the Creative Commons Attribution 4.0 License.
the Creative Commons Attribution 4.0 License.
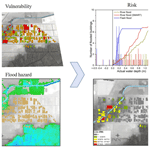
Flood vulnerability and risk assessment of urban traditional buildings in a heritage district of Kuala Lumpur, Malaysia
Dina D'Ayala
Yuan Yan
Helen Smith
Ashleigh Massam
Valeriya Filipova
Joy Jacqueline Pereira
Flood hazard is increasing in frequency and magnitude in major South East Asian metropolitan areas due to fast urban development and changes in climate, threatening people's property and life. Typically, flood management actions are mostly focused on large-scale defences, such as river embankments or discharge channels or tunnels. However, these are difficult to implement in town centres without affecting the value of their heritage districts and might not provide sufficient mitigation. Therefore, urban heritage buildings may become vulnerable to flood events, even when they were originally designed and built with intrinsic resilient measures, based on the local knowledge of the natural environment and its threats at the time. Their aesthetic and cultural and economic values mean that they can represent a proportionally high contribution to losses in any event. Hence it is worth investigating more localized, tailored mitigation measures. Vulnerability assessment studies are essential to inform the feasibility and development of such strategies. In this study we propose a multilevel methodology to assess the flood vulnerability and risk of residential buildings in an area of Kuala Lumpur, Malaysia, characterized by traditional timber housing. The multiscale flood vulnerability model is based on a wide range of parameters, covering building-specific parameters, neighbourhood conditions and catchment area conditions. The obtained vulnerability index shows the ability to reflect different exposure by different building types and their relative locations. The vulnerability model is combined with high-resolution fluvial and pluvial flood maps providing scenario events with 0.1 % annual exceedance probability (AEP). A damage function of generic applicability is developed to compute the economic losses at individual building and sample levels. The study provides evidence that results obtained for a small district can be scaled up to the city level, to inform both generic and specific protection strategies.
- Article
(4485 KB) - Full-text XML
- BibTeX
- EndNote
The Sendai Framework 2015–2030 clearly identifies both climate change and rapid urbanization as disaster risk drivers (UNISDR, 2015). Temperature rise and global warming are strictly correlated to increased rainfall (Min et al., 2011; Wang et al., 2017) and in turn to the increased frequency and extent of droughts and floods (Pall et al., 2011; IPCC, 2013, 2014; Mysiak et al., 2016). Flood risk however is compounded not only by intensified hazard, but also very importantly by increased exposure due to increased urbanization along coastlines, river basins and floodplains (Neumann et al., 2015; Kundzewicz et al., 2014). Such flood risk becomes even more challenging in South and South East Asia, as observed (Najibi and Devineni, 2018) and projected (Harabayashi et al., 2013) flood frequency show dramatic increasing trends.
Following studies on the increased flood risk caused by the increasing rate of impervious surface to drainage capacity in urban areas, (e.g. Ashley et al., 2005; Jacobson, 2011; Jha et al., 2012; Liao, 2012), the shift from control to adaptation in urban flood resilience is increasingly advocated by governmental agencies, experts and developers alike. Structural mitigation measures have the objective of reducing the hazard, i.e. the runoff, by diverting it and channelling it. However, structural measures are mostly planned at a large scale, require substantial investments, long implementation periods and extensive sociopolitical negotiation. As a consequence of this long timeframe, they might turn out to be inadequate, postponed or irreversible (Aerts et al., 2014), and in many cases they prove to be unsuitable for developing countries on economic and financial grounds (Inaoka et al., 2019). Non-structural measures, such as measures at the building scale or small-scale urban rehabilitation measures, however, can provide faster flood risk mitigation, yielding improved adaptability, (Andjelkovic, 2001; Kang et al., 2009), more distributed benefits and, as a result, better governance (Tullos, 2018). Such measures are now widely advocated by governmental and non-governmental agencies in many countries, as specifically suitable to heritage centres (Howard et al., 2017). Other non-structural measures, such as financial incentive and insurance, are not investigated in this study, as there is insufficient evidence of their implementation in the study area (Roslan et al., 2019).
Studies specific to Malaysia have shown that rapidly increasing flood events in recent decades are due to unrestrained occupation of rivers by human activities, destruction of forest and extreme weather events caused by climate change (Aliagha et al., 2015). Statistics show an average of 143 floods per year since 2001, of which more than 90 % are flash floods (Anip and Osman 2017). Such frequently occurring floods cause a high level of threat to Malaysian citizens' personal safety and property, thereby inflicting considerable damage to the country's infrastructure (Nasiri and Shahmohammadi-Kalalagh, 2013). Data from the United Nations Office for Disaster Risk Reduction (UNDRR) Country Disaster and Risk Profile (Preventionweb 2019) show for Malaysia that floods account for 98 % of average annual loss in the period from 1990 to 2014. A report from the Malaysian Department of Irrigation and Drainage (2003) identified an average of 29 000 km2 or 9 % of the country's total land area and more than 4.82 million people (22 % of the population) as affected by flooding every year. The annual losses were evaluated at RM 915 million (DID, 2003). At the beginning of the millennium an integrated flood management strategy was launched, whereby the Malaysian government invested in some major structural measures, along with non-structural measures and community participation (DID, 2003). In terms of urban flood mitigation, among the structural measures, the most conspicuous intervention is certainly the SMART (Stormwater Management and Road Tunnel) project, aimed at alleviating the flooding problem in the city centre of Kuala Lumpur caused by the Klang River, as well as reducing traffic congestion (Abdullah, 2004). The SMART project is a flood diversion measure, realized as a tunnel bypass, diverting catchment discharge from the Klang Basin. Among the non-structural measures the government has also invested in flood detection and warning systems, awareness campaigns, and flood-proofing guidelines for buildings with basements (DID, 2006, 2008). The effect of the SMART tunnel on the flood risk of the studied area is analysed in this study (see Sect. 2.2 and 3.3).
Notwithstanding this proactive approach, the “Malaysia Disaster Management Reference Handbook 2019” states that
Annually, floods account for the most frequent and significant damage, with 38 damaging events in the last 20 years, and are responsible for a significant number of humans lives lost, disease epidemics, property and crop damage, and other losses.
The handbook also points out that risk of floods has increased due to climate change, stating that “Malaysia had the highest percentage of the population (67 %) exposed to floods among ASEAN (Association of Southeast Asian Nations) member states between July 2012 and January 2019” (see CFE-DMHA, 2019, p. 22). With six major events in the last 5 years, flooding remains a major source of risk and losses in Malaysia, with a dramatic 3-fold increase in population exposure in 2 decades. While the Malaysian government has officially adopted a holistic approach to flood risk reduction from preparedness to post-event relief, its implementation has received critical reviews by several researchers (Shafiai and Khalid, 2016).
Flood vulnerability refers to the susceptibility of goods and people in any region to suffer damage and losses. An accurate assessment of such vulnerabilities is essential to devise effective flood risk management (Rehman et al., 2019). Vulnerability assessment studies, focusing on different scales (Kundzewics et al., 2019) and different dimensions (Rehman et al., 2019), have demonstrated the capability of predicting socio-economic damage and risk by floods. In an urban context, flood vulnerability assessment of individual buildings, and the management of the associated risk, has also proven to be an effective way to increase the flood resilience of the whole city (Stephenson and D'Ayala, 2014; Aerts et al., 2014). Two approaches are common in flood vulnerability assessment, the physical approach and empirical approach (Balica et al., 2013). Physical approaches use hydrological models to estimate the flood hazard and compute economic consequences for a particular event or area on the basis of a damage index relating a measure of intensity of the flood to the associated economic loss. Parametric or empirical approaches use a set of quantitative or qualitative indicators to rate the vulnerability of a building or area, with no particular reference to the hazard intensity.
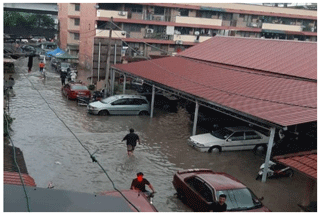
Figure 1Pluvial flood in Kampung Baru, 1 October 2019. Due to poor drainage, water depth of 1 m was reached after 2 h of rain (BERNAMA, 2019).
The present study is part of “Disaster Resilient Cities: Forecasting Local Level Climate Extremes and Physical Hazards for Kuala Lumpur”, an interdisciplinary 3-year project developed through a partnership of UK and Malaysian academia, industry and local government institutions, supported by UKRI, Innovate UK and the Malaysian Industry-Government Group for High Technology (MIGHT). The flood risk to traditional heritage houses in Kuala Lumpur, identified as one of the major contributors to disaster losses in Malaysia (Bhuiyan et al., 2018), is studied by adopting a hybrid approach using a hydrological model to determine the flood hazard and a set of indicators to determine the vulnerability of individual buildings. However, the present model does not compute the mechanical response of the building envelop to water pressure (Custer and Nishijima, 2015).
Two different types of flooding are considered, pluvial flash flooding, caused by thunderstorms characterized by localized rainfall of very high intensity and short duration, and fluvial flooding, caused by monsoonal-type long-duration and low-intensity rainfall over a large area of the catchment. For both types of flood, the expected depths are computed for a reference 0.1 % annual exceedance probability (AEP). To determine the actual risk the present study uses a multiscale approach to assess the vulnerability of traditional houses in Kampung Baru (Fig. 1), thus providing evidence to suggest appropriate mitigation strategies at individual building, local compound and district scales. The empirical vulnerability model used is particularly suitable for studies at the micro- to mesoscale levels, aiming at identifying effective non-structural mitigation measures. It relies on a number of quantifiable and qualitative parameters which allow the identification of construction typologies typical of the district, with diverse vulnerability level. The local elevation around the building footprint and its position with respect to any river courses are also recorded. By conducting on-site and virtual surveys, the parameters that influence vulnerability can be determined and quantified, and the economic losses due to flood hazards can be estimated, allowing the production of maps which identify a ranking of risk at the building and district scales, for a given hazard type. The hazard magnitude used is water depth, calculated by developing 2-D hydrodynamic models to simulate the behaviour of water conveyed by overland flow and river systems in response to rainfall events of different frequencies and intensities. A damage function of generic applicability is developed to compute the economic losses at individual building and at sample levels, considering both envelop and content damage and the loss of value associated with the heritage character.
2.1 Study area
The Kampung Baru district is located in the central area of Kuala Lumpur enclosed between the Klang River in the south-east and the Sungai Bunus in the north-west (Fig. 2a). Kampung Baru is a historic Malay agricultural settlement dating back more than 100 years, spread over 100 ha and home to approximately 19 000 residents. While having witnessed the development of the city, and being currently under pressure of gentrification, this area, which has protected status, still contains a unique building style, retaining the characteristics of both traditional Malay architecture and the ethnic Malay lifestyle. Given its setting and local topography, Kampung Baru is prone to both river flooding and flash floods, partly due to the poor drainage system (Menon, 2009; Bernama, 2019) (see Fig. 2).
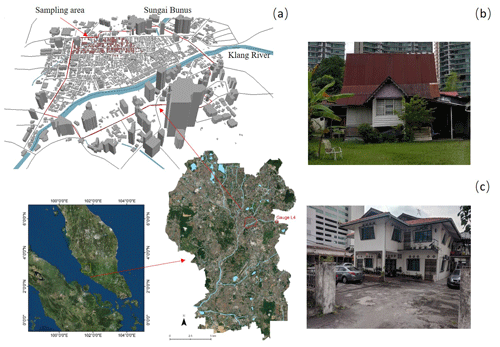
Figure 2(a) Location of Kampung Baru in the centre of Kuala Lumpur (ESRI ArcGIS® base map); (b) traditional vernacular house; (c) modern vernacular house.
Ju et al. (2012) recorded 121 traditional vernacular Malay houses, still inhabited by Malay people, in Kampung Baru area. These represent an important cultural and architectural heritage as well as being a touristic attraction and hence representing an important economic resource to the Malay community. Although these houses might have been altered in time, in terms of materials and form, they still maintain two substantial characteristics related to the local environmental conditions: a steep sloping roof and a floor raised on stilts (Fig. 2b). These two iconic architectural features protect the space within from high-intensity precipitation and frequent flooding, rendering these houses intrinsically resilient to the Malay climate.
Examples of buildings on stilts in the area of study are shown in Fig. 3. Earlier constructions are characterized by buildings on short timber stilts (Fig. 3a). In some cases, the space below is enclosed by timber grids (Fig. 3b). In wealthier construction, the stilts might have been made of stone (Fig. 3c) and in modern construction the stilts have been transformed into an open ground-floor storey (Fig. 3d) to accommodate car parking, endorsed by the Department for Irrigation and Drainage Malaysia as a non-structural flood mitigation measure.
2.2 Flood hazard mapping
Hazard maps showing flood extent and water depth associated with different types of flooding across Kuala Lumpur were developed within the project for a range of return periods. The maps provide water depth for pluvial flooding (also known as flash floods) and for fluvial (riverine) flooding. For fluvial flooding, two scenarios are mapped: an undefended scenario where no mitigation measures (river flood defences) are accounted for and a scenario where the flood protection offered by SMART (see Sect. 1) is incorporated.
The maps were developed by analysing time series data from a selection of rain and river gauges across the Klang Basin to calculate rainfall intensity hyetographs and river hydrographs for return periods of 20, 50, 100 and 200 years. The rainfall intensity and river flows were used as input for 2-D hydraulic modelling using JBA's proprietary JFlow® software (Lamb et al., 2009) to provide estimated depths of inundation. The methods used to calculate the rainfall hyetographs and river hydrographs are described in Sect. 2.2.1. An important input to the flood mapping process is a digital terrain model (DTM). For this study, a 0.5 m resolution bare-earth DTM was provided by the Civil Engineering and Urban Transportation Department, KL city hall and City Planning Department, resampled to 5 m resolution. This scale is commensurable with the size of individual buildings.
JFlow® can be run in different configurations for different purposes. For large rivers, a fluvial model configuration is used to apply hydrographs to the model at regularly spaced inflow points along the drainage network. The volume of water that can be held within the river channel is estimated and removed from the flood simulation. A JFlow® simulation is run for each return period using a solver based upon the two-dimensional shallow water equations. For the SMART scenario a discharge-limited directional culvert is constructed in the JFlow® model to represent the diversion and storage of flood water between Kampung Berembang and the Desa Lake at Salak South and is adjusted for each of the four SMART operational modes as explained in Table 1.
Table 1Parameters of four SMART operational modes.
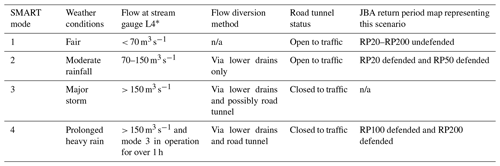
* L4 gauge is situated at the confluence of the Upper Klang and Ampang rivers. n/a stands for not applicable.
For small rivers and pluvial flooding, a direct-rainfall configuration is used. This approach applies the relevant hyetographs to each cell of the DTM. Different runoff and drainage rates are applied to reflect spatial variations in soil type and land cover. Urban drainage systems can be accounted for by removing a proportion of the total rainfall volume prior to running the JFlow® simulation. However, in this study, no such adjustments were made as there was insufficient evidence to support quantification of urban drainage capacity across the city. Water depth in metres is calculated for each flood type (pluvial, fluvial and fluvial with SMART defence) and return period (20, 50, 100 years) and recorded in a set of GeoTIFF raster files for use in Geographical Information Systems (GIS). In this study, flood maps of three flood types for the 100-year return period are used in the estimation of flood hazard and risk, as this is a widely used return period in communication and decision making in flood risk prevention and management.
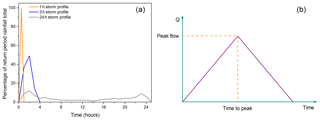
Figure 4(a) Storm profiles used in current flood modelling; (b) schematic diagram of the river hydrograph shape.
Calculation of rainfall hyetographs and river hydrographs
Rainfall totals (in millimetres) were calculated at 11 rain gauge stations within a 6 km radius of the centre of Kuala Lumpur. This was done by extracting peak-over-threshold values from the hourly rainfall record at each gauge and fitting them to a generalized Pareto distribution, to enable return period rainfall totals to be estimated for each gauge. This was done separately for the 1, 3 and 24 h storm durations. Spatial interpolation was then used to convert the estimates at the gauge stations into a set of continuous rainfall surface rasters across the entire study area, providing a rainfall total (mm) for each return period and storm duration on a 110 m × 110 m grid. Each gridded rainfall total was converted into a hyetograph to describe the temporal distribution of the rainfall for each of the three storm durations. Normalized rainfall profiles were developed by analysing hourly rainfall data for 20 events between 1997 and 2016 and calculating a mean 3 h storm profile and a mean 24 h storm profile across all stations. Due to the lack of sub-hourly rainfall data, the 1 h storm profile was assumed to be a simple triangular shape. The storm profiles are illustrated in Fig. 4a below.
River hydrographs were calculated at 2 km intervals along the river network of the study area. Each hydrograph was constructed using a linear function, defined by peak flow and time-to-peak estimates. More advanced methods for deriving the shape of hydrographs are available, but in all but exceptionally flat topographies peak flow can be considered the key variable in hydrograph shape, so for this study a generalized triangular profile was considered appropriate. Firstly, peak flow was calculated at 10 streamflow gauges within the Klang River basin, using non-stationary flood frequency analysis. These values were then regionalized using a linear regression equation for each return period, enabling peak flow to be estimated at all ungauged locations within the study area, based on their catchment area (in square kilometres).
The time to peak at each gauge was calculated by extracting the median time to peak from all discrete flood events recorded at the seven streamflow gauges with hourly flow records available. A linear regression equation was used to estimate time to peak at all ungauged locations within the study area, which correlated time to peak (hours) to catchment area (km2). Figure 4b shows a schematic diagram of the river hydrograph shape. Although the time to peak is not directly relevant to the vulnerability assessment of buildings, it is a necessary step in constructing hydrographs, which are needed to generate the hazard maps for different return periods.
2.3 Data collection
Given the multiscale approach adopted for the assessment of the flood risk in Kampung Baru, data are obtained from multiple sources. A 3-D building dataset and 0.5 m resolution DEM dataset were provided by the UKM Southeast Asia Disaster Prevention Research Initiative (based on the 2013 lidar dataset from the KL city hall). These have been visualized in ArcMap 10.3 (Esri) and manipulated to extract data on building position, footprint and position of the building's base relative to the road. This information is essential to determine the depth of water at a particular building perimeter, given a flood depth at the site. Other data were collected from a field survey and Google Street View (Google, 2019). A preliminary overview of all buildings in the targeted area of Kampung Baru was completed on Google Street View (GSV), to identify the most interesting sector in the district and proceed to an initial screening of the buildings' typologies present and the identification of critical parameters to best target the field survey. The field survey of Kampung Baru was conducted in July 2018, to gather specific data relative to individual buildings. Critical parameters, difficult to identify from the GSV, such as the location and dimensions of the drainage system, were typologically classified and measured on site, along with other geometric parameters. A thorough photographic survey was also conducted at this stage, taking shots for all visible and accessible elevations of sample buildings, as well as larger overview shots of the whole study area. Specific features aimed at mitigating flood damage were also observed and recorded during the field survey.
After detailed data were taken on a small sample of buildings during the field survey, which also allowed for identification of buildings' typologies, a further survey based on Google Street View (GSV) was undertaken to gather additional data and cover a sample of buildings in excess of 160. This procedure was successfully used by one of the authors to survey buildings to determine vulnerability and damage in post-earthquake reconnaissance (Stone et al., 2017, 2018), and it is increasingly used to produce exposure databases in an expedient and economic manner (Pittore et al., 2018). In GSV, a continuous series of 360∘ panoramas, created by sewing multiple overlapping photos together to display the real portrayal of a specific location (Street View, 2018), were observed according to the location and the time when the photos were captured. In Kampung Baru images were collated in three different years of survey, 2013, 2015 and 2017. In this study the latest version was chosen, and a full front view of a target building could be accessed online through the observation points located on each street. During this survey, the qualitative parameters were collected visually, replicating the field survey procedure. For quantification of other parameters, such as height of door threshold and windowsills, measured samples from the field survey were used as a reference to apply a measure of scale.
2.4 Vulnerability model
Research on flood vulnerability and risk assessment encompasses a wide range of methods and focuses (Rehman et al., 2019). In an urban context a substantial component of losses is ascribable to physical damage to vulnerable buildings and their contents (Chen et al., 2016). Current flood risk assessment studies and damage models use either an empirical approach, relying on post-event damage data collection to determine vulnerability functions, or synthetic approaches, whereby the vulnerability functions are based on expert opinion. Empirical methods are basin or catchment specific (Merz et al., 2010) and hence of limited transferability and applicability to other locations without substantial calibration. Synthetic models are more adaptable spatially and temporally; however, they are often based on a single variable relating flood depth to economic loss, possibly mediated by building type (e.g. HAZUS-MH, FEMA, 2013). Dottori et al. (2016) present one of the few synthetic flood damage models based on a component-by-component analysis of direct damage, correlating each damage component to different flood actions and specific building characteristics. The damage functions are designed using an expert-based approach validated on loss adjustment studies and damage surveys carried out for past flood events.
Historic data on flood damage and insured losses are not available for Kuala Lumpur or Kampung Baru. It is increasingly recognized that models need to account for multiple scales, from a single asset to the full catchment area, and be able to consider many variables, in terms of both hazard intensity and asset response (Amadio, 2019). Such models may rely on sophisticated physical modelling of the flood event, while hazard–damage correlations are then determined using artificial neural networks or random forest analysis of past damage data (e.g. Merz et al., 2013; Carisi et al., 2018), or Bayesian networks (Vogel et al., 2014). For the majority of these models, however, while hazard and exposure are treated to a high level of resolution, the individual building's vulnerability descriptors are limited in number and often of a qualitative nature. Papathoma-Köhle et al. (2019) suggest a method for the vulnerability indicator selection that relies on data from systematically documented torrential events to select and weigh critical indicators using an algorithm based on random forest. Although Kelman and Spence (2003), Custer and Nishijima (2015), Hebert et al. (2018), and Milanesi et al. (2018) have used mechanical approaches to determine the structural capacity of individual masonry walls to withstand water pressure and derive vulnerability functions which correlate physical damage to depth of water, such physical models have not so far found direct application at the urban scale.
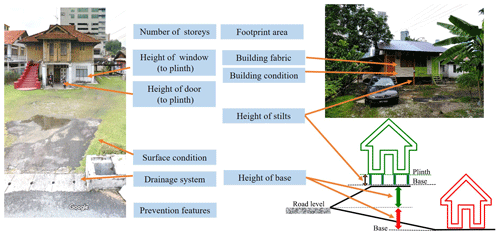
Figure 5Example of traditional buildings in Kampung Baru and indication of the vulnerability index parameters.
In the present study, the PARNASSUS v.3 procedure, based on a vulnerability index approach, is applied to determine the relative vulnerability of individual buildings. The building and its immediate curtilage are here defined as the system exposed to the flood hazard. Therefore, the vulnerability index is obtained by identifying a number of parameters which are considered all equally critical to the response of the system, ranging from its characteristics to its surrounding conditions. The parameters used in the present study for characterizing the building vulnerability are adapted from studies conducted by one of the authors on historic buildings in the UK (Stephenson and D'Ayala, 2014) and the Philippines (D'Ayala et al., 2016). Parameters such as number of storeys and footprint provide indications on the volume of the building, its contents and the bearing pressure on the ground. This has implications for soil failure and subsidence following floods, which could write off the building, hence outweighing the lower proportion of exposure of the total volume of the building, usually assumed for multistorey buildings. This is particularly relevant for the long-term flooding scenarios. Other descriptors such as height of the base, the stilts, the door threshold and the windowsills allow the estimation of vulnerability to water breach in relation to flood depth. Finally, building fabrics and building condition provide a measure of the permeability of the building construction materials and their likelihood to deteriorate when exposed to water. In addition to these building-specific parameters, a classification of drainage systems in the immediate setting of the buildings, of the surface conditions surrounding the building and of any local flood prevention measures are also included as vulnerability indicators. This is because typically flood hazard models, although they take account of these parameters at the urban scale, by assuming certain land uses and generic drainage rates, they do not capture the local differences at the building scale. In this specific case study, as there is no sufficient knowledge of the drainage system at the city scale, such data become a critical indicator of vulnerability at the local scale, and one that can be directly surveyed on site. The full list of parameters is shown in Fig. 5 and Table 2. The attributes for each parameter and the rating scheme adopted are described in the next section.
2.5 Vulnerability ratings
For each parameter a range of attributes varying between three and five is determined through logical derivation of the maximum possible number of responses, and these are assigned a vulnerability rating (VR) on a scale from 10 to 100. Qualitative parameters have three attributes, and quantitative parameters have four or five attributes to ensure important measurement thresholds affecting the building's vulnerability are captured. The scale is divided into equal, unweighted parts according to the number of attributes, with the attribute indicating lowest vulnerability assigned the value 10 and the one indicating the highest assigned the value 100, as shown in Table 2, following the PARNASSUS v.1 procedure (Stephenson and D'Ayala, 2014). For instance, the parameter “drainage system” has three possible outcomes: “good”, “poor” and “no”, so that the numerical rating among these three outcomes can be assigned as 10, 55 and 100, to represent the increase in vulnerability. Table 3 summarizes each parameter range of attributes and its conversion into a vulnerability rating. The surface condition consists of three sub-parameters, and the building fabric consists of two sub-parameters. In both cases, the vulnerability rating is calculated as the average ratings of the sub-parameters.
Table 3Parameters' attributes and corresponding vulnerability rating in PARNASSUS v.3.
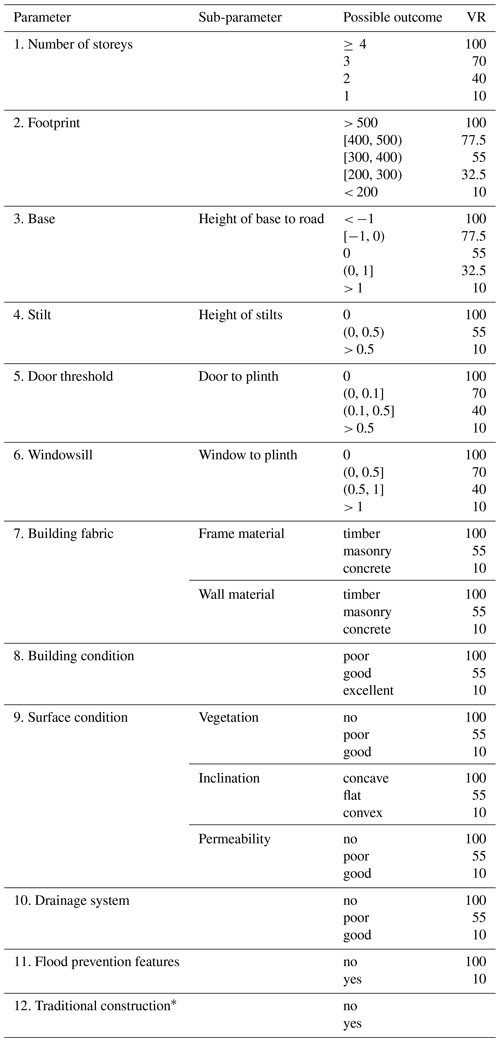
* Factor used in Eq. (6).
Hence for each building and for each parameter a vulnerability rating VRij, can be defined, whereby i, ranging from 1 to 163, denotes the building ID, and j, ranging from 1 to 11, denotes the parameter under consideration. The vulnerability index VIi for each building is therefore computed by summation of the vulnerability rating for each parameter:
The vulnerability index for each building can range from a minimum of 110 for lowest vulnerability to a maximum of 1100 for the highest vulnerability. To compare the cumulative frequency of each parameter and its relevance to the VIi, a normalized vulnerability rating of each parameter nVRij and the total vulnerability index nVIi are calculated based on Eqs. (2) and (3).
Here the normalization is with respect to the mean value of the scoring range and . This normalization also allows comparison among different samples of buildings at different sites.
To further analyse the data, buildings are grouped in four classes by dividing the vulnerability range in four equal parts: very low vulnerability (0.1, 0.325 ⋅ VImax), low vulnerability (0.325 ⋅ VImax, 0.55 ⋅ VImax), high (0.55 ⋅ VImax, 0.775 ⋅ VImax) and very high (0.775 ⋅ VImax, VImax).
In this study, the VIi values of the surveyed buildings are concentrated in the middle two categories. To refine the classification, the low-vulnerability and high-vulnerability categories are further divided into two equal parts: low (0.325 ⋅ VImax, 0.4375 ⋅ VImax), medium low (0.4375 ⋅ VImax, 0.55 ⋅ VImax); medium high (0.55 ⋅ VImax, 0.6625 ⋅ VImax) and high (0.6625 ⋅ VImax, 0.75 ⋅ VImax).
To determine the relative contribution of each parameter to the highest and lowest vulnerability index scores, rVRj is calculated based on Eq. (4):
where j denotes the parameter considered, k denotes the number of buildings in a given vulnerability class and i is the total number of buildings surveyed.
2.6 Economic loss
The vulnerability index VIi derived in the previous section is a suitable measure to provide a scale of criticalities for particular properties in need of attention to improve their flood resilience. However, interventions and investments, whether at the individual property-owner level or at the level of the council or district authorities, are usually justified on the basis of cost-benefit analysis. Typically, this is expressed in terms of a replacement cost function which quantifies the damage in monetary value and relates it to a measure of the flood intensity, such as flood depth (Pistrika et al., 2014). The computation of the economic losses caused by flood events includes different components, which can be classified as tangible costs, including the physical damage to the building and contents, interruption of work, etc., and other intangible costs, such as loss or damage to objects with sentimental or cultural value, difficult to quantify (Kreibich et al., 2014). The economic loss model proposed in this study considers the physical damage to each building and its contents as it can be estimated on the basis of its specific vulnerability (see Sect. 2.5) and a normalized damage factor D(hi) expressed as a function of the flood depth. Two different damage factors Db(hi) and Dc(hi), for the building and contents, respectively, are used in the present study.
The physical damage to individual buildings can be calculated as the total replacement cost Ei:
where i indicates the building identifier and C, D, FVR and AT are the construction cost per unit area of building, the damage factor, the vulnerability factor and the surface area of the building directly affected by the flood, respectively. They are derived as follows.
2.6.1 Building cost
The replacement cost of buildings C(i) includes two parts, the replacement cost of the building CB(i) and the replacement cost of contents CC(i):
where C0(i) is the estimated construction cost in the study area depending on building type and materials, FB(i) is a value factor depending on the perceived value of the building, and FH(i) is a value factor depending on the historic and cultural status of the building. The value factor FB can be used to account for the depreciated cost, i.e. the current remaining value, rather than the replacement value (Huizinga et al., 2017). However, as several of the buildings in the study area are either historic or traditionally built, neither the depreciated cost nor replacement cost might be appropriate to account for their cultural value. Arcadis (2019) uses a range from RM 2415 to 4105 (EUR 525 to 890) per square metre to compute the basic construction cost C0(i) of a detached house in Kuala Lumpur. This value includes the construction and services (electric, hydraulics and mechanical) costs. In this study the building fabric material (timber, masonry, concrete) is used to determine the low-, medium- and high-cost ranges, while the building condition (poor, good and excellent) is used to determine the values of the adjustment factor FB = (0.4, 0.7, 1). If the building is among the ones identified as of traditional construction by Ju et al. (2012), or listed as being of historic value in this study survey, a factor of FH(i)=1.3 is applied to account for the additional cultural value as a touristic attraction.
Replacement cost for damage suffered by contents is also a non-negligible component of the total loss suffered by buildings affected by floods. Huizinga et al. (2017) and FEMA (2013) assume that the replacement cost of content typically ranges between 40 % and 60 % of the building cost for residential properties. However, studies at the microscale (Appelbaum, 1985; Oliveri and Santoro, 2000) show that the proportion of content cost to structure cost also depends on type and quality of construction, level of household income, etc. with a range from 15 % to 60 %. Therefore, the content cost can be expressed as
where kc assumes values in the range 0.15–0.60, which is also determined according to the building condition in this study.
Finally, combining the building replacement cost CB(i) and the content replacement cost CC(i) provides the total replacement cost for each building.
2.6.2 The flood depth–damage ratio function D(hi)
D(hi) is a function of the water depth hi, which in this study is computed as the differential at each building site between the inundation depth FDi computed by the flood hazard model and the elevation of the building plinth above ground, i.e. the height of the stilts (or other structure raising the plinth) HSi.
Depth–damage ratio functions specific for Malaysia or Kuala Lumpur do not exist in literature, as data on losses from past events have not been systematically collected and analysed to date, notwithstanding the frequency of these, even just in the last decade (Romali et al., 2018). The derivation of synthetic depth–damage functions relies on appropriate exposure databases, ad hoc surveys or heuristic information on losses. When conducting studies at the microscale, such as the present one, it is important that the depth–damage ratio function used reflects the damage to single buildings, rather than aggregation at grid cell level or larger, and also reflects the actual response of each single construction to flood. A systematic review of several depth–damage ratio functions produced in literature (Appelbaum, 1985; Lekuthai and Vongvisessomjai, 2001; Dutta et al., 2003; Huizinga et al., 2017; MLIT, 2005; Pistrika et al., 2014; Englhardt, 2019) shows the relevance of parameters such as construction material and quality, number of storeys, conditions, etc. in determining the depth–damage function, leading to a non-negligible variance among the available functions. However, as the proposed vulnerability model discussed in Sect. 2 accounts for these characteristics explicitly in the computation of the vulnerability index VRi for each building, it is appropriate to derive a mean damage ratio function, only dependent on water depth, while the variance due to the building characteristics is accounted for by the vulnerability factor FVR (VRi) in Eq. (5). Figure 6 shows the damage ratio function obtained as a regression from the mean values of several damage functions available in literature, the associated variance for each point in the series and the 95 % confidence bounds. The regression damage function, with a coefficient of determination R2=0.846 (significant at 0.01 level), shows very good correlation with damage functions produced on the basis of actual damage databases, such as the ones proposed by Prettenthaler et al. (2010) not included in the regression sample.
2.6.3 Vulnerability factor FVR
The vulnerability factor FVR(VIi) for each building is computed based on the vulnerability index calculated with Eq. (1) divided by the median value of the distribution of vulnerability indexes in the sample of interest. In this way the replacement cost function is calibrated directly on the local building stock of the study area, while remaining non-dimensional and of generic validity.
2.6.4 Total flooded area of each building At
The total flooded area of each building ATi equals the footprint of the buildings Afi times the number of storeys affected by the flood nfi, which is computed as
where df is the depth of water at the site and hs is the storey height including stilts, where appropriate.
3.1 Vulnerability index of selected buildings
Based on the empirical model described above, the vulnerability rating VRj for each parameter was attributed to each building, and the total VIi was computed. Notwithstanding the relatively small size of the district considered, and the consequent uniformity of building height (mainly two storeys) and footprint, Fig. 7a and b show that the occurrence of each VRj parameter attribute and each VRj cumulative distribution, respectively, are all different, indicating that there is no direct correlation among the parameters chosen to represent the vulnerability of these buildings. Nonetheless, the VIi cumulative distribution shows good agreement with a lognormal function (Fig. 7b), with a coefficient of determination of 0.997 (significant at 0.01 level).
The largest VIi value in the sample is 852.5, and the smallest is 477.5 (Table 4). The distribution of the values normalized with respect to the median is shown in Fig. 8, together with the cumulative distribution. The full normalized range of the VI is divided in four equal intervals, which determine four classes of vulnerability: very low, low, high and very high, as already explained in Sect. 2.5 and shown in Table 4. The classes low and high are further subdivided in low and medium low and medium high and high, respectively. There are no buildings in the extreme classes very low or very high. From Fig. 8 and Table 4 it is evident that the overall distribution of VIi is relatively narrow, with a median greater than the average and the majority of the samples falling in the medium-high-vulnerability class. The low-vulnerability class represents 1.2 % of the sample, and the high-vulnerability class includes 19 % of the buildings. The spatial distribution of the vulnerability index shows a relatively random pattern, without particular alignment to the roads' grid or the relative distance from the river (Fig. 9). This confirms the lack of uniformity of the urban pattern of this district and the importance of assessing the flood vulnerability at the scale of the individual building. As mentioned earlier, the number of storeys and footprint are relatively uniform; hence the curtilage setting and the construction details are really what characterize the variance in vulnerability. This is further explained in the next section.
3.2 Relevance of factors contributing to vulnerability
Given the apparent random spatial distribution of buildings in the high- and low-vulnerability categories, it is worth examining the relevance of the different parameters contributing to the VIi of each building, so that the adverse attributes can be mitigated to reduce risk to flood hazards. For buildings in the bottom and top quintiles of the distribution, as per Eq. (4), the average scoring of each parameter in that category is divided by the average scoring of the same parameter over the whole sample, hence highlighting the parameters that most contribute to the tails of the distribution. This is graphically shown in Fig. 10, where 1 is the normalized value of the mean for each parameter over the whole sample. As there are only two buildings in the low-VI category, another 29 buildings in the lower part of medium-low VI were selected to compare with the 31 high-VI buildings. It is shown that for the high-vulnerability class, poor drainage system and building condition both have a value more than 50 % greater than the average score, representing the most substantial contribution to high values of VIi. The height of the base also contributes to the higher VIi, in accordance with the observation that often houses are built below the road level at a distance from the drainage system and hence are located in concave, undrained settings. This condition is particularly vulnerable in the case of high-intensity and short-duration pluvial floods. Conversely, a good drainage system, presence of stilts on the ground to elevate the plinth height and good building conditions are key parameters in low vulnerability scoring.
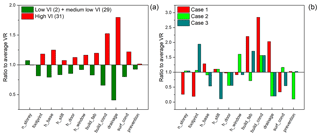
Figure 10Relative values to the average VI for each parameter; (a) for the lower and upper quintiles of the sample; (b) three selected cases as located in Fig. 9.
A further three specific buildings are selected, one located in the eastern part of the district, falling in the high class of VIi; the other two are located in the western region of the district, characterized by a low value of VIi (Fig. 9). For the first case, the parameters that determine the high vulnerability are the lack of stilts, the poor building condition and permeable building materials, the lack of proper drainage and prevention measures, and the setting of the building below the road level, although the curtilage of the building is characterized by permeable and absorbent surface conditions. Topographically, however, the building is set in the highest terrain of the district and hence might be exposed to less hazard than other buildings. On the contrary, for the two low VI cases, although located in the portion of the district at lower topographical elevation and near the river, hence being characterized by high exposure, they are set at the same or higher level as the road or well above. Both have a door threshold set above average, both have good drainage, and finally they either have stilts or good prevention measures, to be overall less vulnerable or, better, more resilient to the flood hazard.
This is a relevant finding, as commonly, for studies at the mesoscale, it is assumed that parameters such as drainage and surface conditions can be assumed to be uniform over an urban block, for instance. In relation to Kampung Baru, the spatial distribution of the results demonstrates that the provision for drainage and permeable ground surfaces might be rather fragmented, even along the same street, in parts owing to plot redevelopments at different times. This further highlights the significance of local-scale prevention to reduce the flood vulnerability and risk.
3.3 Estimation of replacement cost due to different flood scenarios
To estimate the flood damage to buildings, as introduced in Sect. 2.2, three different scenarios are considered: a pluvial flood, a fluvial flood without structural defences and a fluvial flood considering the effect of the SMART tunnel defence (Abdullah, 2004). For all scenarios the reference rainfall with 10 % probability of exceedance in 100 years is considered here, and the extent of flood water for each scenario is presented in Fig. 11a–c, together with the total losses (risk map) associated with Fig. 11d fluvial flood without the SMART system in operation, Fig. 11e fluvial flood with the SMART system in operation and Fig. 11f pluvial flood. The number of buildings flooded and economic loss as a function of water depth at each building are reported in Fig. 12 where the water depth is defined as the difference between height of plinth above ground and inundation depth, which provides a direct measure of the water depth entering the buildings (Eq. 9).
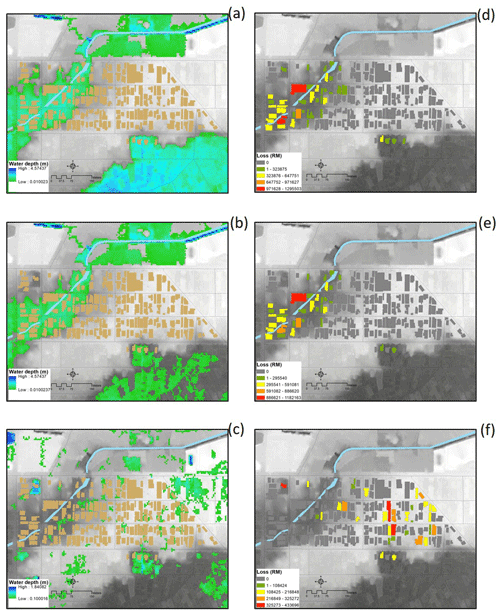
Figure 11Flood maps of different scenarios. (a) River flood without SMART, (b) river flood with SMART, (c) flash flood and the estimated total replacement cost due to river flood without SMART (d), with SMART (e) and under flash flooding (f). All under 100-year return period.
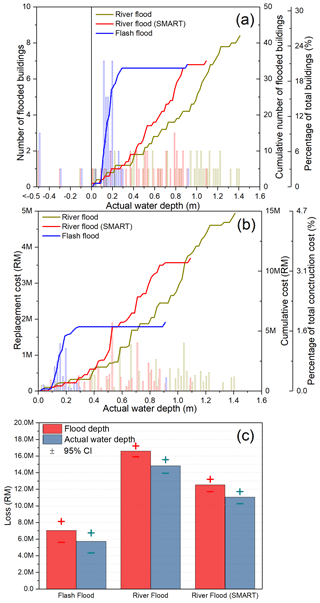
Figure 12Number of flooded buildings (a) and total replacement cost (b) for different flood scenarios. Some buildings with stilts get flooded but have no damage, and hence are reported as having negative actual water depth. (c) The calculated difference in the loss between flood depth and actual water depth.
For fluvial flood, the flooded buildings are mostly located in the west part of the study area, which is close to the Sungai Bunus river. The maximum water depth is around 1.4 m, decreasing to around 1 m with the action of SMART. SMART has a limited effect on flooding extent in the specific area of study, as it mainly operates on the larger Klang River. For the pluvial flood, most buildings are flooded to less than 0.2 m and have a scattered distribution across the study area. Notwithstanding the differences in depth and spatial distribution of the three scenarios, the total number of buildings affected varies little, between 20 % and 24 % of the total number of buildings surveyed in the study area (Fig. 12a). Note that buildings on the south-east portion of the map, close to the Klang River, also suffer fluvial flood; however, these buildings are outside the area of the present study.
The total replacement cost is calculated based on Sect. 2.6. This amounts to around RM 5 M (≈ EUR 1 M) for pluvial flood for the 163 buildings. For river floods, the total cost is considerably higher, around RM 15 M (≈ EUR 3 M) without defence and RM 10 M (≈ EUR 2 M) with SMART in operation. The percentage of cost to the total replacement cost is around 1.6 %, 4.7 % and 3.1 % for pluvial flood, river flood and river flood with SMART, respectively. The majority of economic losses for pluvial flood are concentrated around 0.2 m water depth; for fluvial flood without SMART the majority of losses are concentrated in the range between 0.5 and 1.4 m; finally, for fluvial floods with SMART, losses are distributed mainly around 0.5 to 0.7 m with a maximum of 1.1 m. Figure 12a also shows a number of buildings with negative water depth: these are buildings with stilts, where the flood depth is lower than the position of the plinth above ground, meaning that although the building curtilage gets flooded, this does not affect the building itself. This corresponds to 6 % of the present sample. To emphasize the relevance of the accurate elevation of the point of first breach in the building, i.e. the vertical position of the door threshold with respect to the ground, Fig. 12c shows the difference in total losses for each of the three scenarios considered. The reduction in total losses ranges from a minimum of 13 % for the fluvial flooding with the SMART-activated scenario to a maximum of 20 % for the flash-flooding scenario. Figure 12c also shows the range of variability of the total losses when the 95 % confidence bounds of the damage ratio function are considered.
While major improvements in modelling flood hazard and exposure have been achieved, there is still a lack of compelling evidence on spatio-temporal patterns in vulnerability of societies around the world (Jongman et al., 2015). The South East Asian region is more vulnerable due to the higher population density and higher frequency of rainfall. This study focusses on flood vulnerability of the buildings in a small heritage community, Kampung Baru, in the city centre of Kuala Lumpur, Malaysia. This city has experienced an increasing number of flood events due to the combined effects of observed increasing extreme rainfall referred to as wet–wetter–dry–drier pattern (Allan, 2008, 2010) as well as an increase in urban population, which has nearly doubled since 1980 to the current 1.8 million. As the trends for these two variables are not slowing or reversing, it should be expected in the future that both flood hazard and exposure in this city will continue to increase.
Since buildings are the primary shelter for people, the reduction of their vulnerability is critical in reducing the risk to flood faced by the population. By determining and quantifying the value of vulnerability and risk for each building exposed to specific flooding scenarios, these can be visualized on thematic maps, thus providing evidence to suggest appropriate design or protection strategies specific to each building in the area of study. The present study has identified that higher vulnerability is related to an absence of or poor drainage system, poor building conditions, and poor overall surrounding surface conditions. The buildings with the lowest vulnerability show a combination of good drainage systems and surface condition and/or stilts at the ground floor or other forms of protection. The lognormal vulnerability cumulative function obtained has generic validity and it is a synthetic representation of the vulnerability of the district which can be used at different levels. For building owners, VIi can be used to determine the level of vulnerability of their property and identify features that can be improved to reduce such vulnerability. At the level of the district and with reference to the map as well as to the division in vulnerability classes, it can be seen that buildings belonging to the same class are clustered, meaning that there are local interventions at the scale of a few compounds (such as drainage, surfacing, slope) which can be addressed to reduce such vulnerability. At the municipal level, if this exercise is repeated for different neighbours and districts, then a ranking of them in relation to the mean and dispersion of the VI function can provide support to decision making in terms of non-structural flood defences at the neighbourhood scale. Thus, several possible solutions can be provided to improve the flood vulnerability of buildings in Kampung Baru or similar districts, among which some feasible strategies are as follows.
-
Increasing the ground floor base elevation by either adding pillars or stilts at ground level in new design. The raised floor on stilts is a traditional design of vernacular Malaysian buildings, common of many surveyed cases in Kampung Baru, and such design is being modernized by introduction of an open car park at the bottom of high-rise buildings in Kuala Lumpur. This is considered a soft measure in the Malaysian national flood prevention programme (DID, 2006). Moreover, as the maximum inundation depth due to flash floods for a 100-year return period is around 0.2 m, which is less than the height of most traditional stilts, the stilts are also an effective way to prevent damage from pluvial floods. The present study shows that such a strategy can effectively reduce the flood vulnerability and hence risk for individual buildings. For traditional buildings, which have been altered through time, this feature can be reinstated to restore the traditional character and reduce vulnerability. However, this solution without proper surface treatment and drainage systems may adversely impact neighbouring buildings.
-
Improving the drainage system and surface conditions. Residential buildings which have a proper drainage system or vegetation or permeable surrounding ground surfaces, or alternatively sit on higher ground than the road, ensuring a downward slope from the façade to it, were assessed to be in the low-vulnerability class. These conditions are also reflected in the hazard model by varying the percentage of runoff in each grid, at a 5 m resolution. Improved drainage systems are recognized as an efficient way to improve the flood resilience of residential buildings without altering their traditional or heritage status. As mentioned above, good drainage is essential for the flood resilience to extend from the single-building scale to the urban block to the district.
-
Effectiveness of structural measures. The results obtained highlight that, although the operation of the SMART tunnel can only marginally reduce the spatial extent of the flood and the number of buildings affected, according to the simulation produced in this study, a reduction of about 27 % can be observed in the value of the maximum water depth and of about 50 % in the cumulative value of losses.
Hence a combination of non-structural measures, e.g. use of stilts and proper surface treatment and local drainage, and structural measures, e.g. SMART, appears to be the most effective strategy to increase flood resilience from the building scale to urban scale.
Large major cities in Malaysia, such as Kuala Lumpur, Penang, Petaling Jaya and Shah Alam among others, have been established on floodplains and are increasingly prone to floods and flash floods as they grow in density and area (Chan, 2011). The use of structural measures is currently under consideration to address the issue of flooding associated with further urban development. The findings from the present study offer decision makers an option of increasing building-scale resilience, to make structural measures more effective. This is particularly relevant in historical cities such as Penang, where traditional Malay buildings are prevalent. The combination of structural and non-structural measures is also in line with the aspirations of civil society groups that seek urban resilience within ecological systems (Connolly, 2020) and in line with national and international guidelines on flood prevention damage for historic and traditional buildings.
In this study, a local empirical vulnerability model has been built to evaluate the flood risk to residential buildings in Kampung Baru, Kuala Lumpur. Combining a field survey, Google street view and DEM information, the data of 11 different parameters composing a building level vulnerability model have been collected and scored to rate the flood vulnerability of a sample of 163 buildings. A new economic loss model is developed to quantify the flood risk in terms of replacement cost, considering both specific vulnerability and a normalized depth–damage ratio function. The flood damage and economic loss were then estimated based on the economic loss model under the flood hazards from three different scenarios.
In determining a risk model, a fundamental issue is the level of uncertainty associated with it. In relation to the flood hazard modelling, uncertainty can be identified in the input and the simulation itself. In terms of input, accuracy of water routing is dependent on the DTM accuracy. In the present study a high-resolution DTM (0.5 m resolution lidar) is employed, and checks with aerial imagery and adjustment are made to identify unrealistic flow pathways and amend them. Moreover river locations are defined by analysing the DTM. As a result, the river network may contain false positives; i.e. rivers (and therefore fluvial flood hazard) may be represented in areas where, in reality, there are no streams or watercourses. A second source of input uncertainty is the hydrological input itself, and this is minimized by including in the analysis only gauge data with long and complete records. However it is recognized that gauge data availability in Kuala Lumpur and surrounding areas is poor. Uncertainties in the modelling process arise from two orders of issues: the representation of the flow and the amount of drainage in the model. In relation to the first issue, as each river section is modelled independently, backwater effects at confluences are not represented; furthermore, current individual simulations assume boundary conditions whereby water can exit the model at the downstream boundary, while in reality if the downstream area is also in the flood stage, this assumption is not correct. This is an intrinsic limitation of the current fluvial JFlow® model, and no mitigation has been implemented for this study. In relation to the overall catchment drainage a fundamental epistemic uncertainty is the location of culverts in Kuala Lumpur, which have not been represented in the model. This is not necessarily a conservative assumption as a blocked culvert may locally exacerbate flooding beyond the level expected in an undefended (no culvert) scenario. Finally, the capacity of natural or artificial drainage systems across the study area is represented at a broad scale and does not fully account for site-specific storm drains or other localized features. A detailed land use dataset was combined with soil information and slope to calculate variable percentage runoff rates on a 30 m resolution grid. This resolution is appropriate for the level of detail of the input (land use, soil and slope) information but means that property-level drainage systems cannot be accounted for.
From the perspective of determining the vulnerability, although increasingly the need for micro-level studies is recognized, most published work on flood risk analysis refers to generic building typologies and their incidence on grid cells containing several buildings to characterize the exposure. In this respect the vulnerability model proposed here has two advantages: identifies the vulnerability of each specific asset on the basis of its geometry, material characteristics and level of maintenance, but also in terms of its setting and hydraulic characteristics of its curtilage. This partly compensates for the lack of knowledge on drainage features at the urban scale, from the modelling point of view, but most importantly identifies deficiencies that can be mitigated at the scale of the single property. In developing countries this can become an important tool for communication to stakeholders and community involvement in mitigation strategies, through the mapping and visualization of the vulnerability indicators. The sample used is relatively small, and although the robustness of the rating process has been verified by cross correlating the scoring results of different surveyors, uncertainties on the single buildings are related to the validity of the Google Street View map photo and the accuracy with which measurements can be extracted from such pictures. In order to ensure applicability of the methodology to other locations and to properly calibrate the single parameter's ratings and overall vulnerability classes, larger samples should be studied.
A fundamental source of uncertainties in modelling losses is the choice of an appropriate damage/depth function, and its conversion in monetary terms. The first is usually mitigated by calibrating any model on damage data for historic floods in the area or region and the second by calibrating the replacement cost on insurance claim data. In the present study, both historic damage and insurance claim datasets are not readily available in a format that can be used at this scale and in this context. Therefore, rather than using a single arbitrary damage depth function, a large number of functions derived for building types similar to the ones analysed have been used to obtain a mean damage ratio function by regression. This was then validated by comparison with functions derived by other studies on reach damage datasets. The fact that the damage function is independent of the specific building typology or local exposure model, which are accounted for in the vulnerability model, renders it of generic value and makes it applicable to other situations in Malaysia and worldwide. The economic loss function considers the loss from both the physical damage to each building and its content. The additional cultural value as a touristic attraction was rather crudely accommodated by an arbitrary factor. There is an extensive, but also so far rather inconclusive, debate in literature as to how to compute and quantify the increase in loss associated with the historic value of a property, as it pertains to both its direct and indirect losses. This is an area that should be tackled in future by looking in detail at the additional repairing costs and the loss in revenue from touristic business. The intangible aspects of course deserve a different approach.
Building data were collected from a field survey and Google Street View (https://www.google.com/maps/@3.1636745,101.7056593,16z, last access: October 2019) (Google, 2019). Primary data are strictly used within the project “Disaster Resilient Cities: Forecasting Local Level Climate Extremes and Physical Hazards for Kuala Lumpur”. The data of the research findings are available from the corresponding author (DDA) on reasonable request.
DDA designed the research and analysed the results; KW and YY collected the data, analysed the results and produced the visualization; HS, AM and VP conducted the flood modelling; JJP discussed and extended the findings. All authors discussed the results and drafted the final manuscript.
The authors declare that they have no conflict of interest.
The authors wish to thank Mark Saunders (UCL Department of Space and Climate Physics, Faculty of Maths and Physical Sciences) for providing normalized rainfall profiles and gridded rainfall return levels for the Kuala Lumpur study area, used by JBA to determine the hyetographs for the flood hazard simulations. We would like to thank Marleen de Ruiter and the one anonymous referee for their stimulating and constructive comments.
This research has been supported with funding from the Newton Ungku Omar Fund administrated by Innovate UK and the Malaysian Industry-Government Group for High Technology (MIGHT) for the EPSRC project entitled “Disaster Resilient Cities: Forecasting Local Level Climate Extremes and Physical Hazards for Kuala Lumpur” (EP/P015506/1).
This paper was edited by Sven Fuchs and reviewed by Marleen de Ruiter and one anonymous referee.
Abdullah, K.: Kuala Lumpur: Re-Engineering a Flooded Confluence, Fourteenth Professor Chin Fung Kee Memorial Lecture, Kuala Lumpur, Malaysia, 2004.
Aerts, J. C. J. H., Botzen, W. J. W., Emanuel, K., Lin, N., de Moel, H., and Michel-Kerjan, E. O.: Evaluating Flood Resilience Strategies for Coastal Megacities, Science, 344, 472–474, https://doi.org/10.1126/science.1248222, 2014.
Aliagha, G. U., Iman, A. H. M., Ali, H. M., Kamaruddin, N., and Ali, K. N.: Discriminant factors of flood insurance demand for flood-hit residential properties: a case for Malaysia, J. Flood Risk Manage., 8, 39–51, https://doi.org/10.1111/jfr3.12065, 2015.
Allan, R. P. and Soden, B. J.: Atmospheric warming and the amplification of precipitation extremes, Science, 321, 1481–1484, https://doi.org/10.1126/science.1160787, 2008.
Allan, R. P., Soden, B. J., John, V. O., Ingram, W., and Good, P.: Current changes in tropical precipitation, Environ. Res. Lett., 5, 025205, https://doi.org/10.1088/1748-9326/5/2/025205, 2010.
Amadio, M., Scorzini, A. R., Carisi, F., Essenfelder, A. H., Domeneghetti, A., Mysiak, J., and Castellarin, A.: Testing empirical and synthetic flood damage models: the case of Italy, Nat. Hazards Earth Syst. Sci., 19, 661–678, https://doi.org/10.5194/nhess-19-661-2019, 2019.
Andjelkovic, I.: Guidelines on non-structural measures in urban flood management, International Hydrological Programme (IHP), United Nations Educational, Scientific and Cultural Organization (UNESCO), Paris, 2001.
Appelbaum, S. J.: Determination of Urban Flood Damages, J. Water Res. Pl.-ASCE, 111, 269–283, https://doi.org/10.1061/(Asce)0733-9496(1985)111:3(269), 1985.
ARCADIS: Construction Cost Handbook MALAYSIA 2019, Arcadis (Malaysia) Sdn Bhd, Selangor, Malaysia, 2019.
Ashley, R. M., Balmforth, D. J., Saul, A. J., and Blanskby, J. D.: Flooding in the future – predicting climate change, risks and responses in urban areas, Water Sci. Technol., 52, 265–273, 2005.
Balica, S. F., Popescu, I., Beevers, L., and Wright, N. G.: Parametric and physically based modelling techniques for flood risk and vulnerability assessment: A comparison, Environ. Model. Softw., 41, 84–92, https://doi.org/10.1016/j.envsoft.2012.11.002, 2013.
BERNAMA: Flash flood inundates PKNS shops in Kampung Baru, available at: http://www.bernama.com/en/news.php?id=1773833, last access: October 2019.
Bhuiyan, T., Reza, M., Er, A. C., and Pereira, J.: Direct impact of flash floods in Kuala Lumpur City: Secondary data-based analysis, ASM Sci. J., 11, 145–157, 2018.
Carisi, F., Schröter, K., Domeneghetti, A., Kreibich, H., and Castellarin, A.: Development and assessment of uni- and multivariable flood loss models for Emilia-Romagna (Italy), Nat. Hazards Earth Syst. Sci., 18, 2057–2079, https://doi.org/10.5194/nhess-18-2057-2018, 2018.
CFE-DMHA: Malaysia Disaster Management Reference Handbook 2019, Center for Excellence in Disaster Management and Humanitarian Assistance, Hawaii, USA, 2019.
Chan, N. W.: Addressing flood hazards via environmental humanities in Malaysia, Malays. J. Environ. Manage., 12, 11–22, 2011.
Chen, A. S., Hammond, M. J., Djordjevie, S., Butler, D., Khan, D. M., and Veerbeek, W.: From hazard to impact: flood damage assessment tools for mega cities, Nat. Hazards, 82, 857–890, https://doi.org/10.1007/s11069-016-2223-2, 2016.
Connolly, C.: From resilience to multi-species flourishing: (Re)imagining urban-environmental governance in Penang, Malaysia, Urban Stud., 57, 1485–1501, 2020.
Custer, R. and Nishijima, K.: Flood vulnerability assessment of residential buildings by explicit damage process modelling, Nat. Hazards, 78, 461–496, https://doi.org/10.1007/s11069-015-1725-7, 2015.
D'Ayala, D., Galasso, C., Putrino, V., Fanciullacci, D., Barucco, P., Fanciullacci, V., Bronzino, C., Zerrudo, E., Manalo, M., Fadriquela, C., Regalado, J., Garciano, L., Oreta, A., and Yu, K. K.: Assessment of the Multi-Hazard Vulnerability of Priority Cultural Heritage Structures in the Philippines, in: 1st International Conference on Natural Hazards & Infrastructure, Chania, Greece, 2016.
DID – Department for Irrigation and Drainage: Flood Damage Assessment of 26 April 2001 Flooding Affecting the Klang Valley and the Generalised Procedures and Guidelines for Assessment of Flood Damages, Jabatan Pengairan dan Saliran, Malaysia, 2003.
DID – Department for Irrigation and Drainage: Guidelines on Flood Loss Prevention Measures for Basement Car Parks Department for Irrigation and Drainage, Jabatan Pengairan dan Saliran, Malaysia, 14 pp., 2006.
DID – Department for Irrigation and Drainage: Managing the Flood Problem in Malaysia, Department for Irrigation and Drainage, Kuala Lumpur, Malaysia, 2008.
Dottori, F., Figueiredo, R., Martina, M. L. V., Molinari, D., and Scorzini, A. R.: INSYDE: a synthetic, probabilistic flood damage model based on explicit cost analysis, Nat. Hazards Earth Syst. Sci., 16, 2577–2591, https://doi.org/10.5194/nhess-16-2577-2016, 2016.
Dutta, D., Herath, S., and Musiakec, K.: A mathematical model for flood loss estimation, J. Hydrol., 277, 24–49, https://doi.org/10.1016/S0022-1694(03)00084-2, 2003.
Englhardt, J., de Moel, H., Huyck, C. K., de Ruiter, M. C., Aerts, J. C. J. H., and Ward, P. J.: Enhancement of large-scale flood risk assessments using building-material-based vulnerability curves for an object-based approach in urban and rural areas, Nat. Hazards Earth Syst. Sci., 19, 1703–1722, https://doi.org/10.5194/nhess-19-1703-2019, 2019.
FEMA: Multi-hazard loss estimation methodology HAZUS-MH – Flood Model Technical Manual, Department of Homeland Security, Federal Emergency Management Agency, Mitigation Division, Washington, D.C., 569 pp., 2013.
Google: Google Maps, available at: https://www.google.com/maps/@3.1636745,101.7056593,16z, last access: October 2019.
Herbert, D. M., Gardner, D. R., Harbottle, M., and Hughes, T. G.: Performance of single skin masonry walls subjected to hydraulic loading, Mater. Struct., 51, 97, https://doi.org/10.1617/s11527-018-1222-z, 2018.
Hirabayashi, Y., Mahendran, R., Koirala, S., Konoshima, L., Yamazaki, D., Watanabe, S., Kim, H., and Kanae, S.: Global flood risk under climate change, Nat. Clim. Change, 3, 816–821, https://doi.org/10.1038/Nclimate1911, 2013.
Howard, A. J., Hancox, E., Hanson, J., and Jackson, R.: Protecting the historic environment from inland flooding in the UK: some thoughts on current approaches to asset management in the light of planning policy, changing catchment hydrology and climate change, Hist. Environ. Policy, 8, 125–142, 2017.
Huizinga, J., De Moel, H., and Szewczyk, W.: Global flood depth-damage functions: Methodology and the database with guidelines, EUR 28552 EN, Joint Research Centre, European Commission, Sevilla, Spain, 2017.
Inaoka, M., Takeya, K., and Akiyama, S.: JICA's policies, experiences and lessons learned on impacts of urban floods in Asia, Int. J. Water Resour. D., 35, 343–362, https://doi.org/10.1080/07900627.2018.1444980, 2019.
IPCC: Climate Change 2013: The Physical Science Basis, in: Contribution of Working Group I to the Fifth Assessment Report of the Intergovernmental Panel on Climate Change, edited by: Stocker, T. F., Qin, D., Plattner, G.-K., Tignor, M. M. B., Allen, S. K., Boschung, J., Nauels, A., Xia, Y., Bex, V., and Midgley, P. M., Cambridge University Press, Cambridge, UK and New York, NY, USA, 1535 pp., 2013.
IPCC: Climate Change 2014 – Impacts, Adaptation and Vulnerability: Part A: Global and Sectoral Aspects: Working Group II Contribution to the IPCC Fifth Assessment Report, edited by: Field, C. B., Barros, V. R., Dokken, D. J., Mach, K. J., Mastrandrea, M. D., Bilir, T. E., Chatterjee, M., Ebi, K. L., Estrada, Y. O., Genova, R. C., Girma, B., Kissel, E. S., Levy, A. N., MacCracken, S., Mastrandrea, P. R., and White, L. L., Cambridge University Press, Cambridge, 1140 pp., 2014.
Jacobson, C. R.: Identification and quantification of the hydrological impacts of imperviousness in urban catchments: A review, J. Environ. Manage., 92, 1438–1448, https://doi.org/10.1016/j.jenvman.2011.01.018, 2011.
Jha, A. K., Bloch, R., and Lamond, J.: Cities and flooding: a guide to integrated urban flood risk management for the 21st century, World Bank, Washington, D.C., 638 pp., 2012.
Jongman, B., Winsemius, H. C., Aerts, J. C. J. H., Coughlan de Perez, E., van Aalst, M. K., Kron, W., and Ward, P. J.: Declining vulnerability to river floods and the global benefits of adaptation, P. Natl. Acad. Sci. USA, 112, E2271, https://doi.org/10.1073/pnas.1414439112, 2015.
Ju, S. R., Omar, S., and Ko, Y. E.: Modernization of the Vernacular Malay House in Kampong Bharu, Kuala Lumpur, J. Asian Archit. Build., 11, 95–102, https://doi.org/10.3130/jaabe.11.95, 2012.
Kang, S.-J., Lee, S.-J., and Lee, K.-H.: A study on the implementation of non-structural measures to reduce urban flood damage, J. Asian Archit. Build., 8, 385–392, 2009.
Kelman, I. and Spence, R.: A limit analysis of unreinforced masonry failing under flood water pressures, Masonry Int., 16, 51–61, 2003.
Kreibich, H., van den Bergh, J. C. J. M., Bouwer, L. M., Bubeck, P., Ciavola, P., Green, C., Hallegatte, S., Logar, I., Meyer, V., Schwarze, R., and Thieken, A. H.: Costing natural hazards, Nat. Clim. Change, 4, 303–306, https://doi.org/10.1038/nclimate2182, 2014.
Kundzewicz, Z. W., Kanae, S., Seneviratne, S. I., Handmer, J., Nicholls, N., Peduzzi, P., Mechler, R., Bouwer, L. M., Arnell, N., Mach, K., Muir-Wood, R., Brakenridge, G. R., Kron, W., Benito, G., Honda, Y., Takahashi, K., and Sherstyukov, B.: Flood risk and climate change: global and regional perspectives, Hydrolog. Sci. J., 59, 1–28, https://doi.org/10.1080/02626667.2013.857411, 2014.
Kundzewicz, Z. W., Su, B., Wang, Y., Wang, G., Wang, G., Huang, J., and Jiang, T.: Flood risk in a range of spatial perspectives – from global to local scales, Nat. Hazards Earth Syst. Sci., 19, 1319–1328, https://doi.org/10.5194/nhess-19-1319-2019, 2019.
Lamb, R., Crossley, M., and Waller, S.: A fast two-dimensional floodplain inundation model, P. I. Civil Eng.-Wat. M., 162, 363–370, https://doi.org/10.1680/wama.2009.162.6.363, 2009.
Lekuthai, A. and Vongvisessomjai, S.: Intangible flood damage quantification, Water Resour. Manage., 15, 343–362, 2001.
Liao, K.-H.: A theory on urban resilience to floods – a basis for alternative planning practices, Ecol. Soc., 17, https://doi.org/10.5751/ES-05231-170448, 2012.
MENON: Thousands caught unawares as 2 m-high flash floods hit KL, available at: https://www.thestar.com.my/news/nation/2009/03/04/thousands-caught-unawares-as-2mhigh-flash-floods-hit-kl (last access: October 2019), 2009.
Merz, B., Kreibich, H., Schwarze, R., and Thieken, A.: Review article “Assessment of economic flood damage”, Nat. Hazards Earth Syst. Sci., 1697–1724, https://doi.org/10.5194/nhess-10-1697-2010, 2010.
Merz, B., Kreibich, H., and Lall, U.: Multi-variate flood damage assessment: a tree-based data-mining approach, Nat. Hazards Earth Syst. Sci., 13, 53–64, https://doi.org/10.5194/nhess-13-53-2013, 2013.
Milanesi, L., Pilotti, M., Belleri, A., Marini, A., and Fuchs, S.: Vulnerability to Flash Floods: A Simplified Structural Model for Masonry Buildings, Water Resour. Res., 54, 7177–7197, https://doi.org/10.1029/2018wr022577, 2018.
Min, S. K., Zhang, X. B., Zwiers, F. W., and Hegerl, G. C.: Human contribution to more-intense precipitation extremes, Nature, 470, 378–381, https://doi.org/10.1038/nature09763, 2011.
MLIT: Manual for Economic Evaluation of Flood Control Investment (Draft), Ministry of Land, Infrastructure, Transport and Tourism, Tokyo, Japan, 65 pp., 2005.
Mohd Anip, M. H. and Osman, S.: Flash Flood Forecasting and Warning in Malaysia, in: Steering Committee Meeting of the Southeastern Asia – Oceania Region Flash Flood Guidance, Jakarta, Indonesia, 2017.
Mysiak, J., Surminski, S., Thieken, A., Mechler, R., and Aerts, J.: Brief communication: Sendai framework for disaster risk reduction – success or warning sign for Paris?, Nat. Hazards Earth Syst. Sci., 16, 2189–2193, https://doi.org/10.5194/nhess-16-2189-2016, 2016.
Najibi, N. and Devineni, N.: Recent trends in the frequency and duration of global floods, Earth Syst. Dynam., 9, 757–783, https://doi.org/10.5194/esd-9-757-2018, 2018.
Nasiri, H. and Shahmohammadi-Kalalagh, S.: Flood vulnerability index as a knowledge base for flood risk assessment in urban area, J. Novel Appl. Sci., 2, 269–272, 2013.
Neumann, B., Vafeidis, A. T., Zimmermann, J., and Nicholls, R. J.: Future Coastal Population Growth and Exposure to Sea-Level Rise and Coastal Flooding – A Global Assessment, Plos One, 10, e0118571, https://doi.org/10.1371/journal.pone.0118571, 2015.
Oliveri, E. and Santoro, M.: Estimation of urban structural flood damages: the case study of Palermo, Urban Water, 2, 223–234, https://doi.org/10.1016/S1462-0758(00)00062-5, 2000.
Pall, P., Aina, T., Stone, D. A., Stott, P. A., Nozawa, T., Hilberts, A. G. J., Lohmann, D., and Allen, M. R.: Anthropogenic greenhouse gas contribution to flood risk in England and Wales in autumn 2000, Nature, 470, 382–385, https://doi.org/10.1038/nature09762, 2011.
Papathoma-Köhle, M., Schlögl, M., and Fuchs, S.: Vulnerability indicators for natural hazards: an innovative selection and weighting approach, Sci. Rep.-UK, 9, 1–14, 2019.
Pistrika, A., Tsakiris, G., and Nalbantis, I.: Flood depth-damage functions for built environment, Environ. Process., 1, 553–572, 2014.
Pittore, M., Haas, M., and Megalooikonomou, K. G.: Risk-Oriented, Bottom-Up Modeling of Building Portfolios With Faceted Taxonomies, Front. Built Environ., 4, 41, https://doi.org/10.3389/fbuil.2018.00041, 2018.
Prettenthaler, F., Amrusch, P., and Habsburg-Lothringen, C.: Estimation of an absolute flood damage curve based on an Austrian case study under a dam breach scenario, Nat. Hazards Earth Syst. Sci., 881–894, https://doi.org/10.5194/nhess-10-881-2010, 2010.
PreventionWeb: Basic Country Statistics and Indicators, available at: https://www.preventionweb.net/countries/mys/data/, last access: December 2019.
Rehman, S., Sahana, M., Hong, H. Y., Sajjad, H., and Ahmed, B. B.: A systematic review on approaches and methods used for flood vulnerability assessment: framework for future research, Nat. Hazards, 96, 975–998, https://doi.org/10.1007/s11069-018-03567-z, 2019.
Romali, N., Yusop, Z., Sulaiman, M., and Ismail, Z.: Flood risk assessment: A review of flood damage estimation model for Malaysia, Jurnal Teknologi, 80, 145–153, 2018.
Roslan, R., Omar, R. C., Hara, M., Solemon, B., and Baharuddin, I. N. Z.: Flood insurance rate map for non-structural mitigation, E3S Web Conf., 76, 03002, https://doi.org/10.1051/e3sconf/20197603002, 2019.
Shafiai, S. and Khalid, M. S.: Flood Disaster Management in Malaysia: A Review of Issues of Flood Disaster Relief during and Post-Disaster, in: ISSC 2016 International Conference on Soft Science, Langkawi, Malaysia, 163–170, 2016.
Stephenson, V. and D'Ayala, D.: A new approach to flood vulnerability assessment for historic buildings in England, Nat. Hazards Earth Syst. Sci., 14, 1035–1048, https://doi.org/10.5194/nhess-14-1035-2014, 2014.
Stone, H., D'Ayala, D., and Wilkinson, S.: The use of emerging technology in post-disaster reconnaissance missions, EEFIT Report, The Institution of Structural Engineers, London, 2017.
Stone, H., Putrino, V., and D'Ayala, D.: Earthquake Damage Data Collection Using Omnidirectional Imagery, Front. Built Environ., 4, 51, https://doi.org/10.3389/fbuil.2018.00051, 2018.
Tullos, D.: How to achieve better flood-risk governance in the United States, P. Natl. Acad. Sci. USA, 115, 3731–3734, https://doi.org/10.1073/pnas.1722412115, 2018.
UNISDR: Sendai Framework for Disaster Risk Reduction 2015–2030, UNISDR, Geneva, Switzerland, 2015.
Vogel, K., Riggelsen, C., Korup, O., and Scherbaum, F.: Bayesian network learning for natural hazard analyses, Nat. Hazards Earth Syst. Sci., 14, 2605–2626, https://doi.org/10.5194/nhess-14-2605-2014, 2014.
Wang, G. L., Wang, D. G., Trenberth, K. E., Erfanian, A., Yu, M., Bosilovich, M. G., and Parr, D. T.: The peak structure and future changes of the relationships between extreme precipitation and temperature, Nat. Clim. Change, 7, 268–274, https://doi.org/10.1038/Nclimate3239, 2017.