the Creative Commons Attribution 4.0 License.
the Creative Commons Attribution 4.0 License.
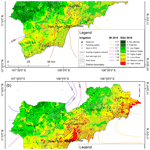
Spatial indicators for desertification in southeast Vietnam
Le Thi Thu Hien
Pham Thi Thanh Huong
Desertification is influenced by different factors that relate to climate, soil, topography, geology, vegetation, human pressure, and land and water management. The quantification of these factors into spatially explicit indicators and subsequent evaluation provides for a framework that allows us to identify areas currently at risk of desertification and to evaluate important contributing biophysical and socio-economic factors. Based on local knowledge of environmental contributing factors to the risk of desertification in the Binh Thuan Province of southeast Vietnam, a baseline 2010 map showed that 14.4 % of the area, mainly along the coast and in the northeast, is desertified with another 35.4 % at severe risk of desertification. The Vietnamese Ministry of Natural Resource and Environment has defined the area with a ratio of rainfall to evapotranspiration smaller or equal to 0.65, which equals 1233 km2 or 15 % of the province, as desertified area, which corresponds well with the baseline 2010 map. The developed framework incorporates the important contributing factors and therefore allows for decision support in a “what if” structure and for the projection of potentially vulnerable areas under future scenarios. With projected climate change and population growth, the desertified area is expected to increase by 122 % (or 137 850 ha) towards 2050. The developed methodology can be extended to neighbouring provinces that experience similar sensitivities to desertification.
Desertification is “land degradation in arid, semiarid and dry, sub-humid areas due to various factors, including climatic variations and human activities” (UNCCD, 2012). Land degradation in this context means the progressive loss of land productivity (Geist and Lambin, 2004). The United Nations Convention to Combat Desertification advocates methods to improve the global monitoring and assessment of dryland degradation to support decision-making in land and water management (UNCCD, 2012). Vietnam is highly affected by weather-related hazards that are projected to increase in frequency with climate change (Gobin et al., 2015). Vietnam is not designated as an arid or semiarid country. However, the coastal provinces in southeast Vietnam are strongly influenced by desertification, and therefore Vietnam ratified the convention in 1998 and formulated a national action programme in 2002.
The biophysical conditions related to desertification have originated models or frameworks involving the different processes of desertification to identify areas at risk (Schlesinger et al., 1990; Dirmeyer and Shukla, 1996; Kefi et al., 2007; Reynolds et al., 2007; Izzo et al., 2013; Jiang et al., 2019). Social, economic and in particular agricultural activities are considered important pressures impacting on land degradation and desertification (Okin et al., 2001; Asner et al., 2004; Zhou et al., 2015; Hamidov et al., 2016). Integrating the monitoring and assessment of human and environmental variables poses major methodological challenges (Winslow et al., 2011) when assessing spatial desertification risks (Izzo et al., 2013; Zhou et al., 2015). Desertified areas have been detected using a multi-factorial approach such as the multi-component geographic information system (GIS) framework for desertification risk assessment (Santini et al., 2010) or the environmentally sensitive areas (ESAs) approach (Kosmas et al., 2006; Basso et al., 2000). Though originally designed for European Mediterranean environments, the ESA methodology has increasingly been used for classifying sensitivity to desertification in different environments ranging from Egypt (Gad and Lotfy, 2008), Iran (Parvari et al., 2011) and Central Asia (Jiang et al., 2019) to the Dominican Republic (Izzo et al., 2013) and northwest China (Zhou et al., 2015). All of these approaches combine regional modelling methods with spatially explicit information to assess the sensitivity of different areas to desertification.
Monitoring and assessment of the underlying drivers of land condition changes help target remedial actions to alleviate true causes of land degradation (Gobin et al., 1999; Winslow et al., 2011; Zhou et al., 2015). The integration of socio-economic and biophysical factors is included in several assessment frameworks such as the dryland development paradigm (Reynolds et al., 2007; Stringer et al., 2017), the monitoring and assessment indicator system (Zucca et al., 2012), global drylands observing system (Verstraete et al., 2011; Bestelmeyer et al., 2015) and monitoring sustainable land management (Thomas, 2008; Hamidov et al., 2016). Focus on the integration of a particular component into a joint biophysical and socio-economic system is further elaborated for economic analysis (Salvati et al., 2008; Requier-Desjardins et al., 2011; Schild et al., 2018), population dynamics (e.g. Salvati and Bajocco, 2011; Schild et al., 2018), institutional knowledge (Stringer et al., 2009) and mainstreaming policies on degradation (Akhtar-Schuster et al., 2011). Eliciting the underlying factors that cause drought and desertification is a prerequisite to the further establishment of a monitoring and assessment system to support decision-making on land and water management. The driving factors often relate to socio-economic developments with land use changes taken as valuable proxy indicators (Geist and Lambin, 2004; Hill et al., 2008; Hamidov et al., 2016). The observed relation between degradation and changes in ecosystem productivity have triggered the incorporation of remote-sensing-derived indicators (Cherlet et al., 2018; Jiang et al., 2019).
We hypothesised that the area currently at severe risk of desertification can be explained by different socio-economic and biophysical factors, using local knowledge of environmental contributing factors as elucidated by Hai et al. (2013, 2014, 2016). The objectives were to develop a framework that integrates the important biophysical and socio-economic factors contributing to the risk of desertification, spatially delineate areas at risk of desertification in the year 2010 as a baseline, and demonstrate the developed framework for potential vulnerable areas under projected climate change and population growth. The method was developed for the Binh Thuan Province located in southeast Vietnam. The framework is designed to support policymakers in making informed decisions on combating desertification, analysing different scenarios and policy options, and formulating policies under “what if” conditions.
Vietnam has narrow deserts stretching along the central coastal areas, concentrated in 10 provinces from Quang Binh to Binh Thuan with a total area of about 419 000 ha. The US Department of Agriculture recognised this problem in Vietnam in its world map of vulnerable desertification (USDA-NRCS, 2003). In August 1998, Vietnam ratified the UNCCD and formulated priority areas for natural resources management. In 2006, the “Vietnamese action plan against desertification for the period 2006–2010 with an outlook to 2020” was adopted. Vietnamese studies on climate change and related environmental problems show that scientific, technological and policy responses are all needed for successful adaptation (Adger et al., 2005; Adger, 1999). Research has been implemented to study climate change phenomena and their effects such as sea level changes (Boateng, 2012; Zeidler, 1997), floods (Thi et al., 2010; Thanh et al., 2004), storms (Kleinen, 2007) and drought (Sinha et al., 2011). Desertification, however, has received little attention in Vietnam.
Binh Thuan Province is located in the southern part of Central Vietnam, covers an area of 7856 km2 and has about 250 km of coastline. Binh Thuan Province and the neighbouring Ninh Thuan Province have a typical semiarid climate with low rainfall, and high evaporation, with a variety of typical desert lands including sand, stone or salt deserts and degraded land. The Tuy Phong and Bac Binh districts (Fig. 1) face 6 to 9 dry months per year with less than 100 mm of monthly rainfall. The province is also subject to high temperatures and strong land winds that contribute to drought. The current desertification has a strong impact on overall production, environment and socio-economic activities.
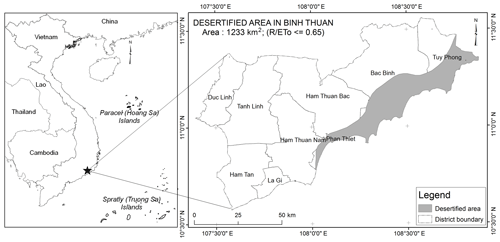
Figure 1Location of the desertified area in Binh Thuan Province in Vietnam, based on rainfall to evapotranspiration ratio smaller or equal to 0.65.
As part of the national action plan to combat desertification, the Vietnam Ministry of Natural Resource and Environment (MONRE) delineated the desertified zone in Binh Thuan based on an aridity index, i.e. ratio of rainfall to evapotranspiration smaller or equal to 0.65. The total desertified area is 1233 km2, corresponding to 15 % of the province (Fig. 1). Desertification, however, is the result of a variety of different factors and we therefore challenge the current delineation which is solely based on meteorological variables.
Based on local knowledge of environmental contributing factors to risk of desertification in the Binh Thuan Province of Vietnam (Hai et al., 2013, 2014, 2016), we included indicators for climate, soil-landscape classification, vegetation, water resources management, population density and human activities into a common framework. Each of these factors required a different methodology since different processes are involved that relate to land degradation and desertification. Indices were elaborated, compared with the current status of desertification and combined into zones at risk of desertification. The proposed scores for each index and indicator were implemented in a relational database, which allowed for transparent definition, the incorporation of socio-economic information and the iterative refinement of the selection rules in the future.
3.1 Database development
Spatial and/or temporal data were collected to characterise the climate, the soil-landscape classification, the vegetation, the water resources and the different human activities in the region. The resulting database contained information on soils (1:100 000); geology, hydro-geology, and hydrology; topography; natural vegetation; land use/cover change; population; and land and water resources. Soil resources, their characteristics and the IUSS WG WRB (2015) classification were obtained from the Department of Natural Resources and Environment of Binh Thuan province. Land cover changes were derived from Landsat enhanced thematic mapper (ETM) in 1994/1995 and SPOT4 in 2009/2010. The water resources potential for household use and agriculture, including irrigation, was derived from the hydrogeological database that includes groundwater reserves and exploitation, surface water distribution and flow characteristics, and operational and planned irrigation systems. Human activities were evaluated from land use in 2005 and 2010 and the province's master planning for 2010–2020 including a vision on 2050 (GSO, 2012). Population density and settlement distribution were obtained from statistics at the community level. In- and outward migration to and from the province included tourist activities. Economic statistics on agricultural activities covered data on cultivated land, crop types, grassland and livestock. Meteorological data included daily rainfall and daily minimum, maximum and mean temperature for the period 1960–2010 obtained from the Vietnam Institute of Meteorology.
3.2 Calculation of indicators
The scoring and scaling is explained for five different quality indicators (QIs): climate (CQI), soil (SQI), vegetation (VQI), water management (WMQI), and the different human activities and demographic pressures (HQI). Each quality indicator comprises different sub-indicators calculated for each square kilometre grid cell of the province (Fig. 2). Soil characteristics, vegetation type and characteristics, land cover/use, and water management were verified during field surveys in 2010. The scoring of the quality indicators was based on a multi-factorial approach combining the multi-component GIS framework for desertification risk assessment by Santini et al. (2010) and the environmentally sensitive areas (ESAs) approach by Kosmas et al. (2006) and Basso et al. (2000).
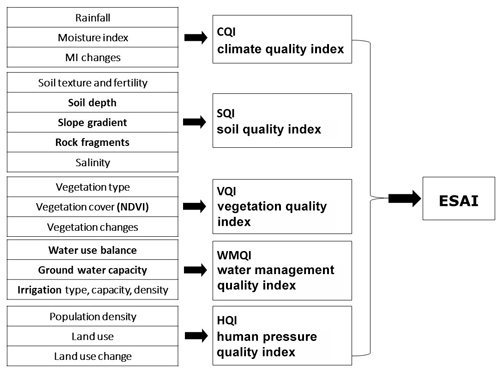
Figure 2Spatially distributed indices for identifying environmentally sensitive areas that are sensitive to desertification.
The climate quality indicator (CQI) is based on the aridity index (AI) and calculated for 13 meteorological stations according to the following:
where ΔAI1991−2010 is the change of the aridity index relative to a longer period, P is the yearly average annual rainfall and PET is the annual average potential evapotranspiration calculated according to the modified Penman–Monteith method (Allen et al., 1998) for the periods 1991–2010 and 1981–2010. Potential evapotranspiration requires air temperature, wind speed, radiation and air humidity variables. The aridity index, originally developed by FAO – UNESCO – WMO (1977) and based on temperature-based evapotranspiration (Thornthwaite, 1948), was subsequently adapted by UNEP (1997) for more recent periods and based on potential evapotranspiration. Further developments included time series analysis to assess temporal changes and climatic impact (e.g. Dai, 2013). We used values for AI below 0.35 to indicate severe risk of desertification, between 0.35 and 0.75 for risk of desertification, and above 0.75 to indicate no risk of desertification. The change during 1991–2010 (ΔAI1991−2010) was quantified as the weighted departure from the average for the period 1981–2010. Values for P, PET, AI and ΔAI were interpolated using a nearest-neighbour interpolation in ArcGIS Spatial Analyst, and classified after a scoring system. The scoring for annual rainfall was 1 (low) for more than 2000 mm; 1.2 for 1500–2000 mm; 1.4 for 1000–1500 mm; 1.6 for 500–1000 mm; 1.8 for 250–500 mm; and 2 for annual rainfall below 250 mm. The aridity index (AI) had score 2 when below 0.35; score 1.6 for values between 0.35 and 0.75; score 1.3 for values between 0.75 and 1.20; and score 1 for values above 1.20. The score was 1.1 for ΔAI values between −0.2 and 0 and 1 for values between 0 and 0.2.
The soil quality indicator (SQI) comprises a weighting of soil fertility, salinity, slope, soil depth, soil type and the presence of rocks. For each of the soil qualities, a higher score indicates a higher vulnerability to desertification and degradation.
The soil texture was derived from the World Reference Base for Soil Resources. Arenosols and Leptosols were considered most sensitive to desertification in the area and received a score of 2, followed by Salic Fluvisols (1.8), Thionic Fluvisols (1.6), Gleyi-Umbric Fluvisols (1.4), Acrisols (1.2), Ferralsols (1.1) and Luvisols (1). A shallow soil depth of less than 30 cm received a high score of 2, followed by 30–50 cm (1.5) and 50–100 cm (1.2); well-developed soils with a profile depth deeper than 100 cm received a score of 1. A slope above 25∘ received a score of 2; a score of 1.5 was given to slopes between 8 and 25∘; slopes between 3 and 8∘ received a score of 1.2; and slopes below 3∘ received a score of 1. The presence of salinity or rock fragments each received a score of 1.2, whereas their absence received a score of 1.
The vegetation quality indicator (VQI) comprises the Normalised Difference Vegetation Index (NDVI), changes in NDVI (ΔNDVI) and vegetation type.
The vegetation type (VT) was extracted from the 2010 forest and nature classification geo-database, which includes 18 vegetation classes ranging from broad-leaved evergreen humid forest to secondary natural dune vegetation. Each vegetation class was subsequently evaluated as having a high (2), medium (1.5) or low risk (1) of degradation. NDVI2010 was calculated as the average square kilometre value based on SPOT Vegetation images from 2010. NDVI changes were calculated from Landsat TM based on 1994/1995 images and SPOT on 2009/2010 images (ΔNDVI1995−2010). Both NDVI and vegetation types were scored according to their vulnerability to desertification and degradation. Dense forests and high NDVI values received lower scores than sparse forest cover or low NDVI values. NDVI values are an indication of vegetation greenness and health; a declining change in NDVI indicates degradation. An NDVI2010 value below or equal to 0.1 received a high scoring of 2; a value between 0.1 and 0.6 received a score of 1.5; and a value equal to or above 0.6 received a score of 1. ΔNDVI1995−2010 values above 0 were scored 2; values equal to 0 were scored 1.5; and values below 0 were scored 1.
The water management quality indicator (WMQI) has three major components that include an assessment of the water use balance, groundwater capacity and irrigation characteristics.
where WB is the water use balance calculated per irrigation perimeter, GW is the groundwater capacity, IT is the irrigation type, IC is the irrigation capacity and CD is the canal density. The water use balance (WB) was assessed from the volume of water extracted for irrigation and expressed as water short to meet irrigation demands in the irrigation perimeter. The water use balance was calculated as the balance between the total water demands for each agricultural crop and the water supply of each irrigation perimeter in 2010. Irrigation water supply discharges and cropping areas were provided by the water board but no exact location of the crops was possible; hence, we chose to score the perimeters on the basis of the water use balance. A shortage in the water use balance of above 5×107 m3 was scored 2, below 5×107 m3 scored 1.5 and no shortage was scored 1. The assessment of the groundwater capacity (GW) was based on discharge data. Zero well discharges received score 2, followed by discharges below 0.5 m3 h−1 (1.6), 0.5–5.0 m3 h−1 (score 1.3) and 5–10 m3 h−1 (score 1). The irrigation characteristics were the irrigation type (IT); the irrigation capacity (IC) in percentage of the area under operational irrigation; and the canal density (CD) calculated as the length of used irrigation canals per square kilometre grid. Three irrigation types were distinguished: no irrigation with score 2, supplementary irrigation with score 1.5 and full irrigation with score 1. For canal density use of 0 km km−2, a score of 2 was assigned; a density between 0 and 0.25 km km−2 received a score of 1.5; and a density above 0.25 received a score of 1. The irrigation capacity was scored as follows: 0 % (score 2); >0 %–30 % (score 1.6); >30 %–50 % (score 1.4); >50 %–<100 % (score 1.2); and 100 % (score 1).
The human pressure and activities quality indicator is assessed on the basis of land use, land use change and population density.
where LU is the land use in 2010; LUC is the land use change between 2005 and 2010; and PD is the 2010 population density. The classification and scoring focused on vulnerability to desertification (Kosmas et al., 1999). The land use was scored as follows: bare land (score 2); agriculture and settlements (score 1.5); and forest and water (score 1). Land use changes in bare land were scored 2; changes in forest and water to other land uses were scored 1.5; and the absence of changes were scored 1. A population density higher than 500 persons per kilometre received score 2; densities between 200 and 500 persons per kilometre received score 1.5; and densities below 200 persons per kilometre received score 1.
All the above quality indicators were combined into one indicator for assessing the risk of each square kilometre grid cell to desertification.
where ESAI is the environmentally sensitive area indicator, CQI is climate quality indicator, SQI is soil quality indicator, VQI is vegetation quality indicator, WMQI is water management quality indicator and HQI is human pressure and activities indicator.
We distinguished four major types of areas at risk based on the environmentally sensitive area indicator (ESAI). Critical areas, denoted “C”, included areas that were already desertified and presented a threat to the environment of the surrounding areas, with C3 having a high critical level (ESAI > 1.53); C2 having a mid-critical level (1.42 ≤ ESAI ≤ 1.53); and C1 having a low critical level (1.38 ≤ ESAI < 1.42). Fragile areas, denoted “F”, were areas in which any change in the balance of natural resources and human activities was likely to bring about degradation and desertification, with F3 having a high fragile level (1.33 ≤ ESAI < 1.38); F2 having a mid-fragile level (1.27 ≤ ESAI < 1.33); and F1 having a low fragile level (1.23 ≤ ESAI < 1.27). Potential areas at risk were areas threatened by degradation under significant climate change if a particular combination of land use was implemented or where off-site impacts would produce severe problems elsewhere (1.17 ≤ ESAI < 1.23). Areas not at risk were areas with a wet climate, well-drained areas, well-developed soils and/or with a dense vegetation cover; they were not considered to be threatened by desertification (ESAI < 1.17).
3.3 Scenarios
A scenario of climate change and population growth was established as an example of how the framework could be used to support policy options.
During the period 1958–2007, the average temperature in Vietnam increased by 0.5–0.7 ∘C. Vietnam's official scenarios for climate change (MONRE, 2009) fits these current trends. The emission scenario corresponds to an increase in temperature of 1 ∘C by 2050 and 2.4 ∘C by 2100 with respect to the 1980–1999 baseline period. Rainfall in the middle of the rainy season would increase 10 %–15 % with respect to the 1980–1999 baseline period in the South Central Coast area. On a yearly basis, rainfall is projected to increase by 1.7 % by 2050 and 3.2 % by 2100. The climate scenario used in this study corresponds to RCP4.5 with a radiative forcing of 4.5 W m−2 and 650 ppm CO2 equivalent in 2100. The climate scenario was downscaled at the different meteorological stations using the MAGICC/SCENGEN software (Wigley, 2008).
Based on governmental statistics (GSO, 2012) the province has a population of 1.1 million people with a population growth of 1.4 % during the last decade. Human activities and population growth were taken from the master planning for 2010–2020 including a vision for 2050. The vision includes planned irrigation schemes which will alter the water use balance in conjunction with climate change.
4.1 Climate
The aridity index (AI) showed the occurrence of semiarid regions in the northern coastal area (Fig. 3). Based on the AI comparison for different periods, drought is increasing in the northern and central coastal areas by up to 26 %, whereas in the northwest of the province AI has increased by 10 %. The value of CQI was high along the northern coastal area (Fig. 3), stretching to the south and gradually moving to the east of the province indicating enhanced sensitivity to desertification.
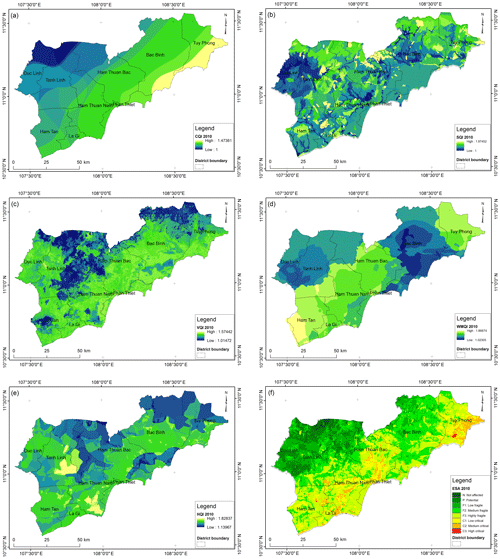
Figure 3Results of the climate quality indicator (CQI, a), soil quality indicator (SQI, b), vegetation quality indicator (VQI, c), water management quality indicator (WMQI, d), human pressure indicator (HQI, e) and the environmentally sensitive area indicator (ESAI, f).
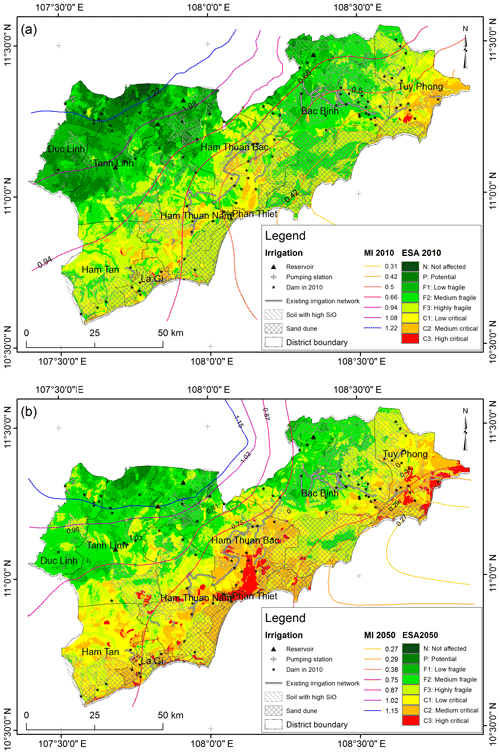
Figure 4Spatial distribution of the environmentally sensitive area indicator (ESAI) in 2010 (a) and projections of ESAI for 2050 (b) under climate change and population growth.
With climate change, an increase of 10.4 % in reference evapotranspiration was projected in the northwestern districts of Duc Linh, Tanh Linh and Ham Thuan Bac (for locations see Fig. 1 and Table 1); a 12.2 % increase in the south-central districts of Ham Thuan Nam, Ham Tan and Phan Thiet; and a 13.9 % increase in the eastern districts of Tuy Phong and Bac Binh. Rainfall was projected to increase by up to 5 % in the northwestern districts, remained the same in the central districts and decreased by more than 10 % in the northeastern districts. Overall this led to a projected decrease in the ratio of rainfall to evapotranspiration. Climate is a key factor as confirmed by similar studies in the region (MONRE, 2009). The distinction between climate regimes and gradients within the province could be seen from the AI isolines (Fig. 4).
Table 1Rainfall to reference evapotranspiration (P∕PET)* for 13 meteorological stations in the Binh Thuan Province for observed periods (1981–1990 and 1991–2010) and projected for 2050 according to MONRE's high climate scenario for Vietnam.
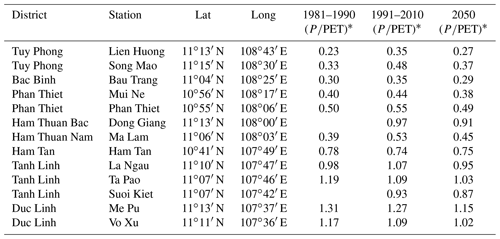
* Where P is rainfall and PET is the reference evapotranspiration calculated according to the modified Penman–Monteith method (Allen et al., 1998); For locations of the districts see Fig. 1.
4.2 Soil and vegetation
The soil quality characteristics were evaluated with areas at risk displaying high SQI values (Fig. 3). Poor soil quality due to shallow profile development, steep slopes or low fertility added to the risk. Deforested or eroded areas are mostly located in the mountains. Riverine areas with a good soil quality (low SQI, Fig. 3) offer opportunities for farming or are vegetated with dense forest. The soil quality indicator (SQI) provided the clearest relation with desertification but could not be interpreted on its own. Thin soils, steep slopes, vulnerability to erosion and sparse vegetation showed a high risk for degradation in the mountains, whereas the plains and coastal areas were at risk due to salinisation and sandy soil textures.
Forests covered nearly half of the province and were located in the province's mountainous regions in the northwest and northeast. The vegetation quality indicator (VQI) displayed a distinct pattern of low values (Fig. 3) in the east, where mountains with dense forests dominate the landscape, and high values in the southeast and coastal areas, where residential areas and agriculture are the principal land use. Low NDVI values indicated the presence of sparse vegetation with increased risks of degradation. At the same time, afforestation or reforestation are important mitigation measures to combat desertification. VQI therefore reflected both adaptation and mitigation measures.
4.3 Water management
Binh Thuan Province has three major rivers with several tributaries mostly originating in the province itself or in the highlands of the neighbouring province. Two major lakes and several artificial lakes add to the surface water bodies that, together with groundwater, provide for water during the dry season. Water resources management such as the development of sustainable irrigation systems help alleviate drought and desertification. A water management quality indicator (WMQI) was therefore included in the ESA methodology. The results showed the effect of irrigation on the WMQI in the northeast (Fig. 3). The canal density and irrigation capacity determined the amount of fields that could be irrigated during the dry season. The WMQI reflected the availability of water resources and exploitation potential. Water supply and management had a large impact on alleviating the negative effects of desertification (Fig. 4), and therefore represented important adaptation measures.
4.4 Human pressure
The human pressure was evaluated using population density and land use intensity, the latter as a combination of land use and land use change (Fig. 3). The population density ranged from around 1000 persons per square kilometre in Phan Thiet to less than 100 persons per square kilometre in the rural areas. As a result of strong population growth in the cities along the coast, urbanisation increased by 1 % during the last decade, making Binh Thuan one of the most urbanised provinces of the South Central Coast in Vietnam.
Table 2Surface area covered by areas at risk of desertification in the Binh Thuan Province in 2010 and projections for 2050, calculated using the environmentally sensitive area indicator.
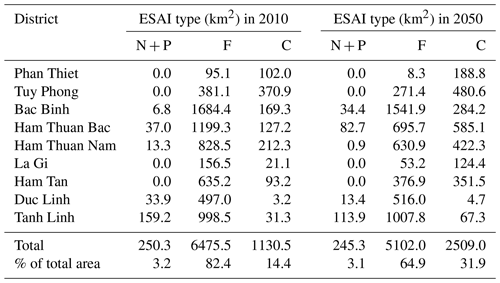
Where N means not affected; P means potential; F means fragile; and includes F1, F2 and F3; and C means critical and includes C1, C2 and C3. For locations, see Fig. 1.
The plains and coastal areas undergo a stronger human influence than the hills and mountains. Despite its large forested area, the province has about 37 % of agricultural land, which is the largest figure among all provinces of the central coast regions (GSO, 2012). One-third of the agricultural land is used to cultivate rice. Though many mountains showed clear signs of deforestation, there is an active policy towards reforestation and protection particularly for the dense forests in the northeast. The consequences of forest degradation may lead to further desertification and drought impacts on the coastal areas and plains. The human pressure and activities quality indicator (HQI) reflected the influence that population density could have on natural resources use. Higher population pressures resulted in land use and vegetation changes and gave rise to a higher water demand for both agricultural and household use.
4.5 Areas at risk of desertification
The areas at risk of degradation in the Binh Thuan Province (Fig. 3) show a zoning of sensitivity with around 85 % of the province. Desertified areas accounted for 14.4 % of the province, mainly the northeast and coastal areas. Another 35.4 % was highly fragile and at immediate risk of desertification; this area is yearly affected by severe drought occurring during the dry season. In the northern districts, the coastal zones suffer from shifting sand dunes and the plains from excessive drought and salinisation causing sparse vegetation and serious degradation. These districts experience a high risk of spreading desertification. According to the analysis, the most vulnerable districts (Table 2; Fig. 1) are located along the coastal areas of Tuy Phong (C: 370.9 km2 or 49.3 % of the district's area; F3: 288.1 km2 or 38.3 %), Ham Thuan Nam (C: 212.3 km2 or 20.1 %; F3: 503.1 km2 or 47.7 %), Bac Binh (C: 169.3 km2 or 9.1 %; F3: 686.7 km2 or 36.9 %) and Ham Thuan Bac (C: 127.2 km2 or 49.3 % of its area; F3: 486.6 km2 or 38.3 %) districts. The densely populated coastal district of Phan Thiet (C: 102 km2 or 51.8 %; F3: 91.2 km2 or 46.3 %) seemed very vulnerable to moving sand dunes.
The trend of desertification under climate change and population growth was towards the southwest with a projected increase of 1379 km2 land totalling 2509 km2 or 31.9 % of the entire province being desertified towards 2050. The affected area is enlarging most in the districts of Ham Thuan Bac by 458 km2, followed by Ham Tan by 258 km2 and Ham Thuan Nam by 210 km2 (Table 2; Fig. 1). The largest increases in desertification are expected in La Gi, Ham Thuan Bac and Ham Tan districts, where desertification is projected to increase by 490 %, 360 % and 277 %, respectively.
According to MONRE, the land affected by drought and desertification is about 43 % of the Binh Thuan Province based on an aridity index below 0.8, which reflects the occurrence of a 6-month dry season. A simple aridity index may provide a good indicator for the meteorological conditions but does not reflect the pressures nor the remedial actions that can be undertaken to alleviate the risk of desertification. In some areas irrigation systems have been developed along with sustainable management practices such as adapted cropping systems and policies to protect water resources through reforestation schemes, e.g. in Bac Binh District.
The world vulnerability map of desertification (USDA-NRCS, 2003) presents the southern coastal zone of Vietnam as sensitive with the northern coastal zone of the Binh Thuan Province highlighted as highly sensitive. Despite the years of difference between our analysis and the USDA map, the general picture is similar with the most sensitive areas being identified in both maps. In comparison to 1998, when Vietnam ratified the UNCCD, desertification has spread towards the southwest, a tendency that is continued under a scenario of climate change and population growth. Furthermore some areas in the centre have benefitted from irrigation schemes, whereas reforestation has taken place in parts of the northeast, changing the sensitivity to desertification and justifying the incorporation of land and water management indicators in the approach.
Desertification and land degradation is the result of many factors, the characteristics of which differ for each region as, for example, in the Dominican Republic (Izzo et al., 2013), Italy (Basso et al., 2000), northwest China (Zhou et al., 2015), Central Asia (Jiang et al., 2019), Nigeria (Gobin et al., 1999) and Iran (Gad et Lotfy, 2008). In the Binh Thuan Province, different indices influence the environmental sensitivity in a different manner as demonstrated for five comprehensive quality indices, i.e. climate, soil, vegetation, water management and human pressure. Climate impacts will alter in the first place the climate quality indicator (CQI) mainly through temperature rises and increased rainfall variability. Temperature rises affect evapotranspiration and the crop water demands, and the uncertain onset of the rainy season will affect crop water management and irrigation demands, both having an impact on water management (WMQI). The concept of the water footprint could help quantify crop water management (Gobin et al., 2017). Population growth alters the dynamics of land use demand and in turn affects water demands and water use balances. Land and water management seems a priority policy domain for alleviating the problems related to land degradation and desertification in the area.
The combination of different indices into the environmentally sensitive area indicator (ESAI) allows for identifying zones at risk of desertification and for eliciting the most important factors influencing desertification; it provides for a method that is applicable across different regions in the world (e.g. Izzo et al., 2013; Jiang et al., 2019). Monitoring the different spatial indicators and quality indices is an important part of an approach to combat desertification and allows for informed decision-making (Winslow et al., 2011). Subsequent studies could enlarge the area to regions at current or potential risk and could benefit from other land-degradation-related risk assessment research (Gobin et al., 1999; Kefi et al., 2007) or the incorporation of different indicators related to causes and processes of land degradation (Kosmas et al., 2014) such as soil morphology (Gobin et al., 2000), erosion (Gobin et al., 2004), or water balance and water stress (Gobin et al., 2017). Depending on data availability, these edaphic soil properties could be included to reflect soil–vegetation relationships. A perceived major shortcoming of the method is the subjective sensitivity scoring system, with scores typically in the range of 1.0 to 2.0 and based on existing research data or on the importance to land degradation and desertification (Kosmas et al., 2014). Future efforts could benefit from data mining techniques and machine learning to establish meaningful relations between the different indices, quality indicators and the risk of desertification. In addition, multi-criteria decision analysis could help optimise natural resources management and reduce the risk of desertification. The present scoring system, however, allows for easy comparison of the different contributing indicators between regions or zones and therefore elucidates the major contributing factors within a certain area.
The spatially explicit regional indices provide a comparative overview (Reynolds et al., 2007) and define areas where more detailed studies or policy attention are needed for determining the contributing factors and mitigating risks (Salvati et al., 2008; Hamidov et al., 2016). With changing scales, the dominant controlling factors such as, for example, land management, water resources development and vegetation cover may change (Gobin et al., 2001; Santini et al., 2010; Zhou et al., 2015). Individual farming practices may be different too such that remediation strategies at the community level must be adapted to this scale. Monitoring at multiple scales therefore provides for a nested strategy of focussing on environmentally sensitive areas (Gobin et al., 2004) which may require remedial measures to be taken at different levels of decision-making.
The approach of identifying environmentally sensitive areas based on a factorial combination of quality indicators, elucidated using local knowledge, allows for mapping current areas at risk of desertification and enables projecting trends. The area at risk of desertification in the Binh Thuan Province of southeast Vietnam is expected to increase from 14.4 % in 2010 to 31.9 % in 2050 under projected climate change and population growth.
The factorial combination of several indices into quality indicators proved useful for identifying environmentally sensitive areas now and under environmental change, and for elucidating relationships between factors and areas at risk of desertification. The key factors related human impacts to land cover, soil management and irrigation water capacity and can be influenced through policies encouraging the sustainable management of natural resources. The developed methodology can be extended to neighbouring areas experiencing similar environmental threats.
Data can be made available by the authors upon request.
LTTH was responsible for conducting surveys, creation and analysis of geographic data (land use, forests, socio-economic data) and creating a geo-database for the region, and contributed to drafting the manuscript. AG was responsible for part of the survey data (soil, water balance, vegetation, land cover), comprehensive geo-database analysis and writing the manuscript. PTTH was responsible for the analysis of the meteorological and hydrological data, and for climate scenarios.
The authors declare that they have no conflict of interest.
This article is part of the special issue “Remote sensing, modelling-based hazard and risk assessment, and management of agro-forested ecosystems”. It is a result of the EGU General Assembly, Vienna, Austria, 8–13 April 2018.
The authors would like to thank the Vietnam Academy of Science and Technology (VAST.DLT.13/13-14) and the Belgian Science Policy Office (BELSPO BL/03/V28) for funding the research under an umbrella bilateral cooperation agreement between Vietnam and Belgium. The authors are indebted to the People's Committee of the Binh Thuan Province; the Department of Science and Technology; the Department of Natural Resources and Environment; the Institute of Meteorology, Hydrology and Environment; and the Institute of Geography for contributing data and scientific reports. The authors are grateful to three anonymous reviewers for their critical comments that helped improve the article.
This research has been supported by the Belspo (grant no. BL/03/V28) and the Vietnam Academy of Science and Technology (grant no. VAST.DLT.13/13-14).
This paper was edited by Ana Maria Tarquis and reviewed by three anonymous referees.
Adger, W. N.: Social vulnerability to climate change and extremes in coastal Vietnam, World Dev., 27, 249–269, 1999.
Adger, W. N., Arnell, N. W., and Tompkins, E. L.: Successful adaptation to climate change across scales, Global Environ. Change, 15, 77–86, https://doi.org/10.1016/j.gloenvcha.2004.12.005, 2005.
Akhtar-Schuster, M., Thomas, R. J., Stringer, L. C., Chasek, P., and Seely, M.: Improving the enabling environment to combat land degradation: Institutional, financial, legal and science-policy challenges and solutions, Land Degrad. Dev., 22, 299–312, https://doi.org/10.1002/ldr.1058, 2011.
Allen, R. G., Pereira, L. S., Raes, D., and Smith, M.: Crop Evapotranspiration: Guidelines for Computing Crop Requirements, Irrigation and Drainage Paper No. 56, FAO, Rome, Italy, p. 300, 1998.
Asner, G. P., Elmore, A. J., Olander, L. P., Martin, R. E., and Harris, A. T.: Grazing systems, ecosystem responses, and global change, Annu. Rev. Environ. Resour., 29, 261–299, https://doi.org/10.1146/annurev.energy.29.062403.102142, 2004.
Basso, F., Bove, E., Dumontet, S., Ferrara, A., Pisante, M., Quaranta, G., and Taberner, M.: Evaluating Environmental Sensitivity at the basin scale through the use of Geographic Information Systems and Remote Sensed data: an example covering the Agri basin southern Italy, Catena, 40, 19–35, https://doi.org/10.1016/S0341-8162(99)00062-4, 2000.
Bestelmeyer, B. T., Okin, G. S., Duniway, M. C., Archer, S. R., Sayre, N. F., Williamson, J. C., and Herrick, J. E.: Desertification, land use, and the transformation of global drylands, Front. Ecol. Environ., 13, 28–36, https://doi.org/10.1890/140162, 2015.
Boateng, I.: GIS assessment of coastal vulnerability to climate change and coastal adaption planning in Vietnam, J. Coast. Conserv., 16, 25–36, https://doi.org/10.1007/s11852-011-0165-0, 2012.
Cherlet, M., Hutchinson, C., Reynolds, J., Hill, J., Sommer, S., and von Maltitz, G.: World Atlas of Desertification, Publication Office of the European Union, Luxembourg, https://doi.org/10.2760/06292, 2018.
Dai, A.: Increasing drought under global warming in observations and models, Nat. Clim. Change, 3, 52–58, 2013.
Dirmeyer, P. A. and Shukla, J.: The effect on regional and global climate of expansion of the world's deserts, Q. J. Roy. Meteorol. Soc., 122, 451–482, https://doi.org/10.1256/smsqj.53007, 1996.
FAO – UNESCO – WMO: World Map of Desertification, Food and Agricultural Organization, Rome, 1977.
Gad, A. and Lotfy, I.: Use of remote sensing and GIS in mapping the environmental sensitivity areas for desertification of Egyptian territory, Earth Discuss., 3, 41–85, 2008.
Geist, H. J. and Lambin, E. F.: Dynamic causal patterns of desertification, Bioscience, 54, 817–829, 2004.
Gobin, A., Campling, P., Deckers, J., and Feyen, J.: Quantifying soil morphology: methods and application in soil-landscape classification, Soil Sci. Soc. Am. J., 64, 1423–1433, 2000.
Gobin, A., Campling, P., Deckers, J., and Feyen, J.: Integrated land resources analysis with an application to Ikem (south-eastern Nigeria), Landsc. Urban Plan., 53, 95–109, https://doi.org/10.1016/S0169-2046(00)00143-2, 2001.
Gobin, A., Jones, R., Kirkby, M., Campling, P., Kosmas, C., Govers, G., and Gentile, A. R.: Pan-European assessment and monitoring of soil erosion by water, J. Environ. Sci. Policy, 7, 25–38, https://doi.org/10.1016/j.envsci.2003.09.004, 2004.
Gobin, A., Nguyen, T. H., Pham, Q. V., and Pham, T. T. H.: Patterns of heavy rainfall in Vietnam and their relation with ENSO cycles, Int. J. Climatol., 36, 1686–1699, https://doi.org/10.1002/joc.4451, 2015.
Gobin, A., Kersebaum, K. C., Eitzinger, J., Trnka, M., Hlavinka, P., Takáč, J., Kroes, J., Ventrella, D., Dalla Marta, A., Deelstra, J., Lalić, B., Nejedlik, P., Orlandini, S., Peltonen-Sainio, P., Rajala, A., Saue T., Şaylan, L., Stričevic, R., Vučetić, V., and Zoumides, C.: Variability in the water footprint of arable crop production across European regions, Water, 9, 93, https://doi.org/10.3390/w9020093, 2017.
Gobin, A. M., Campling, P., Deckers, J. A., Poesen, J., and Feyen, J.: Soil erosion assessment at the Udi-Nsukka Cuesta (southeastern Nigeria), Land Degrad. Dev., 10, 141–160, https://doi.org/10.1002/(SICI)1099-145X(199903/04)10:2<141::AID-LDR325>3.0.CO;2-N, 1999.
GSO: Statistical Yearbooks of Vietnam 1990–2011, General Statistics Office, Statistical Publishing House, Hanoi, 2012.
Hai, L. T., Gobin, A., and Hens, L.: Risk assessment of desertification for Binh Thuan Province, Vietnam, Human Ecol. Risk Assess., 19, 1544–1556, https://doi.org/10.1080/10807039.2012.716688, 2013.
Hai, L. T., Gobin, A., and Hens, L.: Uncovering causes and effects of desertification using a Leopold matrix in Binh Thuan Province, Vietnam, Chin. J. Populat. Resour. Environ., 12, 57–67, https://doi.org/10.1080/10042857.2014.883052, 2014.
Hai, L. T., Gobin, A., and Hens, L.: Select indicators and prioritize solutions for desertification and drought in Binh Thuan, Vietnam, Chin. J. Populat. Resour. Environ., 14, 123–132, https://doi.org/10.1080/10042857.2016.1177315, 2016.
Hamidov, A., Helming, K., and Balla, D.: Impact of agricultural land use in Central Asia: a review, Agron. Sustain. Dev., 36, 6–11, https://doi.org/10.1007/s13593-015-0337-7, 2016.
Hill, J., Stellmes, M., Udelhoven, T., Röder, A., and Sommer, S.: Mediterranean desertification and land degradation. Mapping related land use change syndromes based on satellite observation, Global Planet. Changes, 64, 146–157, https://doi.org/10.1016/j.gloplacha.2008.10.005, 2008.
IUSS WG WRB: International Union of Soil Sciences Working Group World reference base for soil resources 2014 (update 2015), international soil classification system for naming soils and creating legends for soil maps, in: World Soil Resources Reports, FAO, Rome, 2015.
Izzo, M., Araujo, N., Aucelli, P. P. C., Maratea, A., and Sánchez, A.: Land sensitivity to desertification in the Dominican Republic: an adaptation of the ESA methodology, Land Degrad. Dev., 24, 486–498, https://doi.org/10.1002/ldr.2241, 2013.
Jiang, L., Bao, A., Jiapaer, G., Guo, H., Zheng, G., Gafforov, K., Kurban, A., and De Maeyer, P.: Monitoring land sensitivity to desertification in Central Asia: Convergence or divergence?, Sci. Total Environ., 658, 669–683, https://doi.org/10.1016/j.scitotenv.2018.12.152, 2019.
Kefi, S., Rietkerk, M., Alados, C. L., Pueyo, Y., Papanastasis, V. P., ElAich, A., and de Ruiter, P. C.: Spatial vegetation patterns and imminent desertification in Mediterranean arid ecosystems, Nature, 449, 213–2018, https://doi.org/10.1038/nature06111, 2007.
Kleinen, J.: Historical perspectives on typhoons and tropical storms in the natural and socio-economic system of Nam Dinh (Vietnam), J. Asian Earth Sci., 29, 523–531, https://doi.org/10.1016/j.jseaes.2006.05.012, 2007.
Kosmas, C., Kirkby, M., and Geeson, N.: Manual on key indicators of desertification and mapping environmentally sensitive areas (ESA) to desertification, European Commission, Brussels, 1999.
Kosmas, C., Tsara, M., Moustakas, N., Kosma, D., and Yassoglou, N.: Environmental sensitive areas (ESA) and indicators of desertification, in: Desertification in the Mediterranean region. A security issue, NATO Security Through Science Series Volume 3, NATO, Brussels, 2006.
Kosmas, C., Kairis, O., Karavitis, C., Ritsema, C., Salvati, L., Acikalin, S., Alcalá, M., Alfama, P., Atlhopheng, J., Barrera, J., and Belgacem, A.: Evaluation and selection of indicators for land degradation and desertification monitoring: methodological approach, Environ. Manage., 54, 951–970, 2014.
MONRE – Ministry of Natural Resources and Environment, Binh Thuan Department: Climate change and sea level rise scenarios for Vietnam, Hanoi, Vietnam, available at: http://www.preventionweb.net/files/11348_ClimateChangeSeaLevelScenariosforVi.pdf (last access: 1 May 2019), 2009.
Okin, G. S., Murray, B., and Schlesinger, W. H.: Degradation of sandy arid shrubland environments: observations, process modelling, and management implications, J. Arid Environ., 47, 123–144, https://doi.org/10.1006/jare.2000.0711, 2001.
Parvari, S.H., Pahlavanravi, A., Nia, A. R. M., Dehvari, A., and Parvari, D.: Application of methodology for mapping environmentally sensitive areas (ESAs) to desertification in dry bed of Hamoun wetland (Iran), Int. J. Nat. Resour. Mar. Sci., 1, 65–80, https://doi.org/10.5775/fg.2067-4635.2012.031.i, 2011.
Requier-Desjardins, M., Adhikari, B., and Sperlich, S.: Some notes on the economic assessment of land degradation, Land Degrad. Dev., 22, 285–298, https://doi.org/10.1002/ldr.1056, 2011.
Reynolds, J. F., Stafford Smith, D. M., Lambin, E. F., Turner, B. L., Mortimore, M., Batterbury, S. P. J., Downing, T. E., Dowlatabadi, H., Fernandez, R. J., Herrick, J. E., Huber-Sannwald, E., Jiang, H., Leemans, R., Lynam, T., Maestre, F. T., Ayarza, M., and Walker, B.: Global desertification: Building a science for dryland development, Science, 316, 847–851, https://doi.org/10.1126/science.1131634, 2007.
Salvati, L. and Bajocco, S.: Land sensitivity to desertification across Italy: Past, present, and future, Appl. Geogr., 31, 223–231, https://doi.org/10.1016/j.apgeog.2010.04.006, 2011.
Salvati, L., Zitti, M., and Ceccarelli, T.: Integrating economic and environmental indicators in the assessment of desertification risk: A case study, Appl. Ecol. Environ. Res., 6, 129–138, 2008.
Santini, M., Caccamo, G., Laurenti, A., Noce, S., and Valentini, R.: A multi-component GIS framework for desertification risk assessment by an integrated index, Appl. Geogr., 30, 394–415, https://doi.org/10.1016/j.apgeog.2009.11.003, 2010.
Schild, J. E., Vermaat, J. E., de Groot, R. S., Quatrini, S., and van Bodegom, P. M.: A global meta-analysis on the monetary valuation of dryland ecosystem services: The role of socio-economic, environmental and methodological indicators, Ecosyst. Serv., 32, 78–89, https://doi.org/10.1016/j.ecoser.2018.06.004, 2018.
Schlesinger, W. H., Reynolds, J. F., Cunningham, G. L., Huenneke, L. F., Jarrell, W. M., Virginia, R. A., and Whitford, W. G.: Biological Feedbacks in Global Desertification, Science, 247, 1043–1048, https://doi.org/10.1126/science.247.4946.1043, 1990.
Sinha, A., Stott, L., Berkelhammer, M., Cheng, H., Edwards, R. L., Buckley, B., Aldenderfer, M., and Mudelsee, M.: A global context for megadroughts in monsoon Asia during the past millennium, Quaternary Sci. Rev., 30, 47–62, https://doi.org/10.1016/j.quascirev.2010.10.005, 2011.
Stringer, L. C., Dyer, J. C., Reed, M. S., Dougill, A. J., Twyman, C., and Mkwambisi, D.: Adaptations to climate change, drought and desertification: local insights to enhance policy in southern Africa, Environ. Sci. Policy, 12, 748–765, https://doi.org/10.1016/j.envsci.2009.04.002, 2009.
Stringer, L. C., Reed, M. S., Fleskens, L., Thomas, R. J., Le, Q. B., and Lala-Pritchard, T.: A new dryland development paradigm grounded in empirical analysis of dryland systems science, Land Degrad. Dev., 28, 1952–1961, https://doi.org/10.1002/ldr.2716, 2017.
Thanh, D. T., Saito, Y., Dinh, V. H., Nguyen, V. L., Thi, K. O. T., and Tateishi, M.: Regimes of human and climate impacts on coastal changes in Vietnam, Reg. Environ. Change, 4, 49–62, https://doi.org/10.1007/s10113-003-0062-7, 2004.
Thi, T. H. P., Sunada, K., Oishi, S., and Sakamoto, Y.: River discharge in the Kone River basin (Central Vietnam) under climate change by applying the BTOPMC distributed hydrological model, J. Water Clim. Change, 1, 269–279, https://doi.org/10.2166/wcc.2010.046, 2010.
Thomas, R. J.: 10th Anniversary Review: Addressing land degradation and climate change in dryland agroecosystems through sustainable land management, J. Environ. Monit., 10, 595–603, https://doi.org/10.1039/b801649f, 2008.
Thornthwaite, C. V.: An approach towards a rational classification of climate, Geogr. Rev., 38, 55–94, 1948.
UNCCD: Desertification: a visual synthesis, available at: http://www.preventionweb.net/go/29359 (last access: 25 October 2019), 2012.
UNEP: World Atlas of Desertification, 2nd Edn., United Nations Environment Programme, Arnold, London, p. 182, 1997.
USDA-NRCS: United States Department of Agriculture – Natural Resources Conservation Service, Global Desertification Vulnerability Map, available at: https://www.nrcs.usda.gov/wps/portal/nrcs/detail/soils/use/worldsoils/?cid=nercs142p2_054003 (last access: 25 October 2019), 2003.
Verstraete, M. M., Hutchinson, C. F., Grainger, A., Smith, M. S., Scholes, R. J., Reynolds, J. F., Barbosa, P., Leon, A., and Mbow, C.: Towards a Global Drylands Observing System: Observational Requirements And Institutional Solutions, Land Degrad. Dev., 22, 198–213, https://doi.org/10.1002/ldr.1046, 2011.
Wigley T.: MAGICC/SCENGEN 5.3: user manual, National Center for Atmospheric Research, Boulder, Colorado, available at: http://www.cgd.ucar.edu/cas/wigley/magicc/UserMan5.3.v2.pdf (last access: 1 May 2019), 2008.
Winslow, M. D., Vogt, J. V., Thomas, R. J., Sommer, S., Martius, C., and Akhtar-Schuster, M.: Editorial to Science for improving the monitoring and assessment of dryland degradation, Land Degrad. Dev., 22, 145–149, https://doi.org/10.1002/ldr.1086, 2011.
Zeidler, R. B.: Continental shorelines: climate change and integrated coastal management, Ocean Coast. Manage., 37, 41–62, https://doi.org/10.1016/S0964-5691(97)87434-0, 1997.
Zhou, W., Gang, C., Zhou, F., Li, J., Dong, X., and Zhao, C.: Quantitative assessment of the individual contribution of climate and human factors to desertification in northwest China using net primary productivity as an indicator, Ecol. Indicat., 48, 560–569, https://doi.org/10.1016/j.ecolind.2014.08.043, 2015.
Zucca, C., Della Peruta, R., and Salvia, R.: Towards a World Desertification Atlas. Relating and selecting indicators and data sets to represent complex issues, Ecol. Indicat., 15, 157–170, https://doi.org/10.1016/j.ecolind.2011.09.012, 2012.
what ifstructure, which can be extended to regions that experience similar sensitivities.