the Creative Commons Attribution 4.0 License.
the Creative Commons Attribution 4.0 License.
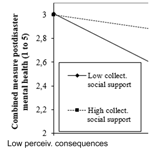
“We can help ourselves”: does community resilience buffer against the negative impact of flooding on mental health?
Torsten Masson
Sebastian Bamberg
Michael Stricker
Anna Heidenreich
Empirical evidence of the relationship between social support and post-disaster mental health provides support for a general beneficial effect of social support (main-effect model; Wheaton, 1985). From a theoretical perspective, a buffering effect of social support on the negative relationship between disaster-related stress and mental health also seems plausible (stress-buffering model; Wheaton, 1985). Previous studies, however, (a) have paid less attention to the buffering effect of social support and (b) have mainly relied on interpersonal support (but not collective-level support such as community resilience) when investigating this issue. This previous work might have underestimated the effect of support on post-disaster mental health. Building on a sample of residents in Germany recently affected by flooding (N=118), we show that community resilience to flooding (but not general interpersonal social support) buffered against the negative effects of flooding on post-disaster mental health. The results support the stress-buffering model and call for a more detailed look at the relationship between support and resilience and post-disaster adjustment, including collective-level variables.
- Article
(779 KB) - Full-text XML
- BibTeX
- EndNote
On the global scale, flooding is one of the most destructive natural hazards, with rising numbers both in terms of the people affected by flooding and the damage attributable to floods (Fattorelli et al., 1999). For example, experts calculated that the annual flood-related losses in Germany may rise from about EUR 500 million in 2001 up to EUR 2 billion by 2100 (Hattermann et al., 2016; Thieken et al., 2005, 2016). However, flooding not only incurs substantial financial costs on societies but also threatens people's health and lives (Alderman et al., 2012). An example of the devastating potential of flooding is Typhoon Haiyan, which killed more than 3900 people when it hit the Philippines in 2013. Previous research has documented the negative effects of severe flooding experiences on people's physical and mental health, such as increased injuries but also increased psychiatric symptoms (e.g., Ahern et al., 2005; Alderman et al., 2012).
A recent review indicates that different factors may be associated with the severity of mental health problems caused by flooding experiences, including flood characteristics (e.g., level of exposure), personal factors (e.g., coping styles, previous flood experience) and social factors (e.g., social support; Fernandez et al., 2015). While a substantial body of literature has investigated how personal and flood characteristics influence post-disaster mental health (cf. Brewin et al., 2000; Lamond et al., 2015), less is known about the effects of social factors (Fernandez et al., 2015; Twigger-Ross et al., 2011; but see Bonanno et al., 2010). Furthermore, past studies have tended to focus on single factors contributing to mental health outcomes, but fewer studies investigated the interplay between different types of factors to explain these outcomes.
The present research aims to advance the understanding of how social factors may interact with flood-related factors (i.e., severity of negative flood-related consequences) in explaining the mental health impacts of flooding. Specifically, it investigates whether different forms of social support, i.e., collective-level social support and interpersonal-level social support, may affect post-disaster mental health in different ways (Cohen and Wills, 1985). We use a measure of community resilience to investigate the effects of collective social support on post-disaster mental health (Pfefferbaum et al., 2011). Community resilience refers to a community's ability to cope with or adapt to hazards efficiently and to recover from their possible catastrophic effects (Berkes and Ross, 2013; Kruse et al., 2019). Resilient communities thus provide their members with the financial and physical resources but also the social resources and capacities needed to anticipate, absorb, or recover from disasters (Doğulu et al., 2016; Kruse et al., 2019; Nelson et al., 2007). Whereas interpersonal social support may have a direct positive effect on post-disaster mental health (main-effect model), community resilience may act as buffer moderating the negative effect of severe flooding experiences on mental health (stress-buffering model, Wheaton, 1985). As previous studies have focused on the main-effect model of social support, they might have underestimated the beneficial effect of social support for post-disaster recovery. To address this shortcoming, we use data from a questionnaire survey gathered immediately after a severe flood event in Germany in 2016 to test for the direct and buffering (moderating) effects of interpersonal social support and community resilience on post-disaster mental health.
Previous reviews collected evidence showing that flooding losses (both financial and nonfinancial) and the stress caused by these losses deteriorate people's mental health conditions: respondents exposed to severe flooding reported more depression, anxiety, and psychosomatic symptoms (headache, bodily pain) and had a higher probability of post-traumatic stress disorder (Alderman et al., 2012). Results also indicate that flooding experiences negatively affected people's psychological well-being and – at least in some studies – led to increased medication usage (Fernandez et al., 2015). Many of the negative impacts of flooding experiences on mental health are transitory and do not develop into clinical disorders (Bonanno et al., 2010; Stein et al., 2007). However, sustained negative health outcomes were also found in a number of studies (Carroll et al., 2009; Du et al., 2010; Kraemer et al., 2009; Medd et al., 2015; Tapsell and Tunstall, 2008; van Ootegem and Verhofstadt, 2016; Whittle et al., 2012; for a review, see Ohl and Tapsell, 2000). For example, Sekulova and van den Bergh (2016) showed that experience of flooding decreased life satisfaction up to 6 years after the flood event (see von Möllendorff and Hirschfeld, 2016, for similar findings).
Floods often have negative mental outcomes. However, not all people exposed to flooding are affected equally in terms of health problems. Previous research has identified several factors that are supposed to mediate or moderate the impact of flooding experiences on mental health, including personal factors, flood characteristics and social factors (Fernandez et al., 2015). Personal factors refer to individual-level characteristics like socioeconomic characteristics and existing health problems but also (cognitive) coping styles (Bei et al., 2013; Carver et al., 1989; Ikizer et al., 2016; Mason et al., 2010) or perceived self-efficacy (Benight and Bandura, 2004; see Höfler, 2014, for a review). For example, high levels of ego resilience, i.e., an individual's capacity for successful adaptation to stressors (Alessandri et al., 2012), were positively associated with more favorable mental health outcomes following traumatic experiences (Philippe et al., 2011). Flood characteristics refer to the severity of exposure or perceived severity of losses. Not surprisingly, severe negative flooding experiences like high property losses or the need to relocate are associated with poorer mental health outcomes (Bubeck and Thieken, 2018; Fernandez et al., 2015; Foudi et al., 2017; Mason et al., 2010).
Social factors refer to general or hazard-related social structures (e.g., flood action groups; Dittrich et al., 2016), which generate the social support needed to cope with losses due to flooding (Bubeck and Thieken, 2018). In contrast to personal factors and flood characteristics, social factors have received less attention when discussing the impacts of flooding on mental health. Previous work has introduced conceptual distinctions between different types of social support (e.g., emotional, informational and tangible help; Norris et al., 2005) and sources of social support (e.g., partner, family, friends, community members or professionals; Kaniasty and Norris, 2009) and between perceived and received social support (Kaniasty and Norris, 2009; Fernandez et al., 2015). Existing empirical evidence indicates that social support is beneficial for post-disaster mental health conditions (see Bonanno et al., 2010; Kaniasty and Norris, 2009, for reviews).
Less agreement exists, however, about the specific ways through which social support can affect mental health outcomes and post-disaster recovery. Previous theorizing has developed three models of how social support may influence the relationship between stress and mental health (Wheaton, 1985). First, the main-effect model (or distress deterrent model) assumes a generalized beneficial effect of support on mental health that originates from people's inclusion in tight-knit social networks (see Fig. 1a). Inclusion in tight-knit social networks provides not only direct material resources but also psychological resources like a sense of predictability and stability in one's life and positive self-worth. Both types of resources can help individuals to maintain positive affect states (Cohen and Wills, 1985). Second, the stress-buffering model states that social support dampens the negative effect of stress on mental health (see Fig. 1b). Statistically, the stress-buffering model assumes that social support moderates the effect of stress on mental health. Past research has identified different stress buffering mechanisms of social support (Cohen and Wills, 1985), for example, people's perception that other (individual or collective) actors from their social networks can provide sufficient resources to reduce or mitigate the negative consequences of a threatening situation. If such resources are available, people may alter their appraisals of stressors or change their coping responses (e.g., more problem-focus coping), leading to better adjustment. As a third approach, the social-support deterioration model assumes that people who experience severe disaster losses perceive less post-disaster social support and social embeddedness (see Fig. 1c; Kaniasty, 2012; Kaniasty and Norris, 2009). Statistically, this model expects a mediating role of social support on mental health.
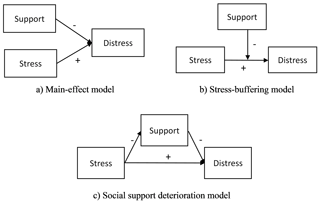
Figure 1Different models of the relationship between social support and mental health outcomes: (a) main-effect model, (b) stress-buffering model and (c) social-support deterioration model.
In flood contexts, empirical evidence for the three models is mixed. A number of studies have provided support for the main-effect model and the social-support deterioration model (Bei et al., 2013; Bubeck and Thieken, 2018; Dai et al., 2016; Kaniasty, 2012; Kaniasty and Norris, 2008; Norris et al., 2005; Ruggiero et al., 2009; Wind et al., 2011; Wind and Komproe, 2012). In contrast, less evidence has been found for the stress-buffering model (Benight, 2004). The mixed empirical evidence for the three models, however, might simply be attributable to the fact that previous disaster research has focused on testing the main-effect model and has paid less attention to the stress-buffering model. Conceptually, Cohen and Wills (1985) have hypothesized that the specific effect of social support (main effect vs. buffering effect) may depend on whether social support is defined as the availability of resources that help to ameliorate the threat (functional measures of social support) or as people's degree of integration in social networks (structural measures of social support). They first provided evidence for their assumption that the buffering effect of social support was more pronounced for functional measures of social support than for structural measures. Likewise, Cohen and Wills (1985) found support for the main-effect model when using structural measures. Other results seem to support this reasoning. Benight (2004) found that the buffering effect on post-disaster distress was stronger for collective efficacy as compared to general social support. The measure of collective efficacy used in this study more closely resembled a functional measure of social support, including questions of the community's (physical, financial, nonmaterial) resources required to respond effectively to disaster events. In contrast, his measure of social support referred to more general (and not necessarily disaster-related) facets of social support, such as the availability of persons to associate with or to talk to about problems (i.e., structural measure of social support). In line with previous findings, Benight (2004) found a main effect of social support (structural measure) but not of collective efficacy (functional measure) on psychological distress. However, as the sample size of the Benight (2004) study was below 50 participants, these findings need further replications to reach firm conclusions.
Taken together, previous research has found evidence for the beneficial effects of social support on people's post-disaster adjustment. Less clarity exists about the ways in which different forms of social support influence the relationship between disaster-related stress and mental health outcomes (main-effect model vs. stress-buffering model). One reason for this might be the lack of studies that have tested both mechanisms in one study using structural, as well as functional social support measures.
The present research has two main objectives. Applying cross-sectional data, we first aim to investigate, in more detail, how flood-related stress (i.e., material and nonmaterial losses due to flooding) and social support are associated with mental health outcomes of flooding, both individually and jointly. We therefore test the (relative) predictive power of the main-effect model and the stress-buffering model of social support based on a German community sample affected by flooding. We assume that previous research on flooding has underestimated the effect of social support on mental health by focusing on main effects. A more rigorous analysis needs to investigate possible main (and interaction) effects of social support to account for the (possibly) multiple ways that support may influence mental health outcomes. Second, previous work has often used measures of interpersonal social support or has focused on personal determinants of protective behavior (Begg et al., 2016; see Bamberg et al., 2017, for a meta-analysis). In contrast, collective-level factors such as a community's capacity to deal with natural hazards (i.e., community resilience) have received less attention (but see Lowe et al., 2015). As natural disasters usually pose a challenge not only to single individuals but also to society at large, more research is needed to investigate the effects of collective-level variables on post-disaster mental health beyond the effects of interpersonal social support measures (see Fritsche et al., 2018, for a similar social psychological approach to addressing global environmental problems). The present research thus applies measures of interpersonal social support to flooding, as well as of community resilience (as a measure of collective social support). Resilient communities are described as having adaptive capacities, involving a community's (material and social) resources as well as the “dynamic attributes” of those resources (e.g., resource redundancy; Norris et al., 2008). Such a notion of community resilience is more in line with a functional notion of social support, whereas measures of interpersonal social support are often more closely related to structural measures of support. Following Cohen and Wills (1985), we expect the buffering effect of social support to be more pronounced when applying measures of community resilience as compared to measures of interpersonal social support.
To further explore the topic, the present research also investigates possible downstream consequences of flood-related losses and social support. Specifically, we ask whether flood-related losses have a conditional indirect effect on life satisfaction through post-disaster mental health, depending on the level of interpersonal or collective social support. Previous research found that exposure to natural hazards decreases people's life satisfaction (von Möllendorff and Hirschfeld, 2016). Extending this work, we test whether post-disaster mental health mediates the relationship between losses and life satisfaction, as a function of interpersonal or collective social support.
In sum, the present research aims to contribute to research on the psychological recovery from flooding by investigating in more detail how interpersonal and collective measures of social support affect the association between negative flooding experiences and post-disaster mental health and well-being. Particularly, our research focuses on the following hypotheses.
-
H1: perceived negative consequences of flooding (e.g., financial and nonfinancial losses) have a negative direct (main) effect on post-disaster mental health.
-
H2: perceived collective social support (community resilience) has a positive direct (main) effect on post-disaster mental health.
-
H3: perceived interpersonal social support has a positive direct (main) effect on post-disaster mental health.
-
H4: perceived collective social support buffers (moderates) the direct impact of negative consequences of flooding on post-disaster mental health.
-
H5: perceived interpersonal support buffers (moderates) the direct impact of perceived negative consequences of flooding on post-disaster mental health.
-
H6: post-disaster mental health has a positive direct effect on life satisfaction.
-
H7: post-disaster mental health mediates the effects of perceived negative consequences of flooding and social support on life satisfaction.
4.1 Study region and sample characteristics
Between May and June 2016 intense rainfall occurred in Central Europe. On 31 May and 1 June, the Rottal-Inn district in the federal state of Bavaria, Germany, was hit by heavy rainfall (120 mm of precipitation within 48 h), leading to severe flash floods as well as mud and debris flows. Seven people lost their lives and the overall insured losses added up to EUR 1.2 billion (GDV, 2017; LfL, 2017). One of the towns mainly affected by the flood event was Simbach am Inn (study region). Simbach am Inn is located at the river Inn, which marks the natural border to the neighboring Austria. It belongs to the Rottal-Inn district and has just below 10 000 inhabitants. The community covers an area of 47.31 km2 and has a population density of 207 inhabitants per km2 (Bayerisches Landesamt für Statistik, 2018).
Approximately 6 weeks after the disaster, a group of researchers from our team conducted a household survey in the town of Simbach am Inn and the surrounding regions. Local town councils provided us with lists of streets affected by the flood event. We distributed 600 paper and pencil surveys and provided households with a link to an online survey. Answers were collected for a period of approximately 2 months. After excluding participants with missing data, the final sample consists of 118 respondents (Npaper and pencil=79) aged between 18 and 80 (46.7 % female, Mage=50.73, SDage=14.70). The majority of the participants were property owners (79.2 %) and approximately one-third of the participants (32.5 %) had previous flood experience.
4.2 Measures
Table 1 presents the mean, standard deviation, Cronbach's alpha coefficient (provided in parentheses) and inter-scale correlation for each of the variables. Unless otherwise noted, all items used five-point Likert scales. To fit the requirements of a field study (space limitations), the scales were operationalized with a limited number of items. Item selection was based on considerations of internal validity (reflect different aspects of a construct) and scale reliability. Due to the field context (surveying people only few weeks after flooding), we also discussed our draft questionnaire with local representatives and persons helping us to disseminate the questionnaire (e.g., representatives from emergency organizations). The final item selection was based on these discussions.
Table 1Mean, standard deviation, Cronbach's alpha coefficient (provided in parentheses) and inter-scale correlations between variables.
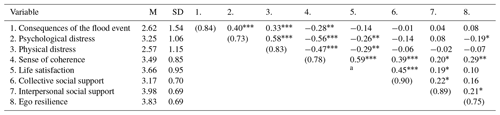
* p<0.05. p<0.01. p<0.001. a Cronbach's alpha not computed (single item measure).
We assessed perceived consequences of the flood event (i.e., flood-related stress) with four items (six-point scale: 0 = not affected, 1 = not very severe, 5 = very severe). The items referred to the severity of the consequences for respondents' house or apartment, other valuables, general financial situation, and their psychological well-being (Begg et al., 2016). Next, we measured post-disaster mental health, including measures of psychological and physical distress, as well as sense of coherence. Participants answered three items on flood-related psychological distress (“How often have you felt [upset, anxious, sad] in the last four weeks?”; 1 = never, 5 = very often) taken from the Short-Form Health Survey (Ware and Sherbourne, 1992). Four items measured flood-related physical distress (“How often have you had [headache, heart palpitations, upset stomach, stomachache] in the last four weeks?”; 1 = never, 5 = very often). As an additional health-related variable, a five-item measure of sense of coherence was included in the questionnaire (Schumacher et al., 2000; e.g., “When you think about your life, you very often: 1 = feel how good it is to be alive, 5 = ask yourself why you exist at all”). Sense of coherence (Antonovsky, 1988) refers to “people's ability to assess and understand the situation they were in, to find a meaning to move in a health promoting direction, also having the capacity to do so” (Eriksson, 2017). We included ego resilience as a covariate, measuring individual differences in respondents' capacity to deal with flood-related stress. Participants then answered a one-item indicator of life satisfaction (“All things considered, how satisfied are you with your life as a whole?”; 1 = completely dissatisfied, 5 = completely satisfied).
Perceived collective social support (community resilience to natural hazards) was measured with the Communities Advancing Resilience Toolkit Assessment Survey (CART; (Pfefferbaum et al., 2013a, 2015). The scale had been translated into German by a back-translation procedure. Due to space limitations, we had to reduce the number of items from 21 to 14 items (e.g., “People in my community feel like they belong to the community” and “My community has resources it needs to take care of community problems [resources include, for example, money, information, technology, tools, raw materials and services]”; 1 = totally disagree, 5 = totally agree). The scale measures community members' perceptions of how well their community can cope with disasters, involving aspects like available resources on the community level or the community's ability to take goal-oriented action (transformative potential). Participants also answered three items on perceived interpersonal social support taken from the social support questionnaire (Fydrich et al., 1999; e.g., “I have people close to me, if I need someone to talk to”; 1 = totally disagree, 5 = totally agree). Finally, participants were asked to answer a five-item measure of ego resilience (or resilient coping) based on Kocalevent et al. (2017). The scale measures individual differences in people's tendency to cope with stress in an adaptive manner and served as a covariate in the analyses (e.g., “Regardless of what happens to me, I believe I can control my reaction to it”; 1 = totally disagree, 5 = totally agree).
5.1 Analysis strategy
The data were analyzed using SPSS software (hierarchical multiple regression) and Mplus 7.3 software (path analysis, multi group comparison). Following Aiken and West (1991), all interactions were probed at one standard deviation above (+1 SD) and one standard deviation below (−1 SD) the mean of the moderator. All continuous predictors were mean-centered prior to the calculation of the interaction terms.
Hierarchical multiple regression analysis results
Based on their substantive positive intercorrelations (see Table 1), we combined the three measures of psychological and physical distress and sense of coherence into a single measure of post-disaster mental health. We recoded the measures so that higher values indicate better mental health. To test our hypotheses, we submitted the combined measure of post-disaster mental health to hierarchical multiple regression analysis with interaction tests. We included perceived negative consequences of the flood event, perceived collective social support (community resilience) and perceived interpersonal support as predictors in Step 1 of the analysis, as well as the two-way interaction terms of perceived consequences and collective and interpersonal social support as additional predictors in Step 2 of the analysis. Results of the regression analyses are shown in Table 2.
Table 2Hierarchical regression of the combined post-disaster mental health measure on perceived negative consequences, perceived collective social support (community resilience), perceived interpersonal social support and their interaction terms.
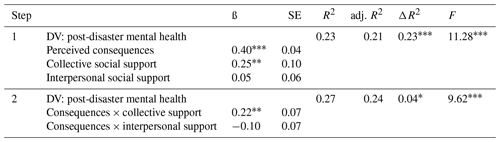
* p<0.05. p<0.01. p<0.001.
In Step 1, the results showed a negative main effect of perceived negative flood consequences (H1), , and p<0.001 and a positive main effect of perceived collective social support (H2), ß=0.25, t(116)=3.00 and p=0.003 on post-disaster mental health. These effects were qualified by the expected interaction effect of perceived negative flood consequences and collective social support (H4) in Step 2: ß=0.22, t(114)=2.46, p=0.016 (see Fig. 2). Simple slope analysis revealed that perceived consequences were negatively correlated with post-disaster mental health only when perceived collective social support was low (−1 SD), unstandardized , and p<0.001 but not at high levels of collective social support (+1 SD), unstandardized , and p=0.199. For the interpersonal social support measure, results showed neither a significant main (H3) nor a significant interaction effect (H5). As expected, these findings provide empirical evidence for a substantive buffering effect of social support (stress-buffering model). Furthermore, they indicate that the buffering effect is more pronounced for perceived collective social support than for perceived interpersonal social support. We also conducted separate regression analyses with psychological and physical distress or sense of coherence as dependent variables. Results showed significant interaction effects of perceived consequences and collective social support (community resilience) for both dependent variables (distress and sense of coherence), thus supporting the robustness of our findings.
To test the stability of our results, we also included ego resilience as a covariate in the analysis. Results showed a positive main effect of ego resilience, indicating that respondents who were more psychologically resilient reported better post-disaster mental health. More importantly, the interaction effect of perceived flood consequences and collective social support remained significant, ß=0.18, t(112)=2.09, p=0.039. Our results thus provide evidence for the beneficial effect of collective-level factors (community resilience) beyond individual-level variables, such as personal coping styles or a person's mental capacity to cope successfully with stress.
5.2 Indirect effects: life satisfaction
Figure 3 presents the results of a path analysis (Mplus 7.3) including life satisfaction as an additional dependent variable. Life satisfaction is interpreted as a subjective resilience indicator. We found no significant main effect of perceived negative flood consequences on life satisfaction () but instead found a positive main effect of collective social support on life satisfaction (ß=0.31). In line with H6, post-disaster mental health showed a statistically significant positive association with life satisfaction (ß=0.44). Comparison of indirect effects showed that post-disaster mental health completely mediated the association between negative flood consequences and life satisfaction and partly mediated the association between collective social support and life satisfaction (H7). Together, mental health and perceived collective social support explain 35 % of the variance in life satisfaction. The model depicted in Fig. 3 fits the empirical covariance matrix well (χ2=1.95, df=1, p=0.16, CFI = 0.99, TLI = 0.93, RMSEA = 0.09).
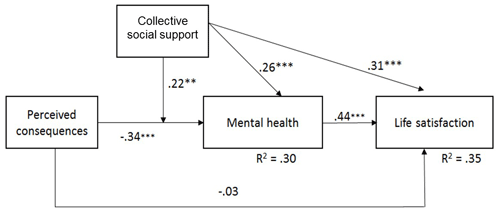
Figure 3Path model with life satisfaction as dependent variable. Note: N=118; standardized path coefficients; R2= explained variance; , ; interpersonal support omitted (not significant).
In a more exploratory manner, we also tested whether the indirect effect of perceived consequences on life satisfaction through mental health was conditional on the level of collective social support (high vs. low collective social support). As we had found a buffering effect of collective social support on post-disaster mental health, we tested whether mental health would mediate this buffering effect on life satisfaction. We used the multiple group option of Mplus to test for a possible conditional indirect effect. More precisely, we estimated simultaneously the same association structure between perceived consequences, post-disaster mental health and life satisfaction for participants with lower levels of collective social support (N=54) and participants with higher levels of collective social support (N=64). The median split of the perceived collective social support variable (Md = 3.14) was used for creating these two subgroups. Figure 4 presents the results of the multiple group analysis.
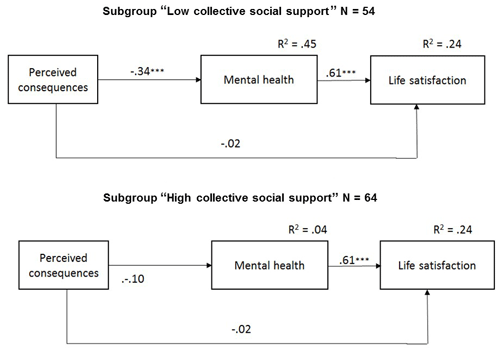
Figure 4Results of the multiple group analysis. Note: unstandardized path coefficients; R2= explained variance; , ; interpersonal support omitted (not significant).
In the multiple group analysis, the significant interaction effect of perceived flood consequences and collective social support should be reflected in the “significantly stronger flood consequences–mental health association” in the low collective social support subgroup (i.e., low community resilience subgroup) as compared to the high collective social support subgroup (i.e., low community resilience subgroup). This assumption can be tested with a χ2 difference test comparing the χ2 value of a multiple group model specifying the “flood consequences–mental health association” as being equal across both subgroups versus a model specifying these path coefficients as free across both groups. The χ2 difference value resulting from the model comparison is statistically significant (χ2=8.42, df=1, p<0.001). That is, fixing the “flood consequences–mental health” path equal across both groups results in a significantly decreased model fit. As depicted in Fig. 4, the estimated negative flood consequences – mental health association is (unstandardized path coefficient) for the subgroup with low collective social support (collective support < median). For the high collective social support subgroup (collective support > median), the estimated path coefficient is only and statistically insignificant. All other path coefficients could be fixed as equal across both subgroups without causing a significant decrease in model fit. The multiple group model depicted in Fig. 4 has a good fit (χ2=0.64, df=2, p=0.72, CFI = 1.00, TLI = 1.07, RMSEA = 0.00).
The indirect effect estimates provided by Mplus can be used for quantifying the indirect buffering effect of collective social support (community resilience) on post-disaster life satisfaction: for the subgroup of participants with lower community resilience, the significant total effect of the perceived negative flood consequences on life satisfaction is 0.21. For the subgroup of participants with higher community resilience, the total effect of the perceived negative flood consequences on life satisfaction is only 0.06, which is statistically insignificant. These results clearly indicate a substantive indirect buffering effect of collective social support on life satisfaction through post-disaster mental health.
The present research had two main objectives: to investigate how negative flood experiences and perceived social support are correlated with post-disaster mental health and life satisfaction and to analyze whether these associations would differ as a function of type of social support (collective vs. interpersonal social support). Our analyses are based on a data set of 118 respondents from Germany, surveyed 6 to 12 weeks after they were affected by a severe flood event.
The results of statistical analyses provide clear answers to both questions. Perceived flood consequences were substantively negatively associated with post-disaster mental health, while perceived collective social support (community resilience) was positively associated with post-disaster mental health. However, the main effect of perceived flood consequences was qualified by a statistically significant positive interaction effect of perceived flood consequences (e.g., flood-related losses) and collective social support. Further analysis of this interaction effect demonstrated that perceptions of the flood event as very severe were associated with worse post-disaster mental health only in case of low levels of perceived community resilience (low collective social support). When the community's capability to effectively deal with catastrophic events was perceived as high (high collective support), even greater flood-related losses were no longer associated with poorer mental health outcomes. This adds further empirical evidence to the so-called stress-buffering model that states that social support dampens the negative effect of stress on mental health (Wheaton, 1985). Including the interaction term of perceived consequences and collective social support in the analysis increased the explanatory power of the statistical model from 23 to 27 percent of the variance explained in post-disaster mental health. In other words, a simple test of the main effect model of social support would have underestimated the beneficial effect of social support on post-disaster mental health and recovery. Previous flooding research has tended to rely on main effects when discussing the role of social support for mental health outcomes. In contrast, our findings suggest that a more detailed look at this issue might be feasible to better account for the multiple ways how social support can affect mental health and recovery in times of crisis. We thus encourage future research to test the stress-buffering model more frequently to better capture the possible interplay of flood-related stress and social support for their role in post-disaster recovery processes. We also encourage future studies to include more objective measures of flood consequences (e.g., amount of financial damage) to complement the self-reported measures used in the current research.
Regarding our second question, the present results provide evidence that the buffering effect of support on mental health might be stronger for more collective forms of social support (community resilience) as compared to more interpersonal forms of social support (general social support from family, friends etc.). After controlling for collective social support, we found no main or interaction effects of interpersonal social support on the dependent variables. Our results partly support Cohen and Wills' (1985) assumptions about the effects of different types of social support on mental adjustment following exposure to stressors. Whereas functional measures of support should have a buffering (i.e., moderator) effect on psychological distress (buffering model), the effects of structural support measures should be more in line with the main effect model. As our measure of collective social support resembles more closely a functional support measure, the present interaction effect of collective support and perceived flood consequences provides evidence for Cohen and Wills' (1985) reasoning. Contrary to the Cohen and Wills' assumptions, however, our data revealed no main effect of interpersonal (i.e., more structural) measures of social support. This might be attributable to the (skewed) distribution of our interpersonal support measure. Mean interpersonal social support (M=3.98) was well above the midpoint of the scale (3), thus possibly restricting the detection of main effects. Another reason might be that the operationalization of the two measures of social support differed not only with regard to their type of support (interpersonal vs. collective support) but also with regard their relevance to flooding. Whereas the collective support measure referred to the community's capacity to deal with natural hazards, the interpersonal support measure referred to general aspects of people's social networks. Although these differences were in part central to our research questions, future research may aim to disentangle the effects of type of support (functional vs. structural) from a possible context effect (flood-related vs. not flood-related). Similarly, our measure of collective social support referred to the community's general capacity to deal with natural hazards but it neither focused on the specific 2016 flood event nor explicitly asked how much support respondents had received from their community. Applying a more focused or direct measure of collective support might have even increased the size of the buffering effect.
More exploratory data analyses also indicated that flooding experiences have a conditional indirect negative effect on life satisfaction, completely mediated by mental health. Subgroup analyses showed that this indirect negative effect on life satisfaction is substantially reduced when collective social support is high: for the subgroup with low collective social support, negative flooding experiences have a more than 3 times higher indirect negative impact on post-disaster life satisfaction than for the subgroup with higher collective social support. Again, these findings support our call to account for possible buffering effects of social support by applying appropriate research designs (e.g., moderator analysis).
The present results underline the significance of the social support construct for improving our understanding of how people cope psychologically with the negative consequences of natural disasters such as floods. The second important insight of the present study consists of the finding that only perceived collective social support but not (general) interpersonal social support was critical for damping the negative psychological effects of severe flood experiences. Although the effects of social capital on mental health outcomes have been studied for some time (McPherson et al., 2014; De Silva et al., 2005), research on post-flooding recovery has not systematically distinguished between more interpersonal and more collective types of support. This might be somewhat surprising given the fact that flood events are collective phenomena that usually can only be mastered by collective effort. From this perspective, it seems quite self-evident that perceptions of one's own community as being more resilient to natural disasters are associated with less negative mental health outcomes at the individual level, as suggested by our results. Nevertheless, our findings have important theoretical and practical implications.
Conceptually, our results suggest that it might be feasible for future research to put a stronger focus on collective-level processes and resources, as well as possible interactive effects of personal, flood-related and social factors, when thinking about how people cope with flood events. Because of the correlational nature of our results, the assumed causality of the described associations between collective social support and post-disaster mental health remains, however, insecure. Thus, longitudinal or (when possible) experimental tests of the effects of the different types of social support are necessary for clarifying causality. Recent findings lend some support to this claim (Lowe et al., 2015; Wind and Komproe, 2012). Applying a longitudinal design, Matsuyama et al. (2016) found that both individual-level and community-level social support independently, and positively, contributed to post-disaster mental health of earthquake survivors in Japan. Future research may investigate how different types of social support interact with personal or flood-related factors to influence mental health outcomes. Such a research focus would also promote a more systematic integration of the psychological literature on coping with stressful events and the sociological literature on the social capital concept. After all, social networks are the central structural component of the social capital concept (Coleman, 1988; Portes, 1998; Putnam, 2000). Social capital does not refer to individuals but to the relationships among individuals. It thus provides access to the resources of social life such as support, assistance, recognition, knowledge and connections. Combining psychological research with research on the different dimensions of social capital (structural, cognitive, relational dimensions; Nahapiet and Ghoshal, 1998) might further our understanding of how personal, flood-related and social factors (jointly) contribute to resilience and post-disaster well-being.
Including collective-level variables (such as community resilience) in models of post-disaster adjustment would also have important practical implications. Currently, most flood intervention programs are targeted at (the promotion of) individual protective behaviors (Bamberg et al., 2017). Focusing on models of collective behavior (Fritsche et al., 2018; Masson and Fritsche, 2014, 2019; Masson et al., 2016) could foster the development of theory-based interventions that also promote collective (e.g., communal) support systems. As an example of such interventions, the Communities Advancing Resilience Toolkit (CART) aims to assist communities in systematically enhancing their resilience to disasters (Pfefferbaum et al., 2013a, 2015). Previous applications of the CART survey instrument have supported the proposed model structure (Pfefferbaum et al., 2013b, 2015), but (longitudinal) evaluations of the community toolkit as an intervention program are a pending task for future research. We are convinced that theory-based development, implementation and evaluation of collective-level interventions provide a feasible avenue for social science disaster research both theoretically and practically.
Data in this paper can be made available for scientific use upon request to the author.
Consequences of the flood event
(six-point scale: 0 = not affected, 1 = not very severe, 5 = very severe)
-
Has the flood damaged your house or apartment? If so, how severe was the damage?
-
Has the flood destroyed any other valuables (e.g., cars or jewelry) of yours? If so, how severe were these losses?
-
Do you have pressing financial concerns because of the flood damages? If so, how severe are they?
-
Have the flooding event and everything connected to it caused psychological strain for you? If so, how severe was or is the strain?
-
Have you experienced a flooding event before? If so, did you experience the event in this place or another place?
Psychological distress
(five-point scale: 1 = never, 5 = very often)
-
How often did you experience the following feelings in the last four weeks: upset, anxious, sad, happy, and balanced?
Physical distress
(five-point scale: 1 = never, 5 = very often)
-
How often did you have the following physical complaints in the last four weeks: headache, heart palpitations, upset stomach, and stomachache?
Sense of coherence
(five-point scale: 1 = very rarely or never, 5 = very often)
-
How often do you feel that you are in an unfamiliar situation and do not know what to do? How often are your feelings confused?
-
Many people, even those with a strong character, feel like losers in certain situations. How often have you felt that way in the past?
-
How often do you feel like the things you do in your everyday life make little sense?
-
When you think about your life, you very often… (1 = ask yourself why you exist at all, 5 = feel how good it is to be alive).
Life satisfaction
(five-point scale: 1 = completely dissatisfied, 5 = completely satisfied)
-
All things considered, how satisfied are you with your life as a whole?
Collective social support
(five-point scale: 1 = totally disagree, 5 = totally agree)
-
People in my community feel like they belong to the community.
-
People in my community are committed to the well-being of the community.
-
People in my community help each other.
-
My community has the resources it needs to take care of community problems (resources include, for example, money, information, technology, tools, raw materials, and services).
-
People in my community are able to get the services they need.
-
People in my community know where to go to get things done.
-
People in my community work together to improve the community.
-
My community looks at its successes and failures so it can learn from the past.
-
My community develops skills and finds resources to solve its problems and reach its goals.
-
My community tries to prevent disasters.
-
My community can provide emergency services during a disaster.
-
My community has services and programs to help people after a disaster.
-
If a disaster occurs, my community provides information about what to do.
-
People in my community trust public officials.
Interpersonal social support
(five-point scale: 1 = totally disagree, 5 = totally agree)
-
I have people close to me, if I need someone to talk to.
-
I have people close to me, if I have a problem and need good advice.
-
I have people close to me that help me when I need to make a hard decision.
Ego resilience
(five-point scale: 1 = totally disagree, 5 = totally agree)
-
I actively look for ways to replace the losses that I encounter in life.
-
I look for creative ways to alter difficult situations.
-
Regardless of what happens to me, I believe I can control my reaction to it.
-
I believe that I can grow in positive ways by dealing with difficult situations.
-
I actively look for ways to balance the losses that I encounter in life.
TM and SB conceptualized the study, carried out the main data analysis and wrote the original draft. MS and AH were involved in the writing, review and editing processes.
The authors declare that they have no conflict of interest.
The authors acknowledge Katrin Brewitt, Anja Schäffler and Klara Kauhausen for their contributions to this work (data collection).
This Article is funded by the Open Access Publication Fund of the Bielefeld University of Applied Sciences and the Deutsche Forschungsgemeinschaft (grant no. 414001623).
This paper was edited by Sven Fuchs and reviewed by two anonymous referees.
Ahern, M., Kovats, R. S., Wilkinson, P., Few, R., and Matthies, F.: Global health impacts of floods: Epidemiologic evidence, Epidemiol. Rev., 27, 36–46, https://doi.org/10.1093/epirev/mxi004, 2005.
Aiken, L. S. and West, S. G.: Multiple regression: Testing and interpreting interactions, SAGE, Newbury Park, Calif., 2003.
Alderman, K., Turner, L. R., and Tong, S.: Floods and human health: A systematic review, Environ. Int., 47, 37–47, https://doi.org/10.1016/j.envint.2012.06.003, 2012.
Alessandri, G., Vecchione, M., Caprara, G., and Letzring, T. D.: The Ego Resiliency Scale Revised, Eur. J. Psychol. Assess., 28, 139–146, https://doi.org/10.1027/1015-5759/a000102, 2012.
Antonovsky, A.: Unraveling the mystery of health: How people manage stress and stay well, 1. edn., The Jossey-Bass health series, Jossey-Bass, San Francisco, 1988.
Bamberg, S., Masson, T., Brewitt, K., and Nemetschek, N.: Threat, coping and flood prevention – A meta-analysis: A meta-analytical research synthesis, J. Environ. Psychol., 54, 116–126, https://doi.org/10.1016/j.jenvp.2017.08.001, 2017.
Bayerische Landesanstalt für Landwirtschaft – LfL: Starkregen, Bodenerosion, Sturzfluten: Beobachtungen und Analysen im Mai/Juni 2016, available at: https://www.lfl.bayern.de/mam/cms07/publikationen/daten/schriftenreihe/starkregen-bodenerosion_sturzfluten_lfl-schriftenreihe.pdf (last access: 22 October 2019), 2017.
Bayerisches Landesamt für Statistik: https://www.statistik.bayern.de/ (last access: 15 July 2019), 2018.
Begg, C., Ueberham, M., Masson, T., and Kuhlicke, C.: Interactions between citizen responsibilization, flood experience and household resilience: Insights from the 2013 flood in Germany, Int. J. Water. Resour. D., 33, 591–608, https://doi.org/10.1080/07900627.2016.1200961, 2016.
Bei, B., Bryant, C., Gilson, K.-M., Koh, J., Gibson, P., Komiti, A., and Judd, F.: A prospective study of the impact of floods on the mental and physical health of older adults, Aging Ment. Health, 17, 992–1002, https://doi.org/10.1080/13607863.2013.799119, 2013.
Benight, C. C.: Collective efficacy following a series of natural disasters, Anxiety, Stress & Coping, An Int. J., 17, 401–420, https://doi.org/10.1080/10615800512331328768, 2004.
Benight, C. C. and Bandura, A.: Social cognitive theory of posttraumatic recovery: The role of perceived self-efficacy, Behav. Res. Ther., 42, 1129–1148, https://doi.org/10.1016/j.brat.2003.08.008, 2004.
Berkes, F. and Ross, H.: Community resilience. Toward an integrated approach, Soc. Nat. Resour., 26, 5–20, https://doi.org/10.1080/08941920.2012.736605, 2013.
Bonanno, G. A., Brewin, C. R., Kaniasty, K., and La Greca, A. M.: Weighing the Costs of Disaster: Consequences, Risks, and Resilience in Individuals, Families, and Communities, Psychol. Sci. Publ. Int., 11, 1–49, https://doi.org/10.1177/1529100610387086, 2010.
Brewin, C. R., Andrews, B., and Valentine, J. D.: Meta-analysis of risk factors for posttraumatic stress disorder in trauma-exposed adults, J. Consult. Clin. Psych., 68, 748–766, https://doi.org/10.1037/0022-006X.68.5.748, 2000.
Bubeck, P. and Thieken, A. H.: What helps people recover from floods? Insights from a survey among flood-affected residents in Germany, Reg. Environ. Change, 18, 287–296, https://doi.org/10.1007/s10113-017-1200-y, 2018.
Carroll, B., Morbey, H., Balogh, R., and Araoz, G.: Flooded homes, broken bonds, the meaning of home, psychological processes and their impact on psychological health in a disaster, Health Place, 15, 540–547, https://doi.org/10.1016/j.healthplace.2008.08.009, 2009.
Carver, C. S., Scheier, M. F., and Weintraub, J. K.: Assessing coping strategies: A theoretically based approach, J. Pers. Soc. Psychol., 56, 267–283, https://doi.org/10.1037/0022-3514.56.2.267, 1989.
Cohen, S. and Wills, T. A.: Stress, social support, and the buffering hypothesis, Psychol. Bull., 98, 310–357, https://doi.org/10.1037//0033-2909.98.2.310, 1985.
Coleman, J. S.: Social capital in creation of human capital, Am. J. Sociol., 94, 95–120, 1988.
Dai, W., Chen, L., Tan, H., Wang, J., Lai, Z., Kaminga, A. C., and Liu, A.: Association between social support and recovery from post-traumatic stress disorder after flood: A 13–14 year follow-up study in Hunan, China, BMC Public Health, 16, 194, https://doi.org/10.1186/s12889-016-2871-x, 2016.
De Silva, M. J., McKenzie, K., Harpham, T., and Huttly, S. R. A.: Social capital and mental illness: A systematic review, J. Epidemiol. Commun. H., 59, 619–627, https://doi.org/10.1136/jech.2004.029678, 2005.
Dittrich, R., Wreford, A., Butler, A., and Moran, D.: The impact of flood action groups on the uptake of flood management measures, Climatic Change, 138, 471–489, https://doi.org/10.1007/s10584-016-1752-8, 2016.
Doğulu, C., Karanci, A. N., and Ikizer, G.: How do survivors perceive community resilience? The case of the 2011 earthquakes in Van, Turkey, Int. J. Disaster Risk Reduct., 16, 108–114, https://doi.org/10.1016/j.ijdrr.2016.02.006, 2016.
Du, W., FitzGerald, G. J., Clark, M., and Hou, X.-Y.: Health Impacts of Floods, Prehospital and Disaster Medicine, 25, 265–272, https://doi.org/10.1017/S1049023X00008141, 2010.
Eriksson, M.: The Sense of Coherence in the Salutogenic Model of Health, in: The Handbook of Salutogenesis, edited by: Mittelmark, M. B., Lindström, B., Bauer, G. F., Espnes, G. A., Pelikan, J. M., Eriksson, M., and Sagy, S., Springer, Berlin, Heidelberg, 91–96, 2017.
Fattorelli, S., Fontana, G. D., and Ross, D.: Flood Hazard Assessment and Mitigation, in: Floods and Landslides: Integrated Risk Assessment, edited by: Casale, R. and Margottini, C., Springer, Berlin, Heidelberg, 19–38, 1999.
Fernandez, A., Black, J., Jones, M., Wilson, L., Salvador-Carulla, L., Astell-Burt, T., and Black, D.: Flooding and mental health: A systematic mapping review, PloS One, 10, e0119929, https://doi.org/10.1371/journal.pone.0119929, 2015.
Foudi, S., Osés-Eraso, N., and Galarraga, I.: The effect of flooding on mental health: Lessons learned for building resilience, Water Resour. Res., 53, 5831–5844, https://doi.org/10.1002/2017WR020435, 2017.
Fritsche, I., Barth, M., Jugert, P., Masson, T., and Reese, G.: A social identity model of pro-environmental action (SIMPEA), Psychol. Rev., 125, 245–269, https://doi.org/10.1037/rev0000090, 2018.
Fydrich, T., Geyer, M., Hessel, A., Sommer, G., and Brähler, E.: Fragebogen zur Sozialen Unterstützung (F-SozU): Normierung an einer repräsentativen Stichprobe, Diagnostica, 45, 212–216, https://doi.org/10.1026//0012-1924.45.4.212, 1999.
GDV – Gesamtverband der Deutschen Versicherungswirtschaft: Naturgefahrenreport 2016, Berlin, 2016. (in German)
Hattermann, F. F., Huang, S., Burghoff, O., Hoffmann, P., and Kundzewicz, Z. W.: Brief Communication: An update of the article “Modelling flood damages under climate change conditions – a case study for Germany”, Nat. Hazards Earth Syst. Sci., 16, 1617–1622, https://doi.org/10.5194/nhess-16-1617-2016, 2016.
Höfler, M.: Psychological Resilience Building in Disaster Risk Reduction: Contributions from Adult Education, Int. J. Disaster Risk Sci., 5, 33–40, https://doi.org/10.1007/s13753-014-0009-2, 2014.
Ikizer, G., Karanci, A. N., and Ikizer, G.: Exploring Factors Associated with Psychological Resilience Among Earthquake Survivors from Turkey, J. Loss Trauma, 21, 384–398, https://doi.org/10.1080/15325024.2015.1108794, 2016.
Kaniasty, K.: Predicting social psychological well-being following trauma: The role of postdisaster social support, Psychol. Trauma-US, 4, 22–33, https://doi.org/10.1037/a0021412, 2012.
Kaniasty, K. and Norris, F. H.: Longitudinal linkages between perceived social support and posttraumatic stress symptoms: Sequential roles of social causation and social selection, J. Trauma Stress, 21, 274–281, https://doi.org/10.1002/jts.20334, 2008.
Kaniasty, K. and Norris, F. H.: Distinctions that matter: Received social support, perceived social support, and social embeddedness after disasters, in: Mental health and disasters, edited by: Neria, Y., Galea, S., and Norris, F. H., University Press, New York, Cambridge, 175–200, 2009.
Kocalevent, R.-D., Zenger, M., Hinz, A., Klapp, B., and Brähler, E.: Resilient coping in the general population: Standardization of the brief resilient coping scale (BRCS), Health Qual. Life Out., 15, 251, https://doi.org/10.1186/s12955-017-0822-6, 2017.
Kraemer, B., Wittmann, L., Jenewein, J., and Schnyder, U.: 2004 Tsunami: Long-term psychological consequences for Swiss tourists in the area at the time of the disaster, Aust. Nz. J. Psychiat., 43, 420–425, https://doi.org/10.1080/00048670902817653, 2009.
Kruse, S., Abeling, T., Deeming, H., Fordham, M., Forrester, J., Jülich, S., Nuray Karanci, A., Kuhlicke, C., Pelling, M., Pedoth, L., Schneiderbauer, S., and Sharpe, J.: The emBRACE Resilience Framework: Developing an Integrated Framework for Evaluating Community Resilience to Natural Hazards, in: Framing Community Disaster Resilience: Resources, Capacities, Learning, and Action, edited by: Deeming, H., Fordham, M., Kuhlicke, C., Pedoth, L., Schneiderbauer, S., and Shreve, X., Wiley & Sons, Chichester, 79–96, 2019.
Lamond, J. E., Joseph, R. D., and Proverbs, D. G.: An exploration of factors affecting the long term psychological impact and deterioration of mental health in flooded households, Environ. Res., 140, 325–334, https://doi.org/10.1016/j.envres.2015.04.008, 2015.
Lowe, S. R., Joshi, S., Pietrzak, R. H., Galea, S., and Cerdá, M.: Mental health and general wellness in the aftermath of Hurricane Ike, Soc. Sci. Med., 124, 162–170, https://doi.org/10.1016/j.socscimed.2014.11.032, 2015.
Mason, V., Andrews, H., and Upton, D.: The psychological impact of exposure to floods, Psychology, Health Medicine, 15, 61–73, https://doi.org/10.1080/13548500903483478, 2010.
Mason, T. and Fritsche, I.: Adherence to climate change-related ingroup norms: Do dimensions of group identification matter?, Eur. J. Soc. Psychol., 44, 455–465, https://doi.org/10.1002/ejsp.2036, 2014.
Mason, T. and Fritsche, I.: Loyal peripherals? The interactive effects of identification and peripheral group membership on deviance from non-beneficial ingroup norms, Eur. J. Soc. Psychol., 49, 76–92, https://doi.org/10.1002/ejsp.2501, 2019.
Masson, T., Jugert, P., and Fritsche, I.: Collective self-fulfilling prophecies: Group identification biases perceptions of environmental group norms among high identifiers, Soc. Influ., 11, 185–198, https://doi.org/10.1080/15534510.2016.1216890, 2016.
Matsuyama, Y., Aida J., Hase A., Sato, Y., Koyama, S., Tsuboya, T., and Osaka, K.: Do community- and individual-level social relationships contribute to the mental health of disaster survivors?: A multilevel prospective study after the Great East Japan Earthquake, Soc. Sci. Med., 151, 187–195, https://doi.org/10.1016/j.socscimed.2016.01.008, 2016.
McPherson, K. E., Kerr, S., McGee, E., Morgan, A., Cheater, F. M., McLean, J., and Egan, J.: The association between social capital and mental health and behavioural problems in children and adolescents: An integrative systematic review, BMC Psychology, 2, 7, https://doi.org/10.1186/2050-7283-2-7, 2014.
Medd, W., Deeming, H., Walker, G., Whittle, R., Mort, M., Twigger-Ross, C., and Kashefi, E.: The flood recovery gap: A real-time study of local recovery following the floods of June 2007 in Hull, North East England, J. Flood Risk Manag., 8, 315–328, https://doi.org/10.1111/jfr3.12098, 2015.
Nahapiet, J. and Ghoshal, S.: Social Capital, Intellectual Capital, and the Organizational Advantage, Acade. Manage. Rev., 23, 242–266, https://doi.org/10.2307/259373, 1998.
Nelson, D. R., Adger, W. N., and Brown, K.: Adaptation to Environmental Change: Contributions of a Resilience Framework, Annu. Rev. Environ. Resour., 32, 395–419, https://doi.org/10.1146/annurev.energy.32.051807.090348, 2007.
Norris, F. H., Baker, C. K., Murphy, A. D., and Kaniasty, K.: Social support mobilization and deterioration after Mexico's 1999 flood: Effects of context, gender, and time, Am. J. Commun. Psychol., 36, 15–28, https://doi.org/10.1007/s10464-005-6230-9, 2005.
Norris, F. H., Stevens, S. P., Pfefferbaum, B., Wyche, K. F., and Pfefferbaum, R. L.: Community Resilience as a Metaphor, Theory, Set of Capacities, and Strategy for Disaster Readiness, Am. J. Commun. Psychol., 41, 127–150, https://doi.org/10.1007/s10464-007-9156-6, 2008.
Ohl, C. A. and Tapsell, S. M.: Flooding and human health: the dangers posed are not always obvious, BMJ Brit. Med. J., 321, 1167–1168, https://doi.org/10.1136/bmj.321.7270.1167, 2000.
Pfefferbaum, R. L., Pfefferbaum, B., and Van Horn, R. L.: Communities advancing resilience toolkit (CART): The CART integrated system, Terrorism and Disaster Center at the University of Oklahoma Health Sciences Center, Oklahoma City, 2011.
Pfefferbaum, R. L., Neas, B. R., Pfefferbaum, B., Norris, F. H., and van Horn, R. L.: The Communities Advancing Resilience Toolkit (CART): Development of a survey instrument to assess community resilience, Int. J. Emerg. Ment. Health., 15, 15–30, 2013a.
Pfefferbaum, R. L., Pfefferbaum, B., van Horn, R. L., Klomp, R. W., Norris, F. H., and Reissman, D. B.: The Communities Advancing Resilience Toolkit (CART): An intervention to build community resilience to disasters, J. Public Health Man., 19, 250–258, https://doi.org/10.1097/PHH.0b013e318268aed8, 2013b.
Pfefferbaum, R. L., Pfefferbaum, B., Nitiéma, P., Houston, J. B., and van Horn, R. L.: Assessing Community Resilience: An application of the expanded CART survey instrument with affiliated volunteer responders, Am. Behav. Sci., 59, 181–199, https://doi.org/10.1177/0002764214550295, 2015.
Philippe, F. L., Laventure, S., Beaulieu-Pelletier, G., Lecours, S., and Lekes, N.: Ego-Resiliency as a Mediator Between Childhood Trauma and Psychological Symptoms, J. Soc. Clin. Psychol., 30, 583–598, https://doi.org/10.1521/jscp.2011.30.6.583, 2011.
Portes, A.: Social Capital: Its Origins and Applications in Contemporary Sociology, Annu. Rev. Sociol., 24, 1–24, https://doi.org/10.1146/annurev.soc.24.1.1, 1998.
Putnam, R.: Bowling Alone: The Collapse and Revival of American Community, Simon & Schuster, New York, 2000.
Ruggiero, K. J., Amstadter, A. B., Acierno, R., Kilpatrick, D. G., Resnick, H. S., Tracy, M., and Galea, S.: Social and psychological resources associated with health status in a representative sample of adults affected by the 2004 Florida hurricanes, Psychiatr., 72, 195–210, https://doi.org/10.1521/psyc.2009.72.2.195, 2009.
Schumacher, J., Wilz, G., Gunzelmann, T., and Brähler, E.: Die Sense of Coherence Scale von Antonovsky. Teststatistische Uberprüfung in einer repräsentativen Bevölkerungsstichprobe und Konstruktion einer Kurzskala [The Antonovsky Sense of Coherence Scale. Test statistical evaluation of a representative population sample and construction of a brief scale], Psychotherapie, Psychosomatik, Medizinische Psychologie, 50, 472–482, https://doi.org/10.1055/s-2000-9207, 2000.
Sekulova, F. and van den Bergh, J. C. J. M.: Floods and happiness: Empirical evidence from Bulgaria, Ecol. Econ., 126, 51–57, https://doi.org/10.1016/j.ecolecon.2016.02.014, 2016.
Stein, D. J., Seedat, S., Iversen, A., and Wessely, S.: Post-traumatic stress disorder: Medicine and politics, The Lancet, 369, 139–144, https://doi.org/10.1016/S0140-6736(07)60075-0, 2007.
Tapsell, S. M. and Tunstall, S. M.: “I wish I'd never heard of Banbury”: The relationship between “place” and the health impacts from flooding, Health Place, 14, 133–154, https://doi.org/10.1016/j.healthplace.2007.05.006, 2008.
Thieken, A. H., Müller, M., Kreibich, H., and Merz, B.: Flood damage and influencing factors: New insights from the August 2002 flood in Germany, Water Resour. Res., 41, 314, https://doi.org/10.1029/2005WR004177, 2005.
Thieken, A. H., Bessel, T., Kienzler, S., Kreibich, H., Müller, M., Pisi, S., and Schröter, K.: The flood of June 2013 in Germany: how much do we know about its impacts?, Nat. Hazards Earth Syst. Sci., 16, 1519–1540, https://doi.org/10.5194/nhess-16-1519-2016, 2016.
Twigger-Ross, C., Coates, T., Deeming, H., Orr, P., Ramsden, M., and Stafford, J.: Community Resilience Research: Final Report on Theoretical research and analysis of Case Studies report to the Cabinet Office and Defence Science and Technology Laboratory, Appendix 1: Workshop Record, Collingwood Environmental Planning Ltd, London, 17 November 2011, 2011.
Van Ootegem, L. and Verhofstadt, E.: Well-being, life satisfaction and capabilities of flood disaster victims, Environ. Impact Asses., 57, 134–138, https://doi.org/10.1016/j.eiar.2015.12.001, 2016.
Von Möllendorff, C. and Hirschfeld, J.: Measuring impacts of extreme weather events using the life satisfaction approach, Ecol. Econ., 121, 108–116, https://doi.org/10.1016/j.ecolecon.2015.11.013, 2016.
Ware, J. E. and Sherbourne, C. D.: The MOS 36-item short-form health survey (SF-36), I. Conceptual framework and item selection, Med. Care, 30, 473–483, 1992.
Wheaton, B.: Models for the Stress-Buffering Functions of Coping Resources, J. Health Soc. Behav., 26, 352–364, https://doi.org/10.2307/2136658, 1985.
Whittle, R., Walker, M., Medd, W., and Mort, M.: Flood of emotions: Emotional work and long-term disaster recovery, Emot. Space Soc., 5, 60–69, https://doi.org/10.1016/j.emospa.2011.08.002, 2012.
Wind, T. R. and Komproe, I. H.: The mechanisms that associate community social capital with post-disaster mental health: A multilevel model, Soc. Sci. Med., 75, 1715–1720, https://doi.org/10.1016/j.socscimed.2012.06.032, 2012.
Wind, T. R., Fordham, M., and Komproe, I. H.: Social capital and post-disaster mental health, Global Health Action, 4, 6351, https://doi.org/10.3402/gha.v4i0.6351, 2011.