the Creative Commons Attribution 4.0 License.
the Creative Commons Attribution 4.0 License.
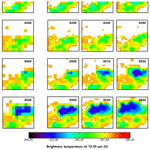
Effects of high-resolution geostationary satellite imagery on the predictability of tropical thunderstorms over Southeast Asia
Kwonmin Lee
Hye-Sil Kim
Tropical thunderstorms cause significant damage to property and lives, and a strong research interest exists in the advances and improvement of thunderstorm predictability by satellite observations. Using high-resolution (2 km and 10 min) imagery from the geostationary satellite, Himawari-8, recently launched over Southeast Asia, we examined the earliest possible time for the prediction of thunderstorms as compared to the potential of low-resolution (4 km and 30 min) imagery of the former satellite. We compared the lead times of high- and low-resolution imageries of 60 tropical thunderstorms that occurred in August 2017. These thunderstorms were identified by the decreasing trend in the 10.45 µm brightness temperature (BT11) by over 5 K per 10 min for the high-resolution imagery and 15 K per 30 min for the low-resolution imagery. The lead time was then calculated over the time from the initial state to the mature state of the thunderstorm, based on the time series of a minimum BT11 of thunderstorm pixels. The lead time was found to be 90–180 min for the high-resolution imagery, whereas it was only 60 min (if detectable) for the low-resolution imagery. These results indicate that high-resolution imagery is essential for substantial disaster mitigation owing to its ability to raise an alarm more than 2 h ahead of the mature state of a tropical thunderstorm.
Climate change adaptation and disaster risk management integration have become increasingly important issues since unpredictable natural hazards have started to appear more frequently and intensively in the recent warmer climate (Shaw et al., 2010). Impacts from recent climate-related extremes, such as heat waves, droughts, floods, cyclones, and wildfires, reveal the significant vulnerability and exposure of some ecosystems and many human systems to current climate variability (Pachauri and Meyer, 2014). These severe events cause extensive economic loss, environmental degradation, and subsequent damage to human life.
Multiple hazards can be involved simultaneously or in quick succession in an extreme weather event. For example, a tropical storm can lead to flooding, a storm surge, coastal inundation, and mudslides with high winds and heavy rain (Nastos et al., 2016). Thus, it is important to quickly detect the early clouds before they have reached their mature state to reduce disaster damage. This early detection and prediction are based on the high possibility that early clouds can be accompanied by heavy rain or lightning in their mature state (Houze Jr. and Betts, 1981). Aimed at disaster risk reduction, the European Operational Program for Exchange of Weather Radar Information (OPERA) continuously provides precipitation data of higher spatial resolution over a large area (Huuskonen et al., 2014). The Next Generation Weather Radar (NEXRAD) system (Klazura and Imy, 1993) has been employed for this purpose in the United States.
However, it is challenging to predict a tropical convective system because numerical weather prediction models generally have coarser spatiotemporal resolution than a deep convective clouds area with short life spans (Avotniece et al., 2017). In Southeast Asia, not only is the prediction model imperfect, but the observational data that support it are also insufficient. To make matters worse, unlike in the middle latitudes, the tropical atmosphere is conditionally unstable, hindering the predictive accuracy of tropical thunderstorms by such models. Hence, alarms for the hazards in the tropics are generally managed by the nowcasting system of real-time observations from radar and meteorological satellites.
The usefulness of the observations by geostationary satellites has been particularly emphasized for convective clouds, considering their extensive spatial coverage (Escrig et al., 2013). Satellites can observe clouds regardless of their location (over land or the ocean) and can also monitor the spans of clouds along their tracks (de Coning et al., 2015). Furthermore, geostationary satellite imagers have greatly advanced in recent years. For example, high-resolution interval imagery with a resolution of 2 km and 10 min by the Himawari-8 satellite operated by the Japan Meteorological Agency (JMA) has been primarily available from geostationary orbit since 2015 (Bessho et al., 2016). Similar-resolution imageries can be obtained by several geostationary satellites: satellites of the Geostationary Operational Environmental Satellite (GOES) network (Menzel and Purdom, 1994), Fengyun-4 (FY-4) (Yang et al., 2017), and the Geostationary Korea Multi-Purpose Satellite-2A (GEO-KOMPSAT-2A; GK2A) (Choi and Ho, 2015). These high-resolution imageries have high potential to improve thunderstorm monitoring. Moreover, these satellites are not susceptible to hazards and continuously provide enhanced thunderstorm information, which is much wider, more specific, and more accurate than that obtained by ground measurements.
As the number of recent meteorological geostationary satellites loading the enhanced imager increases, people expect to receive higher-quality weather information than was available in the past. However, there has been a lack of prior attempts to quantify the predictability of thunderstorms using geostationary infrared imagers. In particular, it has been uncertain how much earlier thunderstorms can be predicted using the improved imagery. Therefore, in this study, we focused on the dependence of tropical thunderstorm predictability on spatiotemporal imagery resolutions. We investigated the predictability of the initial state of thunderstorms over Southeast Asia using the Himawari-8 satellite.
The region examined in this study is from 10 to 20∘ N and from 100 to 120∘ E and is closely monitored by the Mekong River Commission. “The Mekong River Commission (MRC) is the only inter-governmental organisation that works directly with the governments of Cambodia, Lao PDR, Thailand and Viet Nam to jointly manage the shared water resources and the sustainable development of the Mekong River” (MRC, 2018). Unfortunately, this area is known as a vulnerable disaster region because of its high risk of extreme weather. The global impacts of climate change have contributed to changes in the weather patterns that are felt across the Mekong River Commission region. The warmer atmosphere has the potential to contain more moisture, which increases the possibilities for invigorating thunderstorms if other conditions are equal. The temperature increase associated with global climate change was generally assumed to lead to increased thunderstorm intensity and associated heavy precipitation events (Schefczyk et al., 2015).
Disasters occurring after tropical thunderstorms are distinctly common during the wet season. The Himawari-8 Advanced Himawari Imager (AHI) red-green-blue (RGB) image of the study area, taken at 05:50 UTC on 19 August 2015, can be seen in Fig. 1. Several convective clouds (pictured in white) are shown in the southern part of the area. Normally, in the diurnal cycle of the tropics, oceanic deep convection generally tends to reach its maximum in the morning, and continental convection peaks in the evening, although there are interesting regional variations (Yang and Slingo, 2001). Therefore, we conducted observations within the intervals of 03:00–06:50 UTC (daytime) and 21:00–00:50 UTC (nighttime), when the potential frequently thunderstorm occurrence is higher due to the diurnal cycle specific for the tropics. Table 1 lists the number of selected convective clouds that are developing within 2 h. Sixty clouds, excluding those that had already developed, were selected if their cloudy pixels first began to be detected at 03:00–06:50 UTC (daytime) and 21:00–00:50 UTC (nighttime) during July and August 2017.
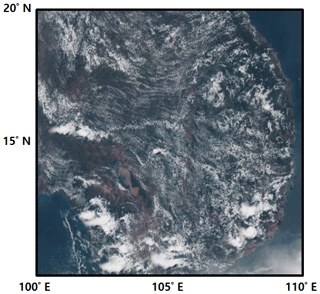
Figure 1Himawari-8 AHI RGB image taken for this study area on 19 August 2015 at 05:50 UTC. Several convective clouds (pictured in white) are visible in the southern part of the area.
Himawari-8 is one of the several launched geostationary meteorological satellites capable of observing Southeast Asia. Himawari-8 has 16 spectral bands, 11 more than the previous satellite, the Multi-Functional Transport Satellite-1R/2 (MTSAT-1R/2), as presented in Table 2. Specifically, the spatial resolution is doubled and the time interval is tripled as compared to those of the former. Himawari-8 can scan five areas, each of which with a different time cycle. The area of Japan and the target area were observed every 2.5 min, and the landmark area was observed every 0.5 min (Table 2). However, in the region of interest of this study, convective cloud observations were possible every 10 min based on the full disk (images from the JMA's Meteorological Satellite Center (JMA/MSC); Himawari-8/9 Imagery (AHI), 2018).
Table 2Spatial resolution and the time interval of the Advanced Himawari Imager (AHI) for Himawari-8 and the MTSAT-1R/2 Imager (images from the JMA/MSC; Himawari-8/9 Imagery (AHI), 2018).
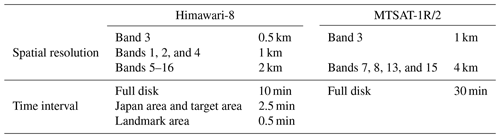
Among the 16 existing bands, the brightness temperature at 10.45 µm (BT11) was used for monitoring the vertical growth of clouds. The wavelength of 10.45 µm was less sensitive to ozone or water vapor in the atmosphere than any other of the infrared window bands (Schmit et al., 2005). Thus, the BT11 for cumulus and convective clouds was closely related to the cloud top temperature. The colder cloud top and larger cloud thickness as the clouds developed vertically effectively reduced the BT11. Of note, in this study, we used only the infrared band at 10.45 µm, which is the channel common to both Himawari-8 and MTSAT-1R/2.
To perform this study, we created virtual data whose resolution was similar to those of MTSAT-1R/2 (Table 2). Specifically, 4 pixels at a resolution of 2 km were converted into 1 pixel at a resolution of 4 km, and the time interval was increased from 10 to 30 min. In other words, it was calculated as the average of 4 pixels with a resolution of 2 km in the process of observing clouds every 30 min. The number of detected cloudy pixels by resolution is illustrated in Fig. 2. A tropical thunderstorm was found to be located in the area of 12 km×12 km pixels. The dark-grey shading indicates the detected cloudy pixel, and the light-grey shading indicates the clear-sky pixel. Using the 4 km resolution imagery only 2 cloudy pixels were detected in the middle area with the 4 km resolution imagery; in contrast, 18 cloudy pixels can be detected with the 2 km resolution imagery. It is noteworthy that the high-resolution imagery was able to detect cloudy pixels located at a curved boundary. However, the low-resolution imagery tended to simplify the change rate of the minimum BT11, and the detection of cloudy pixels at a curved boundary was somewhat hard (Walker et al., 2012). Hereafter, the virtual MTSAT is called the low-resolution (4 km and 30 min) imagery and the Himawari-8 is called the high-resolution (2 km and 10 min) imagery so as to facilitate the intuitive understanding of the spatiotemporal resolution difference. Our final study aim was to quantitatively compare their effectiveness in the advanced predictability of tropical thunderstorms through the imageries of geostationary satellites.
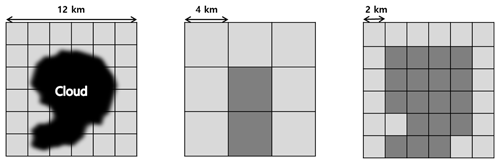
Figure 2Illustrations of 12 km×12 km pixels with different resolutions. The dark-grey shading indicates the cloudy pixel, and the light-grey shading indicates the clear-sky pixel. Only 2 cloudy pixels can be detected with the 4 km resolution imagery; in contrast, 18 cloudy pixels can be detected with the 2 km resolution imagery. The number of pixels at the cloud boundary varies depending on the resolution (Walker et al., 2012).
Sixty thunderstorms were subjectively selected based on the RGB images over Southeast Asia. The size of the selected thunderstorms was determined to be less than 120 km because such convective scales typically accompany precipitation (Houze Jr., 2004). We set the rectangular target boundaries depending on the thunderstorm size. In the target boundary, the BT11 values were monitored to determine the thunderstorm pixels and phases (initial or mature states) for the whole life cycle of thunderstorms. Since temporal changes in the BT11 inform the vertical drift velocity, the current status of the clouds can be a key to diagnosing the probability of imminent heavy rains or lightning (Vila et al., 2008).
The thunderstorm pixels in the target boundaries were identified by the decreasing trend in the BT11 by over 5 K per 10 min for the high-resolution imagery or 15 K per 30 min for the low-resolution imagery. During the observation time, the initial state was defined as the moment when the thunderstorm pixels were initially detected in the target boundaries. The mature state was defined as the last moment when the minimum BT11 among thunderstorm pixels decreased gradually below 230 K. The minimum BT11 near 230 K meant that the ice cloud effective radius in the thunderstorms was maximized (Kahn et al., 2018). The large ice cloud effective radius is closely related to rain formation because the cloud drop size has an impact on the cloud growth rate (Wang, 2013).
The lead time was defined as the time between the initial state and the mature state. Figure 3 clearly shows the method used for the calculation of the lead time from the initial or mature state of a thunderstorm. The solid line in Fig. 3 represents an example of the temporal changes in the minimum BT11 among thunderstorm pixels (10 August 2017, 03:10–05:50 UTC). The high-resolution imagery is depicted as the circle, and the low-resolution imagery is depicted as the triangle. Negative time indicates the time ahead of the mature state of a tropical thunderstorm. Consequentially, the lead time of the targeted thunderstorm for the low-resolution imagery was 30 min, and that for the high-resolution imagery was 160 min.
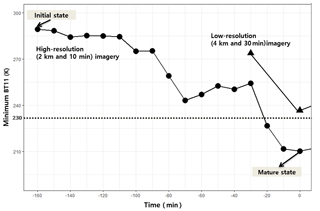
Figure 3An example of temporal changes in the minimum BT11 among thunderstorm pixels (10 August 2017 at 03:10–05:50 UTC) for the high-resolution (2 km and 10 min) imagery (circle) and the low-resolution (4 km and 30 min) imagery (triangle). In this study, the lead time was defined as the time between the initial state and the mature state (time 0). Negative time indicates the time ahead of the mature state of a tropical thunderstorm.
The most interesting point of Fig. 3 is the pattern of temporal changes in the minimum BT11 among the thunderstorm pixels for high-resolution imagery. One can expect that the BT11 of a thunderstorm might gradually decrease, but the BT11 of the targeted thunderstorm initially decreased from the initial state to −70 min and increased slightly from −70 to −30 min. We speculated that the decline of the BT11 was related to the vertical growth of the cloud. This is commonly observed in the life cycle of tropical thunderstorms. It is notable that the BT11 for the low-resolution imagery was too simple to monitor this status of the clouds in detail (the triangle in Fig. 3).
Table 3 lists the lead time of each imagery type. In 60 cloud samples, nos. 1–20 occurred in the daytime of July 2017, nos. 21–30 in the nighttime of July 2017, nos. 31–50 in the daytime of August 2017, and nos. 51–60 in the nighttime of August 2017. In the case of the high-resolution imagery, the lead time ranged from 90 to 180 min. In contrast, the low-resolution imagery began to detect cloud pixels only up to 0–30 min in advance. Here, a lead time of zero indicates that the low-resolution imagery failed to detect the cloud pixel, while the cloud observed with the high-resolution imagery reached the mature state. In this study, the high-resolution imagery had a high predictability of thunderstorms, which was up to 180 min. The low-resolution imagery, on the other hand, had a low predictability of thunderstorms (up to 60 min).
Table 3The lead time of the clouds according to imagery occurred in the daytime of July 2017 for nos. 1–20, in the nighttime of July 2017 for nos. 21–30, in the daytime of August 2017 for nos. 31–50, and in the nighttime of August 2017 for nos. 51–60.
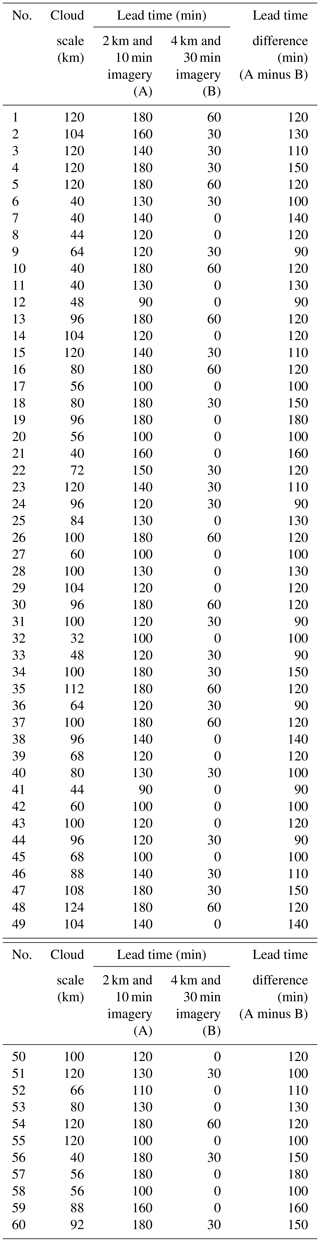
Figure 4 depicts the spatial distribution of the BT11 used to determine the cause of the difference in the lead time between the different resolutions. Figure 4a and b are the result of the low-resolution imagery and that of the high-resolution imagery (10 August 2017 at 03:00–05:50 UTC). The amount of change of the BT11 within the observation time interval is closely related to determining the initial state. In Fig. 4a, pixels with a variation of 15 K or more are recognized as a thunderstorm every 30 min. In contrast, in Fig. 4b, pixels with a variation of 5 K or more are recognized as a thunderstorm every 10 min. This can cause difficulty in detecting the initial state using low-resolution imagery because of the BT11 variation, which is proportional to the observation time interval. Also, Fig. 4a shows that the boundaries of clouds are not clear from 03:00 to 04:00 UTC, and there seems to be a limitation in continuously tracking developing storms from 04:00 to 05:00 UTC because the low-resolution imagery could not detect cloudy pixels whose scale was between 2 and 4 km. Figure 4b shows the BT11 at the boundary, which was nearly 270 K (orange), and the area of the cloudy pixels, which was nearly 250 K (green). Interestingly, in Fig. 4b, it can be seen that small clouds were formed from 04:00 to 04:50 UTC, but one dominant cloud, which was below 230 K (blue), dramatically increased its size from 04:50 to 05:50 UTC. This phenomenon indicates that the properties of a rapidly developing thunderstorm can be captured by the high-resolution imagery. Therefore the high-resolution imagery can accurately monitor the change of the BT11 and be advantageous in the detection of early clouds.
In this paper, we compared high-resolution and low-resolution imagery at an infrared channel of 10.45 µm. It was difficult to track the rapid BT11 changes in the clouds during the process of their development. The lead time of 60 cloud samples determined by the low-resolution imagery was not sufficiently accurately measured to monitor the whole development process of tropical thunderstorms. In contrast, the lead time of the high-resolution imagery was from 90 to 180 min before the cloud reached its mature state. Therefore, the higher spatial and temporal resolution of satellite observations can be valuable as it would allow for an alarm to be raised for tropical thunderstorms over Southeast Asia approximately 2 h earlier than the low-resolution one based on validation using 60 thunderstorm events.
Some limitations of our study have to be acknowledged. First, this method may not work if a thunderstorm happens in the mesoscale convective system. In the mesoscale convective system, an intrusion of other developing clouds could frequently occur. Second, future studies are needed to determine whether thunderstorms are rainy after the lead time. To more accurately examine the lead time, validation with surface precipitation data based on ground observation is further required. Also, the near-real-time radar data can be useful to validate the precipitation if the surface precipitation data have not been well managed. Finally, the lead time can differ depending on the region, since the lead time can be affected by various environmental factors such as wind direction and speed, atmospheric profiles, and the characteristics of geolocation.
The impact of increased spectral observation was not discussed in this study. The additional channels in Himawari-8 are another advantage that improves the accuracy of the forecasts of deep convective clouds, as compared to former satellites. For example, three water vapor channels with different weighting functions can provide more detailed information about the vertical growth of cloud objects. The CO2 absorption channel also gives a chance to monitor the temporal changes in cloud thickness and height. Another infrared channel (8.6 µm) facilitates the examination of the changes in the glaciation of clouds. Therefore, the use of new additional channels in Himawari-8 is critically important to the successful reduction of the false alarm rate in thunderstorm prediction. Implemented operationally under real-life conditions, the high-resolution (2 km and 10 min) imager is of great significance to the provision of practical assistance to effective disaster management. The alarm for evacuation can be turned on 2 h in advance of the event by using the high-resolution imagery. Although many developing countries in Southeast Asia use Himawari-8 satellite data, few countries carefully consider the importance of satellite resolution. A longer lead time is beneficial to the reduction of the risk of natural disasters caused by thunderstorms and may lead to a more timely evacuation in such adverse events. Moreover, the high-resolution imagery is more effective in data storage than the low-resolution imagery.
Thunderstorm prediction using satellites is of vital importance in Southeast Asian developing countries to reduce the risks from heavy rain, lightning, and flooding. The currently used satellites can observe the area more precisely, and their application to pre-disaster management is highly appreciated and demanded. This study examined the advances in the predictability of thunderstorms using geostationary satellite imageries. Our present results show that by using the latest geostationary satellite data (with a resolution of 2 km and 10 min), thunderstorms can be predicted 90–180 min ahead of their mature state. These data can capture the rapidly growing cloud tops before the cloud moisture falls as precipitation. However, thunderstorms cannot be detected, or they can only be detected 60 min ahead of time with low-precision satellite data (with a resolution of 4 km and 30 min). Therefore, the latest geostationary satellite data obtained at a resolution such as 2 km and 10 min can be critically important to the early detection of thunderstorms for enabling prompt preparation and the mitigation of hazards.
The data that support the findings of this study are available from Yong-Sang Choi upon request. The South Korean National Meteorological Satellite Center (http://nmsc.kma.go.kr/; Choi and Ho, 2015) provides the satellite data upon request for research purposes.
KL designed the study, performed the experiments, analyzed the results, and wrote the paper. HSK designed the study, revised the manuscript, and performed supplementary experiments. YSC designed the study and was involved in the discussion of the research. All authors critically read and approved the paper.
The authors declare that they have no conflict of interest.
This study is supported by the Korea Meteorological Administration Research and Development Program (grant no. KMI2018 04110).
This research has been supported by the Korea Meteorological Administration Research and Development Program with a grant from the Korea Meteorological Institute (KMI; grant no. KMI2018 04110) and the Basic Science Research Program of the National Research Foundation of Korea (NRF) funded by the Ministry of Education (grant no. 2018R1A6A1A08025520).
This paper was edited by Paolo Tarolli and reviewed by two anonymous referees.
Avotniece, Z., Aniskevich, S., Briede, A., and Klavins, M.: Long term changes in the frequency and intensity of thunderstorms in Latvia, Boreal Environ. Res., 22, 415–430, https://doi.org/10.2166/nh.2008.033, 2017.
Bessho, K., Date, K., Hayashi, M., Ikeda, A., Imai, T., Inoue, H., and Okuyama, A.: An introduction to Himawari-8/9 – Japan's new generation geostationary meteorological satellites, J. Meteorol. Soc. Jpn. Ser. II, 94, 151–183, https://doi.org/10.2151/jmsj.2016-009, 2016.
Choi, Y. S. and Ho, C. H.: Earth and environmental remote sensing community in South Korea: A review, Remote Sens. Appl.: Soc. Environ., 2, 66–76, https://doi.org/10.1016/j.rsase.2015.11.003, 2015.
de Coning, E., Gijben, M., Maseko, B., and van Hemert, L.: Using satellite data to identify and track intense thunderstorms in South and southern Africa, S. Afr. J. Sci., 111, 1–5, https://doi.org/10.17159/sajs.2015/20140402, 2015.
Escrig, H., Batlles, F. J., Alonso, J., Baena, F. M., Bosch, J. L., Salbidegoitia, I. B., and Burgaleta, J. I.: Cloud detection, classification and motion estimation using geostationary satellite imagery for cloud cover forecast, Energy, 55, 853–859, https://doi.org/10.1016/j.energy.2013.01.054, 2013.
Himawari-8/9 Imagery (AHI): Imaging bands, available at: https://www.data.jma.go.jp/mscweb/en/himawari89/space_segment/spsg_ahi.html, last access: 26 October 2018.
Houze Jr., R. A.: Mesoscale convective systems, Rev. Geophys., 42, RG4003, https://doi.org/10.1029/2004rg000150, 2004.
Houze Jr., R. A. and Betts, A. K.: Convection in GATE, Rev. Geophys., 19, 541–576, https://doi.org/10.1029/rg019i004p00541, 1981.
Huuskonen, A., Saltikoff, E., and Holleman, I.: The operational weather radar network in Europe, B. Am. Meteorol. Soc., 95, 897–907, https://doi.org/10.1175/bams-d-12-00216.1, 2014.
Kahn, B. H., Takahashi, H., Stephens, G. L., Yue, Q., Delanoë, J., Manipon, G., Manning, E. M., and Heymsfield, A. J.: Ice cloud microphysical trends observed by the Atmospheric Infrared Sounder, Atmos. Chem. Phys., 18, 10715–10739, https://doi.org/10.5194/acp-18-10715-2018, 2018.
Klazura, G. E. and Imy, D. A.: A description of the initial set of analysis products available from the NEXRAD WSR-88D system, B. Am. Meteorol. Soc., 74, 1293–1312, https://doi.org/10.1175/1520-0477(1993)074<1293:adotis>2.0.co;2, 1993.
Menzel, W. P. and Purdom, J. F.: Introducing GOES I: The first of a new generation of geostationary operational environmental satellites, B. Am. Meteorol. Soc., 75, 757–782, https://doi.org/10.1175/15200477(1994)075<0757:igitfo>2.0.co;2, 1994.
MRC – Mekong River Commission: About MRC, available at: http://www.mrcmekong.org/about-mrc/, last access: 30 October 2018.
Nastos, P., Dalezios, N. R., and Ulbrich, U. (Eds.): Advances in meteorological hazards and extreme events, Nat. Hazards Earth Syst. Sci., https://www.nat-hazards-earth-syst-sci.net/special_issue190.html, 2016.
Pachauri, R. K. and Meyer, L.: Climate change 2014 Synthesis Report Summary for Policymakers, IPCC, https://doi.org/10.1017/cbo9781107415416.005, 2014.
Schefczyk, L., Gutjahr, O., and Heinemann, G.: Climate change impact on thunderstorms: Using high-resolution COSMO CLM simulations to determine changes in thunderstorm occurrences, 10. Deutsche Klimatagung, 21–24 September 2015, Hamburg, https://doi.org/10.1127/metz/2017/0749, 2015.
Schmit, T. J., Gunshor, M. M., Menzel, W. P., Gurka, J. J., Li, J., and Bachmeier, A. S.: Introducing the next generation Advanced Baseline Imagery on GOES R, B. Am. Meteorol. Soc., 86, 1079–1096, https://doi.org/10.1175/bams-86-8-1079, 2005.
Shaw, R., Pulhin, J., and Pereira, J.: Climate change adaptation and disaster risk reduction: overview of issues and challenges, Emerald Group Publishing, https://doi.org/10.1108/pm.2011.29.5.491.2, 2010.
Vila, D. A., Machado, L. A. T., Laurent, H., and Velasco, I.: Forecast and Tracking the Evolution of Cloud Clusters (ForTraCC) using satellite infrared imagery: Methodology and validation, Weather Forecast., 23, 233–245, https://doi.org/10.1175/2007waf2006121.1, 2008.
Walker, J. R., MacKenzie Jr., W. M., Mecikalski, J. R., and Jewett, C. P.: An enhanced geostationary satellite–based convective initiation algorithm for 0–2-h nowcasting with object tracking, J. Appl. Meteorol. Clim., 51, 1931–1949, https://doi.org/10.1175/jamc-d-11-0246.1, 2012.
Wang, P. K.: Physics and dynamics of clouds and precipitation, Cambridge University Press, Cambridge, https://doi.org/10.1017/cbo9780511794285, 2013.
Yang, G. Y. and Slingo, J.: The diurnal cycle in the tropics, Mon. Weather Rev., 129, 784–801, https://doi.org/10.1175/15200493(2001)129<0784:tdcitt>2.0.co;2, 2001.
Yang, J., Zhang, Z., Wei, C., Lu, F., and Guo, Q.: Introducing the new generation of Chinese geostationary weather satellites, Fengyun 4, B. Am. Meteorol. Soc., 98, 1637–1658, https://doi.org/10.1175/bams-d-16-0065.1, 2017.