the Creative Commons Attribution 4.0 License.
the Creative Commons Attribution 4.0 License.
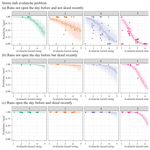
Exploring the relationship between avalanche hazard and run list terrain choices at a helicopter skiing operation
Reto Sterchi
Patrick Mair
While guides in mechanized skiing operations use a well-established terrain selection process to limit their exposure to avalanche hazard and keep the residual risk at an acceptable level, the relationship between the open/closed status of runs and environmental factors is complex and has so far only received limited attention from research. Using a large dataset of over 25 000 operational run list codes from a mechanized skiing operation, we applied a general linear mixed-effect model to explore the relationship between skiing terrain that is deemed appropriate for guiding (i.e., status open) and avalanche hazard conditions. Our results show that the magnitude of the effect of avalanche hazard on run list codes depends on the type of terrain that is being assessed by the guiding team. Ski runs in severe alpine terrain with steep lines through large avalanche slopes are much more susceptible to increases in avalanche hazard than less severe terrain. However, our results also highlight the strong effects of recent skiing on the run coding and thus the importance of prior first-hand experience. Expressing these relationships numerically provides an important step towards the development of meaningful decision aids, which can assist commercial operations to manage their avalanche risk more effectively and efficiently.
- Article
(6945 KB) - Full-text XML
- BibTeX
- EndNote
The majestic mountains and abundant powder snow make western Canada a world-renowned destination for winter backcountry recreation. One of the key players in this activity is the mechanized skiing industry, where professionally trained guides take paying clients to remote untracked powder slopes using helicopter, and snowcats. The industry has been growing since its inception in the 1960s and offers more than 100 000 skier days per winter today (HeliCat Canada, 2016). However, winter backcountry travel is not without risks. Snow avalanches are the most significant hazard affecting daily operations in mechanized skiing in Canada (Bruns, 1996). Walcher et al. (2019) report that between 1997 and 2016, avalanches accounted for 77 % of the overall natural hazard mortality in mechanized skiing in Canada. Operations manage the risk from avalanches by continuously assessing the local hazard conditions and carefully choosing appropriate terrain and travel procedures to limit their exposure and keep the residual risk at an acceptable level while still providing a high-quality skiing experience. In addition, some operations use explosives to directly control avalanche hazard or purposely ski individual runs to control future avalanche hazard by modifying the local snowpack (commonly referred to as “run maintenance”).
In Canada, mechanized skiing operations select terrain for skiing by following a well-established, iterative process. This risk management process has been described as a series of filters occurring at multiple spatial and temporal scales (Israelson, 2015) that progressively eliminate skiing terrain from consideration (Fig. 1). The daily process starts with a morning meeting where the guiding team assesses the current hazard conditions and produces an initial large-scale avalanche hazard forecast across the entire tenure based on the previous day's experiences and the observed overnight changes. This initial hazard assessment is the foundation for the day's “run list”, which represents the first terrain elimination filter. In this step, the guiding team discusses their inventory of predefined ski runs and collectively decides for each run whether it is open or closed for guiding guests under the expected avalanche hazard conditions. It is important to note that depending on the nature of the operation, the scale of ski runs can range from tightly defined ski lines to areas the size of a medium ski resort. However, regardless of their size, the nature of the ski runs is consistent enough that they represent meaningful decision units at this stage of the risk management process. The large-scale, consensus-based run list that emerges from the morning meeting sets the stage for the skiing program of the day. Over the course of a skiing day, the avalanche hazard assessment is refined based on direct field observations and runs that are skied are chosen from the run list accordingly. In most helicopter skiing operations, helicopters serve multiple groups of skiers, each of them led by a guide. It is common practice that the guide of the first group serviced by the helicopter (known as the “lead guide”) decides what runs the groups of this helicopter ski. This run choice represents the second filter in the terrain selection process. The third and final filter of the terrain selection process is the decision of how exactly a particular run is skied, which is the responsibility of the guide of each group. This sequence of (1) run list established by the entire guiding team, (2) run choice made by the lead guide, and (3) ski line choice within run made by individual guides highlights the hierarchical and iterative nature of the terrain selection process. At each filter level, the decisions are refined based on avalanche hazard assessments at increasingly smaller scales. While avalanche hazard is a critical factor in this process, other factors such as weather and flying conditions, flight economics, skiing quality, guest preferences, and skiing abilities also affect the selection and sequencing of the skied runs (Israelson, 2015). This terrain selection process is repeated every day, and guiding teams continuously adjust their terrain choices in response to the observed changes in conditions.
While the steps of the terrain selection process are well defined and easy to describe, the relationship between environmental factors and terrain selection is complex and has so far only received limited attention from research. Grímsdottír (2004) and Haegeli (2010) identified critical terrain and avalanche hazard factors contributing to the terrain decisions at the run scale but did not examine the relationship between avalanche hazard conditions and run list codes in more detail. While Hendrikx et al. (2016) and Thumlert and Haegeli (2018) studied the association between small-scale terrain choices and avalanche conditions quantitatively by analyzing patterns in GPS tracks, they did not account for the fact that these choices are embedded in the higher-level, hierarchical, and continuous terrain selection process described above. Having an in-depth, quantitative understanding of each stage of the terrain selection process is critical for properly tapping into the risk management practices of guiding teams and describing it in a way that offers useful insight into the influencing factors. Only a comprehensive perspective will allow us to capture the existing tacit expertise, isolate the effect of avalanche hazard, and extract information on relevant patterns in a way that facilitates learning from the past and developing decision support tools that can aid the terrain selection process in meaningful ways. The objective of our study is to advance our understanding of the professional avalanche risk management process by quantitatively examining the relationship between appropriate skiing terrain (i.e., open or closed for guiding) and avalanche hazard conditions at the run list scale using recorded avalanche hazard assessments and run list ratings from a commercial helicopter skiing operation.
2.1 Study site
For this study, we collaborated with Northern Escape Heli Skiing (NEH), a commercial helicopter skiing company based out of Terrace, BC, Canada (Fig. 2). NEH's operating tenure is in the Skeena Mountains and spans an area of nearly 6000 km2. The skiing terrain ranges from 500 to 2000 m a.s.l. covering all three elevation bands (alpine, treeline, and below treeline). While their entire tenure has 260 established ski runs, much of their skiing is focused on approximately 60 runs in their home drainage, which is the focus of our study. The character of the local snow climate is maritime with storm slab avalanche problems during or immediately following storms being the primary avalanche hazard concerns (McClung and Schaerer, 2006; Shandro and Haegeli, 2018).
2.2 Dataset
The primary dataset used in this study consists of daily run list and avalanche hazard information for the six winter seasons 2012/13 to 2017/18 (517 operational days between December 1 and 31 March of each season). The run list dataset consists of 26 488 individual run ratings in total, one for every run on each of the 517 operational days. At NEH, the guiding team codes runs as either “open for guiding” (i.e., everybody in the guiding team agrees that there is a least one line that can be skied with guests under the current conditions), “closed for guiding due to avalanche hazard” (i.e., members of the guiding team are not comfortable with taking guests onto that run), “closed for guiding for reasons other than avalanche hazard” (e.g., other mountain hazards such as crevasses, open creeks, ski quality), or “not discussed” (i.e., ski runs in zones not considered are automatically closed for skiing that day).
NEH's avalanche hazard assessment process follows the conceptual model of avalanche hazard (CMAH; Statham et al., 2018), which structures the process around the identification and characterization of avalanche problems. Avalanche problems represent operational concerns about potential avalanches that can be described in terms of the type of avalanche problem, the location in the terrain where the problem can be found, the likelihood of associated avalanches, and their destructive size. The concept of avalanche problem type represents the idea that distinct types of avalanches that emerge from specific snowpack structures and weather events require different risk mitigation approaches. Statham et al. (2018), and describe nine distinct types of avalanche problems (dry loose avalanche problem, wet loose avalanche problem, storm slab avalanche problem, wind slab avalanche problem, persistent slab avalanche problem, deep persistent slab avalanche problem, wet slab avalanche problem, glide avalanche problem, and cornice avalanche problem) that differ in their development, avalanche activity patterns, how they are best recognized and assessed in the field, and what risk management strategies are most effective for managing them. While some avalanche problems are of relatively short duration and can be managed easily by avoiding specific terrain features within runs (e.g., wind-loaded slopes when a wind slab avalanche problem is present), others, such as a persistent slab avalanche problems, can linger for weeks, even months, and require a more conservative risk management approach that excludes a broader range of terrain (Haegeli et al., 2010; Statham et al., 2018).
After the guides at NEH have identified the types of avalanche problems they are concerned about, they describe the terrain in which they expect to encounter these problems in terms of elevation bands (alpine, treeline, and below treeline) and aspect ranges. The likelihood of avalanches combines the sensitivity to triggers and the spatial distribution and is expressed on an ordinal scale using the qualitative terms “unlikely”, “possible”, “likely”, “very likely”, and “almost certain” (Statham et al., 2018). Destructive size is assessed according to the Canadian avalanche size classification (Canadian Avalanche Association, 2014) on a scale ranging from 1.0 (relatively harmless for people) to 5.0 (largest snow avalanche known, could destroy a village or a large forest area of approximately 40 ha). Guides express their uncertainty in hazard assessments by specifying ranges of likelihood and size for each avalanche problem (minimum, typical, and maximum for both parameters). The hazard assessments for each elevation band are concluded by summarizing the overall hazard level that emerges from the combined avalanche problems with a single hazard rating on an ordinal scale from 1 (least hazardous) to 5 (most hazardous) (Canadian Avalanche Association, 2015).
To identify meaningful patterns between avalanche hazard and terrain choices numerically, it is critical to encode the nature of the available ski runs in a way that is insightful, but not too complex for the analysis. To comprehensively capture the complex nature of entire ski runs in our model in a way that reflects how professional guides perceive them, we used the approach introduced by Sterchi and Haegeli (2019), which groups the ski runs into operation-specific terrain classes based on multi-seasonal patterns in run list ratings (i.e., revealed terrain preferences). Sterchi and Haegeli (2019) first identified groups of ski runs by clustering similarly coded ski runs over the course of several winter seasons. Subsequently, they arranged the identified groups into a hierarchy that ranges from runs that are almost always open to runs that are only open when conditions are favourable. To better understand the nature of the revealed ski run classes, the authors had a senior lead guide at each participating operation provide a comprehensive but structured description of their ski runs with respect to access, type of terrain, skiing experience, operational role, hazard potential, and guidability. Since this ski run classification is based on past operational risk management decisions, it reflects the local terrain expertise and avalanche risk management practices in the context of the available terrain and local snow and avalanche climate conditions (Sterchi and Haegeli, 2019). Thus, this approach represents a more meaningful characterization of ski run classes to analyze professional terrain choices in mechanized skiing operations than existing terrain classification systems which have small numbers of universal terrain classes (e.g., ATES; Statham et al., 2006; Campbell and Gould, 2013) or focus primarily on standard terrain characteristics such as slope incline, slope shape, elevation, aspect, and vegetation density (e.g., Hendrikx et al., 2016; Thumlert and Haegeli, 2018).
At NEH, the analysis of Sterchi and Haegeli (2019) identified six distinct classes of ski runs. While the severity of terrain generally increases from Class 1 to Class 6, as illustrated by the average seasonal percentage of the run code “open” for each ski run (Fig. 3) and the terrain photos of example runs (Table 1), the groupings also reflect other run characteristics like accessibility, quality of skiing experience, and operational practices.
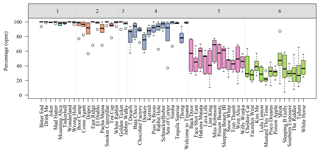
Figure 3Boxplot of average seasonal percentages of run code “open” for the 57 ski runs during the six seasons 2012/13 to 2017/18 with the six identified classes of similarly managed ski runs (Sterchi and Haegeli, 2019). Due to the small group size and their outlier characteristics, the two runs of Class 3 were not included in the present analysis.
The first three classes generally consist of easily accessible and mostly gentle ski runs with no or only limited exposure to avalanche slopes. Most of the skiing is through open slopes at treeline, open canopy snow forest below treeline, and non-glaciated or glaciated alpine areas. The main difference between the first two classes is that the runs of Class 1 provide a better skiing experience. Since Class 1 runs are more attractive, they are typically skied more often, guides have a better handle on the local conditions, and hence the runs are coded open more consistently. The two runs included in Class 3 are of similar general character, but they are located at lower elevations, which makes them more vulnerable to rising freezing levels. Due to the small group size and their outlier characteristics, we excluded them from the present analysis. While most of the ski runs of the first three groups are at and below treeline, Classes 4 to 6 predominantly consist of alpine terrain. Class 4 consists of ski runs in gentle alpine terrain or open slopes at treeline where most ski lines do not cross any avalanche slopes. These ski runs are often accessible and provide generally a good skiing experience with easy or moderately challenging skiing. However, some of the ski runs can be exposed to overhead avalanche hazards during regular avalanche cycles. The ski runs included in Class 5 are also located in alpine terrain but are substantially steeper and cross avalanche slopes more frequently than the runs of Class 4. Furthermore, almost half of the ski runs in Class 5 can be directly affected by overhead hazard during regular avalanche cycles and many pickup locations are threatened by overhead avalanche hazard during large avalanche cycles. While skiing on these runs was characterized as moderately challenging, they offer very good or even “life-changing” skiing experiences for guests. Class 6, the highest group in the NEH ski run hierarchy, mainly consists of runs in the most serious alpine terrain skied at NEH. The runs are rarely skied but can play an important operational role when conditions are appropriate. Most of these runs have moderately steep or steeper slopes that can produce avalanches of size 3.0 or bigger and many pickup locations are exposed to overhead avalanche hazard during regular avalanche cycles. However, they provide good or very good skiing experiences for the guests.
2.3 Statistical analysis
Since our dataset consists of repeated run list codes for the same runs over the course of several winters, traditional regression models that require observations to be independent from each other are inappropriate for our analysis (Long, 2012). Mixed-effect models are an extension of traditional regression models that allow for heterogeneity, nested data, or temporal or spatial correlation in longitudinal and/or clustered datasets by relaxing some of the necessary assumptions (Bolker et al., 2009; Zuur et al., 2009; Harrison et al., 2018). To overcome the issue of repeated measures and nested data, mixed-effect models include both fixed and random effects in the regression equation. The fixed effects, which are equivalent to the intercept and slope estimates in traditional regression models, capture the relationship between the predictor and response variables for the entire dataset. While traditional regression models assign the remaining unexplained variance in the data (i.e., randomness) entirely to the global error term, mixed-effect models partition the unexplained variance that originates from groupings within the dataset into random effects. Thus, random effects can highlight how groups within the dataset deviate from the overall pattern described by the fixed effects. Similar to the parameter estimates for fixed effects, random effects can include both intercept and slope parameters. While random intercepts explain how the average conditions within groups deviate from the average conditions across the entire dataset, random slopes capture group-specific differences in the relationship between the predictor and response variables. The overall response of a particular group to the predictor variables can therefore be described as the linear combination of the overall fixed effects and the group-specific random effects.
Since our target variable, the acceptability of a run, is binary (i.e., open or closed), a logistic regression model is most suited for our analysis. In their basic form, logistic regression models use the logistic function to model the relationship between a binary dependent variable and one or more predictors xi. In such a model, the probability of Runk being open can be expressed with
In this equation, β0 is the intercept, and βi represents the regression parameter estimates associated with the functional forms fi (e.g., transformations such as coding a categorical variable into dichotomous variables) of the predictors xi included in the model. The linear combination of the functional form of the predictors xik multiplied with the parameter estimates βi in the exponent in the denominator represents the log odds (the logarithm of the odds) of Runk being open. The components of the equation can be interpreted as follows: the intercept β0 represents the log odds when all predictors are zero. A parameter estimate of βi=1 or βi=2 means that a one unit increase in fi(xik) increases the log odds of Runk being open by 1 or 2, respectively. This is referred to as the “effect” of the predictor xik. The most common way to express the effect of predictors in logistic regression models is odds ratios (ORs), which can be derived by applying an exponential function to the regression coefficients. Hence, parameter estimates significantly larger than zero result in OR > 1, which means that the odds of Runk being open increase relative to the base level, whereas parameter estimates significantly smaller than zero produce OR < 1 that highlight that the odds of Runk being open decrease.
To examine the acceptability of runs (i.e., being open or closed) under different hazard conditions, we regressed their daily run list codes against the hazard situation with the runs' terrain characteristics, their past use, and their run list codes of the previous day as covariates (Fig. 4). To focus our analysis on the effect of avalanche hazard on open and closed status of runs, we simplified the categorical run list ratings before fitting the regression model. Run list codes indicating that a run was open (i.e., open for guiding) were coded as 1, whereas run list codes indicating that a run was closed because of avalanche concerns (i.e., closed for guiding due to avalanche hazard) were coded as 0. Run list codes indicating that a run was not considered for any other reasons (i.e., closed for guiding for reasons other than avalanche hazard, not discussed) were excluded from the analysis.
Avalanche hazard conditions were represented in the model with the relevant hazard rating of the day and the types of avalanche problems present. Since ski runs can cross several elevation bands (e.g., a ski run can start in alpine terrain, include skiing at treeline and have its pickup location below treeline), multiple avalanche hazard ratings might apply. To circumvent this issue in our analysis, we derived a relevant hazard rating of the day for each run by taking the highest hazard rating of the elevation bands the run crosses. Types of avalanche problems present were implemented in the model with binary covariates (1: present; 0: absent), one for each of the eight1 avalanche problems used by NEH. Because the avalanche problems are also assessed for each elevation band separately, we derived relevant daily avalanche problem values for each run similarly to the relevant hazard rating described above. Since avalanches of size 1.0 are considered relatively harmless to people (McClung and Schaerer, 2006; Canadian Avalanche Association, 2014), we only included avalanche problems in our analysis that were characterized with a maximum destructive size of at least size 1.5. Because of the small number of cases, we also excluded avalanche problems where both typical and maximum likelihood was assessed as “unlikely”. To allow our model to account for the possibility that the effect of avalanche hazard on the acceptability of a run being open might differ among terrain types, we included two-way interactions between the relevant hazard rating and ski run class as well as all eight binary variables for types of avalanche problems present and ski run class.
To account for the iterative character of the terrain assessment process in mechanized skiing, we included two variables in our model that represent critical temporal influences on run list codes. Skied in the previous week represents past use, which offers both first-hand skiing experience and direct weather, snowpack, and avalanche observations for a run. Run code of the previous day was included to account for the direct influence of previous run lists on subsequent days. To acknowledge possible correlations between skied in the previous week and run code of the previous day (i.e., a run needs to be open to be skied), we also added the interaction between these two variables to our model.
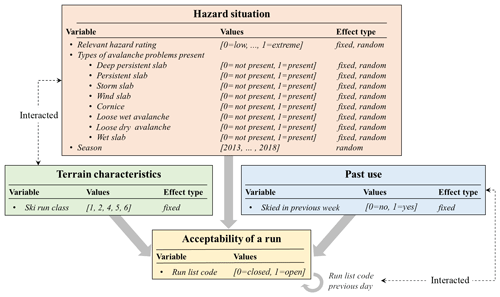
Figure 4Illustration of the model. Our model included variables describing the hazard situation, the terrain characteristics of a ski run, and its past use to examine their relationships with the acceptability of a run (e.g., it being coded “open”). To account for the iterative character of the terrain assessment process, the model also included the run list code from the previous day. In addition to the fixed effects, we included by-run and by-season random effects.
Since our dataset consists of repeated ratings of the same runs (i.e., panel structure), we included random by-run intercepts and slopes for hazard and avalanche problems. This allows the model to capture the run-specific effect of hazard and avalanche problems that goes beyond the ski-run-class-specific effect. We also included a random by-season intercept to account for the unique character of each winter in the model.
We performed the model estimation in a Bayesian framework using the statistical software R (R Core Team, 2019) and the package rstanarm (Stan Development Team, 2016). We estimated the model with 2500 warmup and 2500 sampling iterations for four separate sampling chains with default priors. Model convergence was inspected based on the potential scale reduction factor (Gelman and Rubin, 1992), which compares the estimated between- and within-chain variances between multiple Markov chains for each model parameter. Large differences between these variances indicate that a model did not converge while values close to 1.0 indicate good convergence. The Markov chains exhibit some degree of autocorrelation, where a lower autocorrelation indicates more independent sampling of the posterior. The approximate number of independent draws with the same accuracy as the sample of correlated draws is referred to as the effective sample size (ESS). We consider an ESS of greater than 1000 as an indication of independent sampling of the posterior.
To eliminate the potentially undesirable impact a variable might have purely due to its scale, all variables included in the analysis were scaled to the interval 0 to 1. Hence, relevant hazard rating was included in the model as a numeric variable scaled to range between 0 and 1. Ski run class was included as a dummy-coded categorical variable with Class 1 as the reference class, whereas all other predictors were represented as binary variables. We explored different model configurations including models where the avalanche problems of concern were included as categorical variables including combinations of different avalanche problems. Only parameter estimates with 95 % credible intervals different from 0 were considered significant.
The sampling chains of our model converged successfully as indicated by both the potential scale reduction factor (values of 1.0) and for effective sample size (values > 1000) for all parameter estimates. Since the variable ski run class was dummy coded in our model, the main effects for the variables that were combined with ski run class represent the effect for Class 1, the reference class. The effects for the other classes need to be derived by adding the main effect with the ski-run-class-specific interaction effect.
3.1 Effect of hazard rating and terrain type
The strongly positive main effect intercept indicates that there is a strong base tendency for the runs of Class 1 to be open at hazard Level 1 (parameter estimate = 5.48, Table 2). The intercept ski run class interaction effects for all the other classes are significantly negative (parameter estimates , −2.40, −3.03, and −4.75, Table 3), which means that overall, they are less likely to be open. As expected, the probability of a run being open decreases substantially with increasing hazard for all ski run classes as illustrated by the negative main effect for hazard rating (parameter estimate , Table 2).
Table 2Main effects. Diagnostics and posterior summary statistics of the estimated parameters from the mixed-effect logistic regression model. ESS is the effective sample size for each parameter. Significant parameter estimates are indicated in bold. Non-significant (ns) ORs are omitted.
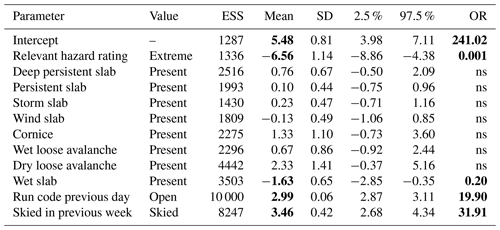
However, the fact that the interaction effects of the different ski run classes (Table 3) differ significantly from each other highlights that the magnitude of this effect strongly depends on the type of ski run being assessed by the guiding team. These patterns are also visible in Fig. 5, which shows the probabilities of runs of different ski run classes being open during a storm slab avalanche problem under different hazard ratings and operational scenarios. We present the following three operational scenarios: (a) ski runs were neither open previously nor skied recently, (b) ski runs were not open the day before but recently skied, and (c) runs were open the day before and recently skied. For each of these scenarios, we plotted the probabilities of ski runs in each ski run class to be open as a function of the hazard rating and included the 50 % and 95 % probability intervals based on 50 draws from the posterior distribution of the individual runs from each ski run class.
Along with the probability curves, average daily percentages of open runs per ski run class are plotted where observations for this scenario existed in the dataset. The charts show that the probability of a run being open decreases more substantially with increasing hazard for runs in Classes 5 and 6, whereas the modelled probability curves are less steep for Classes 1, 2, and 3 (Fig. 5a).
Table 3Interaction effects. Diagnostics and posterior summary statistics of the estimated parameters from the mixed-effect logistic regression model. ESS is the effective sample size for each parameter. Significant parameter estimates and odds ratios (OR) indicated in bold. Non-significant (ns) ORs are omitted.
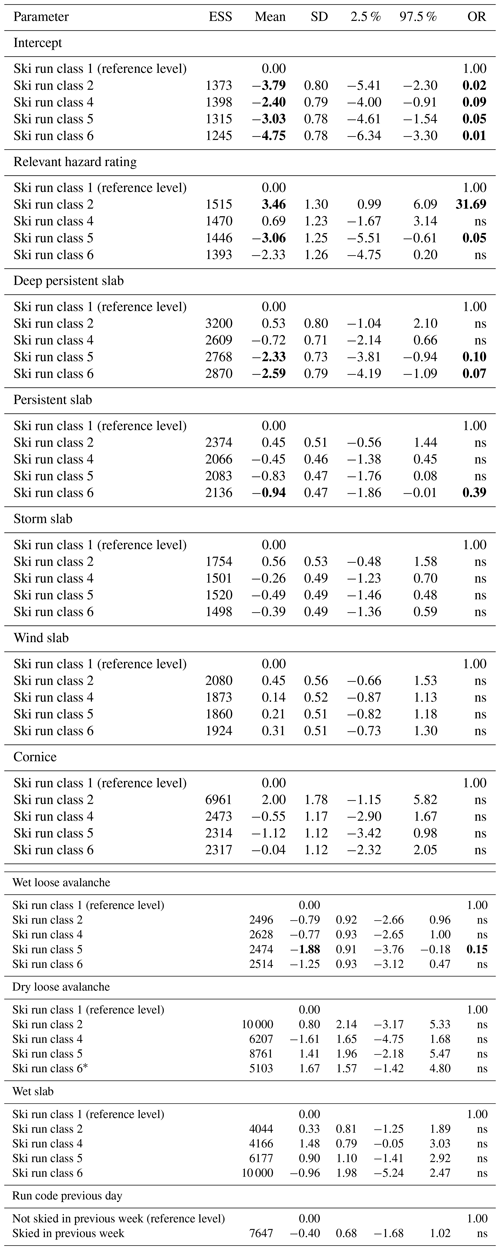
Since our model included both ski-run-class-specific intercepts and ski-run-class-specific slopes for hazard ratings, interpreting the effect of avalanche hazard on run list ratings directly from the parameter estimates is challenging. To present the combined effect of intercept and slope, we calculated OR for each ski run class and hazard rating based on the regression coefficients. Table 4 shows the odds ratios of ski run classes being open with increasing avalanche hazard relative to themselves at hazard Level 1.
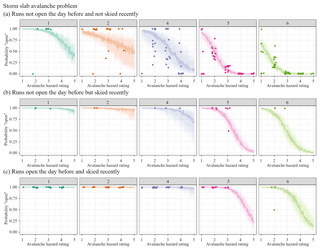
Figure 5Probabilities of ski runs being open for Storm slab avalanche problems shown for increasing hazard levels with (a) a scenario where ski runs were neither open previously nor skied recently, (b) a scenario where runs were not open the day before but recently skied, and (c) a scenario where runs were open the day before and recently skied. The visualizations include probability intervals of 50 % and 95 % for each ski run class as a whole based on 50 draws from the posterior distribution. Average daily percentages of open runs per ski run class are plotted as points where observations for this scenario exist in the dataset.
Table 4Odds ratios of each ski run class being open with increasing avalanche hazard relative to low avalanche hazard.
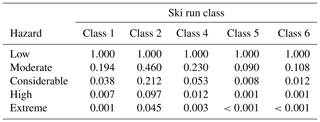
While the odds of runs being open decrease with increasing avalanche hazard ratings in all ski run classes, the magnitude of the decrease varies substantially. The odds of the ski runs in Class 1 being open decrease by a factor of 1000 as avalanche hazard goes from low to extreme. In comparison, the ski runs in Class 2 are only about 20 times less likely to be open with the same increase in avalanche hazard. This means that despite the lower overall tendency of runs included in this class to be open, the run list ratings of the Class 2 runs are less affected by danger ratings. Since many of these ski runs are located at or below treeline, we suspect that the observed pattern reflects that many of these runs offer safe skiing options through trees, even when avalanche hazard is elevated. The alpine terrain classes are much more strongly affected by changes in danger ratings as evident by the large negative slope estimates. The odds of the ski runs in Class 4 being open decrease by a factor of 300 with increasing hazard from low to extreme. The odds of the ski runs in Classes 5 and 6 being open decrease by more than a factor of 1000. These alpine ski runs are substantially steeper. Moreover, many of the ski runs or pickup locations can be affected by overhead hazard.
Table 5 shows the odds ratios of ski run classes being open with increasing avalanche hazard relative to ski run Class 1. While the information presented in this table is based on the same information as Table 4, it offers a different perspective by highlighting the relative importance of the various ski run classes at different hazard ratings. For instance, the odds of the runs in Class 2 being open relative to Class 1 increases with increasing avalanche hazard rating. This pattern emerges from the fact that the odds of being open decrease more quickly in Class 1 than in Class 2 (Table 5). A similar pattern can be observed between ski run Classes 4 and 5. The ski runs of Class 4 are approximately 10 times less likely to be open at low hazard conditions than ski runs of Class 1. Similarly, the ski runs in Class 5 are approximately 20 times less likely to be open at low hazard conditions than Class 1. However, the ski runs of Class 5 are closed much more quickly as avalanche hazard increases. The relative odds for the ski runs in Class 4 being open are more than 5 times smaller for extreme avalanche hazard; the relative odds for ski runs in Class 5 are 500 times smaller. The ski runs in Class 6 are more than 100 times less likely to be open with low hazard and 1000 times less likely with extreme avalanche hazard than the ski runs in Class 1.
As expected, our results confirm that the appropriateness of runs for guiding decreases with increasing hazard. However, they also highlight that the effect of avalanche hazard on run list codes depends heavily on the type of terrain that is being assessed. Gentle and frequently skied terrain in all elevation bands with no or only minor exposure to avalanche slopes is much less affected by avalanche hazard. Severe alpine terrain with exposure to either multiple smaller or even large avalanche slopes on the ski runs or exposure to overhead hazard is much more affected by an increase in avalanche hazard. It is important to note that overhead hazard is not only relevant when it affects a skiing line, but also when the associated pickup locations are threatened.
3.2 Effect of avalanche problems and terrain type
Our results show that only certain avalanche problem types influence run list codes and that their effects differ among ski run classes. The presence of deep persistent slab avalanche problems exhibits a negative effect on the ski runs in Classes 5 and 6. This means that runs in severe alpine terrain are much less likely to be open during times when deep persistent slab avalanche problems are a concern (OR = 0.10 and OR = 0.07, Table 3). A similar trend emerged for persistent slab avalanche problems, but only for the ski runs of Class 6, which showed a significant decrease in the likelihood of being open (OR = 0.39). The presence of wet slab avalanche problems, however, exhibited a negative effect on the likelihood of runs being open for all ski run classes (main effect OR = 0.20, Table 2). Finally, we observed a negative effect of wet loose avalanche problems on the ski runs in Class 5 (OR = 0.15).
Compared to the effect of avalanche hazard ratings, the influence of different avalanche problem types is considerably smaller as indicated by the smaller parameter estimates. While hazard ratings reflect the severity of the avalanche hazard conditions in general and affect run codes more globally, avalanche problem types modulate this effect for the specific avalanche situation. For instance, whereas the presence of a widespread storm slab avalanche problem affects the likelihood of ski runs being open equally across all ski run classes, the presence of a deep persistent slab avalanche problem results in a higher likelihood of ski runs with severe alpine terrain with generally steeper or larger avalanche slopes being closed. Similarly, our results only show a significant effect of wet loose avalanche problems on run list coding of severe alpine terrain. While these avalanches are typically confined to surface layers and therefore often small, they can gain size and speed. As such, terrain with severe consequences (e.g., somebody caught in an avalanche being carried into obstacles or over cliffs) seems to be assessed more cautiously.
3.3 Random effects on run level
The insignificance of the run-level random effects of most ski runs (Table 6) highlights that the ski run classes derived by Sterchi and Haegeli (2019) are able to capture the essence of the ski runs, and the realism of the results confirms the suitability of their ski run characterization approach for analysing professional terrain choices in avalanche terrain in a quantitative way. However, the observed significant random effects provide useful insight into factors affecting run list choices of individual ski runs that are not captured by the fixed effects included in the model. Ski runs that exhibit a significant negative random effect are closed more quickly with respect to the particular hazard (i.e., are more sensitive), whereas runs with a significant positive random effect are closed less quickly (i.e., are less sensitive) (Table 6). The run “Sea of Cortez”, for example, is significantly less open than the rest of the ski runs of Class 4 when deep persistent slab avalanche problems are of concern. We suspect that this difference might be caused by the fact that a more severely exposed line of this ski run can be affected by large overhead avalanche hazard. Similarly, the ski run “Pacha Mama” (Class 2) is significantly less open under conditions with higher hazard than the rest of its class. While the least severe ski line on this run only has minor exposure to avalanche hazard, the more severe sections are also exposed to overhead hazard. Both of these examples highlight that certain individual attributes of ski runs can be responsible for significant deviations from the typical assessment of ski runs of similar terrain type.
3.4 Overall insight into the effect of avalanche hazard
Together, the main effects, interaction effects by ski run class, and by-run random effects provide comprehensive insight into the overall effect of avalanche hazard (i.e., rating and avalanche problem presence) on run list choices. While a significant main effect indicates a consistent general response to changes in hazard across the entire run list, significant interaction effects show that specific ski run groups respond differently from the overall pattern described by the main effect. Finally, significant by-run random effects highlight individual runs that deviate substantially from the general and/or ski-run-group-specific response pattern.
The results of our analysis reveal that the run list ratings respond to the hazard rating and the presence of avalanche problems in different ways. The response to the hazard rating is characterized by a significant main effect (Table 2), significant interaction effects for some of the ski run classes (Table 3), and large variations in the by-run random effects with some of them being significant (Table 6). This means the observed general effect is superimposed with ski-run-group- and ski-run-specific responses. The different avalanche problem types influence the run list ratings as follows. For wet slab avalanche problems, only the main effect is significant (Table 2), indicating that the run list ratings of all ski run classes respond to this avalanche problem the same way (Table 3). For deep persistent slab avalanche problems and persistent slab avalanche problems, only certain ski run classes respond (i.e., no main effect, but ski-run-class-specific interactions, Table 3), and certain individual ski runs significantly deviate from the overall class pattern as indicated by the by-run random effects (Table 6). For wet loose avalanche problems, our model shows a non-significant main effect, some significant interaction effects for the different ski run classes, and non-significant by-run random effects without any significant variability among runs. Finally, our model indicates no effect at all for storm slab, wind slab, cornice, and dry loose avalanche problems. This means that the response of the run list ratings to these avalanche problem types is fully captured by the effect of the hazard rating.
Overall, the observed patterns in run list responses seem to be consistent with the existing understanding of different avalanche problems and the complexity of their management (Haegeli et al., 2010; Wagner and Hardesty, 2014). Since simpler avalanche problem types, such as storm slab, wind slab, or dry loose avalanche problems, are typically widespread and result in relatively short-lived spikes of increased avalanche hazard, the required risk management strategies can be captured by a more general relationship between the avalanche hazard rating and terrain class. On the other hand, because the effects of the more complex wet slab, persistent slab, and deep persistent slab avalanche problems can be more localized and/or persist for extended periods, they require more nuanced, avalanche-problem-specific terrain choices that cannot be explained with the hazard rating alone. This is reflected in the avalanche-problem-specific fixed and random effects that emerged from our analysis.
3.5 Effect of run code of the previous day and recent skiing on a run
Whether a run was open the previous day and whether it was skied within the previous week both have a significant influence on it being open (Table 2). Whereas the effect of a run being open the day before increases its odds of being open by a factor of 20, the effect of having recently skied the run is even larger, as it increases the odds of a run that was closed the day before to be open by a factor of 31 (Table 2). This can also be seen from the modelled probability curves for different hazard levels and operational scenario in Fig. 5b that illustrate the model results for a scenario where runs were not open the day before but were recently skied, whereas Fig. 5c shows a scenario where runs were open the day before and recently skied. In both cases, the curves are shifted to the right compared to the base scenario where runs were neither open the day before nor recently skied.
Our results illustrate the strong effect of the run list from the previous day as terrain choices evolve over the course of a season. Terrain choices in mechanized skiing operations are made in stages and are constantly adjusted based on the conditions on the day before incorporating the incremental daily changes (Israelson, 2015). Moreover, the strong effect of previous skiing supports the often-expressed importance by guides of experiencing the conditions and having recent first-hand field observations. This effect is even more important than being open the previous day. As the season progresses, runs that have been skied before and where the guiding team has recent observations about the specific conditions on that run are opened more quickly than comparable runs where such recent experiences are lacking. Previous skiing is an important part of managing risk in heli-skiing as it is considered a compaction and stabilization factor (Clair Israelson, personal communication, 2019). While these results nicely reflect known guiding practices, we were somewhat surprised that the interaction between these two parameters did not turn out to be significant. Together, these results underline the necessity for analysing professional terrain choices in their temporal context. While revealed terrain preference data from GPS tracking units (e.g., Hendrikx et al., 2016; Thumlert and Haegeli, 2018) offer promising avenues for learning about professional avalanche risk management expertise at spatial scales below the run level, it is important to remember that these terrain decisions cannot be analyzed as independent, isolated samples as they are always made in an operational context. It is therefore imperative to analyze the observations in the proper temporal context (i.e., open previously, skied previously) and spatial context (run list codes, run use, skied line on a run) to extract meaningful relationships between hazard and terrain choices that can be generalized.
3.6 Seasonal differences
The random intercepts for season (Fig. 6) reflect differences in the general propensity of runs being open in each season. Our results show that runs were coded open less than half as often during the 2014 winter season compared to other seasons (OR = 0.3). Overall, winter 2014 was characterized by record low snowpack heights, which especially affected the closure of low-elevation ski runs due to the marginal snowpack or increased skiing hazards for the guests. In addition, a persistent weak layer that was buried mid-season and remained a concern for the remainder of the season was responsible for more frequent closures of the more severe ski runs.
This result highlights that having long-term datasets is critical for identifying meaningful patterns in risk management practices as the unique characteristics of individual winters can affect observed choices considerably. Since we are interested in extracting generalizable terrain choice rules, it is important to work with statistical methods that can account for such random deviations. Hence, mixed-effect models are an excellent approach for analysing terrain choices as they properly account for the nested structure of terrain selection datasets.
3.7 Limitations and future challenges
While the present results offer valuable quantitative insight into the relationship between avalanche hazard and run list codes at NEH, there are several potential avenues for exploring these relationships further and developing operational decision aids that offer value to guiding teams. While the present model only included a relatively crude representation of avalanche hazard (i.e., hazard rating and presence of avalanche problems), a more complete characterization of avalanche hazard according to the CMAH (Statham et al., 2018) could reveal more detailed insights about the suitability of runs under specific avalanche hazard conditions. For example, explicitly including aspect, the likelihood of avalanches and destructive size parameters of the existing avalanche problems in the run list model has the potential to extract more detailed information about the relationship between the avalanche hazard situation and the characteristics of runs with appropriate skiing terrain. Similarly, integrating more detailed ski run characteristics into the analysis might also help to reveal additional insight. Even though using the operation-specific ski run classes of Sterchi and Haegeli (2019) was a conscious choice to limit the complexity of this first quantitative analysis to a reasonable level, future research in this area will need to isolate the operation-specific intricacies so that the identified patterns between avalanche hazard and terrain can be generalized across operations. However, taking this research to this level will require operational datasets of run list choices and avalanche hazard information that are substantially larger than the dataset used in the present study.
Using a large, multi-seasonal dataset of operational run list choices from a mechanized skiing operation, we applied a general linear mixed-effect model to quantitatively explore the relationship between appropriate skiing terrain (i.e., open or closed for guiding) and avalanche hazard conditions at the run list scale for the first time. Our model included an avalanche hazard rating and eight binary variables indicating the presence of different avalanche problem types as predictors and the class of the ski run, whether it was skied in the previous week and how it was rated on the previous day as covariates. In addition, by-run and by-season random effects were incorporated into the model to account for the panel structure of the dataset.
Our results show that the effect of avalanche hazard on run list codes depends heavily on the type of ski run that is being assessed and the nature of the avalanche hazard. While the run list ratings of the gentlest terrain are only marginally affected by hazard ratings, severe alpine terrain is especially susceptible to increasing avalanche hazard. Compared to the effect of the avalanche hazard rating, the effects of the different avalanche problem types on the run list codes are small but represent critical, ski-run-class-specific adjustments. Our results also highlight the strong effect of recent skiing and thus experiencing the conditions and having recent first-hand field observations on run list codes. This result reflects the fact that guides reopen runs they have recently skied more quickly than other comparable runs. The strong effect of the run code of the previous day highlights that terrain choices in mechanized skiing are evolving over the course of a season and further underline the necessity for analysing professional terrain choices in their temporal context.
While our results primarily confirm expectations, we believe this study provides a valuable step towards describing the terrain selection process at mechanized skiing operations numerically in a meaningful way. For the first time, the effect of avalanche hazard has been isolated from the influence of other factors such as the run list code the day before and the effect of recent skiing. Properly isolating these effects is critical for describing the relationship between avalanche hazard and appropriate terrain in a meaningful fashion. In addition to offering insight into the run list coding process, the present research also provides important context for the analysis of small-scale terrain choices in avalanche terrain (e.g., analysis of GPS tracks) since the terrain choices in mechanized skiing are made in stages and the decisions made in the field critically depend on the choices of eliminating unsuitable runs made during the preceding guide meeting.
In the long-term, this body of research will develop the foundation for the design of evidence-based operational decision aids that can help guides to make terrain choices more efficiently. It is important to note that we do not envision that these decision aids will actually make guiding decisions or will be used for external auditing purposes as suggested by Hendrikx et al. (2016). However, if designed correctly, such decision aids may offer independent references that allow guides to check their morning run lists against their own historical decisions under similar conditions. Furthermore, the knowledge gained from these models may create the necessary foundation for the development of evidence-based terrain guidance tools for recreationists in the future.
The operational data used in the study are the intellectual property of the collaborating companies and can therefore not be made available by the author team.
RS, PH, and PM co-led the design of this study. RS conducted the statistical analysis and authored the initial draft of the paper. PM consulted on the statistical analysis. RS, PH, and PM subsequently edited the paper collaboratively to produce the final version of the paper.
The authors declare that they have no conflict of interest.
We would like to thank Northern Escape Heli Skiing for their willingness to participate in this study.
This research was funded through the NSERC Industrial Research Chair in Avalanche Risk Management at Simon Fraser University (SFU), which is financially supported by Canadian Pacific Railways, HeliCat Canada, the Canadian Avalanche Association, and Mike Wiegele Helicopter Skiing in collaboration with the Natural Sciences and Engineering Research Council of Canada and Simon Fraser University.
This paper was edited by Margreth Keiler and reviewed by three anonymous referees.
Bolker, B. M., Brooks, M. E., Clark, C. J., Geange, S. W., Poulsen, J. R., Stevens, M. H. H., and White, J.-S. S.: Generalized linear mixed models: a practical guide for ecology and evolution, Trends Ecol. Evol., 24, 127–135, https://doi.org/10.1016/j.tree.2008.10.008, 2009.
Bruns, W.: Snow Science and Safety for the Mountain Guide, In: Proceedings of the International Snow Science Workshop, Banff, AB, Canada, 203–206, 1996.
Campbell, C. and Gould, B.: A proposed practical model for zoning with the Avalanche Terrain Exposure Scale, in: Proceedings of the International Snow Science Workshop, Grenoble – Chamonix Mont-Blanc, France, 385–391, 2013.
Canadian Avalanche Association: Observation guidelines and recording standards for weather, snowpack and avalanches, Revelstoke, BC, Canada, 2014.
Canadian Avalanche Association: Avalanche Hazard Rating Scale. InfoEx Advisory Committee, available at: http://infoexhelp.avalancheassociation.ca/wiki/Hazard_rating_definition_table (last access: 3 July 2019), 2015.
Gelman, A. and Rubin, D. B.: Inference from Iterative Simulation Using Multiple Sequences, Statist. Sci., 7, 457–472, https://doi.org/10.1214/ss/1177011136, 1992.
Grímsdottír, H.: Avalanche risk management in backcountry skiing operations, Master, University of British Columbia, Vancouver, BC, Canada, 173 pp., 2004.
Haegeli, P.: Examining professional avalanche expertise for the next-generation Avaluator, Avisualanche Consulting, Vancouver, BC, Canada, 2010.
Haegeli, P., Atkins, R., and Klassen, K.: Decision making in avalanche terrain: a field book for winter backcountry users, Canadian Avalanche Centre, Revelstoke BC, Canada, 62 pp., 2010.
Harrison, X. A., Donaldson, L., Correa-Cano, M. E., Evans, J., Fisher, D. N., Goodwin, C. E. D., Robinson, B. S., Hodgson, D. J., and Inger, R.: A brief introduction to mixed effects modelling and multi-model inference in ecology, PeerJ, 6, e4794, https://doi.org/10.7717/peerj.4794, 2018.
HeliCat Canada: A social and economic impact assessment of helicopter and snowcat skiing in British Columbia, available at: https://drive.google.com/file/d/0B_2rsFOgCq8VdkRBdXBlSDRnX2M/view?usp=sharing (last access: 18 January 2019), 2016.
Hendrikx, J., Johnson, J., and Shelly, C.: Using GPS tracking to explore terrain preferences of heli-ski guides, Journal of Outdoor Recreation and Tourism, 13, 34–43, https://doi.org/10.1016/j.jort.2015.11.004, 2016.
Israelson, C.: A suggested conceptual model for daily terrain use decisions and Northern Escape Heli-Skiing, The Avalanche Journal, 110, 39–43, 2015.
Long, J. D.: Longitudinal data analysis for the behavioral sciences using R, SAGE, Thousand Oaks, CA, USA, 542 pp., 2012.
McClung, D. M. and Schaerer, P. A.: The Avalanche Handbook, 3rd ed., The Mountaineers, Seattle, WA, USA, 342 pp., 2006.
R Core Team: R: A language and environment for statistical computing, R Foundation for Statistical Computing, Vienna, Austria, available at: https://www.R-project.org/, last access: 19 January 2019.
Shandro, B. and Haegeli, P.: Characterizing the nature and variability of avalanche hazard in western Canada, Nat. Hazards Earth Syst. Sci., 18, 1141–1158, https://doi.org/10.5194/nhess-18-1141-2018, 2018.
Stan Development Team: rstanarm: Bayesian applied regression modeling via Stan, R package version 2.13.1, available at: http://mc-stan.org/ (last access: 18 January 2019), 2016.
Statham, G., McMahon, B., and Tomm, I.: The avalanche terrain exposure scale, in: Proceedings of the International Snow Science Workshop, Telluride, CO, USA, 491–497, 2006.
Statham, G., Haegeli, P., Greene, E., Birkeland, K. W., Israelson, C., Tremper, B., Stethem, C. J., McMahon, B., White, B., and Kelly, J.: A conceptual model of avalanche hazard, Nat. Hazards, 90, 663–691, https://doi.org/10.1007/s11069-017-3070-5, 2018.
Sterchi, R. and Haegeli, P.: A method of deriving operation-specific ski run classes for avalanche risk management decisions in mechanized skiing, Nat. Hazards Earth Syst. Sci., 19, 269–285, https://doi.org/10.5194/nhess-19-269-2019, 2019.
Thumlert, S. and Haegeli, P.: Describing the severity of avalanche terrain numerically using the observed terrain selection practices of professional guides, Nat. Hazards, 91, 89–115, https://doi.org/10.1007/s11069-017-3113-y, 2018.
Wagner, W. and Hardesty, D.: Travel advice for the avalanche problems: A public forecasting tool, in: Proceedings of the International Snow Science Workshop, Banff, AB, Canada, 2014.
Walcher, M., Haegeli, P., and Fuchs, S.: Risk of Death and Major Injury from Natural Winter Hazards in Helicopter and Snowcat Skiing in Canada, Wild. Environ. Med., 30, 251–259, https://doi.org/10.1016/j.wem.2019.04.007, 2019.
Zuur, A. F., Ieno, E. N., Walker, N., Saveliev, A. A., and Smith, G. M.: Mixed effects models and extensions in ecology with R, Springer New York, https://doi.org/10.1007/978-0-387-87458-6, 2009.
Please note that NEH only uses eight types of avalanche problems as they do not specify glide avalanche problems.