the Creative Commons Attribution 4.0 License.
the Creative Commons Attribution 4.0 License.
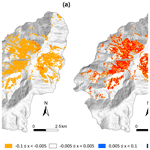
Modelling landslide hazards under global changes: the case of a Pyrenean valley
Séverine Bernardie
Rosalie Vandromme
Yannick Thiery
Thomas Houet
Marine Grémont
Florian Masson
Gilles Grandjean
Isabelle Bouroullec
Several studies have shown that global changes have important impacts in mountainous areas, since they affect natural hazards induced by hydrometeorological events such as landslides. The present study evaluates, through an innovative method, the influence of both vegetation cover and climate change on landslide hazards in a Pyrenean valley from the present to 2100.
We first focused on assessing future land use and land cover changes through the construction of four prospective socioeconomic scenarios and their projection to 2040 and 2100. Secondly, climate change parameters were used to extract the water saturation of the uppermost layers, according to two greenhouse gas emission scenarios. The impacts of land cover and climate change based on these scenarios were then used to modulate the hydromechanical model to compute the factor of safety (FoS) and the hazard levels over the considered area.
The results demonstrate the influence of land cover on slope stability through the presence and type of forest. The resulting changes are statistically significant but small and dependent on future land cover linked to the socioeconomic scenarios. In particular, a reduction in human activity results in an increase in slope stability; in contrast, an increase in anthropic activity leads to an opposite evolution in the region, with some reduction in slope stability.
Climate change may also have a significant impact in some areas because of the increase in the soil water content; the results indicate a reduction in the FoS in a large part of the study area, depending on the landslide type considered. Therefore, even if future forest growth leads to slope stabilization, the evolution of the groundwater conditions will lead to destabilization. The increasing rate of areas prone to landslides is higher for the shallow landslide type than for the deep landslide type. Interestingly, the evolution of extreme events is related to the frequency of the highest water filling ratio. The results indicate that the occurrences of landslide hazards in the near future (2021–2050 period, scenario RCP8.5) and far future (2071–2100 period, scenario RCP8.5) are expected to increase by factors of 1.5 and 4, respectively.
- Article
(19371 KB) - Full-text XML
- BibTeX
- EndNote
Global changes have impacts worldwide, but their effects are even more exacerbated in particularly vulnerable areas, such as mountainous regions. In these areas, a range of socioeconomic sectors (e.g. tourism, forest production, agro-pastoralism and natural resources) have experienced considerable changes in the last 2 centuries, resulting in pressures on natural resources and traditions that are imposed by increasingly industrialized societies (Huber et al., 2005). Some mountainous regions have been extensively transformed, converting them from inaccessible and relatively poor areas into attractive destinations for the wealthy. In other cases, outmigration and an ageing population have led to economic declines in the agro-pastoral and forestry sectors.
Climate change affected and will affect mountainous regions. An increase in the temperature in these areas has already been observed that is comparable to what has been observed in lowland regions (Kohler and Maselli, 2009). Some boundaries, such as the tree line, the limit of snow and the limit indicating the presence of glaciers and permafrost, start to be modified. The hypothesis of global warming has now been validated by various studies (IPCC, 2007, 2014). The climate evolution have significant impacts of natural hazards since most of them are induced by hydrometeorological events, such as floods and different types of landslide. For example, the IPCC (Intergovernmental Panel on Climate Change) notes that “There is high confidence that changes in heatwaves, glacial retreat, and/or permafrost degradation will affect slope instabilities in high mountains, and medium confidence that temperature-related changes will influence bedrock stability. There is also high confidence that changes in heavy precipitation will affect landslides in some regions” (IPCC, 2014). In the Pyrenees, an OPCC-CTP report (2018) mentioned that “It is highly probable that the Pyrenees will see an increase in extreme weather phenomena”. This may lead to more frequent floods, landslides, rockfalls and avalanches. Nevertheless, the quantification of the impacts of climate change on natural hazards related to hydro-geohazards remains a complex issue. Among hydro-geohazards, landslides are very sensitive to the hydrometeorological conditions present in mountainous regions and are complex. Gariano and Guzzetti (2016) indicate that several landslide-triggering parameters may be affected by climate change. Indeed, climate change occurring in mountains may imply future modifications in temperature and precipitation patterns; this may lead to changes in the balance between snow, ice and rainfall, which ultimately will result in changes in precipitation quantity and seasonality. Landslides are also sensitive to the presence of water within the layers that are susceptible to movement. As future trends in the climate may imply some modifications of meteorological parameters such as precipitation and temperature, the resulting underground water table level may evolve.
The sensitivity of landslides to climate change may depend on their type, especially on the size and depth of the landslide (Crozier, 2010). As shallow landslides are generally governed by shorter-duration rainfall, they may be more influenced by the evolution of parameters in the short-term parameter evolution, such as changes in the intensity of rainfall. In contrast, deep-seated landslides may be affected by long-term hydrometeorological evolutions, such as changes in the monthly rainfall, seasonal snow or groundwater. Several studies are related to the analysis of the impact of climate change on landslide occurrence. The review conducted on that topic, described in Gariano and Guzzetti (2016), indicates the increasing number of studies devoted to this issue. The future evolution of hydrometeorological conditions implies that there will be some modification in the frequency of landslide occurrence (Turkington et al., 2016; Gariano et al., 2017; Rianna et al., 2017; Robinson et al., 2017; Alvioli et al., 2018; Peres and Cancelliere, 2018; Sangelantoni et al., 2018) and also in their seasonal temporality (Stoffel et al., 2014); these factors will evolve differently depending on the region of interest.
Landslide hazards may also be affected by global change with the evolution of the socioeconomic contexts, which may have some impacts on landslides through the evolution of land cover, as demonstrated in several studies (Vanacker et al., 2003; Van Beek and Van Ash, 2004; Promper et al., 2014; Reichenbach et al., 2014; Galve et al., 2015; Persichillo et al., 2017; Pisano et al., 2017; Gariano et al., 2018). In particular, the abandonment of agricultural practice and land management result in an increasing number of shallow landslides (Persichillo et al., 2017; Pisano et al., 2017; Gariano et al., 2018).
Indeed, vegetation influences deep-seated landslides very little, but this influence exists for shallow landslides and remains difficult to address. At the same time, the vegetation cover increases the ground weight and thus tends to initiate ruptures, but it also increases the shear strength and modifies the soil moisture content through evapotranspiration and runoff processes. The relative importance of these two contrary effects varies according to the localization of the vegetation cover on the slope. The stability is increased if the vegetation is present on the toe of the slope (Genet et al., 2010; Ji et al., 2012), but this stabilizing effect is reduced if the vegetation is located on the upper part of the slope (Norris, 2008; Genet et al., 2010). As a result, the densification of forest areas may improve the slope stability (Reichenbach et al., 2014).
Many studies have been conducted based on historical observations; these analyses, which use empirical or numerical approaches, permit the analysis of the evolution of different features of landslides (frequency, type, evolution of the mechanisms, etc.). The quantification of future scenarios is a key challenge in estimating the future trends in landslide activity with the future evolution of climate change, and estimating the uncertainties is necessary in these approaches. For this reason, modelling constitutes an approach that is being increasingly developed in two different ways, depending on the size of the investigated area: (a) physical models and (b) statistical models. The majority of physical approaches are local and investigate a portion of a slope, a single slope or a single landslide (Buma and Dehn, 1998, 2000; Dehn and Buma, 1999; Tacher and Bonnard, 2007; Bonnard et al., 2008; Comegna et al., 2013; Rianna et al., 2014; Villani et al., 2015; Collison et al., 2000; Chang and Chiang, 2011; Coe, 2012; Melchiorre and Frattini, 2012). In contrast, many studies based on statistical approaches analyse future climate change impacts on landslides at regional scales (Jakob and Lambert, 2009; Jomeli et al., 2009; Turkington et al., 2016; Lee et al., 2014; Winter and Shearer, 2015; Ciabatta et al., 2016; Fan et al., 2013; Kim et al., 2015; Gassner et al., 2015; Shou and Yang, 2015; Wu et al., 2015; Gariano et al., 2017; Sangelantoni et al., 2018).
The performance of statistical models versus physical models has been compared in several studies (e.g. Cervi et al., 2010; Zizioli et al., 2013; Davis and Blesius, 2015; Ciurleo et al., 2017; Bartelletti et al., 2017; Galve et al., 2017; Oliveira et al., 2017). Some results indicate slightly better results with deterministic models (Ciurleo et al., 2017), whereas some others demonstrate the better performance of statistical ones (Galve et al., 2017; Bartelletti et al., 2017). These variations can depend on the assumptions made on hydrogeological system model and on the knowledge of the input parameters.
The advantages of physical models, in the case of accurate characterization of the sites, are the quantification of the effect of future climate scenarios on landslides at a regional scale, permitting the consideration of the spatial variability of the environmental parameters, and the quantification of the evolution of the hazard. However, it appears that there are still few studies dedicated to the quantification of the impact of future climatic and land cover scenarios on landslides.
In this study, the impacts of global change on landslide hazards is analysed according to two features: (i) the evolution of land cover, which can be analysed as the evolution of vegetation cover and its effects on landslides, and (ii) the evolution of climatic conditions, which result in the evolution of the hydrogeological conditions. Different types of landslides are considered here, and the effects of global changes on each of them are analysed. This analysis is conducted within a Pyrenean valley in a high-elevation context. We first introduce an overview of the method for evaluating the impact of global change on landslide hazards. Then, the mechanical and hydrogeological models are briefly presented, as well as the geological setting of the studied site. The socioeconomic and climatic scenarios and the way they are used in the mechanical and hydrogeological models are described. The last section presents the results of the modelled scenarios and a final discussion of the results.
The evaluation of the impacts of global change on landslide activities is realized through the analysis of the effects of both vegetation cover and meteorological conditions on landslide activities; this analysis was conducted with the following method (Bernardie et al., 2017; Grandjean et al., 2018):
- -
The assessment of future land cover is addressed through the construction of four prospective socioeconomic scenarios and their projection to 2040 and 2100, which are then spatially modelled with land use and land cover change (LUCC) models.
- -
The climate change inputs for this study correspond to two greenhouse gas emissions scenarios. The simulations were performed with the greenhouse gas (GHG) emissions scenarios (RCP: representative concentration pathways, according to the standards defined by the IPCC) RCP4.5 and RCP8.5.
The impacts of land cover and climate change are then addressed through these different scenarios that provide the future evolution of hydromechanical parameter variations used to compute the hazard levels. The hydromechanical model is based on a large-scale slope stability assessment tool that combines a mechanical stability model, a vegetation module that modulates the stability parameters to take into account the effects of vegetation on the mechanical soil conditions (cohesion and overload) and a hydrogeological model that provides the water table level computed from meteorological temporal data. The main algorithm computes a spatially distributed factor of safety (FoS) for the two selected future periods (2040 and 2100) and the different LUCC models.
2.1 ALICE slope stability model
ALICE (Assessment of Landslides Induced by Climatic Events) was developed by the French Geological Survey (BRGM) to support landslide susceptibility assessment and mapping for areas ranging from individual slopes to regions (Sedan et al., 2013; Baills et al., 2011; Vandromme et al., 2015; Thiery et al., 2017; Vandromme et al., 2020). Developed in a GIS (geographic information system) environment, it is a physically distributed model based on a limit equilibrium method that computes FoS along 2D profiles over the entire area. It can integrate different landslide geometries, the spatial and inherent heterogeneity of the surficial deposits and geology and their geotechnical parameters, triggering factors (i.e. water and seismicity), and land cover change. The 3D geometry of the studied area is entered as a dataset in raster format: topography and layer interfaces are represented with different DEMs (digital elevation models). Geomechanical characteristics, namely, cohesion (c′), friction angle (ϕ′) and volumetric weight (γ′), are associated with each geologic and surficial-deposit layer. These parameters can be implemented with a constant value or with probabilistic distributions to take into account the environmental variability of these parameters and the uncertainties associated with the values of these parameters. Like the other geometrical inputs of the model, the ground water table level (GWL) is implemented in raster format, as a piezometric map: a minimum level and a maximum level are required. Then, the GWL can be implemented empirically between a saturation level from 0 (dry conditions, minimum level) to 1 (saturated conditions, maximum ground water level). The 2D profiles are automatically generated from a digital elevation model using the local drain direction. A slope stability assessment is performed on each 2D profile, and each profile covers the whole studied area. The slope stability calculation is performed considering the forces applied on sliding bodies along a potential slip surface that can be circular or along an interface. The rotational slip surfaces are characterized by length, a minimal depth and a maximal depth. The shallow translational slip surfaces are defined by an interface, a length and a scarp angle. The shear strength of the soil along the potential failure plane is given by the Mohr–Coulomb failure criterion. The Morgenstern and Price (1965, 1967) method is used to calculate FoS. Once all parameters (geometrical, geotechnical, landslide type and triggering factor) are implemented in the model, several sliding surfaces are computed along each profile. The sliding surface with the lower FoS is kept, and this value is attributed to every cell concerned by this sliding surface. Moreover, in the objective of improving the accuracy of the calibration and the validation, a strategy for calibrating all the parameters has been developed (Vandromme et al., 2020).
2.2 GARDÉNIA hydrological model
The hydrogeological model GARDÉNIA (modèle Global À Réservoirs pour la simulation de DÉbits et de NIveaux Aquifères; Thiéry, 2003) is a lumped hydrological model. It simulates the main water cycle mechanisms in a catchment basin (rainfall, snowmelt, evapotranspiration, infiltration and runoff) by applying simplified laws of physics to flows through successive reservoirs. It considers a system of three tanks, reproducing the three-layer characteristics of the hydrological behaviour of the soil: (i) the top zone, i.e. the first 10 cm, where evapotranspiration occurs; (ii) the unsaturated zone, where runoff occurs; and (iii) the saturated zone. Once calibrated, the model permits us to (i) calculate the balance of rainfall, snowmelt, evapotranspiration, runoff and infiltration into the underlying aquifer; (ii) analyse the consistency between meteorological observations and observations of flow rates or piezometric levels; (iii) recreate, for a given catchment basin, the flow rates of a river or spring and/or the piezometric levels at a given point in the aquifer during periods when measurements are not available; and (iv) simulate flow rates resulting from dry periods or unusual precipitation sequences, groundwater piezometric levels, observed precipitation and precipitation resulting from scenarios (drought or high-water periods resulting from climate change scenarios). For our purpose, it allows for the estimation of the daily local piezometric level evolution based on the meteorological parameters that might evolve due to climate change.
2.3 Linking slope stability and hydrological models
The spatialized piezometric level can then be integrated into the slope stability assessment equations developed in ALICE. In this approach, a significant approximation is made by spatializing the piezometric level in the surficial formations; the ground reality is much more complex. This so-called piezometric level has to be considered an indicator of the water content of the soil, which will promote slope destabilization. This indicator is called the “water filling ratio”: a value of one means that the water table level is at its maximum, and zero means that the level is at its minimum.
This method results in an analysis of the dynamic evolution of landslide susceptibility in the area of interest. Landslide hazard assessment considers runout, the magnitude and the return period for a given intensity (Varnes, 1984). As in many cases, the hazard analysis is not completed. Notably, runout is not accounted for in this study. Nevertheless, the landslide susceptibility assessment is converted into landslide hazard assessment by expert knowledge (Van Western et al., 2006, 2008; Corominas et al., 2014).
2.4 Validation of the model
Because this study is focused on the probability of slope failure (i.e. initiation), the boundaries of active landslides are classified into two zones: (i) the zone of depletion and (ii) the zone of accumulation (Varnes, 1978).
From the zone of depletion, and the very high and high hazard classes defined from the FoS values, several statistical tests can be computed: the relative error (ξ), receiving operating characteristic curve and associated area under the curve (ROC (AUC)), prediction rate (PR (AUC)), true positive rate (TPr), and false positive rate (FPr). These classical evaluation and validation tests are defined by Brenning (2005), Beguería (2006), Guzzetti et al. (2006), and Vandromme et al. (2020). They allow for the assessment of the predictive power of the simulated maps (Brenning, 2005). ξ measures the model performance; it is defined as the total proportion of observations (i.e. the zones of depletion) correctly classified in the very high and high hazard classes (Thiery et al., 2007). ROC (AUC) indicates the degree to which the zones of depletion are explained by the computed FoS. It is a tool for validating the different models by a threshold-independent measure of discrimination between the proportion of correctly predicted positive cases and the proportion of correctly predicted negative cases (Brenning, 2005). The values fluctuate between 0.5 (no discrimination) and 1 (perfect discrimination). The PR (AUC) is computed on the basis of the different FoS values and the zones of depletion. It is obtained by varying the decision threshold and plotting the respective sensitivities against the total proportions of the zones of depletion. It provides a validation index of the simulated maps (Chung and Fabbri, 2003). Finally, TPr and FPr are the proportion of correctly classified observations and the proportion of incorrectly classified observations, respectively (Thiery et al., 2007; Vandromme et al., 2020).
The Cauterets municipality is representative of the climatic, lithological, geomorphological and land cover conditions observed in the French Central Pyrenees (Viers, 1987). The municipalities are highly affected by several natural hazards, such as landslides, rockfalls, debris flows and flash floods, and the area was restored by Restauration des Terrains de Montagne Survey (RTM) from the National Forests Office (ONF) at the end of the 19th century (De Crécy, 1988). Dominated by ridgelines, with a maximum elevation of approximately 2500 m a.s.l., the municipality is subject to oceanic climatic influences, with an average rainfall of 1157 mm yr−1 and a thermal amplitude of 13 ∘C, typical for a mountain area (Gruber, 1991). However, under the oceanic influence, the valley is marked by a mountainous climate with intense storms during summer and autumn and large snowfalls during winter (it is often the snowiest resort area in France). This enclosed valley juxtaposes several types of fractured lithology and reliefs with different exposures and strong elevation variations (Barrère et al., 1980). Consequently, the open slopes located above 1600 m a.s.l. with eastern exposure have more sunshine in summer and more snowfall in winter than the valley bottom or the slopes exposed to the west.
The test site is located in the middle part of the municipality of Cauterets in an area of approximately 70 km2 and is characterized by a large variety of landslides. It can be subdivided into three geomorphological units. The southern unit is dominated by plutonic magmatic rocks (especially granites) overlapped by limestones, while the western and eastern units are composed of metamorphic rocks (especially schists and sandstone) with intercalation of limestone. The lithology can be overlapped by morainic deposits on gentle slopes and morainic colluviums on the steepest slopes (Barrère et al., 1980; Viers, 1987).
A landslide inventory was compiled at a 1:10 000 scale through a diachronic interpretation of several data sources (i.e. air-photo interpretation between 1950 and 2016, ortho-image analysis, landform analysis with an accurate DTM (digital terrain model) analysis and interpolation of triplets obtained by lidar in 2016, literature analysis) completed by field surveys. A total of 346 objects were identified, including landslides with rotational shear surfaces, landslides with translational shear surfaces and deep-seated gravitational slope deformations. The active one has only been selected for the study (each object was stored in a GIS database with its geometrical – perimeter, area, and maximal length and width – and geomorphological characteristics – type, magnitude and state of activity based on morphological features combined with the age of the event; Wieczoreck, 1984). A confidence mapping index (CMI) based on field recognition, aerial photograph interpretation (API) and literature review (Thiery et al., 2007) is applied for each identified object. Of the objects identified, 82.5 % have a high confidence index score, 12.5 % have a moderate confidence index score and 4 % have a low confidence index score. This type of index is necessary for selecting recently or currently unstable objects and validating the failure simulations for recent and future periods.
The majority of these landslides are translational or rotational (346 out of 426), the others being complex landslides, which are more difficult to classify and understand. In order to respect the modelling capabilities of ALICE, only landslides with translational and rotational shear surfaces (according to the classification from Cruden and Varnes, 1996) were selected in the inventory. Figure 1 and Table 1 depict the morphology and morphometric–environmental characteristics of the different landslide types that have high confidence index values.
We will consider the following four types (one translational and three rotational): (i) shallow translational landslides, which occur mainly in various colluviums and weathered materials (especially in weathered schist) from gentle to very steep slopes (from 12 to 47∘); (ii) shallow rotational landslides, which occur in thin moraine deposits (colluviums, removed-moraine deposits or moraines in place) from moderately to very steep slopes (from 17 to 50∘), the majority of which are located near streams and are triggered by a basal incision caused by torrents; (iii) moderately deep rotational landslides; and (iv) deep rotational landslides, which have a common rotational shear surface in the initiation zone. These occur in materials such as moraine deposits and/or weathered materials at deeper depths. They are located on gentler slopes than shallow landslides (from 11 to 35∘).
Table 2 outlines the main predisposing factors affecting each landslide type. The different thematic data are derived from (i) air-photo interpretation analysis, (ii) airborne lidar surveys, (iii) satellite imagery analyses and (iv) field surveys. The DTM (10 m resolution) is derived from the airborne lidar data. The slope gradient map and the flow accumulation map are derived from the DTM. The lithological map is based on the main lithological units described in a geological map produced by the French Geological Survey (Barrère et al., 1980) at a 1:50 000 scale and is completed by fieldwork. The surficial-formation map is obtained by a geomorphological approach with the segmentation of homogeneous areas of the landscape associating surficial-formation type, their facies and the processes following the approach developed by Thiery et al. (2007). The surficial-formation thickness map is obtained from field observations of surficial-formation outcrops along the stream and the slopes. The thickness of the formations is closely associated with the slope degree. For instance, screes are located on steep slopes, whereas moraine deposits or colluviums are located on gentler slopes. Therefore, an exponential regression function obtained by plotting the thicknesses and the slope degrees derived from the DTM is computed to obtain a spatial prediction and continuous values for each type of surficial formation (Thiery et al., 2017).
4.1 Socioeconomic scenarios and LUCC mechanical effects
The overall methodological approach for constructing socioeconomic scenarios is fully described in Houet et al. (2017). It consists of working with stakeholders to co-construct fine-scale socioeconomic scenarios based on existing national or regional sector pathways to produce spatially explicit local LUCC maps. The method relies on two participatory workshops aiming first to define the narrative scenarios and second to validate the narrative scenarios and pre-identify the areas of future LUCC. Meanwhile, the use of the LUCC model allows for the simulation of the land cover changes induced by the future land use changes which, in turn, can have feedback effects on land cover. Thus, the narrative scenarios are defined to produce relevant inputs for the LUCC model, while the model itself is developed to be able to represent the likely land cover changes identified in the narrative scenarios and to provide quantitative outcomes to illustrate the narratives. Four scenarios have been defined according to this method, resulting in future land cover maps for the four scenarios. Among the few maps obtained through this method, we have considered that those of 2040 and 2100 (Fig. 2) are the most representative of the period 2021–2050 and 2071–2100:
- -
Sc1: abandonment of the territory,
- -
Sc2: sheep and woods,
- -
Sc3: a renowned tourism resort,
- -
Sc4: the green town.
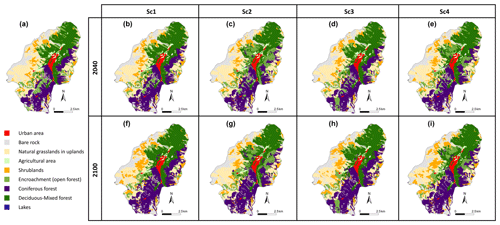
Figure 2Maps of existing and future land cover for the four scenarios in 2040 and 2100 (Houet et al., 2017).
Land cover can have a significant influence on slope stability through different processes: (i) the vegetation can reinforce the surficial stability with reinforcement from roots; (ii) the vegetation adds weight to the slope; and (iii) the vegetation modifies the hydraulic system. We state here that a single mechanical action can encompass these processes by considering the contribution of the cohesion offered by the roots, which is the predominant effect of vegetation on slope stability (Kokutse and al., 2016; Norris et al., 2008). The influence of the roots is therefore taken into account as an additional cohesion value. As roots can vary according to their orientation, most studies consider their influence on the friction angle of the material to be negligible (Norris et al., 2008). Different models can be found for estimating cohesion; their quality varies and requires several types of biological input data (root resistance, root density and root morphology). Site effects are also significant, making it impossible to find a single value for cohesion for each species in the literature. When the site conditions are not limiting, tree root systems are classically categorized into three types: taproots, heart roots and flat roots. Wu et al. (1979) investigated how the three root morphologies lead to breaks during a landslide and found that pivoting systems are likely to mobilize the full potential of root resistance because the stresses are concentrated on the main root. On the other hand, for fasciculate and plate systems, the stresses are distributed over the set of roots that are thinner and yield one after the other, which does not mobilize the full resistance potential of resistance of the system.
In the context of Cauterets, the presence or absence of a forest constitutes the main parameter that may modify the slope stability. This study considers four land cover types that may have a significant influence on slope stability: (a) no trees (urban areas, shrublands, natural grasslands, agricultural areas and mineral surfaces), (b) coniferous forest, (c) deciduous forest and (d) encroachment (open forest).
Table 3 summarizes the additional cohesion provided by each type of forest considered in the study area. The cohesion value for each type of forest is determined from Wu et al. (1979) and Norris et al. (2008).
The land cover in the current and future land cover maps were categorized according to these classifications.
The depth of the influence of vegetation is limited by the maximum extension of the roots; Bischetti et al. (2009) quote Schiechtl (1980), who estimates that in mountainous terrain, the roots do not exceed 1 m in depth. This value is comparable with those indicated by Crow (2005), who found that 90 % to 99 % of the root mass is less than 1 m deep. In this study, we considered the influence of vegetation to be limited to 1 m below the DTM.
Figure 3 shows the changes in the area of the four categories of land cover (coniferous, deciduous, open forest and no forest) for 2040 and 2100 in the fourth scenario; in 2010, a majority of the area was composed of forest (52.0 %), with a balance between coniferous (19.3 %) and deciduous forests (21.7 %) and a smaller open-forest area (11.0 %). The future changes show a slight increase in the forested area in 2040 and a larger increase in 2100, regardless of the scenario. Considering the proportions of the different types of forest, the most important changes concern the scenarios of the abandonment of the territory and sheep and woods. There is a decrease in open forest in the scenario of the abandonment of the territory, whereas open forest increases for the scenario of sheep and woods; in the scenario of the green town, the proportions of the different land cover in 2040 remain similar to those in 2010, and the coniferous-forest surface increases in 2100. Finally, the scenario of a renowned tourism resort shows an increase in the area of coniferous and deciduous forests.
4.2 Climate change scenarios and hydrogeological inferences
To take climate change into account, we selected two greenhouse gas emissions scenarios, labelled in DRIAS (2014) as RCP4.5 and RCP8.5 and computed using the ALADIN-Climate model (ALADIN international team, 1997; Aire Limitée Adaptation Dynamique Développement International; International development for limited-area dynamical adaptation) of Météo-France.
Three periods were defined that are considered to be representative of the current year, 2050 and 2100: (i) reference period, 1981–2010; (ii) short-term period, 2021–2050; and (iii) long-term period, 2071–2100. A 30-year duration is commonly used for meteorological parameters (e.g. precipitation) and defined according to WMO criteria (World Meteorological Organization, 2018).
From a general point of view, the climate models show a tendency toward an increase of the intensity of extreme precipitation events and annual cumulative precipitation in the short and long term. The model results depend on the elevation of the area of interest. In the highest areas, the models show an increase in cumulative precipitation. At the lowest points, they indicate a slight increase in the short term and a small decrease in the long term. Concerning the temperature, the models clearly indicate a significant increase in the temperatures in the short (+1.54 ∘C) and long term (+4 ∘C), resulting in large changes in the precipitation pattern (the balance between snow and rainfall). Figure 4 shows that both scenarios indicate the same tendency in the short term, but they show some significant differences in the long term. Moreover, there is a strong increase in liquid precipitation in the long-term RCP8.5 scenario, coupled with a decrease in solid precipitation over the same period. For the long-term RCP4.5 scenario, liquid precipitation increases slightly compared to that in the short term. The solid precipitation also decreases for the same period.
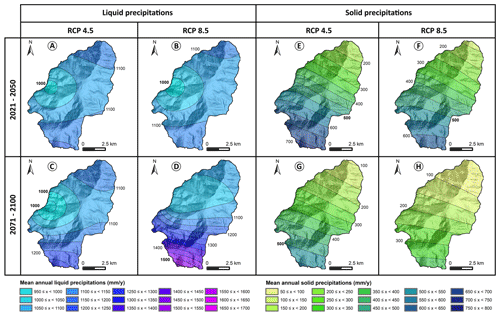
Figure 4Mean annual liquid and solid precipitation in the short and long term for RCP4.5 and RCP8.5 scenarios (from DRIAS, 2014). For liquid precipitation: (a) 2021–2050 period, RCP4.5 scenario; (b) 2021–2050 period, RCP8.5 scenario; (c) 2071–2100 period, RCP4.5 scenario; and (d) 2071–2100 period, RCP8.5 scenario. For solid precipitation: (e) 2021–2050 period, RCP4.5 scenario ; (f) 2021–2050 period, RCP8.5 scenario; (g) 2071–2100 period, RCP4.5 scenario; and (h) 2071–2100 period, RCP8.5 scenario.
With the GARDÉNIA model, which transforms precipitation rates into water table variations, the daily water table level is computed from the daily meteorological parameters. In this way, it is possible to obtain the distribution of the water table level between low and high piezometric levels that reflect the minimum and maximum indicators of the soil water content (i.e. filling ratio). The piezometric level series, when converted into the water filling ratio, allows calculations to determine the water level class (WLC) and the associated frequency of occurrence, f(WLC), for each WLC. Figure 5 shows the frequency distribution of the WLC of water level filling ratios for the current period (1981–2010), two future periods (2021–2050 and 2071–2100) and two climatic scenarios (RCP4.5 and RCP8.5). This figure demonstrates the significant increase in the mean water table level in future periods, especially between 2071 and 2100 under the most extreme scenario (RCP8.5).
5.1 Landslide hazards in 2010
The objective of Fig. 6 is to demonstrate the sensitivity of the model to the evolution of the water filling ratio; thus for each landslide type, 10 simulations were performed according to the 10 ground water filling ratios (from 0 to 1). Figure 6 depicts the surface occupied by the different classes of hazards (according to FoS thresholds conventionally used for stability analyses; Kirsten, 1983; Pack et al., 2001; Table 4)
As seen in Fig. 6, this analysis shows that the highly susceptible surface areas are the largest for deep landslides and the smallest for shallow landslides.
The relationship of the evolution of the hazard to the water table level depends on the type of landslide.
For the translational type (Fig. 6a), the graph indicates that the area of the high hazard class is insignificant until a 0.6 water filling ratio is reached; the hazard is then highly amplified with a strong increase in the areas of very high and high landslide hazards. Similarly, for shallow rotational landslides (Fig. 6b), the area susceptible to landslides is very low at lower water filling ratios until the 0.5 value. At a water filling ratio of 0.8, there is a change in the evolution of the very high and high hazard classes.
In contrast, the behaviour of moderately deep (Fig. 6c) and deep (Fig. 6d) landslides indicates a larger area of high hazards: indeed, the area that is susceptible to landslides remains higher than that for the shallow rotational landslides. The area prone to landslides increases linearly with the lower water filling ratio values. The area then increases substantially at higher water filling ratios.
In summary, a threshold of behaviour appears in the results, changing from low hazard levels and linear evolution to higher hazard levels and exponential evolution with the water filling ratio; this threshold depends on the type of the landslide.
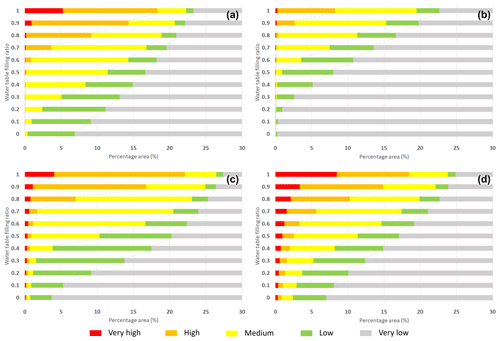
Figure 6Evolution of the hazard level according to the water table level for the four types of landslides (2010): (a) translational, (b) shallow rotational, (c) moderately deep rotational and (d) deep rotational.
Figure 7 illustrates landslide hazard maps for a conservative scenario based on a GWL of 0.9 to explain the landslide events observed in 1992 over the ski resort (Fig. 1B2) and over the Grange de Pan (Fig. 1B1). This type of situation, which corresponds to slope destabilization, is currently observed in moraine deposits overlapping weathering materials either in the French Pyrenees (Lebourg et al., 2003b) or in the southern French Alps with similar geomorphological conditions (Thiery et al., 2017; Vandromme et al., 2020). Each map shows the initiation areas of the different inventoried active landslides.
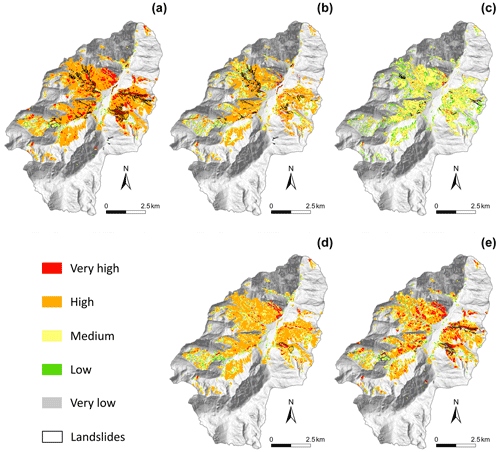
Figure 7Landslide hazard map for 2010: (a) shallow translational, (b) shallow rotational, (c) moderately deep rotational, (d) deep rotational and (e) all landslide types.
The performance of the model is demonstrated through several statistical tests provided in Fig. 8. For shallow translational landslides, the relative error is low (i.e. ξ=0.21), and the true predictive rate is high (i.e. TPr=0.80), indicating that the zones of depletion are well recognized by the model when the water table is high. This situation corresponds to the different field observations. For shallow rotational landslides, the simulated map does not recognize current unstable surfaces very well; the relative error is high, ξ=0.79. However, the ROC (AUC) and PR (AUC) indexes show high values. This is partly due to the low surface area of this type of landslide. For moderately deep rotational landslides, the zones of depletion are well simulated by the model (i.e. ξ=0.37 and TPr=66 %). Finally, for deep rotational landslides, the relative error is relatively low (i.e. ξ=0.35), and the ROC (AUC) and the prediction rate are high (i.e. ROC (AUC) =0.82; PR (AUC) =0.75). This means that the models with such a water table level are able to simulate this particular type of landslide, which has a deep shear surface, well and that the models are discriminating.
5.2 Future evolution of landslide hazards
5.2.1 Impacts of future land cover on landslide hazards
Figure 9 shows maps of the differences in FoS values between the current and future periods for the four socioeconomic scenarios and the two future periods, with the objective of specifically analysing the influence of future land cover on landslide hazards. Climate conditions are considered equal between current and the two future periods and set to the actual conditions. This analysis was performed on shallow rotational landslides; this landslide type might be sensitive to the evolution of land cover. In contrast, the stability of deeper landslides is influenced by the effects of vegetation.
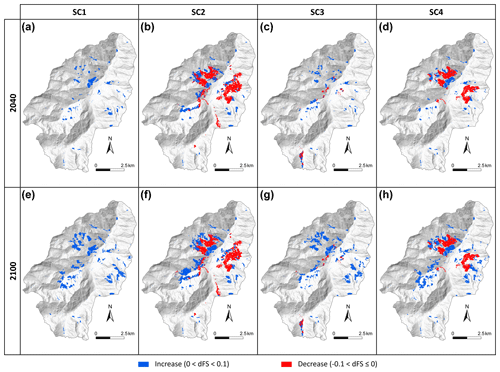
Figure 9Map of differences in FoS between current and future periods for the four scenarios and the two future periods: (a) abandonment of the area in 2040, (b) sheep and woods in 2040, (c) a renowned tourism resort in 2040, (d) the green town in 2040, (e) abandonment of the area in 2100, (f) sheep and woods in 2100, (g) a renowned tourism resort in 2100 and (h) the green town in 2100. The water table is constant.
These maps indicate some differences between the current and future landslide hazards. These differences are characterized by local increases and decreases in slope stability. These confined contrasts are significant, although they remain limited, as the change in the FoS ranges from −0.1 to +0.1, which is quite small.
When considering the impacts of future land cover on hazard level changes in detail, we observe the following.
In the scenario of the abandonment of the territory, we observe a general increase in slope stability, which is in good accordance with the reduction in open forest highlighted in Fig. 4. In the same way, the scenario of a renowned tourism resort is characterized by an increase in stability, linked with the increase in deciduous and coniferous forests and very limited areas of decreasing slope stability. In contrast, the scenario of sheep and woods results in the destabilization of some areas because of the development of open forest. This scenario shows contrasting spatial evolution, as other areas become more stable. Finally, the scenario of the green town results in more localized destabilization areas than the scenario of sheep and woods, with patches of open forests and some improvement in stability in areas where coniferous forests are predicted to increase.
5.2.2 Impacts of future land cover and climate on landslide hazards
We integrated climate change into the analysis of future landslide hazards. Various analyses can be performed on the results. In this paper, one of the indicators that we intend to quantify is the tendency of future hazard evolution. To this end, we computed a “mean” of future landslide hazards. We computed the FoSMEAN indicator, which is the mean FoS value for each cell in the studied area:
where WLC is the water level class; f(WLC) is the frequency of having the filling ratio equal to the WLC, as determined in Fig. 5; and n is the number of filling ratio level classes, which is, in this case, is equal to 11 (see Fig. 5). In other words, we summed the hazard maps corresponding to the water table ratio, weighted according to the distribution of the water level filling ratio classes for the analysed period shown in Fig. 5. The final result of the process is a single map that represents the mean landslide hazard over a given period.
Figures 10 and 11 show maps of the difference in the FoS between the future (long term) and current periods for the “shallow rotational” and “deep rotational” landscape types under the two climate scenarios, RCP4.5 and RCP8.5. Even if all combinations of socioeconomic and climate scenarios have been computed, only one socioeconomic scenario is presented in this paper. Indeed, the results show that the effect of climate on landslide hazards is more significant than the land cover. In these figures, the socioeconomic scenario of the abandonment of the territory is considered.
Regardless of the scenario considered, there is a decrease in slope stability. The FoS change ranges between −0.1 and −0.2. Therefore, even in a case where the future evolution of the forest stabilizes the slopes, the evolution of the groundwater table destabilizes the area more.
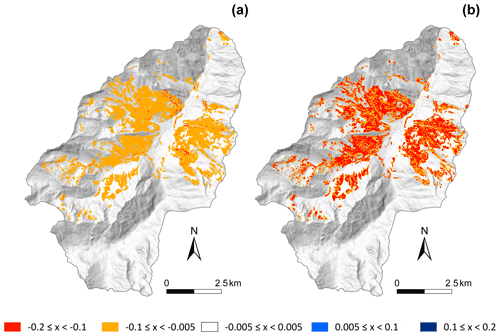
Figure 10Maps of the difference in FoS between the future and current periods – shallow rotational landslide.
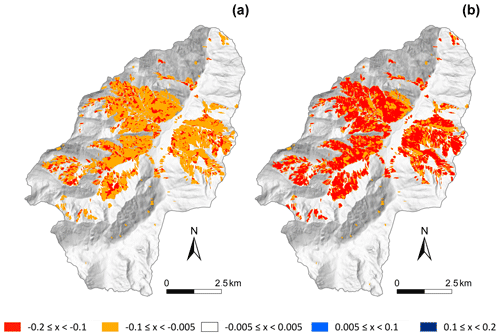
Figure 11Maps of the difference in FoS between the future and current periods – deep rotational landslide.
These figures can be more precisely analysed, as seen in Fig. 12, which provides the surface percentage for each hazard class in the whole study area for each type of landslide, each climatic scenario and the two future periods. For clarity, the area corresponding to the very low hazard class has been removed from the figures.
For the shallow rotational landslides, there is a strong increase of the medium hazard class, from 0.29 % to 3.23 % in 2040 for the RCP4.5 scenario; the high and very high classes remain at the same very low level. Thus, the sum of the surface classified in the three highest levels increases from 0.34 % in 2010 to 2.12 % in 2040 (RCP8.5) and 2.78 % in 2100 (RCP8.5).
For the translational landslides, the most important increase occurs in the high hazard class, whereas there is a decrease in the low hazard class, indicating that there is a transfer from low to medium and high classes. The total area classified in the three highest levels increases from 5.95 % in 2010 to 8.04 % in 2040 (RCP8.5) and 8.49 % in 2100 (RCP8.5). The very high class remains at a very low level.
For the moderately deep landslides, some significant changes are evident, with a large area of the low hazard class changing to the medium hazard class. The total area classified in the three highest levels increases from 4.83 % in 2010 to 9.48 % in 2040 (RCP8.5) and 10.27 % in 2100 (RCP8.5).
The deep landslides evolve differently, as the medium, high and very high hazard levels increase significantly, from 5.94 % in 2010 to 8.81 in 2040 (RCP8.5) and 8.84 % in 2100 (RCP8.5).
This study analyses the evolution of future landslide hazards under the effects of land cover and climate changes. An accurate map provides a reference for establishing a comparison with the current state of landslide hazards. The criteria computed to quantify the accuracy of the model permit the validation of the model. For the four landslide types, the different models computed for 2010 are considered representative of this Pyrenean mountainous context. The triggering conditions used in the model are similar to those observed in the field and proposed by Fabre et al. (2002) and Lebourg et al. (2003a). The statistical results are satisfactory for each landslide type (e.g. the relative error ξ=0.21 for the shallow translational, ξ=0.79 for the shallow rotational, ξ=0.37 for the moderately deep rotational and ξ=0.35 for the deep rotational). Even if the ξ for shallow rotational landslides is slightly lower, we consider the results valid, as the majority of these landslides occur near torrents due to basal incision from the torrents. Hence, ALICE is not dedicated to simulating this specific mechanism, and the results do not indicate a high level of landslide hazards in these particular areas.
All the results show that future global change scenarios imply significant evolution in landslide hazards, regardless of the climate scenario or the period considered. Generally, the strongest effects on landslide hazards are linked to the evolution of the climate rather than the evolution of land cover; indeed, we have previously seen that, despite the incorporation of the effects of land cover in the models, its impact remains quite limited, with an evolution of the FoS ranging from −0.1 to +0.1. These limited changes are, however, significant, depending on the future land cover linked to the socioeconomic scenarios. In particular, the reduction in human presence (e.g. scenario of the abandonment of the territory) results in an increase in slope stability. In contrast, the increase in anthropic activity implies some contrasting evolutions of the area, with some reduction in slope stability. The scenario of sheep and woods illustrates this aspect, with the destabilization of areas due to the development of open forest. These scenarios, which represent four possible land cover evolution trajectories, indicate contrasting spatial evolution patterns.
In contrast, apart from the impacts of land cover change on landslide hazards, the quantification of future changes caused by climate change indicates more significant changes in the size of the area that is susceptible to landslides; the size of this area depends on the landslide type considered. Compared to the current period, the size of the area that is prone to deep landslides is larger in the future than the area prone to shallow landslides (both rotational and translational). On the other hand, the increase rate of areas prone to landslides is higher for the shallow landslide type than for the deep landslide type; indeed, the area classified in the three highest hazard levels is projected to increase approximately 6-fold from 2010 to 2040 (RCP8.5) and 8-fold from 2010 to 2100 (RCP8.5) for shallow rotational landslides, whereas the area classified in the 3 highest hazard levels is projected to increase approximately 1.5-fold from 2010 to 2040 (RCP8.5) as well as to 2100 (RCP8.5) for deep rotational landslides. This result confirms the analyses of McInnes et al. (2007), Moore et al. (2007), and Crozier (2010), which suggested that the future evolution of landslide hazards in any environment depends on the type of landslide and in particular on its depth and volume.
Figure 12 reflects the mean tendency of the future scenarios within a 30-year period. Another feature to be analysed is the occurrence frequency of landslide events. Indeed, as the hydrogeological model considers the antecedent water content, an increase in total rainfall implies that there will be an increase in the frequency of the highest water table level, as indicated in Fig. 5. As a result, when the antecedent water content is high, less water is required to trigger movement (Crozier, 2010). Therefore, landslides will occur more frequently. Figures 13, 14 and 15 correspond to the highest frequency of the water filling ratio for each period (0.5 for the 1980–2010 period; 0.6 for the 2021–2050 period, scenario RCP 8.5; and 0.7 for the 2071–2100 period, scenario RCP8.5). These results indicate that the highest frequency of landslide hazards corresponding to the 2021–2050 period (scenario RCP8.5) (Figs. 13b, 14b and 15b) will increase 1.5-fold, whereas the highest frequency of landslide hazards corresponding to the 2071–2100 period (scenario RCP8.5) (Figs. 13c, 14c and 15c) will increase 4-fold.
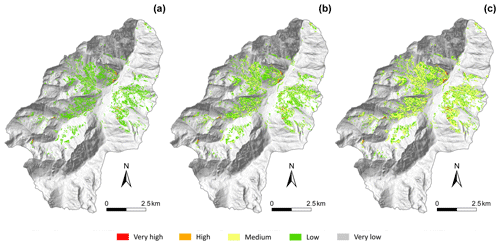
Figure 13Landslide hazard levels corresponding to the highest frequency of the water filling ratio for each period – shallow rotational landslide.
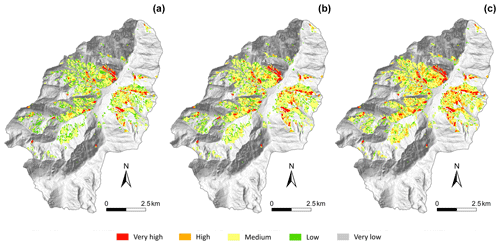
Figure 14Landslide hazard levels corresponding to the highest frequency of the water filling ratio for each period – deep rotational landslide.
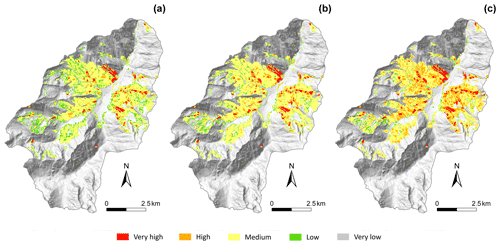
Figure 15Landslide hazard levels corresponding to the highest frequency of the water filling ratio for each period – all landslide types.
These significant results have to be analysed in consideration of the tested hypotheses. Indeed, we have previously seen that the effects of land cover on landslide hazards remains quite limited. This may be inconsistent with some authors which explain that anthropic activities have a high impact on the stability of slopes (Glade, 2003; Remondo et al., 2005). Moreover, Crozier (2010) states “Changes resulting from human activity are seen as a factor of equal, if not greater, importance than climate change in affecting the temporal and spatial occurrence of landslides”. Several features could explain such differences. Indeed, some land cover effects are considered in this study but not all. The effects of the roots on slope stability is considered in the analysis, but it could be more accurate to consider the effects of vegetation (suction and evapotranspiration) linked with the runoff–infiltration balance, which is not spatially realized in this study. The infiltration capacity in forested areas may be higher than that in areas without forest; in some cases, this may result in a reduction of slope stability, despite the soil reinforcement from roots (Crozier, 2010). Moreover, some anthropic modifications that may appear, such as slope modifications, due to infrastructure construction or urbanization, are not considered in this approach. Furthermore, the modification of the land cover is quite small in this territory, as seen in Fig. 3. In conclusion, in this study area human activities may have a minimal effect on the environment, which may explain that the increase of precipitation due to climate change could have more impact than human action.
These results must also be put in perspective of the accuracy of the data. It must be noted that some uncertainties remain in this study with the lack of on-site hydrogeological knowledge to validate the hypotheses. Even if the hypotheses are not totally validated, we can assume that the comparisons between future and current states of hydrogeological context and the stability of the slopes remain valid as we analyse trend evolution.
Some uncertainties are also associated with the future climatic data used, as the mountainous area has high spatial variability, with large changes in elevation over short distances. The 8 km resolution of the input data could be improved, and it may be of high interest to consider data with a higher resolution. Future results from the CLIMPY project (Characterisation of climate change and provision of information for adaptation in the Pyrenees; https://opcc-ctp.org/en/climpy, last access: 6 January 2021) will surely reduce uncertainties in the input data. Moreover, a finer analysis of the balance of rainfall and snow would greatly improve the accuracy of the results.
It could be interesting to apply such an approach to other sites so as to compare the impact of global change in other territories. An accurate assessment of current landslide hazards constitutes the first step for having a good extrapolation to future time. For that, the necessary input parameters – inventory, geological analysis, geotechnical parameters and DTM – have to be of good accuracy. It is then necessary to consider a model that integrates the effects of the vegetation and of the hydrometeorological conditions. It necessitates adequate data resulting from LUCC and climate change models at a fine resolution.
The present work provides a method for quantifying the impacts of global change on a mountainous region at the valley scale. It evaluates and quantifies the influences of both vegetation cover and climate on landslide activities projected to 2100.
First, the results demonstrate the influence of land cover on slope stability through the presence and type of forest. Future land cover changes may lead to modifications in slope stability. Some increase in the stability could be observed in the areas where the forest is developing, whereas slope stability is decreasing in the areas where the forest is disappearing. These changes are significant, although they remain quite limited, as FoS variations range from −0.1 to +0.1.
Climate change may have a significant impact on the water content of the soil; the results indicate a reduction in the FoS in a large part of the study area, depending on the landslide type considered. Regardless of the scenario considered, there is a decrease in slope stability; the drop in FoS evolution ranges from 0.1 to 0.2. Even in a case where the future evolution of the forest stabilizes the slopes, the evolution of the groundwater table destabilizes the area more. These changes are not uniform over the area and are particularly significant for the most extreme scenario, RCP8.5.
Compared to the current period, the percentage of the area that is prone to deep landslides is higher in the future than the area prone to shallow landslides (both rotational and translational). On the other hand, the increase rate of areas prone to landslides is higher for shallow landslides than for deep landslides; indeed, the area classified in the three highest hazard levels is projected to increase approximately 6-fold from 2010 to 2040 (RCP8.5) and 8-fold from 2010 to 2100 (RCP8.5) for shallow rotational landslides, whereas the area classified in the three highest hazard levels is projected to increase approximately 1.5-fold from 2010 to 2040 (RCP8.5) and to 2100 (RCP8.5) for the deep rotational landslides.
Interestingly, the evolution of extreme events is related to the occurrence frequency of the highest water filling ratio, which will increase. The results indicate that the highest frequency of landslide hazards for the 2021–2050 period (scenario RCP8.5; Figs. 13b and 4b) will increase 1.5-fold, whereas the highest frequency of landslide hazards for the 2071–2100 period (scenario RCP8.5; Figs. 13c and 14c) will increase 4-fold.
The uncertainties associated with the future climatic data used constitute an issue that should be addressed. Forthcoming results from the CLIMPY project will surely reduce uncertainties in the climate data. Moreover, a finer analysis of the rainfall–snow balance, based on a more accurate hydrogeological model, will greatly improve the accuracy of the results, including the seasonality analysis.
This study constitutes the first step in the process of risk assessment for different scenarios of climate and economic development to evaluate the resilience of this region. All these methods have been implemented on a web-application platform (Grandjean et al., 2018) dedicated to stakeholders that will allow the stakeholders to obtain indicators of the best solutions for improving the resilience of valleys that are coping with global change.
The data used in this paper can be requested from the corresponding author. We ask interested researchers to please contact the authors of this article.
SB and RV conceived the modelling method, with inputs from YT. YT and IB collected the data for the study site. TH and MG built the socioeconomic scenarios. SB made all calculations. FM provides post processes of the results, as well as most of the figures. SB, RV and YT contributed to the discussion of the results. SB wrote the paper, with contribution from RV and YT. GG coordinated and designed this collaborative study in the frame of the SAMCO project, and reviewed the first version of the paper.
The authors declare that they have no conflict of interest.
This research was funded through the ANR (French Research Agency) project SAMCO (“Society Adaptation for coping with Mountain risks in a global change COntext”; grant no. ANR-12-SENV-0004-01). For more information, see http://www.anr-samco.com/ (last access: 6 January 2021).
The authors would like to thank the collaborators Hélène Bessière and Rodrigo Pedreros for their valuable help.
We would like to thank the two anonymous reviewers for their helpful comments and for their constructive and relevant remarks.
This research has been supported by the ANR (French Research Agency) (grant no. ANR-12-SENV-0004-01).
This paper was edited by Andreas Günther and reviewed by two anonymous referees.
ALADIN International Team: The Aladin project mesoscale modelling seen as basic tool for weather forecasting and atmospheric research, WMO Bull., 46, 317–324, 1997.
Alvioli, M., Melillo, M.,Guzzetti, F., Rossi, M., Palazzi, E., von Hardenberg, J., Brunetti, M., and Peruccacci, S.: Implications of climate change on landslide hazard in Central Italy, Sci. Total Environ., 630, 1528–1543, https://doi.org/10.1016/j.scitotenv.2018.02.315, 2018.
Baills, A., Vandromme, R., Desramaut, N., Sedan, O., and Grandjean, G.: Changing patterns in climate-driven landslide hazard: an alpine test site, The Second World Landslides Forum, October 2011, Rome, Italy, available at: https://hal-brgm.archives-ouvertes.fr/hal-00631008 (last access: 12 January 2021), 2011.
Barrère, P., Bois, J.-P., Soulé, J.-C., and Ternet, Y.: Carte géologique de la France au 1:50 000: Argelès-Gazost, XVI-47, 1070, BRGM, Argelès-Gazost, 1980 (in French).
Bartelletti, C., Galve, J. P., Barsanti, M., Giannecchini, R., Avanzi, G. D. A., Galanti, Y., Cevasco, A., Azañón, J. M., and Mateos, R. M.: GIS-Based Deterministic and Statistical Modelling of Rainfall-Induced Landslides: A Comparative Study, in: Advancing Culture of Living with Landslide, edited by: Mikos, M., Tiwari, B., Yin, Y., and Sassa, K., Springer, Cham, 749–757, https://doi.org/10.1007/978-3-319-53498-5_86, 2017.
Beguería, S.: Validation and evaluation of predictive models in hazard assessment and risk management, Nat. Hazards 37, 315–329, https://doi.org/10.1007/s11069-005-5182-6, 2006.
Bernardie, S., Vandromme, R., Mariotti, A., Houet, T., Grémont, M., Grandjean, G., and Thiery Y.: Estimation of landslides activities evolution due to land–use and climate change in a Pyrenean valley, in: Advancing Culture of Living with Landslides, Ljubljana, Slovenia, 29 May–2 June 2017, Springer, Cham, 859–867, https://doi.org/10.1007/978-3-319-53498-5_98, 2017.
Bischetti, G. B., Chiaradia, E. A., Epis, T., and Morlotti, E.: Root cohesion of forest species in the Italian Alps, Plant Soil, 324, 71–89, 2009.
Bonnard, C., Tacher, L., and Beniston, M.: Prediction of landslide movements caused by climate change: Modelling the behaviour of a mean elevation large slide in the Alps and assessing its uncertainties, in: Landslides and Engineered Slopes. From the Past to the Future, edited by: Chen, Z., Zhang, J.-M., Ho, K., Wu, F.-Q., and Li, Z.-K., CRC Press, London, https://doi.org/10.1201/9780203885284-17, 217–227, 2008.
Brenning, A.: Spatial prediction models for landslide hazards: review, comparison and evaluation, Nat. Hazards Earth Syst. Sci., 5, 853–862, https://doi.org/10.5194/nhess-5-853-2005, 2005.
Buma, J. and Dehn, M.: A method for predicting the impact of climate change on slope stability, Environ. Geol., 35, 190–196, https://doi.org/10.1007/s002540050305, 1998.
Buma, J. and Dehn, M.: Impact of climate change on a landslide in South East France, simulated using different GCM scenarios and downscaling methods for local precipitation, Clim. Res., 15, 69–81, https://doi.org/10.3354/cr015069, 2000.
Cervi, F., Berti, M., Borgatti, L., Ronchetti, F., Manenti, F., and Corsini, A.: Comparing predictive capability of statistical and deterministic methods for landslide susceptibility mapping: a case study in the northern Apennines (Reggio Emilia Province, Italy), Landslides, 7, 433–444, 2010.
Chang, S.-H. and Chiang, K.-T.: The potential impact of climate change on typhoon triggered landslides in Taiwan, 2010–2099, Geomorphology, 133, 143–151, https://doi.org/10.1016/j.geomorph.2010.12.028, 2011.
Chung, C. F. and Fabbri, A. G.: Validation of spatial prediction models for landslide hazard mapping, Nat. Hazards,30, 451–472, https://doi.org/10.1023/B:NHAZ.0000007172.62651.2b, 2003.
Ciabatta, L., Camici, S., Brocca, L., Ponziani, F., Stelluti, F., Berni, N., and Moramarco, T.: Assessing the impact of climate-change scenarios on landslide occurrence in Umbria Region, Italy, J. Hydrol., https://doi.org/10.1016/j.jhydrol.2016.02.007, 2016.
Ciurleo, M., Cascini, L., and Calvello, M.: A comparison of statistical and deterministic methods for shallow landslide susceptibility zoning in clayey soils, Eng. Geol., 223, 71–81, 2017.
Coe, J. A.: Regional moisture balance control of landslide motion: implications for landslide forecasting in a changing climate, Geology, 40, 323–326, https://doi.org/10.1130/G32897.1, 2012.
Collison, A., Wade, S., Griffiths, J., and Dehn, M.: Modelling the impact of predicted climate change on landslide frequency and magnitude in SE England, Eng. Geol., 5, 205–218, https://doi.org/10.1016/s0013-7952(99)00121-0, 2000.
Comegna, L., Picarelli, L., Bucchignani, E., and Mercogliano, P.: Potential effects of incoming climate changes on the behaviour of slow active landslides in clay, Landslides, 10, 373–391, https://doi.org/10.1007/s10346-012-0339-3, 2013.
Corominas, J., van Westen, C., Frattini, P., Cascini, L., Malet, J.-P., Fotopoulou, S., Catani, F., Van Den Eeckhaut, M., Mavrouli, O., Agliardi, F., Pitilakis, K., Winter, M. G., Pastor, M., Ferlisi, S., Tofani, V., Hervás, J., and Smith, J. T.: Recommendations for the quantitative analysis of landslide risk, B. Eng. Geol. Environ., 73, 209, https://doi.org/10.1007/s10064-013-0538-8, 2014.
Crow, P.: The influence of soils and species on tree root depth, Forestry Commission, Edinburgh, Information Note FCINO78, 8 pp., 2005.
Crozier, M. J.: Deciphering the effect of climate change on landslide activity: a review, Geomorphology 124, 260–267. https://doi.org/10.1016/j.geomorph.2010.04.009, 2010.
Cruden, D. M. and Varnes, D. J.: Landslides: investigation and mitigation. Chapter 3-Landslide types and processes, Transportation research board special report, 247, 1996.
Davis, J. and Blesius, L.: A hybrid physical and maximum-entropy landslide susceptibility model, Entropy, 17, 4271–4292, 2015.
De Crécy, L.: L'histoire de la RTM: quelques réflexions d'un praticien, Revue géographique des Pyrénées et du Sud-Ouest, 59, 16–28, https://doi.org/10.3406/rgpso.1988.3103, 1988 (in French).
Dehn, M. and Buma, J.: Modelling future landslide activity based on general circulation models, Geomorphology, 30, 175–187, https://doi.org/10.1016/s0169-555x(99)00053-7, 1999.
DRIAS: Drias les futurs du climat, projections climatiques pour l'adaptation de nos sociétés; Simulations de Jouzel-2014, données Météo-France, CNRM-GAME, CERFACS, IPSL, available at: http://www.drias-climat.fr/ (last access: 28 January 2015), 2014.
Fabre, R., Lebourg, T., and Clément, B.: Typologie et modèles de glissements de terrain: exemples de sites des Pyrénées occidentales et centrales, Rev. Fr. Geotech., 99, 35–48, https://doi.org/10.1051/geotech/2002099035, 2002 (in French).
Fan, J. C., Yang, C. H., Chang, S. C., Huang, H. Y., and Guo, J. J.: Effects of climate change on the potential of the landslides in the basin of Kaoping stream, J. Chin. Soil Water Conserv., 44, 335–350, 2013.
Galve, J. P., Cevasco, A., Brandolini, P., and Soldati, M.: Assessment of shallow landslide risk mitigation measures based on land use planning through probabilistic modelling, Landslides, 12, 101–114, 2015.
Galve, J. P., Bartelletti, C., Notti, D., Fernández-Chacón, F., Barsanti, M., Azañón, J. M., Pérez-Peña, V., Giannecchini, R., Avanzi, G. D. A., Galanti, Y., and Lamas, F. J.: Deterministic and Probabilistic Slope Stability Models Forecast Performance at 1:5000-Scal, Workshop on World Landslide Forum, Springer, Cham, 741–774, 2017.
Gariano, S. L. and Guzzetti, F.: Landslides in a changing climate, Earth Sci. Rev., 162, 227–252, 2016.
Gariano, S. L., Rianna, G., Petrucci, O., and Guzzetti, F.: Assessing future changes in the occurrence of rainfall-induced landslides at a regional scale, Sci. Total Environ., 596–597, 417–426, https://doi.org/10.1016/j.scitotenv.2017.03.103, 2017.
Gariano, S. L., Petrucci, O., Rianna, G., Santini, M., and Guzzetti, F.: Impacts of past and future land changes on landslides in southern Italy, Reg. Environ. Change, 18, 437–449, https://doi.org/10.1007/s10113-017-1210-9, 2018.
Gassner, C., Promper, C., Begueria, S., and Glade, T.: Climate change impact for spatial landslide susceptibility, in: Engineering Geology for Society and Territory Climate Change and Engineering Geology 1, edited by: Lollino, G., Manconi, A., Clague, J., Shan, W., and Chiarle, M., Springer International Publishing, 429–433, 2015.
Genet, M., Stoke, A., Fourcaud, T., and Norris, J. E.: The influence of plant diversity on slope stability in a moist evergreen deciduous forest, Ecol. Eng., 36, 265–275, 2010.
Glade, T.: Landslide occurrence as a response to land use change: a review of evidence from New Zealand, Catena, 51, 297–314, 2003.
Grandjean, G., Thomas, L., Bernardie, S., and the SAMCO Team: A Novel Multi-Risk Assessment Web-Tool for Evaluating Future Impacts of Global Change in Mountainous Areas, Climate, 6, 92, https://doi.org/10.3390/cli6040092, 2018.
Gruber, M.: Les relations climat-végétation dans les Pyrénées centrales françaises, Acta Botanica Malacitana, 16, 405–415, 1991 (in French).
Guzzetti, F., Reichenbach, P., Ardizzone, F., Cardinali, M., and Galli, M.: Estimating the quality of landslide susceptibility models, Geomorphology, 81, 166–184, https://doi.org/10.1016/j.geomorph.2006.04.007, 2006.
Houet, T., Gremont, M., Vacquié, L., Forget, Y., Marriotti, A., Puissant, A., Bernardie, S., Thiery, Y., Vandromme, R., and Grandjean, G.: Downscaling scenarios of future land use and land cover changes using a participatory approach: an application to mountain risk assessment in the Pyrenees (France), Reg. Environ. Change, 17, 2293–2307, https://doi.org/10.1007/s10113-017-1171-z, 2017.
Huber, U. M., Bugmann, H. K. M., and Reasoner, M. A.: Global Change and Mountain Regions: An Overview of Current Knowledge, in: Advances in Global Change Research, Springer, Berlin, 650 pp., 2005.
IPCC: Climate Change 2007: The Physical Science Basis. Contribution of Working Group I to the Fourth Assessment Report of the Intergovernmental Panel on Climate Change, edited by: Solomon, S., Qin, D., Manning, M., Chen, Z., Marquis, M., Averyt, K. B., Tignor, M., and Miller, H. L., Cambridge University Press, Cambridge, UK and New York, USA, 2007.
IPCC: Intergovernmental Panel on Climate Change: Climate Change 2014: Synthesis Report. Contribution of Working Groups I, II and III to the Fifth Assessment Report of the Intergovernmental Panel on Climate Change, Geneva, Switzerland, 151 pp., 2014.
Jakob, M. and Lambert, S.: Climate change effects on landslides along the southwest coast of British Columbia, Geomorphology, 107, 275–284, https://doi.org/10.1016/j.geomorph.2008.12.009, 2009.
Ji, J., Kokutse N. K., Genet M., Fourcaud T., and Zhang Z. Q.: Effect of spatial variation of tree root characteristics on slope stability. A case study on Black Locust (Robinia pseudoacacia) and Arborvitae (Platycladus orientalis) stands on the Loess Plateau, China, Catena, 92, 139–154, 2012.
Jomelli, V., Brunstein, D., Déqué, M., Vrac, M., and Grancher, D.: Impacts of future climatic change (2070–2099) on the potential occurrence of debris flows: a case study in the Massif des Ecrins (French Alps), Clim. Change, 97, 171–191, https://doi.org/10.1007/s10584-009-9616-0, 2009.
Kim, K. G., Lee, D. K., Park, C., Kil, S., Son, Y., and Park, J. H.: Evaluating landslide hazards using RCP 4.5 and 8.5 scenarios, Environ. Earth Sci., 73, 1385–1400, https://doi.org/10.1007/s12665-014-3775-7, 2015.
Kirsten, H. A..: Significance of the probability of failure in slope engineering, Civ. Eng., 25, 17–27, 1983.
Kohler, T. and Maselli, D.: Mountains and Climate Change – From Understanding to Action, published by Geographica Bernensia with the support of the Swiss Agency for Development and Cooperation (SDC), and an international team of contributors, Bern, 2009.
Kokutse, N. K., Temgoua, A. G. T., and Kavazović, Z.: Slope stability and vegetation: Conceptual and numerical investigation of mechanical effects, Ecol. Eng., 86, 146–153, https://doi.org/10.1016/j.ecoleng.2015.11.005, 2016.
Lebourg, T., Fabre, R., Clement, B., and Frappa, M.: High-mountain landslides in the Atlantic Pyrenees: their relationship with the geology and geomorphology, B. Eng. Geol. Environ., 62, 221–223, https://doi.org/10.1007/s10064-002-0180-3, 2003a.
Lebourg, T., Riss, J., Fabre, R., and Clément, B.: Morphological characteristics of till formations in relation with mechanical parameters, Math. Geol., 35, 835–852, 2003b.
Lee, M.-J., Song, W.-K., Won, J.-S., Park, I., and Lee, S.: Spatial and temporal change in landslide hazard by future climate change scenarios using probabilistic-based frequency ratio model, Geocarto Int., 29, 639–662, https://doi.org/10.1080/10106049.2013.826739, 2014.
McInnes, R., Jakeways, J., Fairbank, H., and Mathie, E.: Landslides and Climate Change: Challenges and Solutions, in: Proceedings of the International Conference on Landslides and Climate Change, Ventnor, Isle of Wight, UK, 21–24 May 2007, Taylor & Francis, London, https://doi.org/10.1201/noe0415443180, 2007.
Melchiorre, C. and Frattini, P.: Modelling probability of rainfall-induced shallow landslides in a changing climate, Otta, Central Norway. Clim. Change, 113, 413–436. https://doi.org/10.1007/s10584-011-0325-0, 2012.
Morgenstern, N. R. and Price, V. E.: The analysis of the stability of general slip surfaces, Geotechnique, 15, 79–93, 1965.
Morgenstern, R. and Price, V. E.: A numerical method for solving the equations of stability of general slip surfaces, Comput. J., 9, 388–393, 1967.
Moore, R., Carey, J. M., Mc Innes, R. G., and Houghton, J. E. M.: Climate change, so what? Implications for ground movement and landslide event frequency in the Ventor Undercliff, Isle of Wight, in: Landslides and Climate Change: Challenges and Solutions, in: Proceedings of the International Conference on Landslides and Climate Change, Isle of Wight, UK, 21–24 May 2007, edited by: McInnes, R., Jakeways, J., Fairbank, H., Mathie, E., 335–345, 2007.
Norris J. E., Stokes A., Mickovski S. B., Cammeraat E., van Beek L. P. H, Nicoll B., and Achim, A.: Slope stability and erosion control: ecotechnological solutions, Springer, Dortmund, 2008.
Oliveira, S. C., Zêzere, J. L., Lajas, S., and Melo, R.: Combination of statistical and physically based methods to assess shallow slide susceptibility at the basin scale, Nat. Hazards Earth Syst. Sci., 17, 1091–1109, https://doi.org/10.5194/nhess-17-1091-2017, 2017.
OPCC-CTP: Climate change in the Pyrenees: impacts, vulnerability and adaptation Bases of knowledge for the future adaptation strategy of the Pyrenees, ISBN: 978-84-09-06268-3, 2018.
Pack, R. T.: Assessing Terrain Stability in a GIS using SINMAP, 15th Annual GIS Conference, GIS 2001, p. 9., 2001.
Pack, R. T., Tarboton, D. G., and Goodwin, C. N.: Assessing terrain stability in a GIS using SINMAP, 15th Annual GIS Conference, GIS 2001, Vancouver, p. 9., 2001.
Peres, D. J. and Cancelliere, A.: Modeling impacts of climate change on return period of landslide triggering, J. Hydrol., 567, 420–434, https://doi.org/10.1016/j.jhydrol.2018.10.036, 2018.
Persichillo, M. G., Bordoni, M., and Meisina, C.: The role of land use changes in the distribution of shallow landslides, Sci. Total Environ., 574, 924–937, https://doi.org/10.1016/j.scitotenv.2016.09.125, 2017.
Pisano, L., Zumpano, V., Malek, Ž., Rosskopf, C. M., and Parise, M.: Variations in the susceptibility to landslides, as a consequence of land cover changes: A look to the past, and another towards the future, Sci. Total Environ., 601–602, 1147–1159, https://doi.org/10.1016/j.scitotenv.2017.05.231, 2017.
Promper, C., Puissant, A., Malet, J.-P., and Glade, T.: Analysis of land cover changes in the past and the future as contribution to landslide risk scenarios, Appl. Geogr., 53, 11–19, https://doi.org/10.1016/j.apgeog.2014.05.020, 2014.
Reichenbach, P., Mondini, A. C., and Rossi, M.: The influence of land use change on landslide susceptibility zonation: the Briga catchment test site (Messina, Italy), Environ. Manage., 54, 1372–1384, https://doi.org/10.1007/s00267-014-0357-0, 2014.
Remondo, J., González-Díez, A., Soto, J., Díaz de Terán, J. R., and Cendrero, A.: Human impact on geomorphic processes and hazards in mountain areas, Geomorphology, 66, 69–84, 2005.
Rianna, G., Zollo, A. L., Tommasi, P., Paciucci, M., Comegna, L., and Mercogliano, P.: Evaluation of the effects of climate changes on landslide activity of Orvieto clayey slope, Proc. Earth Plan. Sci., 9, 54–63, https://doi.org/10.1016/j.proeps.2014.06.017, 2014.
Rianna, G., Reder, A., Mercogliano, P., and Pagano, L.: Evaluation of Variations in Frequency of Landslide Events Affecting Pyroclastic Covers in Campania Region under the Effect of Climate Changes, Hydrology, 4, 34, https://doi.org/10.3390/hydrology4030034, 2017.
Robinson, J. D., Vahedifard, F., and AghaKouchak, A.: Rainfall-triggered slope instabilities under a changing climate:comparative study using historical and projected precipitation extremes, Can. Geotech. J., 54, 117–127, https://doi.org/10.1139/cgj-2015-0602, 2017.
Sangelantoni, L., Gioia, E., and Marincioni, F.: Impact of climate change on landslides frequency: the Esino river basin case study (Central Italy), Nat. Hazards, 93, 849–884, https://doi.org/10.1007/s11069-018-3328-6, 2018.
Schiechtl, H. M.: Bioengineering for land reclamation and conservation, Univ. of Alberta Press, Edmonton, Canada, 1980.
Sedan, O., Desramaut, N., and Vandromme, R.: Logiciel ALICE version 7-Guide d'utilisateur, BRGM, RP-60004, 2013 (in French).
Shou, K. J. and Yang, C. M.: Predictive analysis of landslide susceptibility under climate change conditions – a study on the Chingshui River Watershed of Taiwan, Eng. Geol., 192, 46–62, https://doi.org/10.1016/j.enggeo.2015.03.012, 2015.
Stoffel, M., Tiranti, D., and Huggel, C.: Climate change impacts on mass movements – case studies from the European Alps, Sci. Total Environ., 493, 1255–1266, https://doi.org/10.1016/j.scitotenv.2014.02.102, 2014.
Tacher, L. and Bonnard, C.: Hydromechanical modelling of a large landslide considering climate change conditions, in: Landslides and Climate Change: Challenges and Solutions Proceedings of the International Conference on Landslides and Climate Change, Ventnor, Isle of Wight, UK, 21–24 May 2007, edited by: McInnes, R., Jakeways, J., Fairbank, H., and Mathie, E., Taylor & Francis, London, 131–141, 2007.
Thiéry, D.: Logiciel GARDÉNIA version 6.0 – Guide d'utilisation. BRGM report, RP-52832-FR, 104 pp., 2003 (in French).
Thiery, Y., Malet, J.-P., Sterlacchini, S., Puissant, A., and Maquaire, O.: Landslide susceptibility assessment by bivariate methods at large scales: application to a complex mountainous environment, Geomorphology, 9, 38–59, 2007.
Thiery, Y., Vandromme R., Maquaire O., and Bernardie S.: Landslide Susceptibility Assessment by EPBM (Expert Physically Based Model): Strategy of Calibration in Complex Environment, in: Advancing Culture of Living with Landslides, https://doi.org/10.1007/978-3-319-53498-5_104, 917–926, 2017.
Turkington, T., Remaître, A., Ettema, J., Hussin, H., and van Westen, C.: Assessing debris flow activity in a changing climate, Clim. Change, 137, 293–305, https://doi.org/10.1007/s10584-016-1657-6, 2016.
Vanacker, V., Vanderschaeghe, M., Govers, G., Willems, E., Poesen, J., Deckers, J., and De Bievre, B.: Linking hydrological, infinite slope stability and land-use change models through GIS for assessing the impact of deforestation on slope stability in high Andean watersheds, Geomorphology, 52, 299–315, 2003.
Van Beek, L. P. H. and Van Asch, T. W.: Regional assessment of the effects of land-use change on landslide hazard by means of physically based modelling, Nat. Hazards, 31, 289–304, 2004.
Vandromme, R., Desramaut, N., Garnier, C., and Bernardie, S.: A novel approach to integrate effects of vegetation changes on slope stability, in: Lollino, G., Giordan, D., Crosta, G., Corominas, J., Azzam, R., Wasowski, J., and Sciarra, N., Engineering geology for society and territory, Springer, 2, 975–978, 2015.
Van Westen, C. J., Van Asch, T. W. J., and Soeters, R.: Landslide hazard and risk zonation - Why is it still so difficult?, B. Eng. Geol. Environ., 65, 167–184, https://doi.org/10.1007/s10064-005-0023-0, 2006.
Van Westen, C. J., Castellanos, E., and Kuriakose, S. L.: Spatial data for landslide susceptibility, hazard, and vulnerability assessment: An overview, Eng. Geol., 102, 112–131, https://doi.org/10.1016/j.enggeo.2008.03.010, 2008.
Varnes, D. J.: Slope Movement Types and Processes, in Special Report 176: LAndslides: Analysis and Control, edited by Schuster, R. L. and Krizek, R. J. National Research Council, Washington, D.C., USA, 11-33, 1978.
Varnes, D. J. and COMMISSION ON LANDSLIDES AND OTHER MASS MOVEMENTS: Landslide hazard zonation: a review of principles and practise, in: B. Int. Assoc. Eng. Geol., 23, 13–14, https://doi.org/10.1007/BF02594720, 1984.
Vandromme, R., Thiery, Y., Bernardie, S., and Sedan O.: ALICE (Assessment of Landslides induced by Climatic Events): a single tool to integrate shallow and deep landslides for susceptibility and hazard assessment, Geomorphology, 367, 107307, https://doi.org/10.1016/j.geomorph.2020.107307, 2020.
Viers, G.: Climat Et Climats Des Pyrénées Orientales, Ann. Geogr., 96, 339–343, 1987.
Villani, V., Rianna, G., Mercogliano, P., and Zollo, A. L.: Statistical approaches versus weather generator to downscale RCM outputs to slope scale for stability assessment: a comparison of performances, Electron. J. Geotech. Eng., 20, 1495–1515, 2015.
Wieczorek, G. F.: Preparing a detailed landslide-inventory map for hazard evaluation and reduction, B. Assoc. Eng. Geol., 21, 337–342, 1984.
Winter, M. G. and Shearer, B.: Climate change and landslide hazard and risk in Scotland, in: Engineering Geology for Society and Territory Climate Change and Engineering Geology, edited by: Lollino, G., Manconi, A., Clague, J., Shan, W., and Chiarle, M., Springer International Publishing, 1, 411–414, 2015.
World Meteorological Organization (WMO): General Meteorological Standards and Recommended Practices, Technical regulations, WMO-no. 49, Vol. 1, ISBN: 978-92-63-10049-8, 2018.
Wu, T. H., McKineel, W. P., and Swanston, D. N.: Strength of the tree roots and landslides on Prince of Wales Island, Alaska, Can. Geotech. J., 16, 19–33, 1979.
Wu, X., Chen, X., Zhan, F. B., and Hong, S.: Global research trends in landslides during 1991–2014: a bibliometric analysis, Landslides, 12, 1215–1226, https://doi.org/10.1007/s10346-015-0624-z, 2015.
Zizioli, D., Meisina, C., Valentino, R., and Montrasio, L.: Comparison between different approaches to modeling shallow landslide susceptibility: a case history in Oltrepo Pavese, Northern Italy, Nat. Hazards Earth Syst. Sci., 13, 559–573, https://doi.org/10.5194/nhess-13-559-2013, 2013.