, this plausible slight southward bias in the radar track seems to be confirmed by the locations of two ATCF AMSU satellite center fixes (Fig. 3b). The rain bands and the convective activity in the eyewall are well developed 2 h after the starting time (Fig. 3c). The underestimated observational reflectivity is probably linked with the large distance from the radar (Fig. 3d).
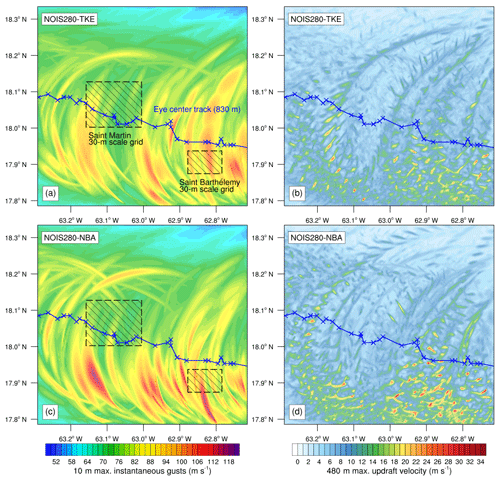
Figure 4Comparison between the TKE SFS scheme and the NBA SFS scheme at 280 m scale: non-island experiment NOIS280. (a, c) Maximum instantaneous gusts (m s−1) occurring at 10 m during the 6 h of simulation (history output interval of 1 min). (b, d) Maximum updraft velocity (m s−1) occurring at 480 m during the 6 h of simulation.
4.2 TKE vs. NBA simulated gusts at 280 m “terra incognita” scale
In order to analyze how this SFS scheme choice affects the eyewall dynamical processes driving surface gusts, without taking into account terrain island effects, the NOIS280 results are presented here with a history output interval of 1 min (Fig. 4). The NBA simulated surface gusts are clearly stronger than the TKE ones all along the study track (Fig. 4a and c). During the 6 h of simulation and in the entire 280 m scale domain, the peak gust values reach 109 and 120 m s−1 for TKE and NBA SFS scheme, respectively. These TKE underestimated gusts are linked with weaker updrafts than in NBA outputs (Fig. 4b and d). Overall, strong updrafts characterized by a vertical velocity above 20 m s−1 (at 480 m level) occurred 585 times in the NBA simulations against only 209 times in the TKE simulations. These comparison results confirm the Mirocha et al. (2010) and the Green and Zhang (2015) ones which claimed that the NBA scheme performs better than the TKE scheme at large LES scales. Following this conclusion and to ensure consistency, the NBA scheme is selected to parametrize the turbulence in the three LES nested grids (280, 90, and 30 m).
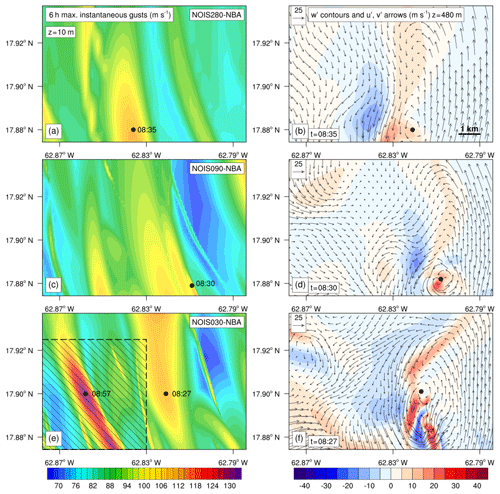
Figure 5Comparison between the three resolutions: 280, 90, and 30 m scale in the Saint Barthélemy 30 m scale domain area (non-island experiments: NOIS280, NOIS090, and NOIS030). The results are not interpolated. (a, c, e) Maximum instantaneous gusts (m s−1) occurring at 10 m during the 6 h of simulation (history output interval of 1 min). (b, d, f) At the 480 m level, perturbation vertical velocity (m s−1) and perturbation horizontal wind vectors at 08:35 (b), 08:30 (d), and 08:27 (f) UTC.
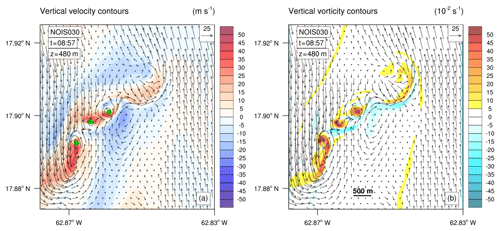
Figure 6Tornado-scale vortices at 480 m level linked with the maximum simulated gust occurring at 08:57 UTC in NOIS030 dashed area (Fig. 4). (a) Perturbation vertical velocity contours (m s−1). (b) Vertical vorticity contours (10−2 s−1). Perturbation horizontal wind vectors are plotted in the two panels.
4.3 Effects of resolution on gusts and small-scale vortices
Figure 4 reveals that Saint Barthélemy island which is located in the path of the most intense quadrant of the eyewall is affected by stronger surface gusts than Saint Martin. The resolution effects assessment is performed in the 30 m scale Saint Barthélemy grid area (size about 9 km per 6.5 km). The open-water results (NOIS) are not interpolated on the same grid: 280, 90, and 30 m grids cover 34×24 points, 98×69 points, and 294×207 points, respectively, in the focus area (Fig. 5). The three resolution outputs reproduced a similar 110 m s−1 intensity peak gust at 08:27, 08:30, and 08:35 UTC for 30, 90, and 280 m scale, respectively. The associated vertical and horizontal perturbation winds (at 480 m level) are examined in the right column after removing the 280 m scale mean wind components (e.g. horizontal wind speed of 70 m s−1 and the north wind direction of 2∘ N). Figure 5 shows that these 110 m s−1 surface gusts are induced by a dynamical structure combining updraft–downdraft couplets and a horizontal kilometer-scale vortex, also called a tornado-scale vortex (Wu et al., 2019). The resolution tends to increase the linked maximum updraft vertical velocity: 24, 33, and 47 m s−1 at 280, 90, and 30 m scale, respectively. The linear patterns in the left column would correspond to these updraft–downdraft couplets and/or small-scale vortices flowing through mean tangential winds. The 280 m resolution and the 90 m resolution allowed medium kilometer-scale vortices and the associated surface instantaneous gust of 110 m s−1 to be reproduced with location errors. An extreme peak gust of 132 m s−1 occurring at 08:57 UTC is simulated in the 30 m scale domain. This instantaneous surface gust value, seeming unreal, has already been measured when category 5 Hurricane Orson (1989) passed over an offshore platform (Harper et al., 2010). Based on observed and simulated dropsonde assessment, Stern and Bryan (2018) concluded that it seems likely that 120–140 m s−1 instantaneous gusts are present in category 5 hurricanes. The 132 m s−1 extreme gusts simulated here are linked with a particular dynamical structure combining three 400 m scale vortices (Fig. 6). These very intense vortices are characterized by a vertical relative vorticity higher than 0.50 s−1 and a maximum vertical velocity reaching 50 m s−1 at the altitude of 480 m. As shown by Fig. 7, while the 90 m scale model reproduces tornado-scale vortices well with a maximum vertical vorticity of 0.68 s−1 over the 360 min of simulation and the vertical column below 600 m level, the 30 m scale is necessary to simulate structures of multiple subtornadic-scale vortices linked with maximum vertical vorticity above 1 s−1 and leading to extreme peak gusts. This kind of structure with multiple very small-scale vortices (diameter lower than 500 m) has been already observed in a violent tornado (Wurman, 2002). The different timescales of the simulated gusts linked with the three resolutions need to be taken into account in this discussion. Indeed, the time-step values of 0.883, 0.278, and 0.093 s for the 280, 90, and 30 m scale, respectively, could also suggest a better sampling of the extreme peak gusts at the finest grid scale. To limit computational costs, the surface hurricane gusts over the two islands were only simulated at the 30 m scale. This choice corresponds to the objective of this study: to reproduce expected extreme category 5 hurricane gusts (i.e. 130 m s−1) as well as the most realistic topography and land-use effects. Additional multiscale numerical experiments would be necessary to analyze the improvement linked with the scale of topography and land use.
4.4 Effects of Saint Barthélemy island terrain on gusts
The REAL030 experiment outputs show that the maximum surface hurricane winds are very sensitive to the terrain of the 9 km wide Saint Barthélemy island, no matter how small (Fig. 8). During the Irma landfall, the windward north coast was globally affected by stronger surface winds than the leeward south coast. Unfortunately, there are no observational wind data which would allow the simulated gusts during the Irma landfall to be evaluated. However, the last 68 m s−1 instantaneous gust recorded at 08:07 UTC by the weather station located in Gustavia (leeward southwest coast) suggests even higher peak value in mountainous windward areas (Rey et al., 2019). To quantify wind enhancement or reduction linked with real island terrain (topography and land use), the island gust speed-up factor is computed: the REAL030 maximum gust values are divided by the NOIS030 ones (Fig. 8c). As predicted with the Froude number analysis, strong instantaneous gusts (>140 m s−1), large sustained winds (>100 m s−1), and high island speed-up factors (>1.5) occur on the mountain crests (>150 m). On the other hand, NOIS030 maximum gust values may be halved in inland low areas and on the leeward coast. The peak gust value averaged on all built-up areas of the island is equal to 95 m s−1, which corresponds to the EF5 maximum enhanced Fujita scale, suggesting immense damage to structures (WSEC, 2006). Unusual extreme peak gusts (>160 m s−1) simulated in the northeast mountainous areas would suggest the crossing of an eyewall small-scale vortex. The 10 Hz simulated surface wind time series are studied at two locations (Figs. 8 and 9): SEA located upstream and over the sea and TOP located at the hilltop. Figure 9 highlights the strong correlation between the two signals (SEA and TOP) before the eye center passage and the induced change in wind direction (SEA location becomes downstream). The TOP extreme gust of 188 m s−1 linked with the maximum gust simulated at SEA location does not seem inconsistent or unreal in comparison with the associated 1 min averaged wind of 143 m s−1 (gust factor with a typical value of 1.3). It also needs to be noted that in the same time period, the increase in the 1 min averaged winds sharply exceeds 20 m s−1 (SEA) and 45 m s−1 (TOP). This unusual gust value occurring at 08:28 UTC is produced by a local high enhancement of the surface winds along a tornado-scale vortex flowing from SEA to TOP locations, as simulated at the same time in NOIS030 (Fig. 5f). For a better understanding of the TOP/SEA wind enhancement factor, the vertical profile of the wind speed was examined at SEA and TOP locations before and during the peak gust time (i.e. 08:00 and 08:27 UTC). The study of the wind speed at 280 (i.e. the height of the surface winds at the hilltop of Saint Barthélemy) highlights the fact that the same level winds flowing upstream over the sea are accelerated at the hilltop (Fig. 10). This local wind speed-up factor induced by the air column constriction at the hilltop has closed values at the two times: 1.35 and 1.33 at 08:00 and 08:27 UTC, respectively. Moreover, the analysis of these high-frequency time series points out the fact that in our 30 m scale outputs, probably due to the insufficiently developed model turbulence, the instantaneous gusts may be equated to 3 s averaged gusts. Indeed, out of 18 000 data points the mean absolute difference between the instantaneous wind speeds and the 3 s averaged wind speeds is equal to 0.02 m s−1.
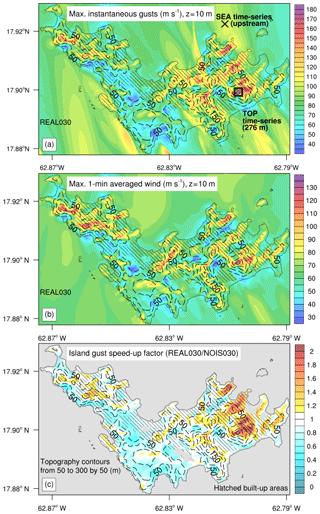
Figure 8Saint Barthélemy 30 m scale maximum surface winds during the 6 h of simulation (history output interval of 1 min). (a) Maximum instantaneous gust (m s−1) with locations of two numerical time-series stations: SEA and TOP. (b) Maximum 1 min averaged wind in m s−1. (c) Island gust speed-up factor (REAL030/NOIS030). Topography contours in meters and hatched built-up areas are plotted on the three panels.
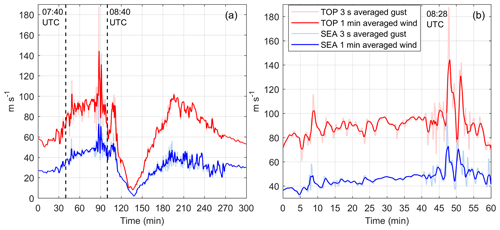
Figure 9REAL030 time series in Saint Barthélemy: comparison between the upstream surface winds over the sea (SEA, Fig. 6) and the orographic surface winds over the mountain top (TOP, Fig. 8). (a) From 07:00 to 12:00 UTC. (b) From 07:40 to 08:40 UTC before landfall on Saint Barthélemy.
4.5 Effects of Saint Martin island terrain on gusts
As described above, Saint Martin island, which is located further north than the path of the most intense eyewall quadrant, is affected by weaker hurricane boundary layer vortices and weaker surface gusts than Saint Barthélemy (Fig. 11). The highest values of simulated instantaneous gusts slightly exceed 110 m s−1 in some coastal and mountain crest areas. However, the peak gust value averaged on all built-up areas of the island is equal to 72 m s−1, which corresponds to the low limit of the EF4 enhanced Fujita scale linked with devastating damage to structures (WSEC, 2006). These maximum gusts are linked with a peak island gust speed-up factor of 1.6. On the other hand, in some inland valley areas, the island terrain may reduce NOIS030 gust value by 80 %. In the case of Saint Martin, which is 4 times wider than Saint Barthélemy and which includes more lowland areas, it seems necessary to also examine the land-use effects on the surface gusts (Fig. 12). As expected, while the topography has an enhancing effect globally, the land use (with roughness length higher than the 0.01 cm water bodies' roughness length) has a reducing effect. The open-water gusts may be halved over the mixed forest category characterized by a roughness length of 50 cm. However, surface radiative processes need to be taken into account to explain why the built-up areas category with the highest roughness length induces lower gust reduction. Moreover, the Irma landfall occurs during the nocturnal radiative cooling. With its high heat storage and the anthropogenic heat emissions inhibiting the nocturnal radiative cooling, the skin surface temperature over urban areas is globally 1 ∘C higher than over the vegetation categories (Fig. 12d). In the present case, the 3 h averaged skin surface temperature is also more strongly correlated with a land-use gust reduction factor than the roughness length: the Pearson correlation coefficients are equal to 0.63 and 0.27, respectively.
4.6 Relationships between simulated gusts and remote sensing building damage
The post-Irma remote sensing building damage assessment (Copernicus EMSN049, 2018a) that focused on Saint Martin (FR) and Saint Barthélemy is examined here, compared with the simulated gusts. Due to many uncertainties, the maps provided by the Copernicus Emergency Management Service at building scale are very hard to interpret and to discuss. Firstly, some uncertainties in damage grading levels (especially weak damage) are related to the technical limitations of satellite image acquisition: cloud cover, dust, and mist over areas of interest; image resolution of 50 cm insufficient to analyze some details and diversity of identification criteria (Dorati et al., 2018). Moreover, Copernicus EMSN049 (2018a) data include neither building types nor wind vulnerability. However, it has been clearly proven that wind resistance depends on building design, masonry techniques, and material quality (Prevatt and Roueche, 2019). Therefore, it seems difficult to finely correlate damage intensities with surface peak gusts. In coastal areas, remote sensing building damage may also include surge and wave effects (Rey et al., 2019). In order to smooth the effects from remote sensing uncertainties, an improved method is presented here. Destruction ratio values are computed over the 100 m grid cells including at least 10 buildings to keep consistency. This ratio is equal to the number of seriously damaged buildings (i.e. EMNS049 gradings “Severe damaged” and “Destroyed”) divided by the total number of buildings in the 100 m grid cell. This severe damage linked with significant or total roof loss (Dorati et al., 2018) is less ambiguous to identify by remote sensing. Figure 13a shows that only a few urban areas were not affected by partial or total roof loss in Saint Martin (FR) and Saint Barthélemy. Large disparities in destruction ratio are visible within and between the two islands. Within the islands, these disparities may be explained by local gust variations deepened by speed-up effects on windward slopes or mountain crests and also hurricane swell effects in coastal areas. In view of the similar topographic and coastal destruction contribution factors in the two islands, the damage disparities between Saint Martin and Saint Barthélemy would reveal the high socio-economic inequalities between these territories (Table 2). Despite the fact that built-up areas in Saint Barthélémy were affected by stronger gusts (i.e. mean of 92 m s−1), the mean remote sensing destruction ratio is equal to 12 %. The territory of Saint Martin (i.e. GDP difference in Table 2), which is half as developed, includes a mean peak gust value of 72 m s−1, and the mean destruction ratio is equal to 35 % over all urban areas. Figure 13b highlights these higher destruction ratio values in Saint Martin, while in Saint Barthélemy this ratio rarely exceeds 50 % despite stronger gusts. The peak destruction ratios are more easily reached in Saint Martin (saturation effects) which reflects the high weakness of built-up structures. In Saint Barthélemy, the more stretched scatter plot globally indicates a greater building resistance. Figure 13b also allows a gust threshold value of around 60 m s−1, beyond which damage becomes significant over the two islands, to be identified. This gust threshold also corresponds to 6 degrees of damage on the enhanced Fujita scale (i.e. large sections of roof structure removed; most walls remain standing) for the building types “One- or Two-Family Residences” and “Apartments, Condominiums and Townhouses” (WSEC, 2006).
A 30 m scale WRF–LES framework was used to reconstruct the devastating surface peak gusts generated by category 5 Hurricane Irma during landfall on Saint Barthélemy and Saint Martin islands. This innovative modeling approach aimed at combining the most realistic simulated strongest gusts driven by tornado-scale vortices within the eyewall and the most realistic effects of the small mountainous island complex terrain.
The intensity and the track of the category 5 Hurricane Irma vortex were accurately reproduced by the model at kilometer scale: simulated maximum sustained winds reached 81 m s−1 (obs. 80 m s−1) and the model minimum central pressure was 919 hPa (obs. 914 hPa).
With strong updrafts occurring 3 times more in the NBA simulation, our numerical results confirmed the Mirocha et al. (2010) and the Green and Zhang (2015) ones which claimed that the NBA SFS stress model performs better eyewall turbulent processes than the TKE scheme at large LES scales like 280 m (i.e. turbulence gray zone). The 280 m resolution and the 90 m resolution allowed medium kilometer-scale vortices and the associated surface instantaneous gust of 110 m s−1 to be reproduced with location errors. Moreover, while the 90 m resolution simulates tornado-scale vortices well, the 30 m resolution seems necessary to simulate intense structures of multiple 400 m scale vortices (i.e. subtornadic-scale vortices) which may lead to extreme peak gusts like 132 m s−1 in open-water conditions. Based on the literature, such extreme gust values have already been observed and are expected for category 5 hurricanes like Irma (Harper et al., 2010; Stern and Bryan, 2018).
To limit computational costs, the surface hurricane gusts over the two islands were only simulated at the 30 m scale. This choice corresponds to the objective of this study: to reproduce expected extreme category 5 hurricane gusts (i.e. 130 m s−1) as well as the most realistic topography and land-use effects. Additional multiscale numerical experiments would be necessary to analyze the improvement linked with the scale of topography and land use. The 30 m scale experiment outputs showed that the maximum surface hurricane winds are very sensitive to the complex terrain of the two small islands.
To quantify wind enhancement or reduction linked with real island terrain (topography and land use), the island gust speed-up factor was computed. Risk areas associated with terrain gust speed-up factors greater than 1 have been identified for the two islands. The highest island speed-up factors (>1.4) associated with the strongest surface gusts (>110 m s−1 in Saint Martin and >140 m s−1 in Saint Barthélemy) occurred on the mountain crests. This speed-up factor exceeded 1.8 during the crossing of a small-scale vortex over the hilltop of Saint Barthélemy, inducing an extreme unusual peak gust of 188 m s−1. While the topography had an enhancing effect globally, the land-use categories (with roughness length higher than the 0.01 cm water bodies' roughness length) had a reducing effect. However, our numerical experiments over Saint Martin highlighted the fact that surface radiative processes need to be take into account: the skin surface temperature was more strongly correlated with the land-use gust reduction factor than the roughness length.
Based on remote sensing building damage (Copernicus EMSN049, 2018a), a destruction ratio map was computed for severe damage. The comparison between the simulated gusts and the remote sensing building damage highlighted the major role of structure strength linked with the socio-economic development of the territory. Despite the fact that built-up areas in Saint Barthélemy were affected by stronger gusts, the mean destruction ratio was 3 times lower than in the less developed territory of Saint Martin (FR), including commonly weaker buildings with vulnerable sheet-metal roofs.
In view of the high vulnerability of the Lesser Antilles islands to cyclonic hazards, the complex terrain of these small islands, and the lack of observational data, realistic very fine scale numerical simulation of hurricane-induced winds is essential to prevent and manage risks. The present 30 m scale numerical method could be easily extended to other small mountainous islands exposed to hurricane gust hazards. On the one hand, it could be useful to improve the understanding of the observed past damage. On the other hand, this modeling approach applied to prospective or past cyclonic disaster should allow areas with terrain gust speed-up effects to be identified to develop safer urban management and appropriate building standards (strengthening of the structures).
Data from this research are not publicly available. Interested researchers can contact the corresponding author of this article.
The study was mainly conceptualized and written by RC. DB, YK, GA, EB, and AB provided comments for the results and reviewed the paper. FL, TC, and MP led the analysis of the remote sensing building damage and its comparison with the simulated winds. YK and GA worked on the Holland-type synthetic vortex and the initial conditions of the simulations. EB helped with computation and programming. DB, FL, PP, and NZ prepared the C3AF project and the ANR/TIREX project which funded the present research.
The authors declare that they have no conflict of interest.
The radar observational data were obtained from the French Met Office (Météo France). The authors gratefully acknowledge Martin Robustelli for producing the 30 m scale land use map for the two focused islands: Saint Martin and Saint Barthélemy. The WRF-LES simulations were computed on the Wahoo cluster (Intensive Computing Center (C3I), University of the French West Indies). The authors wish to thank Danièle Frison who helped with the translation.
This research has been supported by the ERDF/C3AF project (grant no. CR/16-115) and the ANR/TIREX project (grant no. ANR-18-OURA-0002-05).
This paper was edited by Piero Lionello and reviewed by three anonymous referees.
Bhatia, K. T., Vecchi, G. A., Knutson, T. R., Murakami, H., Kossin, J., Dixon, K. W., and Whitlock, C. E.: Recent increases in tropical cyclone intensification rates, Nat. Commun., 10, 635, https://doi.org/10.1038/s41467-019-08471-z, 2019.
Cangialosi, J. P., Latto, A. S., and Berg, R.: Hurricane Irma 2017, Tropical Cyclone Report, National Hurricane Center, Miami, FL, USA, 111 pp., available at: https://www.nhc.noaa.gov/data/tcr/AL112017_Irma.pdf (last access: 15 January 2020), 2018.
Cécé, R., Bernard, D., d'Alexis, C., and Dorville, J.-F.: Numerical simulations of island-induced circulations and windward katabatic flow over the Guadeloupe archipelago, Mon. Weather Rev., 142, 850–867, https://doi.org/10.1175/MWR-D-13-00119.1, 2014.
Cécé, R., Bernard, D., Brioude, J., and Zahibo, N.: Microscale anthropogenic pollution modelling in a small tropical island during weak trade winds: Lagrangian particle dispersion simulations using real nested LES meteorological fields, Atmos. Environ., 139, 98–112, https://doi.org/10.1016/j.atmosenv.2016.05.028, 2016.
Copernicus EMSN049: Damage Assessment Map – Post IRMA Analysis, scale 1:25 000, published: 25 April 2018, product version: v2, quality approved, available at: https://emergency.copernicus.eu/mapping/list-of-components/EMSN049 (last access: 1 February 2020), 2018a.
Copernicus EMSN049: Land Use and Land Cover Map, scale 1:25 000, published: 25 April 2018, product version: v1, quality approved, available at: https://emergency.copernicus.eu/mapping/list-of-components/EMSN049 (last access: 1 October 2019), 2018b.
Done, J. M., Ge, M., Holland, G. J., Dima-West, I., Phibbs, S., Saville, G. R., and Wang, Y.: Modelling global tropical cyclone wind footprints, Nat. Hazards Earth Syst. Sci., 20, 567–580, https://doi.org/10.5194/nhess-20-567-2020, 2020.
Dorati, C., Kucera, J., Marí i Rivero I., and Wania, A.: Product User Manual of Copernicus EMS Rapid Mapping, JRC Technical Report JRC111889, available at: https://emergency.copernicus.eu/mapping/ems/product-user-manual-cems-rapid-mapping (last access: 1 February 2020), 2018.
Duvat, V., Pillet, V., Volto, N., Krien, Y., Cécé, R., and Bernard, D.: High human influence on beach response to tropical cyclones in small islands: Saint-Martin Island, Lesser Antilles, Geomorphology, 325, 70–91, https://doi.org/10.1016/j.geomorph.2018.09.029, 2019.
EM-DAT: The Emergency Events Database, Université catholique de Louvain (UCL) – CRED, Brussels, Belgium, available at: http://www.emdat.be, last access: 1 October 2019.
ESA: Land Cover CCI Product User Guide Version 2, Tech. Rep., available at: http://maps.elie.ucl.ac.be/CCI/viewer/download/ESACCI-LC-Ph2-PUGv2_2.0.pdf, last access: 1 October 2019.
Green, B. W. and Zhang, F.: Impacts of air–sea flux parameterizations on the intensity and structure of tropical cyclones, Mon. Weather Rev., 141, 2308–2324, https://doi.org/10.1175/MWR-D-12-00274.1, 2013.
Green, B. W. and Zhang, F.: Numerical simulations of Hurricane Katrina (2005) in the turbulent gray zone, J. Adv. Model. Earth Syst., 7, 142–161, https://doi.org/10.1002/2014MS000399, 2015.
Harper, B. A., Kepert, J. D., and Ginger, J. D.: Guidelines for converting between various wind averaging periods in tropical cyclone conditions, WMO Tech. Rep. WMO-TD-1555, 64 pp., available at: https://library.wmo.int/doc_num.php?explnum_id=290 (last access: 15 July 2020), 2010.
Holland, G. J.: An analytic model of the wind and pressure profiles in hurricanes, Mon. Weather Rev., 108, 1212–1218, https://doi.org/10.1175/1520-0493(1980)108<1212:AAMOTW>2.0.CO;2, 1980.
Hong, S. and Lim, J.: The WRF Single-Moment 6-Class Microphysics Scheme (WSM6), J. Korean Meteor. Soc., 42, 129–151, 2006.
Hong, S.-Y., Noh, Y., and Dudhia, J.: A new vertical diffusion package with an explicit treatment of entrainment processes, Mon. Weather Rev., 134, 2318–2341, https://doi.org/10.1175/MWR3199.1, 2006.
Iacono, M. J., Delamere, J. S., Mlawer, E. J., Shephard, M. W., Clough, S. A., and Collins, W. D.: Radiative forcing by long-lived greenhouse gases: Calculations with the AER radiative transfer models, J. Geophys. Res., 113, D13103, https://doi.org/10.1029/2008JD009944, 2008.
Ito, J., Oizumi, T., and Niino, H.: Near-surface coherent structures explored by large eddy simulation of entire tropical cyclones, Sci. Rep.-UK, 7, 3798, https://doi.org/10.1038/s41598-017-03848-w, 2017.
Jury, M. R., Chiao, S., and Cécé R.: The Intensification of Hurricane Maria 2017 in the Antilles, Atmosphere, 10, 590, https://doi.org/10.3390/atmos10100590, 2019.
Kain, J. S.: The Kain–Fritsch convective parameterization: An update, J. Appl. Meteorol., 43, 170–181, https://doi.org/10.1175/1520-0450(2004)043<0170:TKCPAU>2.0.CO;2 2004.
Knapp, K. R., Kruk, M. C., Levinson, D. H., Diamond, H. J., and Neumann, C. J.: The International Best Track Archive for Climate Stewardship (IBTrACS): Unifying tropical cyclone best track data, B. Am. Meteorol. Soc., 91, 363–376, https://doi.org/10.1175/2009BAMS2755.1, 2010.
Knapp, K. R., Diamond, H. J., Kossin, J. P., Kruk, M. C., and Schreck, C. J.: International Best Track Archive for Climate Stewardship (IBTrACS) Project, Version 4, NOAA National Centers for Environmental Information, https://doi.org/10.25921/82ty-9e16, 2018.
Kosović, B.: Subgrid-scale modelling for the large-eddy simulation of high-Reynolds-number boundary layers, J. Fluid Mech., 336, 151–182, https://doi.org/10.1017/S0022112096004697, 1997.
Krien, Y., Arnaud, G., Cécé, R., Ruf, C., Belmadani, A., Khan, J., Bernard, D., Islam, A., Durand, F., Testut, L., Palany, P., and Zahibo, N.: Can we improve parametric cyclonic wind fields using recent satellite remote sensing data?, Remote Sens.-Basel, 10, 1963, https://doi.org/10.3390/rs10121963, 2018.
Lilly, D. K.: The representation of small-scale turbulence in numerical simulation experiments, in: Proc. IBM Scientific Computing Symp. on Environmental Sciences, IBM, White Plains, New-York, 195–210, 1967.
Miller, C., Gibbons, M., Beatty, K., and Boissonnade A.: Topographic Speed-Up Effects and Observed Roof Damage on Bermuda following Hurricane Fabian (2003), Weather Forecast., 28, 159–174, https://doi.org/10.1175/WAF-D-12-00050.1, 2013.
Mirocha, J. D., Lundquist, J. K., and B. Kosović, B.: Implementation of a Nonlinear Subfilter Turbulence Stress Model for Large-Eddy Simulation in the Advanced Research WRF Model, Mon. Weather Rev., 138, 4212–4228, https://doi.org/10.1175/2010MWR3286.1, 2010.
Pillet, V., Duvat, V. K. E., Krien, Y., Cécé, R., Arnaud, G., and Pignon-Mussaud, C.: Assessing the impacts of shoreline hardening on beach response to hurricanes: Saint-Barthélemy, Lesser Antilles, Ocean Coast. Manage., 174, 71–91, https://doi.org/10.1016/j.ocecoaman.2019.03.021, 2019.
Prevatt, D. O. and Roueche D. B.: Survey and Investigation of Buildings Damaged by Category-III, IV & V Hurricanes in FY 2018–2019 – Hurricane Michael, Florida Department of Business and Professional Regulation, Florida, USA, 106 pp., available at: http://www.floridabuilding.org/fbc/publications/Research_2018-2019/Prevatt-UF-Hurricane_Michael_Report_Final-06-18-2019.pdf (last access: 15 July 2020), 2019.
Rey, T., Leone, F., Candela, T., Belmadani, A., Palany, P., Krien, Y., Cécé, R., Gherardi, M., Péroche, M., and Zahibo, N.,: Coastal Processes and Influence on Damage to Urban Structures during Hurricane Irma (St-Martin & St-Barthélemy, French West Indies), J. Mar. Sci. Eng., 7, 215, https://doi.org/10.3390/jmse7070215, 2019.
Rotunno, R., Chen, Y., Wang, W., Davis, C., Dudhia, J., and Holland, G. J.: Large-Eddy Simulation of an Idealized Tropical Cyclone, B. Am. Meteorol. Soc., 90, 1783–1788, https://doi.org/10.1175/2009BAMS2884.1, 2009.
Skamarock, W. C., Klemp, J. B., Dudhia, J., Gill, D. O., Barker, D. M., Duda, M. G., Huang, X. Y., Wang, W., and Powers, J. G.: A Description of the Advanced Research WRF version 3, Tech. Rep. NCAR/TN-475+STR, National Center for Atmospheric Research, available at: https://opensky.ucar.edu/islandora/object/technotes:500/datastream/PDF/view (last access: 15 January 2021), 2008.
Stern, D. P. and Bryan, G. H.: Using Simulated Dropsondes to Understand Extreme Updrafts and Wind Speeds in Tropical Cyclones, Mon. Weather Rev., 146, 3901–3925, https://doi.org/10.1175/MWR-D-18-0041.1, 2018.
Wang, X., Barker, D. M., Snyder, C., and Hamill, T. N.: A hybrid ETKF–3DVAR data assimilation scheme for the WRF model. part I: Observing system simulation experiment, Mon. Weather Rev., 136, 5116–5131, https://doi.org/10.1175/2008MWR2444.1, 2008.
Worsnop, R. P., Lundquist, J. K., Bryan, G. H., Damiani, R., and Musial, W.: Gusts and shear within hurricane eyewalls can exceed offshore wind turbine design standards, Geophys. Res. Lett., 44, 6413– 6420, https://doi.org/10.1002/2017GL073537, 2017.
WSEC: A recommendation for an enhanced Fujita scale (EF-Scale), Texas Tech University Wind Science and Engineering Center, Tech. Rep., Lubbock, Texas, 111 pp., available at: https://www.spc.noaa.gov/efscale/ef-ttu.pdf (last access: 15 July 2020), 2006.
Wu, L., Liu, Q., and Li, Y.: Tornado-scale vortices in the tropical cyclone boundary layer: numerical simulation with the WRF–LES framework, Atmos. Chem. Phys., 19, 2477–2487, https://doi.org/10.5194/acp-19-2477-2019, 2019.
Wurman, J.: The Multiple-Vortex Structure of a Tornado, Weather Forecast., 17, 473–505, https://doi.org/10.1175/1520-0434(2002)017<0473:TMVSOA>2.0.CO;2, 2002.
Wurman, J. and Kosiba, K.: The Role of Small-Scale Vortices in Enhancing Surface Winds and Damage in Hurricane Harvey (2017), Mon. Weather Rev., 146, 713–722, https://doi.org/10.1175/MWR-D-17-0327.1, 2018.
Wyngaard, J. C.: Toward Numerical Modeling in the “Terra Incognita”, J. Atmos. Sci., 61, 1816–1826, https://doi.org/10.1175/1520-0469(2004)061<1816:TNMITT>2.0.CO;2, 2004.