the Creative Commons Attribution 4.0 License.
the Creative Commons Attribution 4.0 License.
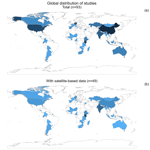
Satellite-based data for agricultural index insurance: a systematic quantitative literature review
Shahbaz Mushtaq
Jarrod Kath
Thong Nguyen-Huy
Louis Reymondin
Index-based insurance (IBI) is an effective tool for managing climate risk and promoting sustainable development. It provides payouts based on a measurable index. Remote sensing data obtained from satellites, planes, UAVs, or drones can be used to design index-based insurance products. However, the extent to which satellite-based data has been used for different crop types and geographical regions has not been systematically explored. To bridge this gap, a systematic quantitative literature review was conducted to examine the use of satellite-based datasets in designing index-based insurance products. The review analyzed 89 global studies on four major types of crops: cereals, pastures and forages, perennial crops, and others (i.e., vegetables, oilseed crops, fruits, nuts, etc.). The analysis revealed a rising interest of developing index-based insurance solutions utilizing satellite-based data, particularly after 2015. Datasets from land surface Earth observation satellites were utilized in 91 % of studies with satellite-based data, outnumbering those from weather satellites. The Normalized Difference Vegetation Index (NDVI) was the most prominent satellite-retrieved vegetation index, featured in 61.2 % of studies utilizing satellite imagery, revealing its effectiveness at designing and developing IBI for various crops. It has also been found that satellite-based vegetation health indices outperform weather indices and reduce basis risk with higher-spatial-resolution data. Most studies have focused on cereal crops, with fewer studies focusing on perennial crops. Countries in Asia and Africa were the most interested regions. However, research has focused on specific countries and has not been adequately spread across different regions, especially developing countries. The review suggests that satellite-based datasets will become increasingly important in designing crop-index-based insurance products. This is due to their potential to reduce basis risk by providing high resolution with adequately long and consistent datasets for data-sparse environments. The review recommends using high-spatial- and high-temporal-resolution satellite datasets to further assess their capability to reduce basis risk.
- Article
(3940 KB) - Full-text XML
-
Supplement
(317 KB) - BibTeX
- EndNote
Agricultural insurance plays a crucial role in managing risks and building resilience against natural disasters. It is a vital part of major global initiatives such as the Sendai Framework for Disaster Risk Reduction, the COP21 Paris Agreement, and the G7 InsuResilience. The goal of the G7 InsuResilience initiative is to offer insurance coverage to an additional 500 million households worldwide by 2025 (Fisher et al., 2019). Index-based insurance is a type of agricultural insurance with payouts generally based on external easy-to-measure indices that are correlated with individual losses or outcomes (Barnett et al., 2008; Barnett and Mahul, 2007; Carter, 2014, 2016; Chantarat et al., 2013; Skees, 2008; Turvey and Mclaurin, 2012). The payouts in index-based insurance are triggered when the actual measurement of the index exceeds or falls below a pre-defined threshold. It can aid smallholder farmers in strengthening their resilience to the impacts of weather extremes, such as drought or flood, while also stimulating investments in productivity in favorable years to optimize profitability (Stoeffler et al., 2022).
Agricultural index-based insurance can be classified into three broad groups: (i) area-yield index insurance, which is based on the average yield of a crop over a specific area; (ii) weather-based index insurance, which is based on a specific weather parameter (such as rainfall, temperature, etc.), measured using data from weather stations, gridded climate information from satellites, or reanalysis climate data; and (iii) vegetation-based or satellite-based index insurance with indices developed using a time series dataset from satellite imagery (Index Insurance Forum, 2024).
Index-based insurance can overcome two significant problems associated with traditional indemnity-based insurance, namely adverse selection and moral hazard, as these indices are beyond the control of any individual (Adeyinka et al., 2022; Mushtaq et al., 2020). Furthermore, index-based insurance is a cost-effective and efficient option for farmers to transfer climate risk as they only need to monitor the index. In contrast, traditional multi-peril options require expensive loss assessment (Carter et al., 2017; De Leeuw et al., 2014; Miranda and Farrin, 2012).
However, the adoption of index-based insurance is often constrained by several factors, such as being restricted to certain measurable perils, insufficient technical capacity, lack of expertise, limited data availability, and basis risk – one of the most commonly cited limitations (Carter et al., 2017; Lichtenberg and Iglesias, 2022; Miranda and Farrin, 2012). Basis risk refers to the difference between an index's estimate of an individual farmer's losses and actual losses, a situation where payouts may occur when no losses happen or vice versa.
There are three common sources of basis risks, including (i) design basis risk, which occurs when the index correlates poorly with losses; (ii) spatial basis risk, which arises when the loss estimated by the index at the measured location is unlikely to occur at the insured farm location, which is geographically far away; and (iii) temporal basis risk, which results from imperfect choices of the time window for the index measurement (Boyd et al., 2019; Dalhaus and Finger, 2016; Dalhaus et al., 2018; Nieto et al., 2010; Woodard and Garcia, 2008). Despite its potential advantages, these limitations can make it challenging for farmers to access and benefit from index insurance (Kath et al., 2018, 2019).
The use of remote sensing (RS) data, acquired from satellites, planes, UAVs, or drones, has been identified as a way to reduce basis risk when developing index-based insurance products. These products require reliable, broad coverage and long historical data archives (De Leeuw et al., 2014). Over the years, researchers have focused on developing highly correlated indices that can accurately predict individual losses at lower costs. In several studies, satellite-based vegetation indices have been used to create effective index-based insurance for crops such as maize, wheat, cotton, and others. This has been successfully implemented in countries such as Canada, India, Mali, Kenya, Kazakhstan, Syria, and Zimbabwe (Bobojonov et al., 2014; Bokusheva et al., 2016; Makaudze and Miranda, 2010; Miranda and Farrin, 2012; Turvey and Mclaurin, 2012).
Normalized difference vegetation indices (NDVIs) extracted from satellite images have also been employed to design index-based livestock insurance in Kenya (Chantarat et al., 2013) and have shown their potential to develop an insurance index for drought risk under limited or even absent weather data (Bobojonov et al., 2014). Brock Porth et al. (2020) developed pasture production indices (PPIs) using multiple data sources. The results showed that these indices had stronger correlations with ground truth forage yield data than weather-based indices that relied on ground weather station data. Satellite-derived vegetation and biophysical parameter indices, such as the NDVI, green NDVI (GNDVI), enhanced vegetation index (EVI), leaf area index (LAI), or fraction of absorbed photosynthetically active radiation (FPAR), used in PPI showed a maximum correlation of 62 % and a minimum correlation of 43.8 %. In some years, the best-performing satellite-based PPIs reached almost 90 % correlation with yield losses. In demonstrating crop-index-based insurance, remote-sensing-based vegetation health indices (e.g., the Vegetation Condition Index (VCI), Vegetation Health Index (VHI), and Temperature Condition Index (TCI)) outperformed weather indices (e.g., temperature, precipitation) (Kölle et al., 2021; Möllmann et al., 2019).
Satellite-based indices are gaining popularity, but their potential applications in cropping systems, especially in developing countries, need to be further investigated. This involves identifying challenges and opportunities, particularly with the availability of new high-resolution satellite-retrieved indices that better address the issue of basis risk. More importantly, it is essential to conduct a comprehensive review to determine the crops and locations where satellite-retrieved data have been utilized as the primary inputs to create index-based insurance solutions. Additionally, it is important to identify the commonly used satellite-based time series datasets in such solutions.
We conducted a systematic quantitative literature review (SQLR) to identify gaps in index-based insurance research and build a comprehensive understanding of the potential use of satellite-based data for index-based insurance. The goal is to examine the current use of satellite-based data in developing crop-specific index-based insurance and identify gaps where future research is most needed. The review also aims to evaluate the potential role of satellite-based data in reducing basis risk and improving index-based insurance solutions. As a global-scale systematic review, we believe the findings will help identify future priorities of study regions and crops to promote crop-specific index insurance solutions.
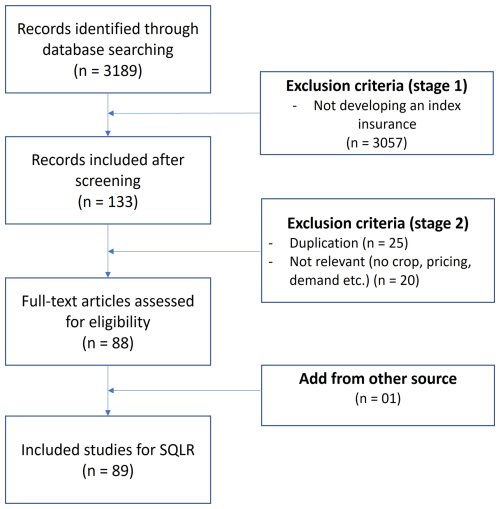
Figure 1PRISMA flowchart showing steps for conducting a systematic quantitative literature review, where n is the number of research papers (adapted from Moher et al., 2009).
The focus of the paper is on four key areas: (1) the locations where index-based insurance studies have been conducted and the crops targeted in those studies, (2) the trends in using satellite-based data to directly develop index-based insurance products, (3) the most commonly used satellite-based datasets for the development of the index, and (4) a discussion of the advantages and disadvantages of using satellite data in index insurance and future research directions.
The SQLR has been carried out following the method by Pickering and Byrne (2014). The keywords used in the review are identified from two fields of study: remote sensing and index insurance. For the field of utilizing remotely sensed satellite-based data, the keywords included “remote sensing” and “satellite-based”, while for the field of index insurance, the keywords included “index-based insurance”, “index insurance”, “parametric insurance”, “weather derivatives”, “agricultural insurance”, “crop insurance”, and “basis risk”. The logical function “OR” was used to link the searched keywords in each field, and the logical function “AND” was used to link keywords from both fields; parentheses were used to separate two groups of keywords corresponding to two fields of study. The searched terms were (“index insurance” OR “index-based insurance” OR “parametric insurance” OR “weather derivatives” OR “crop insurance” OR “agricultural insurance” OR “basis risk” OR “satellite-based insurance”) AND (“remote sensing” OR “satellite-based”).
The keywords were searched over all fields of journal articles, including title, abstract, keywords, and main text. There was no limitation with respect to the publishing year and type of articles. Non-English publications and reviews were excluded. The searched terms were used consistently over multiple databases: ScienceDirect, SpringerLink, Scopus, Taylor & Francis, and MDPI. The results below were as of November 2024.
The studies from searched results were screened in a three-stage process, with the number of papers included and excluded shown in the Preferred Reporting Items for Systematic Reviews and Meta-Analyses (PRISMA) flowchart (Fig. 1).
-
Stage one. Abstracts from the records identified through database search were screened. A paper was excluded if it did not develop any index-based insurance.
-
Stage two. After the first stage of screening, all papers from different databases were combined to scan for duplication and relevant topics. Papers were excluded if (i) there was a duplication from other databases; (ii) the study was on irrelevant topics such as pricing, demand, or willingness to pay for an index insurance product; or (iii) crop insurance was not the focus of the study. The full texts of the remaining papers after exclusion were obtained for further steps.
-
Stage three. Papers that met the searching criteria but were not indexed in the selected database but from other sources were added to the final list to be included in the SQLR.
A structured table with categories and sub-categories was developed to present information from selected articles that were used to complete tasks (1) and (2) above. A subset of papers that directly used satellite-retrieved data to develop the index-based insurance was used to complete tasks (3) and (4) with data on the satellite-based datasets, extracted index, and the spatial and temporal resolution of satellite images (see Table S1 in the Supplement for a complete data table). These review tasks only focused on data from satellites that were used to observe the land surface of the Earth from orbits for the purposes of environmental monitoring, such as vegetation cover, atmospheric content, ocean color, sea state, and ice fields. These review tasks did not include other satellites for communication, navigation, or weather purposes. The indices, especially vegetation indices such as NDVI, EVI, LAI, FAPAR, and so on, were computed from spectral bands collected by sensors on board the satellites.
To identify the types of crops in our studies, we divided them into four main categories based on the FAO crop classification system (FAO, 2011). These categories were cereals (including grain crops like rice, wheat, maize, sorghum, millets, etc.), pasture and forages (crops grown mainly for animal feed), perennial crops (permanent crops as defined by FAO, such as coffee, cocoa, olive, sugar cane, etc.), and others (including root and tuber crops, vegetables, oilseed crops, fruits, nuts, etc.).
The data sources utilized in the studies were classified into three primary groups: (i) non-satellite-based data, which include studies relying on ground measurements from weather station networks, either location based or spatially interpolated into gridded data, or integrated with reanalysis climate data, which combine ground measurements with modeling results; (ii) satellite-based weather data, encompassing studies that utilize at least one dataset from weather satellites, such as CHIRPS and European Space Agency (ESA) CCI climate variable data; and (iii) satellite-based land surface data, involving studies that incorporate at least one dataset from land surface or Earth observation satellites. Studies employing a combination of datasets, including non-satellite, weather satellite, and land surface satellite data, were categorized under all relevant groups.
Based on the data sources, the indices for insurance were categorized into a non-satellite-based index, a satellite-based weather index, a satellite-based land surface index, and a composite index that was developed using multiple sources of non-satellite and satellite-based data.
The satellite-based land surface datasets that were used directly in the studies to develop the index for insurance were classified into medium- and high-resolution groups based on their spatial resolution: medium-spatial-resolution datasets included AVHRR, MODIS, SPOT-VGT and Proba-V, and GRASS LAI, and high-spatial-resolution datasets included Landsat and Sentinel.
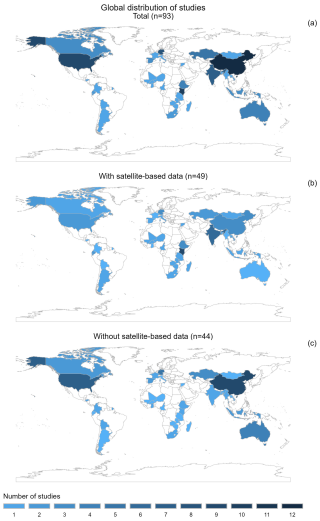
Figure 2The distribution of studies on index-based insurance across the globe from 2007 to November 2024. (a) Total number of studies (n=93), (b) number of studies using satellite data (n=49), (c) number of studies not using satellite data (n=44). Note: there is one study that was conducted in three countries and two studies that were conducted in two countries, so the total number of studies (n=93), counted on the number of countries that the studies were conducted in, is larger than the total papers in the review (n=89).
3.1 African, Asian, and European countries are where index-based insurance is focused
The global distribution (Fig. 2a) reveals a comparable level of interest in Asian and African countries, both with 30 and 27 studies in index-based insurance, respectively. This is followed by Europe and North America, which have 14 and 13 studies. On the other hand, there are fewer studies focused on South America (five studies) and Australia (four studies). Within Southeast Asia, only a handful of studies (five in total) were included in the review, with Indonesia accounting for three and Myanmar and Lao PDR each represented by one study.
There are 34 countries presented within this SQLR; among them, countries that attract the most interest are China (n=12), Kenya (n=10), the United States (n=9), India (n=8), and Germany (n=8). A total of 21 other countries appear only once, while 8 others appear in two to six papers.
Africa is the region with the highest number of studies that used satellite-based data, with 23 out of 27 studies in Africa using satellite-based data (accounting for 85.2 %). South America has the second-highest percentage of satellite data used in the analysis (60.0 % of total studies in the region). On the other hand, Asia, Europe, and North America show a lower share of studies with satellite data, with percentages of 50.0 %, 35.7 %, and 23.1 % of total studies of the region, respectively. In Australia, there is not yet any study using satellite data directly in developing index-based insurance solutions (Fig. 2b).
Among the 49 studies that used satellite-based data, almost half of them (n=23, 46.9 %) were conducted in African countries. Asian countries accounted for 30.6 % (n=15) of the satellite-based studies, while European countries accounted for 10.2 % (n=5). On the other hand, North and South American countries had the lowest number of studies using satellite data, with only three studies (6.1 % of the total satellite-based studies) in each region.
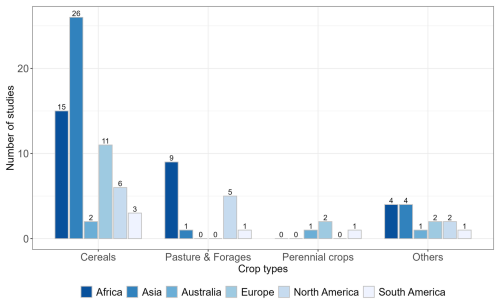
Figure 3Types of insured crops to be studied in different continents. Crops are categorized into four major groups: cereals, pasture and forages, perennial crops, and others (such as root and tuber crops, vegetables, oilseed crops, fruits, nuts, etc.).
Among the 27 countries where satellite-based data were utilized, Kenya stands out with the highest number of studies. A total of 9 out of 10 studies conducted in Kenya made use of at least one satellite-based dataset, representing 33.3 % of all satellite-based studies conducted in Africa. In most of the countries where satellite-based data have been used (19 out of 27, approximately 70.4 %), only a single study was conducted. Of the remaining six countries, half of them (n=3) had two studies each, while the other half (n=3) had three studies in each country.
Among the top three countries with the highest number of studies, China (12 studies) and the United States (9 studies) predominantly relied on non-satellite data. In contrast, Kenya (10 studies) had the majority of its studies incorporating at least one satellite-based dataset.
3.2 Grain cereal crops are the most studied type of index-based insurance
Among the four major insured crop types, cereals have gained the most attention, with almost two-thirds of the total studies (63 out of 97, approximately 64.9 %) focusing on them. Pasture and forages come in second with approximately 16.5 % (16 out of 97) of the studies (Fig. 3). Approximately 14.4 % of the studies (14 out of 97) explored the group of others, including vegetables, oilseed crops, fruits and nuts, root and tuber crops (potato), and other crops (cotton). Perennial crops, however, have the lowest share of 4.1 %, with only four studies conducted on four different crops in three countries. These crops include sugar cane in Australia, coffee in Colombia, and grapes and olives in Spain.
Cereals stand out as the most studied crop across all continents (Fig. 3), representing more than half of studies in each region, except for North America, where they accounted for 46.2 % (6 out of 13 studies). In Asia, cereals are the focus of 82.1 % of studies (23 out of 28), while in Europe, they gained the interest of 77.8 % of studies (14 out of 18). In Africa, Australia, and South America, cereals also constitute a significant portion, ranging from approximately 50.0 % to 53.6 % of total studies in each region.
The studies in pasture and forages are the highest in Africa, representing more than half of studies globally (9 out of 16 studies, approximately 56.3 %). North American countries followed in second place with five studies (31.3 %). In Asia and South America, each region had a single study, while no study developing an index-based insurance for pasture and forages was conducted in Australia and Europe.
On the other hand, perennial crops are the least studied globally, with no study conducted in Asia, Africa, or North America. In Australia and South America, only a single study was conducted in each region, focusing on sugar cane and coffee, respectively, while in Europe, only two studies were conducted, exploring olives and grapes.
The remaining studies on crops categorized under the others group were fairly evenly distributed across continents, with one to four studies per region. This group encompassed a diverse range of crops, including vegetables, oilseed crops, fruits and nuts, root and tuber crops, and crops classified by the FAO under others (such as cotton).
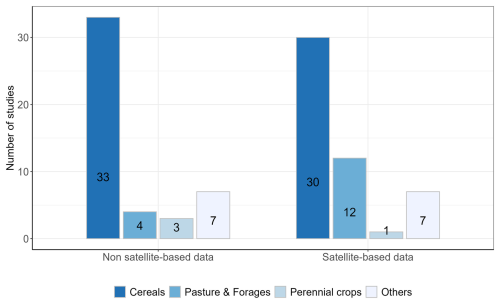
Figure 4Use of satellite data for different types of insured crops. Crops are categorized into four major groups: cereals, pasture and forages, perennial crops, and others (such as vegetables, oilseed crops, fruits, nuts, etc.)
Among the studies that utilized satellite-based data to develop the index for insured crops (Fig. 4), cereals have the highest number of studies (n=30), accounting for 60 % of the total studies using satellite-based data. Pasture and forages show the second-highest share with 24 % (n=12). The others group follows with 14 % (n=7), while only one study on perennial crops utilized satellite-based data. When comparing the use of satellite-based data across crop groups, only pasture and forages show a significantly higher count of studies utilizing satellite-based data (12 studies) compared to those without it (4 studies), with the former being 3 times greater. On the other hand, both the cereals and the others groups display a nearly equal distribution of studies using satellite-based versus non-satellite data.
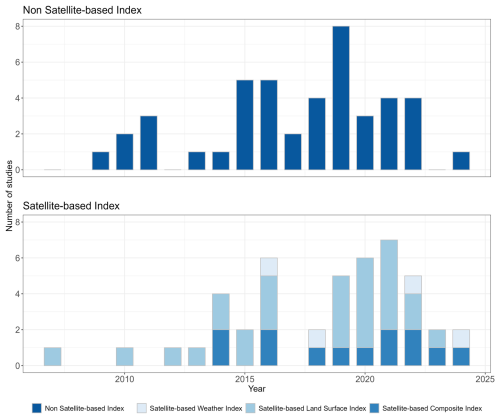
Figure 5Number of studies that developed index-based insurance through time from 2007 to 2024, divided into a non-satellite-based index and a satellite-based index depending on data sources. The satellite-based index includes a satellite-based weather index (data from weather satellites), a satellite-based land surface index (data from land surface Earth observation satellites), and a satellite-based composite index (a combination of multiple sources of non-satellite and satellite-based data).
3.3 The number of studies is increasing over time
The total number of studies over time shows a sharp increase after 2010, particularly after 2015 (Fig. 5). Over the three periods of approximately 5 years each, 2007–2014, 2015–2019, and 2020–2024, the number of studies grew by 62.5 % and 53.85 % in each subsequent period. Notably, the data for the most recent period only extend up to November 2024, meaning the full year's count is not yet complete. A closer year-by-year analysis reveals a sharp rise in the number of studies in 2016 and again between 2019 and 2021, while huge declines were observed in 2017 and 2023.
3.4 The development of non-satellite-based and satellite-based indices is balanced, while satellite-based land surface indices are gaining more interest
The analysis indicates that the total number of studies developing non-satellite-based and satellite-based indices was balanced, with 44 and 49 studies, respectively. The use of non-satellite-based data for index-based insurance increased from the start of the study period to 2015 and has been staying stable since then. On the other hand, the satellite-based indices for insurance have grown quickly since 2014 until 2024, except for a sharp decline in 2017.
Satellite-based land surface datasets were the most commonly used as inputs in 91 % of studies (41 out of 49 studies) with satellite-based data. They were employed either independently to develop land surface indices (29 studies) or in combination with other weather datasets to develop composite indices (12 studies). In contrast, only four studies utilized data from weather satellites. The trend of developing satellite-based land surface indices has also increased rapidly, particularly after 2019, while the development of satellite-based composite indices firstly appeared in 2014 and remained relatively stable with one or two studies each year over the past decade, except for 2015, 2017, and (most recently) 2024.
NDVI is the most prominent vegetation index among satellite-based land surface data, featured in 30 out of 49 studies (61.2 %) utilizing satellite imagery. Other vegetation indices, including EVI, LAI, FAPAR, VCI, VHI, and TCI, have also been applied in studies using satellite-derived datasets, either independently (7 studies) or alongside NDVI (16 studies).
As components in composite indices (12 studies), vegetation indices (10 studies) also stood out as the most popular over soil moisture (2 studies) retrieved from land surface satellite data.
3.5 Biweekly medium-spatial-resolution dataset is preferred, and high-spatial-resolution dataset has been gaining more favor in recent years
During the period covered by this review from 2007–2024, satellite datasets from different sources have been employed in 49 studies, among which data from weather satellites were utilized in 11 studies, and those from land surface or Earth observation satellites were employed in 41 studies.
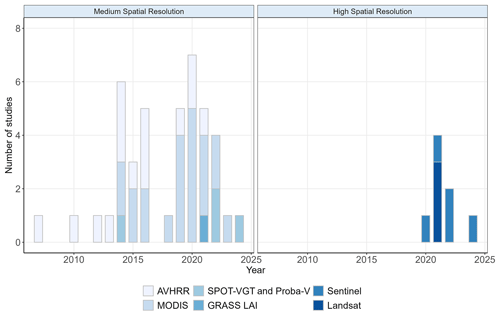
Figure 6The use of medium- and high-spatial-resolution Earth observation satellite (EOS) datasets over time.
Table 1The use of satellite-based datasets as time series with different spatial resolutions. Studies that used satellite data at specific times of the year, rather than as continuous time series, were excluded.
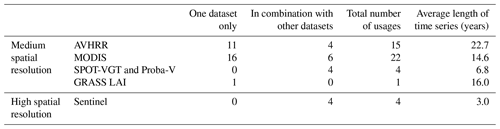
Detailed analysis on datasets from Earth observation satellites (EOSs) reveals the dominance of data at medium spatial resolution, including AVHRR, MODIS, SPOT-VGT/Proba-V, and GRASS LAI. They were accounted for in 84 % of the EOS datasets (42 out of 50) used in developing index-based insurance. Among the medium-spatial-resolution EOSs, AVHRR (with a varied spatial resolution of 1, 4, and 8 km) and MODIS (different products with a spatial resolution of 250 and 500 m) are the most popular datasets that have been used 15 and 22 times, respectively, accounting for 33.3 % and 52.4 % of all medium-spatial-resolution datasets, with a relatively steady increase over time from 2007 to 2022 (Fig. 6 and Table 1). SPOT-VGT and Proba-V have been used four times, while GRASS LAI has been employed once.
Since 2020, high-spatial-resolution satellite datasets like Landsat and Sentinel have been used either independently or paired with medium-spatial-resolution datasets three and five times, respectively. However, among the studies, high-spatial-resolution data were not used as time series four times with Sentinel images, while Landsat images were only selected at specific times of the year, corresponding to key phenological phases of crops or events of interest. Moreover, in each of the studies, depending on the data preparation process, the high-spatial-resolution satellite datasets were kept at their original spatial resolution or resampled to a certain resolution to ensure consistency with other datasets used in the analysis. Two studies utilized high-spatial-resolution satellite data (i.e., Landsat 8 and Sentinel-2) without any spatial aggregation, directly calculating the index for insurance at the plot level. In other studies, high-spatial-resolution datasets were resampled to coarser resolution to match with other datasets in the analysis or aggregated to the insurance unit, whose size varies from farm level to village, division, district, county, zone, agroecological homogeneous zone (AHZ), and country levels.
In terms of temporal dimension, satellite data have been employed in the studies as a time series with varied length and temporal resolution. Studies using medium spatial resolution have much longer time series than those with high spatial resolution (Table 1). AVHRR datasets, available from 1981 until 2019, have been employed with the highest average length of the time series (22.7 years) with a maximum length of 38 years in one study and 32 years in two other studies. MODIS datasets, available from 2000 until present, have also been used with long time series, with an average of 14.6 years. However, the Landsat dataset, a high-spatial-resolution dataset with the longest archive, was not used as a time series. The images were only selected at some dates with specific criteria of cloud cover and time coverage over growing seasons. Sentinel datasets, including Sentinel-1, Sentinel-2, and Sentinel-3, were utilized in a short time series of 3 years.
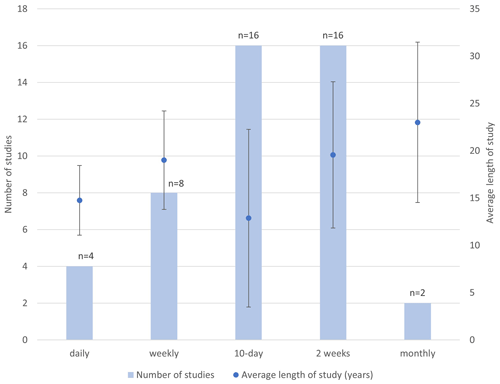
Figure 7Average length of study (years) and temporal resolution of EOS satellite data. The blue columns show the number of studies using datasets with different temporal resolutions, such as daily, weekly, 10 d, biweekly, and monthly. The blue dots and error bars represent the average length and standard deviation of the study period (number of years) of the corresponding temporal resolution. Note: there are six studies that are not included in this figure because they used the datasets containing satellite images at different points in time through the study period without a fixed frequency. The average length among the six studies is 4.67 years.
The various time series datasets were used at different temporal resolutions. Biweekly and 10 d are the most popular temporal resolutions and were employed 16 times each in the studies. Weekly datasets were utilized eight times, while daily and monthly datasets were used the least, with four and two occurrences, respectively (Fig. 7).
The average length of the time series from satellite-based land surface datasets was 15.6 years; however, they were varied at different temporal resolutions (Fig. 7). The monthly time series had the longest average length of 23 years, followed by the biweekly and weekly datasets, with an average length of 19.6 and 19 years, respectively. The 10 d and daily datasets had the shortest average length of 12.9 and 14.8 years, respectively.
However, the temporal resolution of the time series might be different from that of the index insurance, which is based on crop phenology or a general calendar. Therefore, approximately 66 % of the raw time series data were processed to generate statistics such as average, maximum, minimum, anomaly, etc., over a specified period, such as a month or a crop-growing phase or season. In some other cases where the studies employed time series with different temporal resolutions, satellite-derived datasets were resampled to a specific temporal resolution to have consistent datasets for analysis.
The objective of the systematic quantitative literature review (SQLR) was to investigate the usage and scope, especially for smallholder farmers in developing countries, of satellite data in agricultural index-based insurance. The review comprises 89 peer-reviewed journal articles from 34 countries, covering four major types of crops. Cereals were the most frequently studied crop, likely because of their global extent and essential role in food security. Perennial crops were underrepresented, with only four studies, accounting for 4.7 % of all studies.
Academic research in index-based insurance using satellite data is primarily focused on developing countries in Africa and Asia. However, this research is not uniformly distributed, as it tends to concentrate on a few specific countries like Kenya and Ethiopia in Africa and China in Asia. This could be because international donor agencies have historically focused on these countries to manage climate risks, thus leaving a gap in multiple important agricultural production regions such as Southeast Asia, South America, and other countries in Africa.
The use of satellite imagery has been increasing over time, mostly leveraging freely available datasets with long historical archives and medium spatial resolution. Since 2020, there has been an increase in studies using high-spatial-resolution satellite data, which are often combined with medium-spatial-resolution datasets and aggregated to coarser resolution, depending on the desired insurance solutions.
To develop an index insurance solution, the employed datasets have an average length of approximately 15.6 years, which is much longer than that of high-spatial-resolution datasets. Biweekly and 10 d frequencies are the most favored over other temporal resolutions, although satellite images are usually accumulated over a specific period of time or correspond to crop-specific growth phases.
The review suggests that only a few studies have developed index-based insurance for perennial crops. This may be due to the fact that these crops are not included in government subsidy programs, and growers have substantial equity to manage financial risks, making insurance unnecessary. However, as the climate becomes more variable, there could be an opportunity to develop index-based insurance for perennial crops in developing countries, especially where these crops are crucial to economic values and food security. To develop suitable satellite-based insurance solutions that provide targeted coverage, more attention is required to assess the capability of satellite-based data in reducing basis risk at multiple spatial and temporal resolutions.
4.1 African, Asian, and European countries are where index-based insurance is focused
There is a moderate balance between the total number of studies in Africa and Asia, which are prominent regions for developing index-based insurance for crops due to several interconnected factors. Firstly, these regions are highly vulnerable to climate risks, with many countries heavily dependent on rain-fed agriculture; thus, index-based insurance solutions are essential to help mitigate risks from climate variability and extreme weather events such as droughts, floods, and other climatic shocks that significantly affect agricultural productivity. Secondly, large populations in these regions are dependent on agriculture for livelihood. Providing insurance solutions to such smallholder farmers would enable them to safeguard their income and adopt climate-resilient practices. Thirdly, index-based insurance, with its advantages of relying on pre-determined indices, offers a more viable alternative to the traditional indemnity-based insurance, which is often inaccessible or expensive in these regions due to the high administrative costs and the lack of data infrastructure for loss assessment. Last but not least, initiatives and support from international organizations, such as the World Bank, CGIAR, and regional governments, have recognized and implemented various index-based insurance programs in the regions, particularly in Africa, to improve financial solutions and build resilience among smallholder farmers (Barnett et al., 2008; Barnett and Mahul, 2007; Osumba et al., 2023; Skees, 2008).
However, this review also reveals that within the most interested regions in the world, current studies have focused on specific countries such as China in Asia and Ethiopia and Kenya in Africa. Developing countries, especially in Southeast Asia and South America, on the other hand, have not been attracting interest from academia to develop index-based insurance solutions, despite their important role in global agriculture production, especially regarding various cash crops with high economic values, and their vulnerability to climate variability and extreme weather events. Hence, more studies are needed in those underrepresented regions to support smallholder farmers in agricultural risk management.
4.2 Grain cereal crops are the most studied type of index-based insurance
Cereals, the group of annual grain crops, are the most studied globally. This result is well aligned with their vital role in human diets, global food security, and economics. Cereals, including rice, wheat, and maize, are staple crops that form the backbone of diets for a significant portion of the global population. Their reliability and production are crucial for global food security, especially in regions prone to climate risks like Asia and Africa. According to production data from FAOSTAT in 2021, the global harvested areas for cereals reached 739.4×106 ha, with a production of over 3×109 t (FAOSTAT). Moreover, cereals contribute substantially to agricultural GDP in many countries. For smallholder farmers, particularly in developing nations, protecting cereal production is vital for maintaining livelihoods and reducing poverty. Nevertheless, cereals are highly susceptible to climate variability and extreme weather events, such as droughts and floods. These vulnerabilities make them a critical focus for risk management strategies like index-based insurance, which aims to mitigate the impacts of weather-related crop losses. In addition, studies have shown that satellite-based data, such as vegetation health indices, are particularly suited to monitoring cereal crops, providing science-based evidence on the potential to be utilized in the development of index-based insurance solutions (Abdi et al., 2022; Bobojonov et al., 2014; Bokusheva et al., 2016; Nieto et al., 2010; Stojanovski et al., 2015). Hence, grain cereal crops have consistently been at the forefront of agricultural research and development, including efforts to create effective risk transfer solutions.
Furthermore, there was a greater interest in pasture and forages in Africa than in other continents due to their essential role in providing livelihoods to populations in the region. Livestock production constitutes a significant part of Africa's agricultural economy, representing approximately one-third of the global livestock population and contributing about 40 % of the agricultural GDP of African countries (Balehegn et al., 2021). Pasture and forage availability directly affects livestock health and productivity, making it a vital resource for pastoralist and agro-pastoralist communities. Extreme weather events, particularly severe droughts from 2008 to 2011, have led to billions of dollars in losses due to livestock deaths, causing thousands of pastoralists to lose their primary source of livelihood, and have underscored the need for robust risk management solutions. Addressing such urgency for tailored insurance mechanisms to protect pastoralists from climate shocks, several programs from international donors, such as Index-Based Livestock Insurance (IBLI), have been implemented in African countries, especially in Ethiopia and Kenya. Such initiatives utilizing satellite-based data have sparked a growing interest in index-based insurance solutions for livestock in the region.
In contrast, perennial crops have not received as much attention as other crops, possibly due to the complexity of their crop cycles and lack of interest from local governments and international donors. Firstly, perennial crops have an extended lifespan, involving multiple phases, such as establishment, maturation, production, and decline (Cannell, 1985; Júnior et al., 2013; Moriondo et al., 2015; Villalobos et al., 2023). The impacts of environmental factors on yield loss can thus vary substantially across different stages of their prolonged life cycle, making it difficult to establish a clear and consistent correlation between yield losses and stressors. Consequently, this lack of predictability poses significant challenges to the development of index-based insurance solutions for perennial crops, as such solutions rely on robust and reliable correlations to function effectively. Secondly, these crops are often cash crops, which means they may not be eligible for government subsidies and may have enough equity to recover from climate disasters. Any existing studies on perennial crops may only be found in private-sector-specific programs, making them inaccessible to the public and out of the scope of this review.
Despite the limited studies available, a recent study on perennial crops shows promising results. The study developed an index-based insurance using satellite data for non-irrigated olives in Spain. Three satellite indices related to vegetation conditions (VCI, VHI, and TCI), derived from MODIS with meteorological indices as benchmarks, were used to develop different index insurance contracts. The hedging effectiveness result shows that VCI- and VHI-based index insurance contracts outperformed the traditional weather index with only weather variables (Kölle et al., 2021). Moreover, the applications of satellite-based indices for annual crops also show that such data are suitable for developing index insurance (Bokusheva et al., 2016) and even outperformed weather-based IBI options (Brock Porth et al., 2020; Möllmann et al., 2019). As climate change results in increased variability and financial losses, and with the availability of high-resolution satellite data, it will become increasingly important to develop insurance solutions appropriate for perennial crops, in addition to traditional crops.
4.3 Satellite-based datasets show potential to reduce basis risk
4.3.1 High-resolution satellite data can reduce basis risk, but longer time series are needed
The analysis shows that medium-spatial-resolution satellite datasets are favored over high spatial resolution (Fig. 6), which might be due to a lack of evidence on the effectiveness of high spatial resolution in reducing spatial basis risk and adequately long time series. To the best of the authors' knowledge, there are only two studies exploring the potential to reduce basis risk of high-resolution data by comparing hedging effectiveness of insurance contracts developed using data at different spatial resolutions. Kölle et al. (2021) compared MODIS (at two spatial resolutions) and Landsat 5 and Landsat 8 using different models to determine hedging effectiveness. The results show that the hedging effectiveness of the insurance products was positive but varied considerably among indices and study areas. Among them, VHI-, VCI-, and TCI-based insurance solutions showed higher average hedging effectiveness (38 %, 38 %, and 29 %, respectively) than precipitation- and temperature-based insurance contracts (29 % and 16 %, respectively). The study by Eltazarov et al. (2023a) found that comparison to original resolution using downscaled gridded climate data increased the hedging effectiveness for precipitation, temperature, and soil moisture. They also found that the hedging effectiveness varied among indices and regions, between a minimum of 1 % for temperature in Kazakhstan and a maximum of 9 % for precipitation in Mongolia, except for soil moisture in Kazakhstan, where no improvement was shown. However, the two studies only considered wheat. Therefore, further research is required to assess the effectiveness of index-based insurance contracts with distinct datasets for different crops, regions, and spatial resolutions.
The review also shows that high-resolution satellite datasets were not used alone but in combination with other medium-resolution datasets (Table 1). The reason for this might be the effect of cloud cover, resulting in limited high-resolution satellite images available over a specific time period (Kölle et al., 2021). To overcome cloud cover, Kölle et al. (2021) selected only Landsat 5 and Landsat 8 without clouds, and MODIS images were chosen correspondingly. However, this solution limited the study within some selected years as there are trade-offs regarding spatial and temporal resolutions of satellite imagery (Warner et al., 2009). Utilizing a fusion of different datasets from multiple sensors might be a promising solution to gain a high spatial resolution with adequately long historical time series for insurance purposes due to potential effects on insurance premiums (Vrieling et al., 2014). Furthermore, data fusion is not restricted to optical sensors but can also include radar data, which are available throughout any weather condition, either day or night, ensuring continuous and gap-free time series (Pott et al., 2021; Van Tricht et al., 2018). Moreover, commitments from the United States Geological Survey (USGS) and the European Space Agency (ESA) to provide long-term satellite constellations free of charge will maintain the long historical archive of high-resolution satellite data for public access for multiple purposes. This presents an opportunity for further exploration into the use of such data to develop index-based insurance solutions in developing countries.
Furthermore, the development of composite insurance indices has shown a steady increase in recent years (Fig. 5). This trend is likely driven by the promising results of combining multiple variables within a single index. Such composite indices allow multiple variables to complement and enhance one another, resulting in a more comprehensive proxy for crop performance, overcoming the limitations of using a single variable as a proxy to crop yield (Eze et al., 2020; Murthy et al., 2024). Moreover, studies have also highlighted the value of integrating multiple independent satellite-based datasets into index-based insurance solutions. This approach offers a broader representation of the hydrological cycle and improves the ability to capture spatial variations in extreme weather events, especially at regional and global scales. The use of diverse datasets not only enhances the reliability of the indices but also increases their adaptability to different environmental and agricultural contexts, ultimately improving the resilience of insurance systems (Enenkel et al., 2018; Tarnavsky et al., 2018).
Additionally, high-resolution satellite images can also contribute indirectly to reducing spatial basis risk by capturing commodity dissimilarities within a complex and heterogeneous landscape or diversified crop rotations that were put together within an insurance unit, especially at higher levels such as district compared to provincial or regional levels (Eltazarov et al., 2023a, b; Möllmann et al., 2019; Van Tricht et al., 2018). Hence, a precise commodity map, possibly developed from high-resolution satellite images, would be considered in data preparation to ensure consistent information on a specific commodity delineation for model inputs.
4.3.2 Biweekly dataset is the most preferred, and more frequent data might be useful to map crop phenology to adjust time period of index insurance
The results presented in Fig. 7 indicate that satellite-based data with biweekly, weekly, and 10 d frequency resolutions are preferred over daily data. Such products are usually produced by compositing daily data into a lower frequency to remove cloud cover. Additionally, input data were pre-processed to accumulate over a specific time window corresponding to a normal calendar or a crop growth phase, which is usually a few months long. Thus, the temporal resolutions from weekly to biweekly might be adequate. However, high-frequency data can capture temporal fluctuations and outliers that might be reduced after aggregation. Hence, further studies are needed to explore the effectiveness of using data at higher temporal resolution in developing index-based insurance.
To link with spatial resolution, it is necessary to note that all datasets with favored temporal frequency are at medium spatial resolution. Because of cloud cover, high-spatial-resolution satellite data can only be acquired at certain periods. Thus, it is challenging to obtain a consistent, no-gap time series of sufficient length for insurance purposes. The issue of data availability has limited the use of high-spatial-resolution datasets with much shorter lengths of study (Table 1). Again, the fusion of different datasets is proposed to create longer historical time series as inputs to models to better capture temporal variations in developing an index for insurance (Vrieling et al., 2014).
Moreover, studies have shown the potential to reduce temporal basis risk with crop phenological phases or flexible index design at different time windows compared to using fixed-period accumulated data (Conradt et al., 2015; Dalhaus et al., 2018; Leblois et al., 2014). However, traditional crop phenological data collection methods involve field observations, which are costly and site-specific and can lead to spatial basis risk when applied to other locations over long distances. To overcome this data limitation, high-frequency satellite data with the capability to map key crop growth phases, even in near real time, can indirectly contribute to reducing temporal basis risk by identifying crop phenology, especially anomalies of an early or a late start of the growth phases with impacts on crop production (Gao and Zhang, 2021; Murthy et al., 2024). Furthermore, phenological information extracted from satellite-based data can also capture the heterogeneity in the timing of specific crop growth stages, which are used not only to disaggregate predictor values to improve crop yield estimation but also to adjust the time period of index insurance contracts (Afshar et al., 2021; Gao et al., 2017).
4.4 Satellite-data-based index crop insurance solutions are expected to be prevalent in developing countries
While weather-driven index-based insurance is an affordable and transparent method of providing insurance coverage, widespread adoption has been limited due to a lack of available data, particularly in developing countries, and risks associated with the policy. Satellite-based index insurance can help solve these issues by providing datasets with appropriate lengths and spatial resolutions, leading to an increased adoption of index-based insurance in developing countries. Given the availability of satellite data and the fact that many datasets are freely available, satellite-based data will become increasingly important in designing crop insurance, especially in data-sparse environments in developing countries.
The insurance industry is increasingly relying on satellite data to assess and manage risks more effectively. However, to fully leverage satellite datasets, some challenges need to be addressed. One of the most significant challenges is to ensure that the data remain continuously available. Without this, the practical and enhanced use of satellite data will be limited. Therefore, besides making free datasets available, ensuring the data's accessibility will be a crucial task for the industry to overcome.
4.5 Limitations of the study
As already mentioned in the “Materials and methods” section, the review only includes peer-reviewed papers that are accessible through the databases mentioned previously. Materials from gray literature, including working papers, project reports, and so on, are not included, especially those that are under commercial property rights, and are not accessible for research purposes. Given the importance of such materials, the results of this systematic quantitative literature review could be improved with different points of view and discussions from such limited-access literature. Finally, our study was quantitative (e.g., it focused on assessing the number of studies using satellite-based data across different countries and crop types to help identify important knowledge gaps). However, we do acknowledge the need for future qualitative analysis (e.g., using NVivo), which could be useful for identifying important themes and trends in the literature that were not captured with our quantitative approach.
Our analyses have indicated the increasing trend in developing index-based insurance since 2010, especially after 2015 until 2024, with a more than 50 % increase rate for each subsequent 5-year period. Asian and African regions have attracted the highest interest, with the highest number of studies. However, attention has been given to specific countries and not adequately spread across different regions, especially developing countries. Most studies have focused on grain cereal crops, with fewer studies focusing on perennial crops.
Satellite-based data have been employed and have shown their effectiveness in designing index-based insurance for diverse crops. Among them, satellite datasets at a biweekly and 10 d frequency and medium spatial resolution are the most favored. NDVI is the most commonly used satellite-retrieved vegetation index, while some vegetation health indices even outperformed weather variables.
The review suggests that satellite-based datasets will become increasingly important in designing and developing crop-index-based insurance products. This is due to their potential to reduce basis risk by providing high resolution with adequately long and consistent datasets for data-sparse conditions. The review recommends using high-spatial- and high-temporal-resolution satellite-based datasets to further assess their capability to reduce basis risk for index-based insurance solutions.
The code and datasets generated and analyzed during this study are available from the corresponding author upon reasonable request.
The supplement related to this article is available online at https://doi.org/10.5194/nhess-25-913-2025-supplement.
TN, SM, JK, TNH, and LR: conceptualization; TN curated the data, designed the methodology, conducted the formal analysis, visualized the data, and wrote the original draft; TN, SM, JK, TNH, and LR: writing – review and editing; SM: funding acquisition.
The contact author has declared that none of the authors has any competing interests.
Publisher's note: Copernicus Publications remains neutral with regard to jurisdictional claims made in the text, published maps, institutional affiliations, or any other geographical representation in this paper. While Copernicus Publications makes every effort to include appropriate place names, the final responsibility lies with the authors.
The authors are thankful for the support from the International Climate Initiative (IKI) of the German Federal Ministry for Economic Affairs and Climate Action (BMWK).
This research has been supported by the International Climate Initiative (IKI) of the German Federal Ministry for Economic Affairs and Climate Action (BMWK) (grant no. 16519/2018-1.4 CLW/AGM).
This paper was edited by Philip Ward and reviewed by two anonymous referees.
Abdi, M. J., Raffar, N., Zulkafli, Z., Nurulhuda, K., Rehan, B. M., Muharam, F. M., Khosim, N. A., and Tangang, F.: Index-based insurance and hydroclimatic risk management in agriculture: A systematic review of index selection and yield-index modelling methods, Int. J. Disast. Risk Re., 67, 102653, https://doi.org/10.1016/j.ijdrr.2021.102653, 2022.
Adeyinka, A. A., Kath, J., Nguyen-Huy, T., Mushtaq, S., Souvignet, M., Range, M., and Barratt, J.: Global disparities in agricultural climate index-based insurance research, Climate Risk Management, 35, 100394, https://doi.org/10.1016/j.crm.2022.100394, 2022.
Afshar, M. H., Foster, T., Higginbottom, T. P., Parkes, B., Hufkens, K., Mansabdar, S., Ceballos, F., and Kramer, B.: Improving the Performance of Index Insurance Using Crop Models and Phenological Monitoring, Remote Sens.-Basel, 13, 924, https://doi.org/10.3390/rs13050924, 2021.
Balehegn, M., Kebreab, E., Tolera, A., Hunt, S., Erickson, P., Crane, T. A., and Adesogan, A. T.: Livestock sustainability research in Africa with a focus on the environment, Animal Frontiers, 11, 47–56, https://doi.org/10.1093/af/vfab034, 2021.
Barnett, B. J. and Mahul, O.: Weather Index Insurance for Agriculture and Rural Areas in Lower-Income Countries, Am. J. Agr. Econ., 89, 1241–1247, https://doi.org/10.1111/j.1467-8276.2007.01091.x, 2007.
Barnett, B. J., Barrett, C. B., and Skees, J. R.: Poverty Traps and Index-Based Risk Transfer Products, World Dev., 36, 1766–1785, https://doi.org/10.1016/j.worlddev.2007.10.016, 2008.
Bobojonov, I., Aw-Hassan, A., and Sommer, R.: Index-based insurance for climate risk management and rural development in Syria, Clim. Dev., 6, 166–178, https://doi.org/10.1080/17565529.2013.844676, 2014.
Bokusheva, R., Kogan, F., Vitkovskaya, I., Conradt, S., and Batyrbayeva, M.: Satellite-based vegetation health indices as a criteria for insuring against drought-related yield losses, Agr. Forest Meteorol., 220, 200–206, https://doi.org/10.1016/j.agrformet.2015.12.066, 2016.
Boyd, M., Porth, B., Porth, L., and Turenne, D.: The Impact of Spatial Interpolation Techniques on Spatial Basis Risk for Weather Insurance: An Application to Forage Crops, North American Actuarial Journal, 23, 412–433, https://doi.org/10.1080/10920277.2019.1566074, 2019.
Brock Porth, C., Porth, L., Zhu, W., Boyd, M., Tan, K. S., and Liu, K.: Remote Sensing Applications for Insurance: A Predictive Model for Pasture Yield in the Presence of Systemic Weather, North American Actuarial Journal, 24, 333–354, https://doi.org/10.1080/10920277.2020.1717345, 2020.
Cannell, M. G. R.: Physiology of the Coffee Crop, in: Coffee, edited by: Clifford, M. N. and Willson, K. C., Springer US, Boston, MA, https://doi.org/10.1007/978-1-4615-6657-1_5, 108–134, 1985.
Carter, M., De Janvry, A., Sadoulet, E., and Sarris, A.: Index Insurance for Developing Country Agriculture: A Reassessment, Annu. Rev. Resour. Econ., 9, 421–438, https://doi.org/10.1146/annurev-resource-100516-053352, 2017.
Carter, M. R.: Index-based weather insurance for developing countries: a review of evidence and a set of propositions for up-scaling, Working Paper Development Policies, Vol 111, Fondation pour les études et recherches sur le développement international, https://ferdi.fr/dl/df-kLB5hYBaUiR2Vysm2cH5DfCP/ferdi-p111-index-based-weather-insurance-for-developing (last access: 20 February 2025), 2014.
Carter, M. R., Cheng, L., and Sarris, A.: Where and how index insurance can boost the adoption of improved agricultural technologies, J. Dev. Econ., 118, 59–71, https://doi.org/10.1016/j.jdeveco.2015.08.008, 2016.
Chantarat, S., Mude, A. G., Barrett, C. B., and Carter, M. R.: Designing Index-Based Livestock Insurance for Managing Asset Risk in Northern Kenya, J. Risk Insur., 80, 205–237, https://doi.org/10.1111/j.1539-6975.2012.01463.x, 2013.
Conradt, S., Finger, R., and Spörri, M.: Flexible weather index-based insurance design, Climate Risk Management, 10, 106–117, https://doi.org/10.1016/j.crm.2015.06.003, 2015.
Dalhaus, T. and Finger, R.: Can Gridded Precipitation Data and Phenological Observations Reduce Basis Risk of Weather Index–Based Insurance?, Weather Clim. Soc., 8, 409–419, https://doi.org/10.1175/WCAS-D-16-0020.1, 2016.
Dalhaus, T., Musshoff, O., and Finger, R.: Phenology Information Contributes to Reduce Temporal Basis Risk in Agricultural Weather Index Insurance, Sci. Rep., 8, 46, https://doi.org/10.1038/s41598-017-18656-5, 2018.
De Leeuw, J., Vrieling, A., Shee, A., Atzberger, C., Hadgu, K., Biradar, C., Keah, H., and Turvey, C.: The Potential and Uptake of Remote Sensing in Insurance: A Review, Remote Sens.-Basel, 6, 10888–10912, https://doi.org/10.3390/rs61110888, 2014.
Eltazarov, S., Bobojonov, I., Kuhn, L., and Glauben, T.: Improving risk reduction potential of weather index insurance by spatially downscaling gridded climate data – a machine learning approach, Big Earth Data, 7, 1–24, https://doi.org/10.1080/20964471.2023.2196830, 2023a.
Eltazarov, S., Bobojonov, I., Kuhn, L., and Glauben, T.: The role of crop classification in detecting wheat yield variation for index-based agricultural insurance in arid and semiarid environments, Environmental and Sustainability Indicators, 18, 100250, https://doi.org/10.1016/j.indic.2023.100250, 2023b.
Enenkel, M., Farah, C., Hain, C., White, A., Anderson, M., You, L., Wagner, W., and Osgood, D.: What rainfall does not tell us-enhancing financial instruments with satellite-derived soil moisture and evaporative stress, Remote Sens.-Basel, 10, 1819, https://doi.org/10.3390/rs10111819, 2018.
FAO: Crops statistics – Concepts, definitions and classifications, FAO; Rome, Italy; 15 pages; https://openknowledge.fao.org/handle/20.500.14283/cb2462en (last access: 20 February 2025), 2011.
Eze, E., Girma, A., Zenebe, A. A., and Zenebe, G.: Feasible crop insurance indexes for drought risk management in Northern Ethiopia, Int. J. Disast. Risk Re., 47, 101544, https://doi.org/10.1016/j.ijdrr.2020.101544, 2020.
FAOSTAT: Food and agriculture data, FAO, https://www.fao.org/faostat/en/#data (last access: 8 February 2024), 2024.
Fisher, E., Hellin, J., Greatrex, H., and Jensen, N.: Index insurance and climate risk management: Addressing social equity, Dev. Policy Rev., 37, 581–602, https://doi.org/10.1111/dpr.12387, 2019.
Gao, F. and Zhang, X.: Mapping Crop Phenology in Near Real-Time Using Satellite Remote Sensing: Challenges and Opportunities, J. Remote Sens., 2021, 8379391, https://doi.org/10.34133/2021/8379391, 2021.
Gao, F., Anderson, M. C., Zhang, X., Yang, Z., Alfieri, J. G., Kustas, W. P., Mueller, R., Johnson, D. M., and Prueger, J. H.: Toward mapping crop progress at field scales through fusion of Landsat and MODIS imagery, Remote Sens. Environ., 188, 9–25, https://doi.org/10.1016/j.rse.2016.11.004, 2017.
Index Insurance Forum: What are the difference types of ”crop” index insurance?, Global Index Insurance Facility, https://www.indexinsuranceforum.org/faq/what-are-different-types-crop-index-insurance (last access: 8 February 2024), 2024.
Júnior, A. F. C., Júnior, O. A. D. C., Martins, É. D. S., and Guerra, A. F.: PHENOLOGICAL CHARACTERIZATION OF COFFEE CROP (Coffea arabica L.) FROM MODIS TIME SERIES, Rev. Bras. Geof., 31, 569, https://doi.org/10.22564/rbgf.v31i4.338, 2013.
Kath, J., Mushtaq, S., Henry, R., Adeyinka, A., and Stone, R.: Index insurance benefits agricultural producers exposed to excessive rainfall risk, Weather and Climate Extremes, 22, 1–9, https://doi.org/10.1016/j.wace.2018.10.003, 2018.
Kath, J., Mushtaq, S., Henry, R., Adeyinka, A. A., Stone, R., Marcussen, T., and Kouadio, L.: Spatial variability in regional scale drought index insurance viability across Australia's wheat growing regions, Climate Risk Management, 24, 13–29, https://doi.org/10.1016/j.crm.2019.04.002, 2019.
Kölle, W., Martínez Salgueiro, A., Buchholz, M., and Musshoff, O.: Can satellite-based weather index insurance improve the hedging of yield risk of perennial non-irrigated olive trees in Spain?, Aust. J. Agr. Resour. Ec., 65, 66–93, https://doi.org/10.1111/1467-8489.12403, 2021.
Leblois, A., Quirion, P., Alhassane, A., and Traoré, S.: Weather Index Drought Insurance: An Ex Ante Evaluation for Millet Growers in Niger, Environ. Resour. Econ., 57, 527–551, https://doi.org/10.1007/s10640-013-9641-3, 2014.
Lichtenberg, E. and Iglesias, E.: Index insurance and basis risk: A reconsideration, J. Dev. Econ., 158, 102883, https://doi.org/10.1016/j.jdeveco.2022.102883, 2022.
Makaudze, E. M. and Miranda, M. J.: Catastrophic drought insurance based on the remotely sensed normalised difference vegetation index for smallholder farmers in Zimbabwe, Agrekon, 49, 418–432, https://doi.org/10.1080/03031853.2010.526690, 2010.
Miranda, M. J. and Farrin, K.: Index Insurance for Developing Countries, Appl. Econ. Perspect. P., 34, 391–427, https://doi.org/10.1093/aepp/pps031, 2012.
Moher, D., Liberati, A., Tetzlaff, J., Altman, D. G., and The PRISMA Group: Preferred Reporting Items for Systematic Reviews and Meta-Analyses: The PRISMA Statement, PLoS Med., 6, e1000097, https://doi.org/10.1371/journal.pmed.1000097, 2009.
Möllmann, J., Buchholz, M., and Musshoff, O.: Comparing the Hedging Effectiveness of Weather Derivatives Based on Remotely Sensed Vegetation Health Indices and Meteorological Indices, Weather Clim. Soc., 11, 33–48, https://doi.org/10.1175/WCAS-D-17-0127.1, 2019.
Moriondo, M., Ferrise, R., Trombi, G., Brilli, L., Dibari, C., and Bindi, M.: Modelling olive trees and grapevines in a changing climate, Environ. Model. Softw., 72, 387–401, https://doi.org/10.1016/j.envsoft.2014.12.016, 2015.
Murthy, C. S., Choudhary, K. K., Pandey, V., Srikanth, P., Ramasubramanian, S., Kumar, G. S., Poddar, M. K., Milesi, C., and Nemani, R.: Transformative crop insurance solution with big earth data: Implementation for potato in India, Climate Risk Management, 45, 100622, https://doi.org/10.1016/j.crm.2024.100622, 2024.
Mushtaq, S., Kath, J., Stone, R., Henry, R., Läderach, P., Reardon-Smith, K., Cobon, D., Marcussen, T., Cliffe, N., Kristiansen, P., and Pischke, F.: Creating positive synergies between risk management and transfer to accelerate food system climate resilience, Climatic Change, 161, 465–478, https://doi.org/10.1007/s10584-020-02679-5, 2020.
Nieto, J. D., Cook, S. E., LäDERACH, P., Fisher, M. J., and Jones, P. G.: Rainfall index insurance to help smallholder farmers manage drought risk, Clim. Dev., 2, 233–247, https://doi.org/10.3763/cdev.2010.0050, 2010.
Osumba, J., Recha, J. W. M., and Demissie, T.: State of Index-Based Crop Insurance Interventions for Smallholder Farmers and Agribusinesses in East Africa. AICCRA Status Report. Accelerating Impacts of CGIAR Climate Research in Africa (AICCRA), https://hdl.handle.net/10568/131162 (last access: 20 February 2025), 2023.
Pickering, C. and Byrne, J.: The benefits of publishing systematic quantitative literature reviews for PhD candidates and other early-career researchers, High. Educ. Res. Dev., 33, 534–548, https://doi.org/10.1080/07294360.2013.841651, 2014.
Pott, L. P., Amado, T. J. C., Schwalbert, R. A., Corassa, G. M., and Ciampitti, I. A.: Satellite-based data fusion crop type classification and mapping in Rio Grande do Sul, Brazil, ISPRS J. Photogramm., 176, 196–210, https://doi.org/10.1016/j.isprsjprs.2021.04.015, 2021.
Skees, J. R.: Challenges for use of index-based weather insurance in lower income countries, Agricultural Finance Review, 68, 197–217, https://doi.org/10.1108/00214660880001226, 2008.
Stoeffler, Q., Carter, M., Guirkinger, C., and Gelade, W.: The Spillover Impact of Index Insurance on Agricultural Investment by Cotton Farmers in Burkina Faso, World Bank Econ. Rev., 36, 114–140, https://doi.org/10.1093/wber/lhab011, 2022.
Stojanovski, P., Dong, W., Wang, M., Ye, T., Li, S., and Mortgat, C. P.: Agricultural Risk Modeling Challenges in China: Probabilistic Modeling of Rice Losses in Hunan Province, Int. J. Disast. Risk Sc., 6, 335–346, https://doi.org/10.1007/s13753-015-0071-4, 2015.
Tarnavsky, E., Chavez, E., and Boogaard, H.: Agro-meteorological risks to maize production in Tanzania: Sensitivity of an adapted Water Requirements Satisfaction Index (WRSI) model to rainfall, Int. J. Appl. Earth Obs., 73, 77–87, https://doi.org/10.1016/j.jag.2018.04.008, 2018.
Turvey, C. G. and Mclaurin, M. K.: Applicability of the Normalized Difference Vegetation Index (NDVI) in Index-Based Crop Insurance Design, Weather Clim. Soc., 4, 271–284, https://doi.org/10.1175/WCAS-D-11-00059.1, 2012.
Van Tricht, K., Gobin, A., Gilliams, S., and Piccard, I.: Synergistic Use of Radar Sentinel-1 and Optical Sentinel-2 Imagery for Crop Mapping: A Case Study for Belgium, Remote Sens.-Basel, 10, 1642, https://doi.org/10.3390/rs10101642, 2018.
Villalobos, F. J., López-Bernal, Á., García-Tejera, O., and Testi, L.: Is olive crop modelling ready to assess the impacts of global change?, Front. Plant Sci., 14, 1249793, https://doi.org/10.3389/fpls.2023.1249793, 2023.
Vrieling, A., Meroni, M., Shee, A., Mude, A. G., Woodard, J., de Bie, C. A. J. M. (Kees), and Rembold, F.: Historical extension of operational NDVI products for livestock insurance in Kenya, Int. J. Appl. Earth Obs.,, 28, 238–251, https://doi.org/10.1016/j.jag.2013.12.010, 2014.
Warner, T. A., Nellis, M. D., and Foody, G. M.: The SAGE Handbook of Remote Sensing, SAGE Publications Ltd, London, https://doi.org/10.4135/9780857021052, 2009.
Woodard, J. D. and Garcia, P.: Basis risk and weather hedging effectiveness, Agricultural Finance Review, 68, 99–117, https://doi.org/10.1108/00214660880001221, 2008.