the Creative Commons Attribution 4.0 License.
the Creative Commons Attribution 4.0 License.
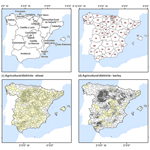
The impact of drought on the productivity of two rainfed crops in Spain
Marina Peña-Gallardo
Sergio Martín Vicente-Serrano
Fernando Domínguez-Castro
Santiago Beguería
Drought events are of great importance in most Mediterranean climate regions because of the diverse and costly impacts they have in various economic sectors and on the environment. The effects of this natural hazard on rainfed crops are particularly evident. In this study the impacts of drought on two representative rainfed crops in Spain (wheat and barley) were assessed. As the agriculture sector is vulnerable to climate, it is especially important to identify the most appropriate tools for monitoring the impact of the weather on crops, and particularly the impact of drought. Drought indices are the most effective tool for that purpose. Various drought indices have been used to assess the influence of drought on crop yields in Spain, including the Standardized Precipitation Evapotranspiration Index (SPEI), the Standardized Precipitation Index (SPI), the Palmer drought indices (Palmer Drought Severity Index, PDSI; Palmer Z Index, Z Index; Palmer Hydrological Drought Index, PHDI; Palmer Modified Drought Index, PMDI), and the Standardized Palmer Drought Index (SPDI). Two sets of crop yield data at different spatial scales and temporal periods were used in the analysis. The results showed that drought indices calculated at different timescales (SPI, SPEI) most closely correlated with crop yield. The results also suggested that different patterns of yield response to drought occurred depending on the region, period of the year, and the drought timescale. The differing responses across the country were related to season and the magnitude of various climate variables.
- Article
(9218 KB) - Full-text XML
-
Supplement
(1723 KB) - BibTeX
- EndNote
The Mediterranean region is one of the major areas in Europe likely to be subject to the potential impacts of climate change. Many semiarid regions of southwestern Europe are expected to undergo a critical decline in water availability as a consequence of reduced precipitation and an increase in interannual and intra-annual rainfall variability (IPCC, 2014, EEA, 2017). It is also expected that future changes in the precipitation regime, along with a rise in temperature, will inevitably bring more extreme and severe weather events (Giorgi and Lionello, 2008; Webber et al., 2018; Wigley, 2009) that will impact ecosystems and economic sectors (Asseng et al., 2014; Tack et al., 2015). It has been suggested that precipitation and temperature changes in the western Mediterranean region will lead to more severe and longer drought events in coming decades (Alcamo et al., 2007; Dai, 2011; Forzieri et al., 2016; Giorgi and Lionello, 2008; Spinoni et al., 2018; Vicente-Serrano et al., 2014). This is significant because agriculture plays a key role in food supply; in 2017 it accounted for 2.59 % of GDP in Spain, 1.92 % in Italy, and 3.53 % in Greece (World Bank, 2017).
The agriculture sector is highly vulnerable to drought, as it depends directly on water availability (Hanjra and Qureshi, 2010; Meng et al., 2016; Tsakiris and Tigkas, 2007). Although each crop differs in its resilience to water stress (Liu et al., 2016; Lobell et al., 2011), droughts can cause crop failure if the weather conditions are adverse during the most sensitive stage of crop growth (Lobell and Field, 2007). The adverse impacts of drought have been highlighted in recent severe events, including in 2003 when the agricultural and forestry losses from drought in France, Italy, Germany, Spain, Portugal, and Austria were approximately EUR 13 billion (Fink et al., 2004; García-Herrera et al., 2010). The most recent drought, which mostly affected north–central Europe, caused European farmers to claim agricultural aid because of the low production that resulted (European Commission, 2018).
For these reasons the vulnerability of agricultural production to extreme events and the quantification of drought impacts on crop yields have become a focus of interest. In recent years diverse studies in the Mediterranean region have assessed these issues from multiple perspectives. For example, Capa-Morocho et al. (2016) investigated the link between seasonal climate forecasts and crop models in Spain, Loukas and Vasiliades (2004) used a probabilistic approach to evaluate the spatiotemporal characteristics of drought in an agricultural plain region in Greece, and Moore and Lobell (2014) estimated the impacts of climate projections on various crop types across Europe.
Droughts are difficult to measure and quantify (Vicente-Serrano et al., 2016), and consequently a wide range of drought indices have been developed to provide tools for quantifying the effects of drought across different sectors (Zargar et al., 2011). In this respect, drought indices are the most widely used method for monitoring drought impacts on agriculture; examples of their use available in the scientific literature include that in Europe (Hernandez-Barrera et al., 2016; Potopová et al., 2016a; Sepulcre-Canto et al., 2012; Vergni and Todisco, 2011), America (McEvoy et al., 2012; Quiring and Papakryiakou, 2003), and Asia (Ebrahimpour et al., 2015; Wang et al., 2016a). However, there is no general consensus on the most suitable indices for this purpose (Esfahanian et al., 2017). Despite the existing literature, very few studies (Peña-Gallardo et al., 2018a; Tian et al., 2018) have compared drought indices to identify their appropriateness for monitoring drought impacts on agriculture and for various crop types.
Among Mediterranean countries, agriculture in Spain is particularly sensitive to climate because of the low average precipitation level and its marked interannual variability (Vicente-Serrano, 2006). Spain has been subject to multiple episodes of drought (Domínguez-Castro et al., 2012), with those in the last century being amongst the most severe to have occurred in Europe (González-Hidalgo et al., 2018; Vicente-Serrano, 2006). In 2017 the agricultural and livestock losses caused by drought were estimated to be at least EUR 3600 million (UPA, 2017), highlighting the need to establish appropriate tools for monitoring drought impacts on crops. Recent studies such as that conducted by Ribeiro et al. (2019) in the Iberian Peninsula stressed the risk of this region of suffering from yield losses in the context of climate change. For that purpose, these authors analyzed the exposure of cereal rainfed crops to drought conditions using remote sensing information and performing a multi-scalar drought index.
Information on crop production is commonly limited in terms of spatial or temporal availability. Recent studies in Spain have analyzed the impact of climate on various crops since the early 21st century at national or provincial scales (Cantelaube et al., 2004; Hernandez-Barrera et al., 2016; Páscoa et al., 2016; Ribeiro et al., 2019), but few have used yield data at finer resolution (García-León et al., 2019). In this study we compared different drought indices using two datasets at different spatial scales: provincial information provided by the national statistical services and a regional dataset specifically developed for the study. The objectives of this study were (1) to determine the most appropriate and functional drought index among four Palmer-related drought indices (Palmer Drought Severity Index, PDSI; Palmer Hydrological Drought Index, PHDI; Palmer Z Index, Z Index; Palmer Modified Drought Index, PMDI) and the Standardized Precipitation Evapotranspiration Index (SPEI), the Standardized Precipitation Index (SPI), and the Standardized Palmer Drought Index (SPDI); (2) to identify the temporal response of two main herbaceous rainfed crops (wheat and barley) to drought; and (3) to determine whether there were common spatial patterns, by comparing the two datasets at different spatial scales.
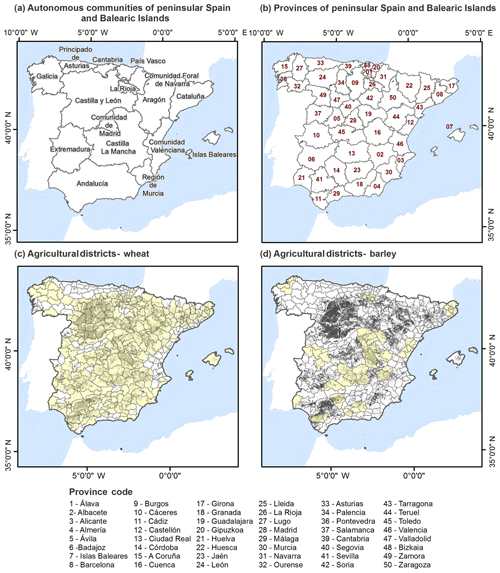
Figure 1Location of Spanish autonomous communities (a) and provinces (b) and the distribution of agricultural districts with data available (yellow) for wheat (c) and barley (d) yields for the period 1993–2015. Areas where rainfed cereal crops are cultivated (Corine Land Cover 2006) are shown in grey.
2.1 Crop yield data
The statistical analysis was conducted using an annual dataset of crop yields for peninsular Spain and the Balearic Islands at two spatial scales for the two main herbaceous rainfed crops (barley and wheat). We obtained provincial annual yield data from the National Agricultural Statistics Annuaries published by the Spanish Ministry of Agriculture, Fishing and Environment (MAPA), available at https://www.mapa.gob.es/es/estadistica/temas/publicaciones/anuario-de-estadistica/default.aspx (last access: March 2018); these include agricultural statistics since the early 20th century. We used data from 1962 to 2014 to match climate data that were available for this period. The Gipuzkoa and Vizcaya provinces were not used in the analysis at the province scale as wheat has not been cultivated there since 1973 and 1989, respectively. We used crop production data collected by the Encuesta sobre Superficies y Rendimientos de Cultivos (ESYRCE; Survey on surface and crop yields), an agrarian yield survey that has been undertaken by the MAPA since 1990. This survey records information about crop production at parcel scale every year from a sample of parcels. Yield observations were aggregated to the main spatial unit defined for agricultural districts by the MAPA (Fig. 1). As not all territories were included in this survey until 1993, we only considered the period 1993–2015. Data on barley production are limited in the ESYRCE database, and the agricultural districts considered in this study did not correspond to all the areas where this crop is cultivated.
For both datasets the unit of measure was the harvested production per unit of harvested area (kg ha−1); it did not include any measure of production related to the area of the crop planted in each province or region. To consider the total area covered by the crops we used the defined rainfed crop delimited area for Spain, derived from the Corine Land Cover 2000 database (http://centrodedescargas.cnig.es/CentroDescargas/catalogo.do?Serie=MPPIF, last access: March 2018).
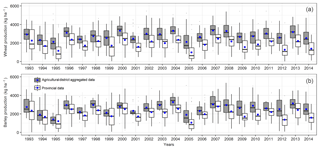
Figure 2Temporal series of wheat (a) and barley (b) yields for the provincial data and the aggregated agricultural district data at the province scale for the common period 1993–2014. The solid black line shows the median, and the blue dot shows the mean.
The spatial resolution of yield data can influence the interpretation of drought impacts on agriculture. Figure 2 shows a comparison of crop yields for the common period of available information in both datasets (1993–2014). Overall, the average production was greater at the agricultural district scale than at the provincial scale. Tables S1 and S2 (in the Supplement) summarize the relationships between the datasets for each province for the available common period, based on Pearson's correlations coefficients for wheat and barley yields, respectively. It was surprising that both datasets showed very different temporal variability in crop yields in the analyzed provinces. Wheat yields showed good agreement and highly significant correlations between both datasets in provinces including Ávila (r=0.77), Barcelona (r=0.69), Burgos (r=0.82), Cuenca (r=0.86), Guadalajara (r=0.87), León (r=0.69), Palencia (r=0.73), Salamanca (r=0.87), Segovia (r=0.94), Teruel (r=0.83), Valladolid (r=0.92), and Zamora (r=0.75), while in other provinces including Castellón, Málaga, Murcia, and Navarra the correlations were nonsignificant or negative. Thus, the national statistics for these districts were unreliable. For barley yields the available regional data were more limited, but similar relationships with good agreement and more highly significant correlations were found among the datasets for the provinces where wheat was also cultivated, including Cáceres (r=0.48), Cuenca (r=0.88), Granada (r=0.51), Guadalajara (r=0.86), La Rioja (r=0.76), and Tarragona (r=0.88); however, for Sevilla the correlation was negative and significant ().
Mechanization and innovation in agriculture have increased in the last century, resulting in a trend of increased yields (Lobell and Field, 2007), which is also evident in data for Spain. To remove bias introduced by non-climate factors, and to enable comparison of yields between the two crop types, the original series were transformed to standardized yield residuals series (SYRS) using the following quadratic polynomial equation:
where yd denotes the residuals of the de-trended yield obtained by fitting a linear regression model, μ is the mean of the de-trended series, and σ is the standard deviation of the de-trended yield.
This methodology has been applied in other similar studies (Chen et al., 2016; Tian et al., 2018). First announced as SYRS by Potopová et al. (2015), the full procedure of the following methodology is described by Lobell and Asner (2003) and Lobell et al. (2011). In Fig. S1 (Supplement) an example of the positive trend (more evident in the provincial data due to the length of available data) and the temporal evolution of SYRS is illustrated for both type of crops and spatial scale.
2.2 Climate data
We used a weekly gridded dataset of meteorological variables (precipitation, maximum and minimum temperature, relative humidity, and sunshine duration) at 1.1 km resolution for peninsular Spain and the Balearic Islands for the period 1962–2015. The grids were generated from a daily meteorological dataset provided by the Spanish National Meteorological Agency (AEMET), following quality control and homogenization of the data. Further details on the method and the gridding procedure are provided by Vicente-Serrano et al. (2017). Reference evapotranspiration (ETo) was calculated using the FAO-56 Penman–Monteith equation (Allen et al., 1998). Weekly data were aggregated at the monthly scale for calculation of the various drought indices.
2.3 Methods
2.3.1 Drought indices
Palmer drought indices
Palmer (1965) developed the Palmer Drought Severity Index (PDSI). Variations of this index include the Palmer Hydrological Drought Index (PHDI), the Palmer Moisture Anomaly Index (Z Index), and the Palmer Modified Drought Index (PMDI). Computation of the Palmer indices is mainly based on estimation of the ratio between the surface moisture and the atmospheric demand. Subsequent studies have revealed that spatial comparison among regions is problematic (Alley, 1984; Doesken and Garen, 1991; Heim, 2002). In this context we followed the variation introduced by Wells et al. (2004); this enables spatial comparison when determining a suitable regional coefficient, developing the self-calibrated Palmer indices. Palmer indices are also referred to as uni-scalar indices, which can only be calculated at fixed and unknown timescales (Guttman, 1998; Vicente-Serrano et al., 2010); this is a limitation of these indices.
Standardized Precipitation Index (SPI)
The Standardized Precipitation Index (SPI) was introduced by Mckee et al. (1993) and provided a new approach to the quantification of drought at multiple timescales. The index is based on the conversion of precipitation series to a standard normal variable, with a mean equal to 0 and variance equal to 1, by adjusting an incomplete gamma distribution. The SPI is a meteorological index used worldwide and is especially recommended by the World Meteorological Organization (WMO, 2012) for drought monitoring and early warning.
Standardized Precipitation Evapotranspiration Index (SPEI)
Vicente-Serrano et al. (2010) proposed the Standardized Precipitation Evapotranspiration Index (SPEI) as a drought index that takes into consideration the effect of atmospheric evaporative demand on drought severity. It provides monthly climate balances (precipitation minus reference evapotranspiration), and the values are transformed to normal standardized units using a three-parameter log-logistic distribution. Following the concept of the SPI, the SPEI enables comparison of drought characteristics at various timescales among regions, independently of their climatic conditions. The SPEI has been widely used in drought-related studies, including to investigate the impacts of drought on various crops worldwide (Chen et al., 2016; Kuhnert et al., 2016; Peña-Gallardo et al., 2018b; Potopová et al., 2016b; Vicente-Serrano et al., 2012).
Standardized Precipitation Drought Index (SPDI)
The Standardized Precipitation Drought Index (SPDI) was developed by Ma et al. (2014) and relies on the concept of timescales. It is considered to be a combined version of the PDSI and the SPEI because the SPDI accumulates the internal water balance anomalies (D) obtained in the PDSI scheme at various timescales, and the values are later transformed into z units following a standard normal distribution. For this purpose a log-logistic distribution has been used because this has been shown to be effective at the global scale (Vicente-Serrano et al., 2015).
The SPEI, SPI, and SPDI are referred to here as multi-scalar indices and the Palmar drought indices as uni-scalar indices. Thus, the multi-scalar indices were computed at scales of 1, 12, 18, and 24 months and along with the Palmer drought indices series were de-trended by adjusting a linear regression model to enable accurate comparisons with de-trended crop yield information. Following the same procedure used for the yield series, the residual of each monthly series was summed to the average value for the period.
2.3.2 Correlation between drought indices and crop yields
The relationship between the drought indices and the SYRS for both datasets was assessed by calculating polynomial correlation coefficients (c) (Baten and Frame, 1959). We used a second-order polynomial regression model, given the common nonlinear relationship between drought indices and crop production (Páscoa et al., 2016; Zipper et al., 2016). Hereafter, the references made to correlations refer to results obtained using the polynomial approach. The months of August and September were excluded from the analysis because they correspond to the post-harvest period, and we were considering only the period from sowing to harvest.
As the month of the year when the greatest correlation between the drought index and the crop yield was not known beforehand, all 10 monthly series for each index were correlated with the annual yield, and the highest correlation value was used. In the case of the multi-scalar indices, for each monthly series and timescale we obtained 10 correlations (one for each of the 10 months and the 14 timescales considered in the analysis). Thus, 140 correlations were obtained for each crop and spatial unit considered in the analysis (only correlations significant at p<0.05 were considered). In addition, we used the timescale (in the case of multi-scalar drought indices) and the month in which the strongest correlation was found.
A t test was performed to assess the significance of the differences in the polynomial regression correlation coefficients obtained from the drought–yield relationships, to determine whether there were significant similarities or differences among the indices.
2.4 Identification of spatial patterns for crop yield response to drought
A principal component analysis (PCA) was performed to identify general patterns in the effect of drought on crop yields, in relation to seasonality of the effects. PCA is a mathematical technique that enables the dimensionality of a large range of variables to be reduced, by fitting linear combinations of variables. We conducted a T-mode analysis, and used the varimax method to rotate the components to obtain more spatially robust patterns (Richman, 1986). The monthly series of the monthly maximum correlation values found from the yield–drought relationship were the variables (one data point per month), and the provinces and agricultural districts were the cases. We selected two principal components (PCs) that in combination explained >60 % of the variance (individually the other components explained <5 % of the variance) and aggregated each province or agricultural district according to the maximum loading rule (i.e., assigning each spatial unit to the PC for which the highest loading value was found). The loadings were expressed in the original correlation magnitudes using the matrix of component weights.
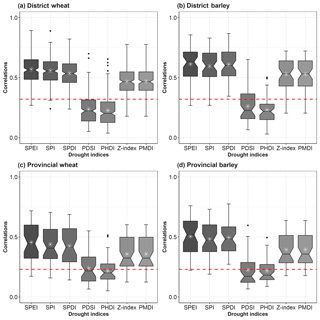
Figure 3Box plots showing the strongest correlation coefficients found between drought indices and wheat and barley yields at the agricultural district (a and b) and provincial (c and d) scales, for all districts and provinces analyzed. The solid black line shows the median, the white asterisk shows the mean, and the dashed red lines show the p<0.05 significance level.
3.1 Relationship of drought indices to crop yields
Figure 3 shows the strongest correlation found between the crop yield for each dataset and the monthly drought indices. The correlations differed substantially between the two groups of indices. Independently of the crop type, month of the year, or the drought timescale considered, the correlation coefficients for the multi-scalar indices were much higher than those for the uni-scalar indices. In both cases weaker correlations were found for the wheat crops compared with the barley crops. The PDSI, PHDI, and PMDI correlations were nonsignificant (p<0.05), but the correlations for the Z Index and the multi-scalar indices were significant for most provinces and agricultural districts. The correlation values for the three multi-scalar drought indices were similar. At district scale the average values were c=0.57 and c=0.6 for wheat and barley, respectively, and c=0.41 and c=0.48 at the provincial scale. Thus, the datasets showed a stronger correlation for the drought indices at district scale than at the provincial scale. In addition, more variability was found in the provincial data than in the regional data, associated with the length of the available records.
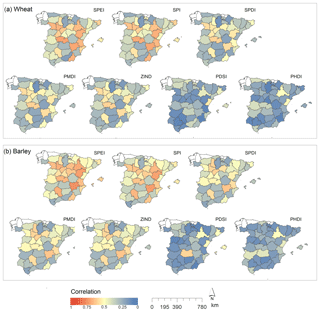
Figure 4Spatial distribution of the highest correlation coefficients between the drought indices and the wheat (a) and barley (b) yields at the provincial scale, independently of the timescale.
The spatial distribution of the maximum correlation coefficients between the drought indices and the crop yields is shown in Figs. 4 and 5, for the province and district scales, respectively. The wheat and barley yield–drought correlations showed a similar spatial pattern among indices at the province scale. Stronger correlations (c≥0.7) were found for the SPEI and SPI for the provinces of Castilla y León (Valladolid, Zamora, Segovia, and Soria), Aragón (Zaragoza and Teruel), Castilla La Mancha (Guadalajara, Albacete, and Toledo), and Valencia (particularly the cereal agricultural districts). The weakest correlations were found for the southern (Andalusian) provinces. For the Palmer drought indices, the PMDI and Z Index showed similar spatial patterns to the multi-scalar indices (especially in the central and northern provinces), but the correlations were weaker (c=0.25–0.6). For most provinces the weakest correlations were found for the PDSI and PHDI (c=0.1–0.25) for both crops, with no clear spatial difference in the correlations.
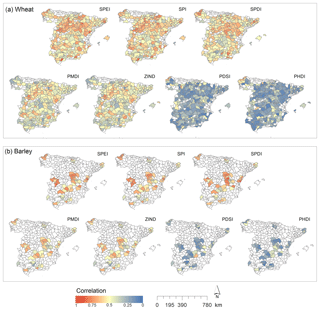
Figure 5Spatial distribution of the highest correlation coefficients between the drought indices and the wheat (a) and barley (b) yields at the agricultural district scale, independently of the timescale.
The spatial distribution of correlations between wheat yields and the drought indices at the agricultural district scale showed clearer patterns than those for the province level. Thus, the response of drought indices at district scale is similar to the response observed at provincial scale, showing stronger correlations for the multi-scalar indices and weaker correlations for the Palmer indices, especially the PDSI and PHDI. The distribution of correlations among the multi-scalar indices was very similar. The most correlated agricultural districts (c≥0.8) were in Castilla y León, especially Valladolid, Segovia, north of Ávila, and northeast of Salamanca. Similar correlations were found for areas of northeast Spain. There was a gradient in correlations from north to south, with the exception of some districts in northwestern Málaga, where wheat is extensively cultivated. In addition, in some districts of Galicia, where expansion of the planted wheat area has not been large, there was a strong relationship between drought indices and crop yields. The results for barley suggest a similar spatial relationship for the various drought indices. The highest coefficients were found for the multi-scalar indices, followed by the Z Index and the PMDI, with districts north of Cáceres, north of Galicia, and in Guadalajara showing correlations on the order of c=0.8, while the correlations were weaker (c=0.25–0.4) in districts in the south of Córdoba and Jaén.
3.2 Relationship of drought indices to crop yields: temporal responses
Table 1 summarizes the timescales at which the strongest correlations were found for each of the three multi-scalar indices. Strongest correlations were found for short timescales (1–3 months) for both datasets and both crops, in general with little difference between the indices. For wheat, for 52.6 % of the agricultural districts the yield was most strongly correlated with all three drought indices at a timescale of 1–3 months; this was also the case for 49.6 % of provinces. In agricultural districts where wheat is cultivated the strongest correlations were predominantly at the 1-month scale (20.37 %), especially for the SPDI, while for most of the provinces this occurred at the 3-month scale, particularly for the SPEI and SPI (23.26 %). For barley, 57.4 % of the districts and 58.7 % of provinces where this crop was grown, the strongest correlations were predominantly at 1- to 3-month timescales. Among the various indices for districts, the SPI showed the strongest correlation at the 1-month scale, while for provinces the SPEI showed the strongest correlation at the 3-month scale (33.33 %).
Table 2Percentage of analyzed agricultural districts and provinces where wheat and barley are cultivated, for which the maximum correlations with the multi-scalar indices were found. Information in parentheses shows the timescale at which the provinces and agricultural districts correlate most and the percentage of the provinces and district.
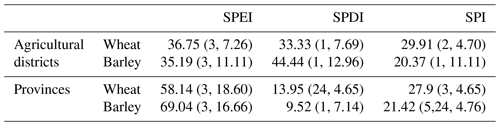
The multi-scalar drought indices showed similar results. Among these, the SPEI was the index most strongly correlated with yield in the highest percentage of provinces and districts (Table 2). For wheat crops the SPEI was the most strongly correlated index with yield in ∼37 % of the agricultural districts and ∼58 % of the provinces; these correlations were found predominantly at the 3-month timescale. For this crop the SPDI was most strongly correlated with yield in a similar proportion of districts (∼33 %), primarily at the 1-month scale, but only ∼14 % at the province scale. In general, most of the maximum correlations corresponded to short timescales.
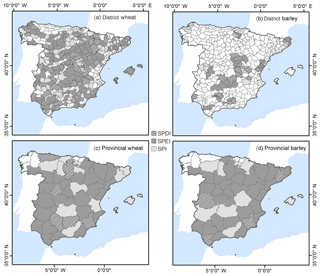
Figure 6Spatial distribution of the drought indices with the strongest correlations with wheat (a, c) and barley (b, d) at the province (c, d) and agricultural district (a, b) scales.
Figure 6 shows the spatial distribution of the most strongly correlated drought indices. For most of the provinces the SPEI was the index most strongly correlated with crop yield. For the agricultural districts there was substantial spatial variability and, along with the provincial results, no well-defined spatial pattern that distinguished specific areas for which one index was most effective at monitoring drought. For barley the SPDI showed the best correlation with yield among districts (∼44 %), while in provinces the SPEI was best correlated (∼69 %). No clear spatial patterns were evident. The similarities in the magnitude of the correlations between multi-scalar drought indices and crop yields were statistically significant. A t test (Fig. S2) was used to determine whether there were significant differences in the magnitude of correlations obtained using the various multi-scalar drought indices. This showed significant differences between the SPEI and the SPDI in ∼30 % of agricultural districts where wheat was grown; these were districts that showed a weaker correlation of yield with drought indices. The results suggest that, for districts with strong correlations between drought indices and crop yields, the two indexes were equally useful. A lower proportion of districts where barley is planted showed that statistical differences among indices exist. In contrast, for provinces no significant differences were found. Overall, this suggests the appropriateness of using any of these multi-scalar indices indistinctly.
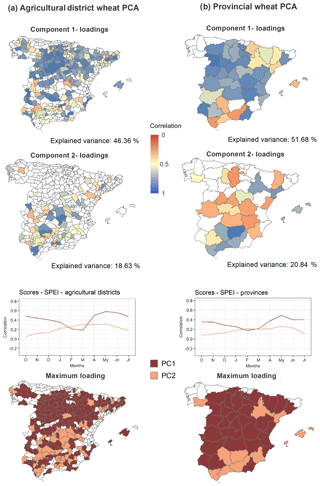
Figure 7PC loadings, PC scores, timescales, and maximum loading rules from the PCA for monthly maximum correlation coefficients between the SPEI and wheat yields at the agricultural district (a) and provincial (b) scales, independently of the timescale. The PC loadings and maximum loadings were significant at p<0.05.
3.3 Spatial patterns of drought index correlations at the monthly scale
Regionalization of the crop yield response to drought based on monthly correlations with the drought indices was undertaken in relation to the most correlated drought index in each region, independently of the month in which this maximum correlation occurred. Thus, in this analysis the results obtained using the various multi-scalar drought indices were merged. General spatial patterns in the effect of drought conditions on yield were identified using a T-mode PCA. Figures 7 and 8 show the results for the provincial and regional datasets, respectively. We selected two components that explained more than the 60 % of the variance in each case. This classification reinforced the north–south pattern of correlations previously found for both datasets. Figure 9 shows the timescales for which the maximum monthly correlations were found for the provinces and agricultural districts for each of the defined components, using a maximum loading rule.
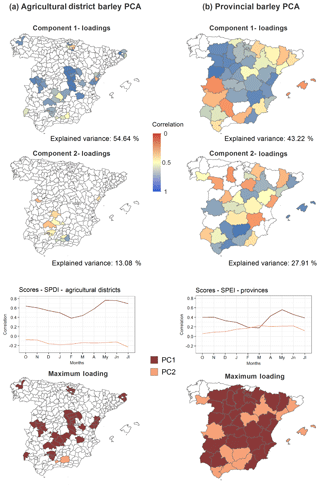
Figure 8PC loadings, PC scores, timescales, and maximum loading rules from the PCA for monthly maximum correlation coefficients between the SPEI and barley yields at the agricultural district scale (a) and the SPDI and barley yields at the provincial scale (b), independently of the timescale. The PC loadings and maximum loadings were significant at p<0.05.
3.3.1 Wheat
Agricultural district scale
At the district scale the PCA for wheat (Fig. 7a) showed more defined spatial patterns than the PCA did at the provincial scale. The first component (PC1) explained 43.36 % of the variance and was characterized by stronger correlations (c=0.7–0.9) in districts mainly located on the north and central plateau; these were stronger than those recorded for the same locations at the provincial scale. Weaker correlations (c=0.15–0.5) were dispersed, although these were found predominantly in the south and northwest. The scores for PC1 showed particular sensitivity to drought during spring, although strong correlations were also found during autumn. The second component (PC2) explained 18.63 % of the variance, and the loading coefficients also showed a clear spatial pattern, with the agricultural districts north of Sevilla and east of Castilla La Mancha having the highest values. The weakest correlations were found for the districts of Andalucía, Extremadura, and Aragón. Lower scores in PC2 characterized the interannual response to drought relative to PC1. These districts in PC2 also showed a stronger response during spring but not autumn, as was found for PC1. The distribution of PCs according to the maximum loading rule enabled identification of a north–south component in the sensitivity of wheat yields to the drought index. The timescales at which wheat yields in agricultural districts responded most during spring varied from shorter timescales (3 months) in districts in PC1 to longer timescales (5 to 6 months) for those in PC2 (Fig. 9e, f), which also showed greater variability in most months relative to districts from PC1. Greater variability for wheat at the district scale was observed relative to that at the provincial scale. Due to the major number of observations considered, the response to drought in Spain when considering district scale shows more heterogeneity than at provincial scale.
Provincial scale
The results for wheat at the provincial scale (Fig. 7b) showed that the first (PC1) and second (PC2) components explained 51.7 % and 20.8 % of the variance, respectively. The loadings of the first component were higher for the central plateau and the east of Spain. These represent provinces in the Castilla y León and Castilla y La Mancha districts and the provinces of Castellón, Valencia, Alicante, Cantabria, and Huelva, and Sevilla and Almería in Andalucía. In these provinces there was a strong correlation between drought indices and crop yields, especially during spring, with particularly strong correlations in May. In contrast, during winter the correlations were weaker, especially in February. PC2 showed greater spatial heterogeneity, with strong correlations in the east (Zaragoza and Tarragona provinces) and south (Cádiz, Córdoba, Málaga, Granada, and Jaén provinces) of Spain. For this component the temporal response to drought was not as strong as that for PC1, but the maximum correlation was also found during May. The distribution of the maximum loadings showed a dispersed pattern, with PC1 grouping provinces in the central plateau and east of Spain and PC2 grouping those in southern and some northeastern provinces. The averaged temporal response to drought during spring is set at medium timescales (4–7 months). In particular, in May most of the provinces correlated at 5 months (Fig. 9a, b), indicating the importance of climatic conditions during winter and spring to the crop yields obtained. This was also evident for the longer timescales at which most of the provinces correlated during the winter months (11–18 months). It is noteworthy that there was great variability in the temporal response of provinces in PC1 in October, February, March, and April.
3.3.2 Barley
Agricultural district scale
For barley crops (Fig. 8a) both components showed strong correlations (c=0.6–0.9) in most of the agricultural districts. In general, the districts showing the strongest correlations in PC1 and PC2 were those located in Castilla La Mancha and north of Cáceres and Córdoba. Scores for PC1 for barley crops were similar to those for PC1 for wheat during spring and autumn, but the results for PC2 suggest that there was little interannual sensitivity to drought. Most of the correlations for spring indicate that barley responded to drought conditions at the 3–4-month scale, mainly in those districts associated with PC1. Barley yields in districts associated with PC2 were more affected by drought conditions in May at 7–9-month timescales (Fig. 9g, h).
Provincial scale
For barley at the provincial scale (Fig. 8b) we found more variability in the magnitude of correlations. For PC1 (explaining 43.22 % of the variance) strong correlations (r=0.7–0.9) were found for the north and central provinces of Castilla y León, the central provinces of Castilla y la Mancha, and Madrid, Teruel, Valencia, and Castellón. The provinces associated with PC2 (explaining 27.91 % of the variance) were more dispersed than those in PC1, and those showing strong correlations included Zaragoza and Guadalajara in the north, Barcelona and Balearic Islands in the northeast and east, Cáceres in the west, and Cádiz, Córdoba, Málaga, Granada, and Jaén in the south. Provinces showing weaker correlations in PC1 were spread in the northeast (e.g., Navarra, Zaragoza, and Lleida) and west of Spain (e.g., Cáceres and Badajoz). Component scores for PC1 were higher than for PC2, although for wheat crops both showed maximum scores during spring (March) and minimum scores in autumn and winter. More provinces in May were correlated with drought indices at medium drought timescales (4–8 months). During spring, provinces in PC1 showed correlations at longer timescales (7–8 months), while provinces in PC2 showed responses at shorter timescales (3–4 months) (Fig. 9c, d).
3.3.3 General climatological characteristics for the PCA components
Figures S3–S12 show the distribution of climatic characteristics including precipitation, atmospheric evaporative demand (AED), maximum and minimum temperature, and the hydroclimatic balance (precipitation minus AED) at the district scale for the two PCA components. For those districts where wheat was cultivated, no major differences in AED values were found among the components. However, minor differences were observed in precipitation among districts belonging to different PCA components. Those in PC2 had on average less precipitation than those in PC1, especially during autumn, but the difference was not substantial. Greater differences were observed for temperature, with PC1 mainly characterized by districts that had higher maximum temperatures in autumn and spring, and with higher minimum temperatures than the districts in PC2. These results highlight the important role of temperature in the different responses of crop yield to drought and demonstrate that, contrary to what may have been expected, temperature and not precipitation was the main factor constraining crop growth. Thus, changes in extreme temperature levels may influence future crop yields. Districts in PC2 where the barley yield correlated with drought indices were characterized by lower levels of precipitation and higher maximum and minimum temperatures than districts represented by PC1 and by higher AED, especially from April to July. Extremes of temperature also seemed to be the major factor determining barley crop yield.
In this study we investigated the impacts of drought on two rainfed crops in Spain, as measured by a variety of drought indices. We used two datasets of annual crop yields, one from agricultural statistics at the provincial scale spanning the period 1962–2013 and the other a new database at the agricultural district scale from the available parcel data from the national survey covering the period 1993–2015. To identify the best indicator of the impact of drought on yields and their sensitivity to climate, we evaluated the performance of seven drought indices. The selection of drought indices was based on those commonly used to monitoring droughts worldwide, including the Standardized Precipitation Evapotranspiration Index (SPEI), the Standardized Precipitation Index (SPI), the Palmer drought indices (PDSI, Z Index, PHDI, and PMDI), and the Standardized Palmer Drought Index (SPDI).
Independently of the type of crop and the temporal scale considered, our results showed that drought indices calculated at different timescales (the SPEI, the SPI, and the SPDI) had greater capacity to reflect the impacts of climate on crop yields, relative to uni-scalar drought indices. The better performance of these multi-scalar drought indices was mainly because of their flexibility in reflecting the negative impacts of drought over a range of regions with very different characteristics (Vicente-Serrano et al., 2011). This issue is especially relevant in agriculture, as vegetation components do not respond equally to water deficit. The sensitivity and vulnerability of each type of crop to drought and the characteristics of the specific region influence the variability evident in the response to droughts (Contreras and Hunink, 2015). Nonetheless, the results of the assessment of the performance of the Palmer drought indices demonstrated that correlations varied markedly among them, showing some exceptions that may affect their usefulness for monitoring purposes. Overall, our results showed that the PHDI had the weakest relationship to crop yields, followed by the PDSI and the PMDI. The better performance of the PDSI over the PHDI was expected, as the latter was primarily developed for hydrological purposes. Likewise, our results confirmed a better performance of the PMDI (a modified version of the PDSI) over the original PDSI for both crops. Our results are consistent with those of previous studies assessing agricultural drought impacts on crop yields at the global (Vicente-Serrano et al., 2012) and regional (Peña-Gallardo et al., 2018b) scales. The Z Index was the best uni-scalar index among the set analyzed in our study. This index measures short-term moisture conditions, which is a major factor in crop stress (Quiring and Papakryiakou, 2003). Thus, the Z Index was more closely correlated with crop yield than any of the other Palmer indices, indicating its usefulness relative to other Palmer drought indices (Karl, 1986).
Although our findings point to poorer performance of the Palmer drought indices relative to the multi-scalar drought indices, they remain among the most widely accepted indices. Numerous studies have used the Palmer indices in assessments of the use of drought indices for monitoring agricultural drought in various regions worldwide and have reported the superiority of the Z Index (Mavromatis, 2007; Quiring and Papakryiakou, 2003; Sun et al., 2012; Tunalıoğlu and Durdu, 2012; our results confirm its usefulness among the Palmer drought indices.
Nevertheless, it is important to stress that the usefulness of Palmer drought indices is less than drought indices that can be computed at different timescales (Vicente-Serrano et al., 2012). We demonstrated that the three multi-scalar drought indices in our study (SPEI, SPI, and SPDI) were able to detect drought at different timescales, enabling past weather conditions to be related to present conditions in regions characterized by diverse climatic conditions. This is consistent with previous comparative studies in various regions that reported multi-scalar drought indices were effective for monitoring drought impacts on agricultural lands (Blanc, 2012; Kim et al., 2012; Potopová, 2011; Potopová et al., 2016a; Tian et al., 2018; Zhu et al., 2016; Zipper et al., 2016). Although previous studies reported differences among some of the above three indices (e.g., the SPDI and the SPEI; Ghabaei Sough et al., 2018), others have reported similarities in their performance in assessing agricultural drought impacts (Labudová et al., 2016; Peña-Gallardo et al., 2018a). The similar magnitudes of their correlations suggest a similar ability to characterize the impact of drought on crop yields. However, minor differences among these indices suggested the SPEI performed best. First, for both crops slightly stronger correlations were observed with the SPEI, although the SPDI was superior in relation to barley yields at the agricultural district scale. In general, the SPEI was found to be the most suitable drought index in the majority of agricultural districts and provinces, in accordance to Ribeiro et al. (2018) who also found it suitable in Spain for relating drought conditions and yields variability. This suggests that inclusion of AED in the drought index calculation, as occurs in the SPEI, provides greater capacity to predict drought impacts on crop yields compared with the use of precipitation only. Variation in the maximum and minimum temperatures has been found to be the major factor differentiating agricultural districts and provinces with greater sensitivity to drought. Previous studies have stressed the risks associated with an increase in global temperatures, particularly maximum temperatures, and the possible effects on crop yields (Lobell and Field, 2007; Moore and Lobell, 2014). Thus, a ∼5.4 % reduction in grain yields resulting from an increase in average temperature is expected to occur under the current global warming scenario (Asseng et al., 2014; Zhao et al., 2017).
The temporal and spatial effects of drought on yields seem to be very complex, given the observed variability in Spain. In this respect, significant yield effects of drought were found in both datasets. Nevertheless, at the agricultural district scale there was a more evident spatial effect of drought on agricultural yields. This is a key finding for spatial-scale analyses, although the lack of long time series datasets on regional yields is a common constraint.
Drought effects on barley and wheat were similar in space and time, although their sensitivity to drought differed, as shown by differences in the magnitude of the correlations with the drought indices, with wheat yields showing stronger correlations than barley yields. This can be explained by the different physiological characteristics of the two crops, as barley is less dependent on water availability at germination and the grain filling stage than wheat (Mamnouie et al., 2006). Although the transpiration coefficient for barley is higher, this crop is not as subject as wheat to water stress under drought conditions (Fischer et al., 1998). Our results indicate that the temporal responses of barley and wheat to drought conditions were very similar, despite the fact that in Spain barley is typically cultivated later than wheat and in soils with poor moisture retention. Therefore, the phenological characteristics of each type of crop determine how drought affects yields. The results showed that temperature had a more important role than precipitation, suggesting that extreme variations in average temperature conditions during the most sensitive growth stages may have a negative impact on crops.
Overall, crop yields in Spain tend to respond to short drought timescales (1–3 months). However, the sensitivity of crops to drought is greater during spring at medium (4–6 months) timescales. These results are in line with previous studies conducted in the Iberian Peninsula with a similar database at provincial scale that also point to shorter timescales, mostly during spring months (1–6 months) (Ribeiro et al., 2018). This highlights that moisture conditions during winter (the period corresponding to planting and the first growth stages of tillering and stem elongation) are crucial for the successful development of the plants (Çakir, 2004; Moorhead et al., 2015; Wang et al., 2016a, b).
We found a stronger response of crops to climatic conditions in provinces and agricultural districts in the central plateau and an unexpectedly weaker response in southwestern districts. This reflects the inconsistencies reported for the Iberian Peninsula by Páscoa et al. (2016), who argued that spatial differences can be explained mainly by the differing productivities in the various districts; we noted this for the mainly agrarian areas of peninsular Spain (Castilla y León and Castilla La Mancha) and the characteristically heterogeneity of this territory. In the southwestern agricultural areas, where the precipitation rates are lower and temperatures higher, the correlations of yield with drought were weaker. In addition, conclusions achieved by Gouveia et al. (2016) in the same region supported the statement of the strong control of drought on plants activity, especially in semiarid areas. Even though our findings from crop yields suggest the contrary due to the predominance of cereal croplands in north–central regions of Spain, this can be attributed to episodes of abnormal extreme temperatures, such as the very low temperatures in early spring or warmer than usual temperatures in winter. These would affect the expected low evapotranspiration rates during the cold season (Fontana et al., 2015; Kolář et al., 2014). A recent study by Hernandez-Barrera et al. (2016) demonstrated that during autumn and spring, precipitation deficit is the most influential climate factor affecting wheat growth, while an increase in the diurnal temperature range causes a reduction in wheat yield. We found no major differences in precipitation among districts belonging to any of the two defined components but found other differences including in the average maximum and minimum temperatures. These findings highlight the complexity in choosing a useful drought index that encompasses the specificities of each crop, including its sensitivity to moisture and environmental conditions throughout the entire growth cycle, and its seasonality. This underscores the importance of testing and comparing the appropriateness of different drought indices to ensure accurate identification of the multi-temporal impacts of drought on natural systems.
The main findings of this study are summarized below.
-
Assessment of the efficacy of drought indices for monitoring the effect of climate on agricultural yields demonstrated the better performance of multi-scalar indices. The ability to calculate these indices at various timescales enabled drought impacts to be more precisely defined than with the use of indices lacking this characteristic. The multi-scalar drought indices assessed also had fewer computational and data requirements (particularly the SPEI and the SPI), which is a significant consideration when performing analyses based on scarce climate data.
-
From a quantitative evaluation of the relationship of drought indices to crop yields we determined that both of the multi-scalar drought indices tested were useful for the assessment of agricultural drought in Spain. However, the SPEI had slightly better correlations and is the most highly recommended for the purpose.
-
The spatial definition of yield responses to drought was clearer at the district scale, where the finer spatial resolution enabled better definition of the patterns of responses because the climatic variability of each region was better captured at this scale.
-
Barley and wheat yields were more vulnerable to drought during spring, both at short (1–3 months) and medium (4–6 months) timescales. Moisture conditions during late autumn and winter also had an impact on the crop yields.
-
The strongest relationships between drought indices and crop yields were found for the northern and central agricultural districts. The relationships for the southern districts were weaker because of the difficulty of characterizing drought impacts over the diverse and complex territory involved.
-
The climatic and agricultural conditions in Spain are very diverse. The large spatial diversity and the complexity of droughts highlight the need to establish accurate and effective indices to monitor the variable evolution of drought in vulnerable agriculture areas. Climate change is likely to lead to yield losses because of increased drought stress on crops, so in this context effective monitoring tools are of utmost importance. The authors consider that further analysis complementing this study may help to unravel the climate mechanisms that influence the spatiotemporal responses of yields to climate in Spain.
Crop yield data are publicly available and can be accessed for free upon request from the Spanish Ministry of Agriculture, Fisheries and Food. Climatic information used to create the gridded datasets was provided privately by the Spanish Meteorological Agency (AEMET). It can be accessed by users upon request to the agency.
The supplement related to this article is available online at: https://doi.org/10.5194/nhess-19-1215-2019-supplement.
SMVS and SBP designed the research. MPG performed the formal analysis and drafted the paper. SMVS, SB, and FDC contributed to writing the review and editing the paper.
The authors declare that they have no conflict of interest.
This article is part of the special issue “Hydroclimatic extremes and impacts at catchment to regional scales”. It is not associated with a conference.
Authors thank the three anonymous reviewers for their constructive comments in the review of this paper and the anonymous judges that evaluated this study during the OSPP-EGU 2018.
This research has been supported by the European Geosciences Union (OSPP Award 2018 grant), the Spanish Commission of Science and Technology and FEDER (grant nos. PCIN-2015-220 and CGL2014-52135-C03-01), the Water Works 2014 and European Commission (IMDROFLOOD grant), the JPI Climate, funded by FORMAS (SE), DLR (DE), BMWFW (AT), IFD (DK), MINECO (ES), and ANR (FR), with co-funding by the European Union (INDECIS, ERA4CS, and ERA-NET grants, grant no. 690462), and the Spanish Ministry of Economy and Competitiveness (grant no. FPI2015 – BES-2015-072022).
This paper was edited by Chris Reason and reviewed by three anonymous referees.
Alcamo, J., Flörke, M., and Märker, M.: Future long-term changes in global water resources driven by socio-economic and climatic changes, Hydrol. Sci. J., 52, 247–275, https://doi.org/10.1623/hysj.52.2.247, 2007.
Allen, R. G., Pereira, L. S., Raes, D., and Smith, M.: No Title, Crop Evapotranspiration Guidel, Comput. Crop Water Requir., ISBN 92-5-104219-5, 1998.
Alley, W. M.: The Palmer Drought Severity Index: Limitations and Assumptions, J. Clim. Appl. Meteorol., 23, 1100–1109, https://doi.org/10.1175/1520-0450(1984)023<1100:TPDSIL>2.0.CO;2, 1984.
Asseng, S., Foster, I., and Turner, N. C.: The impact of temperature variability on wheat yields, Glob. Chang. Biol., 17, 997–1012, https://doi.org/10.1111/j.1365-2486.2010.02262.x, 2011.
Asseng, S., Ewert, F., Martre, P., Rötter, R. P., Lobell, D. B., Cammarano, D., Kimball, B. A., Ottman, M. J., Wall, G. W., White, J. W., Reynolds, M. P., Alderman, P. D., Prasad, P. V. V., Aggarwal, P. K., Anothai, J., Basso, B., Biernath, C., Challinor, A. J., De Sanctis, G., Doltra, J., Fereres, E., Garcia-Vila, M., Gayler, S., Hoogenboom, G., Hunt, L. A., Izaurralde, R. C., Jabloun, M., Jones, C. D., Kersebaum, K. C., Koehler, A.-K., Müller, C., Naresh Kumar, S., Nendel, C., O'Leary, G., Olesen, J. E., Palosuo, T., Priesack, E., Eyshi Rezaei, E., Ruane, A. C., Semenov, M. A., Shcherbak, I., Stöckle, C., Stratonovitch, P., Streck, T., Supit, I., Tao, F., Thorburn, P. J., Waha, K., Wang, E., Wallach, D., Wolf, J., Zhao, Z., and Zhu, Y.: Rising temperatures reduce global wheat production, Nat. Clim. Change, 5, 143–147, https://doi.org/10.1038/nclimate2470, 2014.
Blanc, E.: The Impact of Climate Change on Crop Yields in Sub-Saharan Africa, Am. J. Clim. Change, 1, 1–13, https://doi.org/10.4236/ajcc.2012.11001, 2012.
Çakir, R.: Effect of water stress at different development stages on vegetative and reproductive growth of corn, F. Crop. Res., 89, 1–16, https://doi.org/10.1016/j.fcr.2004.01.005, 2004.
Cantelaube, P., Terres, J., and Doblas-Reyes, F.: Influence of climate variability on European agriculture-analysis of winter wheat production, Clim. Res., 27, 135–144, https://doi.org/10.3354/cr027135, 2004.
Capa-Morocho, M., Ines, A. V. M., Baethgen, W. E., Rodríguez-Fonseca, B., Han, E., and Ruiz-Ramos, M.: Crop yield outlooks in the Iberian Peninsula: Connecting seasonal climate forecasts with crop simulation models, Agric. Syst., 149, 75–87, https://doi.org/10.1016/J.AGSY.2016.08.008, 2016.
Chen, T., Xia, G., Liu, T., Chen, W., Chi, D., Chen, T., Xia, G., Liu, T., Chen, W., and Chi, D.: Assessment of Drought Impact on Main Cereal Crops Using a Standardized Precipitation Evapotranspiration Index in Liaoning Province, China, Sustainability, 8, 1069, https://doi.org/10.3390/su8101069, 2016.
Contreras, S. and Hunink, J. E.: Drought effects on rainfed agriculture using standardized indices: A case study in SE Spain, in Drought: Research and Science-Policy Interfacing, 2015.
Dai, A.: Drought under global warming: a review, Wiley Interdiscip. Rev. Clim. Change, 2, 45–65, https://doi.org/10.1002/wcc.81, 2011.
Doesken, N. J. and Garen, D.: Drought Monitoring in the Western United States using a Surface Water Supply Index, Preprints, Seventh Conference on Applied Climatology, Salt Lake City, UT, American Meteorological Society, 266–269, 1991.
Domínguez-Castro, F., Ribera, P., García-Herrera, R., Vaquero, J. M., Barriendos, M., Cuadrat, J. M., and Moreno, J. M.: Assessing extreme droughts in Spain during 1750–1850 from rogation ceremonies, Clim. Past, 8, 705–722, https://doi.org/10.5194/cp-8-705-2012, 2012.
Ebrahimpour, M., Rahimi, J., Nikkhah, A., and Bazrafshan, J.: Monitoring Agricultural Drought Using the Standardized Effective Precipitation Index, J. Irrig. Drain. Eng., 141, 04014044, https://doi.org/10.1061/(ASCE)IR.1943-4774.0000771, 2015.
Esfahanian, E., Nejadhashemi, A. P., Abouali, M., Adhikari, U., Zhang, Z., Daneshvar, F., and Herman, M. R.: Development and evaluation of a comprehensive drought index, J. Environ. Manage., 185, 31–43, https://doi.org/10.1016/j.jenvman.2016.10.050, 2017.
European Commission, Agriculture and Rural Development: Commission offers further support to European farmers dealing with droughts, available at:https://ec.europa.eu/info/news/commission-offers-further-support-european-farmers-dealing-droughts-2018-aug-02-0_en, last access: 26 October 2018.
European Environment Agency (EEA): Climate change, impacts and vulnerability in Europe 2016, An indicator-based report, Luxembourg, 424 pp., 2017.
Fink, A. H., Brücher, T., Krüger, A., Leckebusch, G. C., Pinto, J. G., and Ulbrich, U.: The 2003 European summer heatwaves and drought – synoptic diagnosis and impacts, 59, 209–216, https://doi.org/10.1256/wea.73.04, 2004.
Fischer, R. A., Rees, D., Sayre, K. D., Lu, Z.-M., Condon, A. G., and Saavedra, A. L.: Wheat Yield Progress Associated with Higher Stomatal Conductance and Photosynthetic Rate, and Cooler Canopies, Crop. Sci., 38, 1467, https://doi.org/10.2135/cropsci1998.0011183X003800060011x, 1998.
Fontana, G., Toreti, A., Ceglar, A., and De Sanctis, G.: Early heat waves over Italy and their impacts on durum wheat yields, Nat. Hazards Earth Syst. Sci., 15, 1631–1637, https://doi.org/10.5194/nhess-15-1631-2015, 2015.
Forzieri, G., Feyen, L., Russo, S., Vousdoukas, M., Alfieri, L., Outten, S., Migliavacca, M., Bianchi, A., Rojas, R., and Cid, A.: Multi-hazard assessment in Europe under climate change, Clim. Change, 137, 105–119, https://doi.org/10.1007/s10584-016-1661-x, 2016.
García-Herrera, R., Díaz, J., Trigo, R. M., Luterbacher, J., and Fischer, E. M.: A Review of the European Summer Heat Wave of 2003, Crit. Rev. Environ. Sci. Technol., 40, 267–306, https://doi.org/10.1080/10643380802238137, 2010.
García-León, D., Contreras, S., and Hunink, J.: Comparison of meteorological and satellite-based drought indices as yield predictors of Spanish cereals, Agr. Water Manage., 213, 388–396, https://doi.org/10.1016/J.AGWAT.2018.10.030, 2019.
Ghabaei Sough, M., Zare Abyaneh, H., and Mosaedi, A.: Assessing a Multivariate Approach Based on Scalogram Analysis for Agricultural Drought Monitoring, Water Resour. Manag., 32, 3423–3440, https://doi.org/10.1007/s11269-018-1999-0, 2018.
Giorgi, F. and Lionello, P.: Climate change projections for the Mediterranean region, Glob. Planet. Change, 63, 90–104, https://doi.org/10.1016/j.gloplacha.2007.09.005, 2008.
Gouveia, C. M., Trigo, R. M., Beguería, S., and Vicente-Serrano, S. M.: Drought impacts on vegetation activity in the Mediterranean region: An assessment using remote sensing data and multi-scale drought indicators, Glob. Planet. Change, 151, 15–27, https://doi.org/10.1016/j.gloplacha.2016.06.011, 2016.
Guttman, N. B.: Comparing the Palmer Drought Index and The Standardized Precipitation Index, J. Am. Water Resour. Assoc., 34, 113–121, https://doi.org/10.1111/j.1752-1688.1998.tb05964.x, 1998.
Hanjra, M. A. and Qureshi, M. E.: Global water crisis and future food security in an era of climate change, Food Policy, 35, 365–377, https://doi.org/10.1016/J.FOODPOL.2010.05.006, 2010.
Heim, R. R.: A Review of Twentieth-Century Drought Indices Used in the United States, B. Am. Meteorol. Soc., 83, 1149–1165, https://doi.org/10.1175/1520-0477(2002)083<1149:AROTDI>2.3.CO;2, 2002.
Hernandez-Barrera, S., Rodriguez-Puebla, C., and Challinor, A. J.: Effects of diurnal temperature range and drought on wheat yield in Spain, Theor. Appl. Climatol., 129, 503–519, https://doi.org/10.1007/s00704-016-1779-9, 2016.
IPCC 2014: Climate Change 2014: Mitigation of Climate Change, Contribution of Working Group III to the Fifth Assessment Report of the Intergovernmental Panel on Climate Change, edited by: Edenhofer, O., Pichs-Madruga, R., Sokona, Y., Farahani, E., Kadner, S., Seyboth, K., Adler, A., Baum, I., Brunner, S., Eickemeier, P., Kriemann, B., Savolainen, J., Schlömer, S., von Stechow, C., Zwickel, T., and Minx, J. C., Cambridge University Press, Cambridge, United Kingdom and New York, NY, 1454 pp., USA.
Karl, T. R.: The Sensitivity of the Palmer Drought Severity Index and Palmer's Z-Index to their Calibration Coefficients Including Potential Evapotranspiration, J. Clim. Appl. Meteorol., 25, 77–86, https://doi.org/10.1175/1520-0450(1986)025<0077:TSOTPD>2.0.CO;2, 1986.
Kim, B.-S., Sung, J.-H., Kang, H.-S., and Cho, C.-H.: Assessment of Drought Severity over South Korea using Standardized Precipitation Evapo-transpiration Index (SPEI), J. Korea Water Resour. Assoc., 45, 887–900, https://doi.org/10.3741/JKWRA.2012.45.9.887, 2012.
Kolář, P., Trnka, M., Brázdil, R., and Hlavinka, P.: Influence of climatic factors on the low yields of spring barley and winter wheat in Southern Moravia (Czech Republic) during the 1961–2007 period, Theor. Appl. Climatol., 117, 707–721, https://doi.org/10.1007/s00704-013-1037-3, 2014.
Kuhnert, M., Yeluripati, J., Smith, P., Hoffmann, H., van Oijen, M., Constantin, J., Coucheney, E., Dechow, R., Eckersten, H., Gaiser, T., Grosz, B., Haas, E., Kersebaum, K.-C., Kiese, R., Klatt, S., Lewan, E., Nendel, C., Raynal, H., Sosa, C., Specka, X., Teixeira, E., Wang, E., Weihermüller, L., Zhao, G., Zhao, Z., Ogle, S., and Ewert, F.: Impact analysis of climate data aggregation at different spatial scales on simulated net primary productivity for croplands, Eur. J. Agron., 88, 41–52, https://doi.org/10.1016/j.eja.2016.06.005, 2016.
Labudová, L., Labuda, M., and Takáč, J.: Comparison of SPI and SPEI applicability for drought impact assessment on crop production in the Danubian Lowland and the East Slovakian Lowland, Theor. Appl. Climatol., 1–16, https://doi.org/10.1007/s00704-016-1870-2, 2016.
Liu, B., Asseng, S., Müller, C., Ewert, F., Elliott, J., Lobell, D. B., Martre, P., Ruane, A. C., Wallach, D., Jones, J. W., Rosenzweig, C., Aggarwal, P. K., Alderman, P. D., Anothai, J., Basso, B., Biernath, C., Cammarano, D., Challinor, A., Deryng, D., Sanctis, G. D., Doltra, J., Fereres, E., Folberth, C., Garcia-Vila, M., Gayler, S., Hoogenboom, G., Hunt, L. A., Izaurralde, R. C., Jabloun, M., Jones, C. D., Kersebaum, K. C., Kimball, B. A., Koehler, A.-K., Kumar, S. N., Nendel, C., O'Leary, G. J., Olesen, J. E., Ottman, M. J., Palosuo, T., Prasad, P. V. V., Priesack, E., Pugh, T. A. M., Reynolds, M., Rezaei, E. E., Rötter, R. P., Schmid, E., Semenov, M. A., Shcherbak, I., Stehfest, E., Stöckle, C. O., Stratonovitch, P., Streck, T., Supit, I., Tao, F., Thorburn, P., Waha, K., Wall, G. W., Wang, E., White, J. W., Wolf, J., Zhao, Z., and Zhu, Y.: Similar estimates of temperature impacts on global wheat yield by three independent methods, Nat. Clim. Change, 6, 1130–1136, https://doi.org/10.1038/nclimate3115, 2016.
Lobell, D. B. and Asner, G. P.: Climate and management contributions to recent trends in U.S. agricultural yields, Science, 80, 1032, https://doi.org/10.1126/science.1078475, 2003.
Lobell, D. B. and Field, C. B.: Global scale climate-crop yield relationships and the impacts of recent warming, Environ. Res. Lett., 2, 014002, https://doi.org/10.1088/1748-9326/2/1/014002, 2007.
Lobell, D. B., Schlenker, W., and Costa-Roberts, J.: Climate Trends and Global Crop Production Since 1980, Science, 333, 6042, 616–620, 2011.
Loukas, A. and Vasiliades, L.: Probabilistic analysis of drought spatiotemporal characteristics inThessaly region, Greece, Nat. Hazards Earth Syst. Sci., 4, 719–731, https://doi.org/10.5194/nhess-4-719-2004, 2004.
Ma, M., Ren, L., Yuan, F., Jiang, S., Liu, Y., Kong, H., and Gong, L.: A new standardized Palmer drought index for hydro-meteorological use, Hydrol. Process., 28, 5645–5661, https://doi.org/10.1002/hyp.10063, 2014.
Mamnouie, E., Ghazvini, R. F., Esfahany, M., and Nakhoda, B.: The Effects of Water Deficit on Crop Yield and the Physiological Characteristics of Barley (Hordeum vulgare L.) Varieties, available at: http://jast.modares.ac.ir/article-23-5420-en.pdf (last access: 24 November 2018), 2006.
Mavromatis, T.: Drought index evaluation for assessing future wheat production in Greece, Int. J. Climatol., 27, 911–924, https://doi.org/10.1002/joc.1444, 2007.
McEvoy, D. J., Huntington, J. L., Abatzoglou, J. T., Edwards, L. M., McEvoy, D. J., Huntington, J. L., Abatzoglou, J. T., and Edwards, L. M.: An Evaluation of Multiscalar Drought Indices in Nevada and Eastern California, Earth Interact., 16, 1–18, https://doi.org/10.1175/2012EI000447.1, 2012.
Mckee, T. B., Doesken, N. J., and Kleist, J.: The relationshio of drought frequency and duration to time scales, Eighth Conf. Appl. Climatol., 17–22 available at: http://www.droughtmanagement.info/literature/AMS_Relationship_Drought_Frequency_Duration_Time_Scales_1993.pdf (last access: 30 May 2018), 1993.
Meng, Q., Chen, X., Lobell, D. B., Cui, Z., Zhang, Y., Yang, H., and Zhang, F.: Growing sensitivity of maize to water scarcity under climate change, Sci. Rep., 6, 19605, https://doi.org/10.1038/srep19605, 2016.
Moore, F. C. and Lobell, D. B.: Adaptation potential of European agriculture in response to climate change, Nat. Clim. Change, 4, 610–614, https://doi.org/10.1038/nclimate2228, 2014.
Moorhead, J. E., Gowda, P. H., Singh, V. P., Porter, D. O., Marek, T. H., Howell, T. A., and Stewart, B. A.: Identifying and Evaluating a Suitable Index for Agricultural Drought Monitoring in the Texas High Plains, JAWRA J. Am. Water Resour. Assoc., 51, 807–820, https://doi.org/10.1111/jawr.12275, 2015.
Palmer, W. C.: Meteorological Drought, Research Paper, U.S. Dep. Commer. Weather Bur. Washington, DC, Research P., 45, p. 58., 1965.
Páscoa, P., Gouveia, C. M., Russo, A., and Trigo, R. M.: The role of drought on wheat yield interannual variability in the Iberian Peninsula from 1929 to 2012, Int. J. Biometeorol., 61, 1–13, https://doi.org/10.1007/s00484-016-1224-x, 2016.
Peña-Gallardo, M., Vicente-Serrano, S., Domínguez-Castro, F., Quiring, S., Svoboda, M., Beguería, S., and Hannaford, J.: Effectiveness of drought indices in identifying impacts on major crops across the USA, Clim. Res., 75, 221–240, https://doi.org/10.3354/cr01519, 2018a.
Peña-Gallardo, M., Quiring, S., Svoboda, M., Hannaford, J., Tomas-Burguera, M., Martín-Hernández, N., Domínguez-Castro, F. and El Kenawy, A.: Response of crop yield to different time-scales of drought in the United States: Spatio-temporal patterns and climatic and environmental drivers, Agr. Forest Meteorol., 264, 40–55, https://doi.org/10.1016/j.agrformet.2018.09.019, 2018b.
Potopová, V.: Evolution of drought severity and its impact on corn in the Republic of Moldova, Theor. Appl. Climatol., 105, 469–483, https://doi.org/10.1007/s00704-011-0403-2, 2011.
Potopová, V., Štěpánek, P., Možný, M., Türkott, L., and Soukup, J.: Performance of the standardised precipitation evapotranspiration index at various lags for agricultural drought risk assessment in the Czech Republic, Agr. Forest Meteorol., 202, 26–38, https://doi.org/10.1016/J.AGRFORMET.2014.11.022, 2015.
Potopová, V., Štěpánek, P., Farda, A., Türkott, L., Zahradníček, P., and Soukup, J.: Drought stress impact on vegetable crop yields in the Elbe River lowland between 1961 and 2014, Cuad. Investig. Geográfica, 42, 127, https://doi.org/10.18172/cig.2924, 2016a.
Potopová, V., Boroneanţ, C., Boincean, B., and Soukup, J.: Impact of agricultural drought on main crop yields in the Republic of Moldova, Int. J. Climatol., 36, 2063–2082, https://doi.org/10.1002/joc.4481, 2016b.
Quiring, S. M. and Papakryiakou, T. N.: An evaluation of agricultural drought indices for the Canadian prairies, Agr. Forest Meteorol., 118, 49–62, https://doi.org/10.1016/S0168-1923(03)00072-8, 2003.
Ribeiro, A. F. S., Russo, A., Gouveia, C. M., and Páscoa, P.: Modelling drought-related yield losses in Iberia using remote sensing and multiscalar indices, Theor. Appl. Climatol., 136, 203–220. https://doi.org/10.1007/s00704-018-2478-5, 2019.
Richman, M. B.: Rotation of principal components, J. Climatol., 6, 293–335, https://doi.org/10.1002/joc.3370060305, 1986.
Sepulcre-Canto, G., Horion, S., Singleton, A., Carrao, H., and Vogt, J.: Development of a Combined Drought Indicator to detect agricultural drought in Europe, Nat. Hazards Earth Syst. Sci., 12, 3519–3531, https://doi.org/10.5194/nhess-12-3519-2012, 2012.
Spinoni, J., Vogt, J. V., Naumann, G., Barbosa, P., and Dosio, A.: Will drought events become more frequent and severe in Europe?, Int. J. Climatol., 38, 1718–1736, https://doi.org/10.1002/joc.5291, 2018.
Sun, L., Mitchell, S. W., and Davidson, A.: Multiple drought indices for agricultural drought risk assessment on the Canadian prairies, Int. J. Climatol., 32, 1628–1639, https://doi.org/10.1002/joc.2385, 2012.
Tack, J., Barkley, A., and Nalley, L. L.: Effect of warming temperatures on US wheat yields., P. Natl. Acad. Sci. USA, 112, 6931–6936, https://doi.org/10.1073/pnas.1415181112, 2015.
Tian, L., Yuan, S., and Quiring, S. M.: Evaluation of six indices for monitoring agricultural drought in the south-central United States, Agr. Forest Meteorol., 249, 107–119, https://doi.org/10.1016/J.AGRFORMET.2017.11.024, 2018.
Tsakiris, G. and Tigkas, D.: Drought Risk in Agriculture in Mediterranean Regions. Case Study: Eastern Crete, in Methods and Tools for Drought Analysis and Management, Springer Netherlands, Dordrecht, 399–414, 2007.
Tunalıoğlu, R. and Durdu, Ö. F.: Assessment of future olive crop yield by a comparative evaluation of drought indices: a case study in western Turkey, Theor. Appl. Climatol., 108, 397–410, https://doi.org/10.1007/s00704-011-0535-4, 2012.
UPA, Unión de Pequeños Agricultores y Ganaderos: La sequía y sus efectos sobre la agricultura y la ganadería, available at: https://www.upa.es/upa/uControlador/index.php?nodo=1021&hn=2232 (last access: 25 October 2018), 2017.
Vergni, L. and Todisco, F.: Spatio-temporal variability of precipitation, temperature and agricultural drought indices in Central Italy, Agr. Forest Meteorol., 151, 301–313, https://doi.org/10.1016/j.agrformet.2010.11.005, 2011.
Vicente-Serrano, S. M.: Spatial and temporal analysis of droughts in the Iberian Peninsula (1910–2000), Hydrol. Sci. J., 51, 83–97, https://doi.org/10.1623/hysj.51.1.83, 2006.
Vicente-Serrano, S. M., Beguería, S., and López-Moreno, J. I.: A Multiscalar Drought Index Sensitive to Global Warming: The Standardized Precipitation Evapotranspiration Index, J. Climate, 23, 1696–1718, https://doi.org/10.1175/2009JCLI2909.1, 2010.
Vicente-Serrano, S. M., Beguería, S., and López-Moreno, J. I.: Comment on “Characteristics and trends in various forms of the Palmer Drought Severity Index (PDSI) during 1900–2008” by Aiguo Dai, J. Geophys. Res., 116, D19112, https://doi.org/10.1029/2011JD016410, 2011.
Vicente-Serrano, S. M., Beguería, S., Lorenzo-Lacruz, J., Camarero, J. J., López-Moreno, J. I., Azorin-Molina, C., Revuelto, J., Morán-Tejeda, E., Sanchez-Lorenzo, A., Vicente-Serrano, S. M., Beguería, S., Lorenzo-Lacruz, J., Camarero, J. J., López-Moreno, J. I., Azorin-Molina, C., Revuelto, J., Morán-Tejeda, E., and Sanchez-Lorenzo, A.: Performance of Drought Indices for Ecological, Agricultural, and Hydrological Applications, 16, 1–27, https://doi.org/10.1175/2012EI000434.1, 2012.
Vicente-Serrano, S. M., Lopez-Moreno, J.-I., Beguería, S., Lorenzo-Lacruz, J., Sanchez-Lorenzo, A., García-Ruiz, J. M., Azorin-Molina, C., Morán-Tejeda, E., Revuelto, J., Trigo, R., Coelho, F., and Espejo, F.: Evidence of increasing drought severity caused by temperature rise in southern Europe, Environ. Res. Lett, 9, 44001–44009, https://doi.org/10.1088/1748-9326/9/4/044001, 2014.
Vicente-Serrano, S. M., Van der Schrier, G., Beguería, S., Azorin-Molina, C., and Lopez-Moreno, J.-I.: Contribution of precipitation and reference evapotranspiration to drought indices under different climates, J. Hydrol., 526, 42–54, https://doi.org/10.1016/j.jhydrol.2014.11.025, 2015.
Vicente-Serrano, S. M., Camarero, J. J., Olano, J. M., Martín-Hernández, N., Peña-Gallardo, M., Tomás-Burguera, M., Gazol, A., Azorin-Molina, C., Bhuyan, U., and El Kenawy, A.: Diverse relationships between forest growth and the Normalized Difference Vegetation Index at a global scale, Remote Sens. Environ., 187, 14–29, https://doi.org/10.1016/j.rse.2016.10.001, 2016.
Vicente-Serrano, S. M., Tomas-Burguera, M., Beguería, S., Reig, F., Latorre, B., Peña-Gallardo, M., Luna, M. Y., Morata, A., and González-Hidalgo, J. C.: A High Resolution Dataset of Drought Indices for Spain, Data, 2, 22, https://doi.org/10.3390/data2030022, 2017.
Wang, H., Vicente-serrano, S. M., Tao, F., Zhang, X., Wang, P., Zhang, C., Chen, Y., Zhu, D., and Kenawy, A. El: Monitoring winter wheat drought threat in Northern China using multiple climate-based drought indices and soil moisture during 2000–2013, Agr. Forest Meteorol., 228, 1–12, https://doi.org/10.1016/j.agrformet.2016.06.004, 2016a.
Wang, Q., Wu, J., Li, X., Zhou, H., Yang, J., Geng, G., An, X., Liu, L., and Tang, Z.: A comprehensively quantitative method of evaluating the impact of drought on crop yield using daily multi-scale SPEI and crop growth process model, Int. J. Biometeorol., 1–15, https://doi.org/10.1007/s00484-016-1246-4, 2016b.
Webber, H., Ewert, F., Olesen, J. E., Müller, C., Fronzek, S., Ruane, A. C., Bourgault, M., Martre, P., Ababaei, B., Bindi, M., Ferrise, R., Finger, R., Fodor, N., Gabaldón-Leal, C., Gaiser, T., Jabloun, M., Kersebaum, K.-C., Lizaso, J. I., Lorite, I. J., Manceau, L., Moriondo, M., Nendel, C., Rodríguez, A., Ruiz-Ramos, M., Semenov, M. A., Siebert, S., Stella, T., Stratonovitch, P., Trombi, G., and Wallach, D.: Diverging importance of drought stress for maize and winter wheat in Europe, Nat. Commun., 9, 4249, https://doi.org/10.1038/s41467-018-06525-2, 2018.
Wells, N., Goddard, S., Hayes, M. J., Wells, N., Goddard, S., and Hayes, M. J.: A Self-Calibrating Palmer Drought Severity Index, J. Climate, 17, 2335–2351, https://doi.org/10.1175/1520-0442(2004)017<2335:ASPDSI>2.0.CO;2, 2004.
Wigley, T. M. L.: The effect of changing climate on the frequency of absolute extreme events, Clim. Change, 97, 67–76, https://doi.org/10.1007/s10584-009-9654-7, 2009.
WMO: Standardized Precipitation Index User Guide, 24 pp., 2012.
World Bank: World Bank Data, Agriculture, forestry, and fishing, value added (% of GDP) Data, available at: https://data.worldbank.org/indicator/nv.agr.totl.zs (last access: 25 October 2018), 2017.
Zargar, A., Sadiq, R., Naser, B., and Khan, F. I.: A review of drought indices, Environ. Rev., 19, 333–349, https://doi.org/10.1139/a11-013, 2011.
Zhao, C., Liu, B., Piao, S., Wang, X., Lobell, D. B., Huang, Y., Huang, M., Yao, Y., Bassu, S., Ciais, P., Durand, J.-L., Elliott, J., Ewert, F., Janssens, I. A., Li, T., Lin, E., Liu, Q., Martre, P., Müller, C., Peng, S., Peñuelas, J., Ruane, A. C., Wallach, D., Wang, T., Wu, D., Liu, Z., Zhu, Y., Zhu, Z., and Asseng, S.: Temperature increase reduces global yields of major crops in four independent estimates., P. Natl. Acad. Sci. USA, 114, 9326–9331, https://doi.org/10.1073/pnas.1701762114, 2017.
Zhu, Y., Wang, W., Singh, V. P., and Liu, Y.: Combined use of meteorological drought indices at multi-time scales for improving hydrological drought detection, Sci. Total Environ., 571, 1058–1068, https://doi.org/10.1016/J.SCITOTENV.2016.07.096, 2016.
Zipper, S. C., Qiu, J., and Kucharik, C. J.: Drought effects on US maize and soybean production: spatiotemporal patterns and historical changes, Environ. Res. Lett., 11, 094021, https://doi.org/10.1088/1748-9326/11/9/094021, 2016.