the Creative Commons Attribution 4.0 License.
the Creative Commons Attribution 4.0 License.
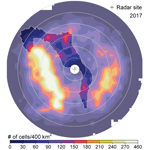
Radar-derived convective storms' climatology for the Prut River basin: 2003–2017
Sorin Burcea
Roxana Cică
Roxana Bojariu
Weather radar measurements are used to study the climatology of convective storms and their characteristics in the transboundary Prut River basin. The Storm Cell Identification and Tracking (SCIT) algorithm was used to process the volumetric reflectivity measurements, in order to identify, characterize, and track the convective storm cells. The storm attribute table output of the algorithm was used to separate the convective from the stratiform storm cells, by applying a simple selection criterion based on the average vertically integrated liquid (VIL) values. The radar-derived characteristics of convective storms were used to document the spatial and temporal distributions and storm properties in terms of duration, distance travelled, movement direction, and intensity. The results show that 94.3 % of all convective storm cells were detected during May–August, with the peak in July. The peak time for convective storm cells' occurrence was in the afternoon and evening hours between 10:00 and 18:00 UTC. The median duration of a convective storm was 42 min, the median distance travelled was 23 km, and the median movement speed was 7.7 m s−1. The average movement of storms varied with months, but overall most convective storms move from the south-west and south–south-east. Also, the analysis shows that the longer-lasting convective storms were the most intense. The spatial distribution of the convective cells reveals yearly variation patterns and hotspots but also highlights the limitations of radar measurement at longer distances. Reanalysis data suggest that low values of sea level pressure over the Black Sea can act as a dynamical driver of convective storms in the analysed area.
- Article
(9478 KB) - Full-text XML
- BibTeX
- EndNote
Weather events associated with convective storms have a large impact on society and natural systems and can lead to loss of life and property. For instance, large rivers or small catchment flash floods can cause landslides, while urban areas can be heavily affected if the capacity of the sewer systems is exceeded. During the past decades, heavy rainfall has led to loss of life and caused significant damage in various areas of Romania. To mitigate the effects of such hazards and to improve the local adaptation to climate-change-related natural hazards by increasing the awareness and preparedness of both individuals and stakeholders, knowledge about the spatio-temporal distribution of convective storms is of the utmost importance.
A specific challenge is the high variability in time and space of convective weather. Also, regional variations in topography, local-scale flow, and mesoscale flow can also significantly affect the convective storms' development. Consequently, high-resolution spatio-temporal datasets are needed to perform detailed statistics.
Remote sensing of the atmosphere is nowadays performed on a large scale, with instruments like ground-based weather radars providing high-resolution observations of weather systems. Weather radar measurements represent a valuable supplement for thunderstorm and convective events' climatology and mapping (Peter et al., 2015; Goudenhoofdt and Delobbe, 2013). Thunderstorm identification and tracking using weather radars are a key tool for severe weather forecasting and warning and have become, lately, an asset for convective storms' climatology as well. Compared to point measurements (i.e. weather stations), the intensity, swath, and spatial extent of the convective events can be derived from radar data.
Lately, based on weather radar data, studies on the statistical characteristics of convective storms around the world have been performed. For instance, thunderstorm climatology in Hungary, using Doppler radar data, has been developed by Seres and Horvath (2015). The study was performed over the period 2004–2012, finding that most storm days occurred in the late spring and summer of 2007 and 2010, with the daily frequencies peaking in the late afternoon. Statistical characteristics of convective storms in Belgium were studied by Goudenhoofdt and Delobbe (2013), using volumetric weather radar observations over a 10-year period (2002–2011). Their main findings can be summarized to the fact that the probability to observe a high number of storms reaches a maximum in June and in the early afternoon, and the motion of convective storms is slower in summer and in the afternoon, while regions with slightly higher convective initiation are related to orography. Over north-western Italy, a 6-year warm season (2005–2010, April–September) radar-based analysis of convective storms revealed that storms are more frequent and intense in July and August and during the afternoon hours (Davini et al., 2012). Also, the spatial distribution of convective initiation highlights that the most suitable regions for the generation of convective cells are located in the proximity of hills or mountains, suggesting an active role of the topography in triggering storms.
In Asia, Wang et al. (2014) studied the statistical characteristics of convective initiation in the Beijing–Tianjin region, using radar data over the period 2008–2013. Results showed that there are dense convective initiation activities around 200 m elevation, meaning that convective storms are more easily triggered over foothills; the highest convective initiation density was found in urban areas, while the second highest convective initiation density was found in the forest-type land cover areas. Radar-derived statistics of convective storms and their concomitant changes with thermodynamic variability in South-east Queensland, Australia, was performed by Peter et al. (2015), concluding that convective storms are found to form and maintain along elevated topography, westerly regime storms occur less frequently and have shorter lifetimes, and westerly regime storms are primarily driven by large-scale forcing, whereas northerly and trade wind regime storms are more responsive to surface characteristics. Mohee and Miller (2010) derived a climatology of thunderstorms for North Dakota, using radar and surface thunderstorm data over the 2002–2006 period. It was found that convective storm cells peak in June and July and in the late afternoon to early morning. Also, their results reveal that the average movement of storm cells varied with months, and the storms moved toward the north, the north-east, and the east.
For Romania, radar-based convective storms' identification, observation, and their associated damage were also studied but considering only particular storm events or small periods of time. For example, assessing the severe hailstorm and hail risk of a total of 52 hail events that occurred in May 2013 in southern Romania, using weather radar data, Cică et al. (2015) found that the areas where hail and damage were reported are well captured by the footprints and magnitude of the radar variables considered in the study. Severe weather situations that occurred in south-eastern Romania during 2003–2007 were investigated by Cărbunaru et al. (2013), using weather radar data, their results revealing various Doppler velocity field configurations associated with the intensity of the tropospheric flow, while each configuration of Doppler velocity field was found to be associated with various characteristics of severe convective cells. Lemon et al. (2003) document the strong, long-track tornado (F3+) that occurred in August 2003, in south-eastern Romania, relying on weather radar data for storm characteristics' assessment.
Therefore, even some recent studies on convection and the associated weather events in Romania have been performed, but catchment- to regional-scale radar-based statistics and mapping of convective storms were not derived. The aim of this paper is to derive a climatology of convective storms in the area of Prut River basin, based on a 15-year weather radar dataset, to better understand the spatio-temporal characteristics of convective storms that occurred in this particular area, which includes the second largest water reservoir in Romania (at the Stânca-Costeşti hydropower station). In the summer of 2008, this reservoir reached 98 % of its capacity due to a historical flow, which was generated by high amounts of rainfall recorded in the Prut River basin (Romanescu et al., 2011). A similar situation happened in the summer of 2010 (Romanescu and Stoleriu, 2017), raising the issue of better monitoring and understanding the climate variability of convective systems in the Prut area. Hopefully, the outcomes of our study will contribute to the assessment of precipitation-related hazards and associated risks in the context of basin-integrated water management and to the climatology of storms in Europe.
This article is structured as follows. The study area and datasets are described in Sect. 2, and the methodology is presented in Sect. 3. Results are detailed within Sect. 4, followed by the concluding remarks in Sect. 5.
The Prut River begins from the forested Carpathian Mountains in Ukraine and flows into the Danube River, in Romania. The Prut River is 967 km long, and its catchment area is 27 540 km2, with an overall drop of the river of approximately 1500 m. The basin of the Prut River is transboundary and is located in the territory of three countries: Ukraine, Republic of Moldova, and Romania, with corresponding area percentages of 33 %, 28 %, and 39 % respectively (Fig. 1). On the Prut River, the Stânca-Costeşti Dam (located between Stânca, Romania, and Costeşti, Republic of Moldova) was built in the 1970s for the lake to serve as a reservoir for a hydropower station. However, the main goal of building the dam was to protect villages down the river from floods.
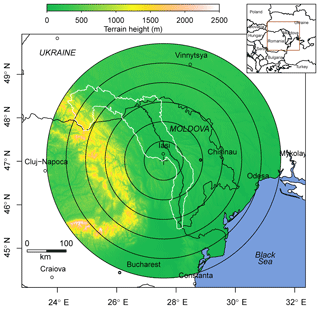
Figure 1Map of the study area, showing the Prut River basin (white polygon), Bârnova WSR-98D radar location (“+” sign), orography, and borders. The range rings around the radar are 50 km apart, extending to 300 km.
The Prut River basin is covered by the Weather Surveillance Radar – 98 Doppler (WSR-98D) located at Bârnova, Iaşi County, Romania (the “+” sign in Fig. 1). The radar is a single-polarization S-band system, operating Nexrad Volume Coverage Pattern 21 (VCP21), performing a complete volume scan every 6 min at nine different elevation angles. Small parts of the basin, in the south and north-west, are covered by another two WSR-98D radars, but the distance from the radar location to these areas exceeds 200 km. Hence, only data from Bârnova WSR-98D were chosen for this study.
The data used in this study are the output of the Storm Cell Identification and Tracking (SCIT) algorithm (Johnson et al., 1998), covering the period 2003–2017. The SCIT algorithm is a centroid-based storm cell algorithm that processes volumetric reflectivity to identify, characterize, track, and forecast the short-term movement of storm cells identified in the coverage area of the weather radar. The algorithm uses seven reflectivity thresholds (30, 35, 40, 45,50, 55, 60 dBZ) and a series of adaptable parameters to identify the storm cell centroid. The SCIT runs operationally after each volume scan, resulting in tabular, alphanumeric, and graphic products. In this study, the storm attribute table was used, a table that contains storm features like storm ID, azimuth and range, cell movement direction and speed, and maximum reflectivity.
Although the analysis performed by Johnson et al. (1998) revealed that SCIT detected more than 90 % of all storm cells correctly, the algorithm has limitations. The most obvious among these is poor cell detection close to the radar site because of the cone of silence and at greater distances because of low vertical resolution (beam spreading). Nevertheless, it is beyond the scope of this paper to perform an evaluation of the SCIT algorithm.
The radar data covering the 15-year period considered in this study were extracted from the archive, which starts on 1 May 2003, and then processed in several steps. For each of the 15 years, the days with missing data were identified. The missing days are due to both technical failures of the radar system or to maintenance intervals. One may assume that there is no convective activity during the maintenance intervals, as the radar was shut down for maintenance during the days with clear sky. Also, if during a day more than 10 % of data were missing (∼2 h), then the day was flagged as missing and excluded from the analysis.
The number of days with valid radar data, selected using the above criterion, within each year, is illustrated in Fig. 2. The year 2003 has the smallest number of selected days, as the radar data were available starting from May. The number of selected days per year varies for the interval 2004–2017, being approximately constant for the last 3 years of the period. Thus, from the total number of days excluded from the analysis (13.7 % from all calendar days), 3.9 % are days with no data, and 9.8 % are days with more than 10 % data missing. After this step, 4624 days were chosen for the analysis, with approximately 1.1 million scans processed to extract the storm attribute tables.
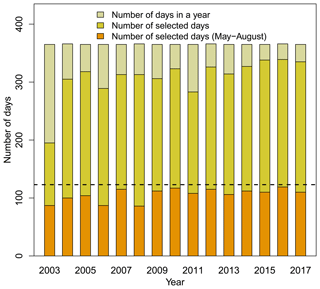
Figure 2Number of days per year, within the period 2003–2017, with valid data selected for the analysis. The number of selected days between May and August is also depicted, the dotted line representing the number of days (i.e. 123 days) between 1 May and 31 August.
After extraction of the storm attribute tables, the storm cell selection was performed. The performance of the SCIT algorithm is affected by the distance to the radar; storms located at close ranges are likely to be missed because of the cone of silence, while at greater distances only storms characterized by large vertical development will be identified. To have a full coverage of the Prut River basin, storm cells located within the 20–300 km range interval were kept for further processing, even though according to Johnson et al. (1998) there is some indication that storms located more than 150 km from the radar site are more likely to be missed.
After the above selection criteria were applied, a total number of just over 2.2 million storm cells resulted, for the whole study period and for the whole radar coverage area within 20–300 km from the radar. Given the azimuth and range of each of the storm cells detected, the geographical coordinates (i.e. longitude and latitude) were calculated and included in the storm attribute table to facilitate the mapping process and the extraction of the storm cells within the Prut River basin area. After extracting the storm cells detected within the Prut River basin, a number of around 336 000 entries resulted.
In order to keep only the convective storm cells, further selection criteria were applied. When SCIT identifies a storm cell for the first time, a unique name (ID) consisting of letter–number combinations is assigned (e.g. A0), and for the whole time the algorithm detects and tracks that particular ID, and the storm attribute table is updated. So, during its lifetime, a unique storm has associated multiple storm cells which are found under the same ID within the table. By looking at the storm attribute tables, we noticed that the algorithm identifies many storms during one or a few consecutive volume scans. According to the American Meteorological Society glossary (2018), ordinary convective cells last from 20 to 30 min but often more when forming other types of convective storms (e.g. multi-cell). To exclude the underdeveloped and fast decaying cells, the condition that a unique storm had a lifetime of at least approximately 20 min was applied as a further selection criterion. For this, all the unique IDs were identified in the attribute tables, and it was checked if the storm was identified in at least four consecutive scans. To ensure that all the storms within the Prut River basin are captured well, the storms that initiated outside the basin, but during their lifetime at least four consecutive scans were detected inside the basin, were retained for analysis.
Based on the cell vertically integrated liquid (VIL) (Greene and Clark, 1972), a supplemental selection was performed to get the final convective storms attribute tables. The VIL (kg m−2) output of SCIT is the radar estimate of the total amount of liquid in the column, for a given storm cell, and this parameter is correlated with updraft strength. Zhang and Qi (2010) used a single VIL threshold of 6.5 kg m−2, found through subjective analysis of a large number of cases, to separate convective from stratiform rainfall, while Qi et al. (2013) used the same VIL threshold but within a more complex convective–stratiform segregation algorithm. Within this study, a VIL threshold of 10 kg m−2 was used to separate convective from stratiform storm cells. This value was obtained also through subjective analysis, by arbitrarily checking the storm cell characteristics from different seasons and years. Smaller values of VIL were observed for stratiform cells, shallow isolated single cells, and for high reflectivity cores (> 45 dBZ) within stratiform precipitation systems, where the radar beam intersects the bright band region, while greater values of VIL (> 10 kg m−2) were observed for convective storm cells. Therefore, if for a unique storm (ID), the average VIL value for its entire lifetime was greater than or equal to the threshold value, the storm was retained for analysis, and if the average VIL value was smaller than the threshold value, the storm was discarded. Consequently, a final number of 9507 unique convective storms associated with just over 84 000 storm cells were considered for the analysis for the area of the Prut River basin and during 2003–2017.
The mapping of the spatial distribution of the convective storm cells was performed on a 20 km resolution grid, by counting the number of convective storm centroids within each grid cell. The monthly (hourly) convective cells' frequency is equal to the average detections in a given month (hour) over the whole 15-year period. Based on the final convective storm attribute table, 2-D histograms comparing radar-derived storm attribute speed, direction of movement, and day of the convective season were obtained. For each day, and for each direction (i.e. from 1 to 360∘), the number of convective storm cells was counted and plotted on a 2-D histogram of 10∘ × 3 d resolution. A direction of 180∘ (plotted as south) represents a convective storm cell moving from south to north. The storm speed as a function of the direction of movement was plotted on a 2-D histogram of 5 knots × 10∘ resolution, illustrating the number of convective storm cells moving with a certain speed in a given direction.
In addition, the mapping of the spatial distribution of the convective storm cells was also performed on a 10 km resolution grid to match the gridded precipitation data over the Prut basin, for comparison purposes. We correlated the monthly number of convective systems in the river basin with the basin-averaged precipitation amounts. Then, we analysed the correlation coefficients linking the monthly number of convective systems in the Prut basin with sea level pressure (SLP), convective available potential energy (CAPE), and total column water to identify the large-scale drivers of storminess in the area of interest. SLP, CAPE, and total column water were extracted from the ERA 5 reanalysis product (Copernicus Climate Change Service, 2017) to cover the Atlantic–European sector.
4.1 Yearly, monthly, and hourly distribution
The annual distribution of the number of convective storm cells detected by the weather radar, within the Prut River basin area, from 2003 to 2017, is depicted in Fig. 3, revealing an inter-annual variation. However, no clear trend is observable, but there is a small decrease of the number of convective cells during 2014–2015, followed by an abrupt increase in 2016 when the largest number of convective cells (9766) was detected. The smallest number of cells (2746) corresponds to year 2003, and even if the radar data were available starting from May, we speculate that this number would be representable of this particular year.
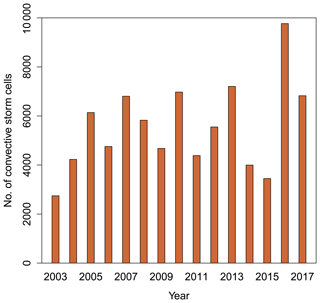
Figure 3Annual distribution of the number of convective storm cells in the Prut River basin, for the period 2003–2017.
The monthly variation of the mean number of the convective storm cells (Fig. 4) detected within the Prut River basin area, during the 15-year period, reveals that convection occurs predominantly during the warm season, the frequency being extremely low in the cold season. The convection prevails during May–August, when 94.3 % of the total number of convective storms cells were detected, reaching a maximum in July (30.7 % of the total number of convective cells).
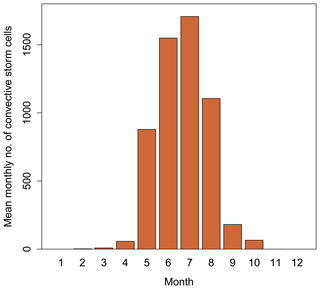
Figure 4Monthly distribution of the mean number of convective storm cells in the Prut River basin, for the period 2003–2017.
The mean number of convective storm cells in the Prut River basin, for the period 2003–2017, was plotted against UTC hours to investigate the diurnal variation of convective cells' occurrence (Fig. 5). The majority of convective storm cells (72.3 %) occur in the afternoon and evening hours, being detected between 10 UTC (13:00 LT) and 18:00 UTC (21:00 LT), while the rest of the cells were detected within the 19:00–10:00 UTC (22:00–13:00 LT) interval. One can also note that during the night hours, convection occurs more frequently than in the morning.
4.2 Spatial distribution
Given the results of the monthly distribution of convective storm cells within the study area, and over the 15-year period considered for the analysis, the spatial distribution of the convective storm cells was derived for the warm season only (May–August). The mapping of the detected convective cells was performed on the entire coverage area of the weather radar, to capture the regions more prone to convection within the Prut River basin but also to highlight the limitations of the radar measurements.
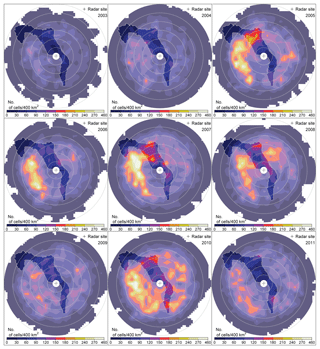
Figure 6Spatial distribution of the convective storm cells in the study area, for the period May–August, for each year between 2003 and 2011. The year is denoted in the upper right corner of each panel, starting from top to bottom and from left to right.
The spatial distribution of the convective storm cells is illustrated in Figs. 6 and 7 for each active season from 2003 to 2017. In these maps, the area of the Prut River basin is highlighted. For plotting reasons, the distribution was smoothed. The annual variability of the number of convective cells detected within the Prut River basin is revealed, the spatial distribution showing various patterns of convection occurrence. Although convective cells were detected over the whole area of the basin, there are regions where convection occurred more frequently in some years. The spatial distribution is relatively uniform during 2003, 2004, 2009, 2014, and 2015. Although the distribution follows the same pattern for 2006, 2011, and 2012, larger values of the number of convective cells are observed in the eastern edge and southern, north-eastern, and western parts of the basin, respectively. Convection occurred more frequently in southern, central, and northern parts of the basin during 2007, 2008, 2010, and 2017, while in 2005 large values are observed in its northern part. The largest values of the number of convective cells are observed in the southern, central, and northern areas of the basin during 2013 and 2016. For the entire 15-year period, the smallest values of the number of convective storms are depicted over the north-western part of the basin. This pattern could be explained by the limitation of detection at longer distances from the radar location, where only the very intense and strong vertical developed storms are detected.
4.3 Storm properties
Various storm characteristics have been deducted from the analysis of the convective storms detected within the Prut River basin between May and August 2003–2017. The storm parameters considered herein are the storm duration, distance travelled, movement speed, maximum reflectivity, and VIL. As these characteristics are not normally distributed, a median estimator was used to measure the central tendency, being more robust than the average (Wilks, 2006). The yearly median values of the above parameters are shown in Table 1. For the whole studied period, the median duration of a convective storm was found to be 42 min. Also, one observes that a small yearly variation exists, the median storm duration being smaller during 2004, 2008, 2012, and 2017. The overall median values for the other storm characteristics are 23 km, 7.7 m s−1, 53 dBZ, and 17 kg m−2 for distance travelled, movement speed, maximum reflectivity, and VIL, respectively. A small yearly variation is observable for these parameters as well.
Table 1Characteristics of convective storms in the Prut River basin, for different years of the 2003–2017 period.
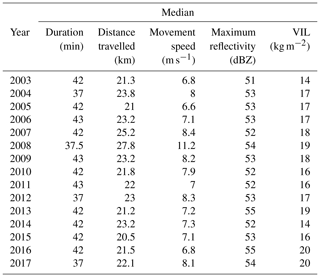
While the median duration of a convective storm was found to be 42 min, for all the storms with a duration greater than this value, the median was found to be 66 min, and for all the storms with a duration of more than 1 h, the median was discovered to be 79 min. The longest-lasting convective storm, detected within the basin, had a duration of 368 min (6 h 8 min) and was recorded in August 2016. The duration of the longest-lasting convective storm in a particular month, between 2003 and 2017, was found to be 242, 215, 235, and 368 min in May, June, July, and August, respectively. Of all the convective storms, it was found that 65.86 %, 26.9 %, and 3.2 % had a duration of more than 30, 60, and 120 min, respectively.
For all storms, the median distance travelled was found to be 23 km, while the longest track was 224 km. Of all the storms, only 10.6 % were both more than 60 min in duration and more than 50 km in distance travelled. The median maximum reflectivity and VIL values of these convective storms were 53.8 dBZ and 19.0 kg m−2, respectively, being not much larger than the overall median of 53 dBZ and 17 kg m−2, respectively. These storms have a median movement speed of 10.2 m s−1, which was higher than the overall median of 7.7 m s−1. For storms that lasted more than 60 min and travelled more than 100 km, the median maximum reflectivity and VIL values were 54.8 dBZ and 21.3 kg m−2, respectively, which were larger than the overall median values. Their median movement speed is 12.9 m s−1, higher than the overall median above. From this analysis, it also resulted that the convective storms with median reflectivity < 50 dBZ had a duration of 36 min and 12.1 kg m−2, while those with median reflectivity > 55 dBZ had a duration of 43 min and 27.9 kg m−2. Consequently, a relation between the storm duration and storm intensity exists, the longer-lasting storms being more intense.
The frequency of occurrence is related to the intensity of severe weather events, approximately following a log-linear decrease with increasing intensity (Brooks and Doswell, 2001; Brooks and Stensrud, 2000). Based on this approach, the characteristics of storms, detected between May and August 2003–2017, were divided into groups (Table 2) and plotted against their percentages from the total number of convective storms (Fig. 8). For example, the graph shows a decrease of the number of convective storms with increasing reflectivity. Of the total number of convective storms detected between May and August 2003–2017 (8984), 69.95 % (6284 storms) are associated with maximum reflectivity between 40 and 55 dBZ, and 25.24 % (2268 storms) are associated with maximum reflectivity between 55 and 60 dBZ, while 4.81 % (432 storms) of all convective storms had a maximum reflectivity equal to or greater than 60 dBZ. This behaviour is characteristic of all the investigated parameters of convective storms, their distribution revealing that the frequency of occurrence of intense convective storms tends to follow an approximately log-linear decrease with increasing intensity, implying that weaker convective storms occur more often than stronger convective storms. This finding is consistent with the results of other studies (e.g. Goudenhoofdt and Delobbe, 2013; May and Ballinger, 2007).
Table 2Radar-derived parameters of convective storms, range of values, and their associated percentages from the total number of convective storms detected within the Prut River basin.
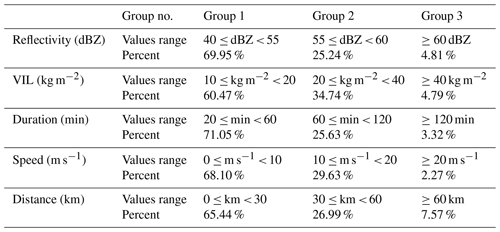
The 2-D histogram comparing the radar-derived direction of movement of storm cells and the day of the convective season (between May and August 2003–2017), for the Prut River basin (Fig. 9), shows that the direction of movement varies each month. Moreover, the histogram reveals that there are daily intervals within a month when a great number of storm cells travel in preferential directions. In May, the greatest number of convective storms cells occurred during the second half of the month, the largest number of storm cells moving from the south–south-east. During June, convective storms occurred, overall, throughout the month, the maximum number of storm cells moving from the south-west. July is characterized by the greatest number of convective storm cells, as depicted by the monthly distribution as well (Fig. 4), the largest number occurring during the last third of the month. In this case, the preferred direction of movement was from the south-east, east–south-east, and south-west. In August, the majority of convective storms cells occurred during the first days and at the beginning of the second half of the month. For the former period, the movement of most of the cells was from the south-west and east–south-east, while for the latter period the movement was preferentially from the south-east.
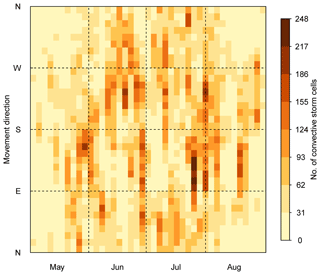
Figure 9The 2-D histogram of the number of convective storm cells as a function of month and movement direction, for the Prut River basin, between 2003 and 2017 (May–August).
Overall, during May–August, from the total number of unique convective storms (8984), 12.2 % (1099 storms) had an average movement direction from the south-west (between 210 and 240∘), and 10.8 % (967 storms) had an average movement direction from the south–south-east (between 140 and 170∘). The maximum of 4.72 % (424 storms) was found in the direction between 230 and 240∘, while the minimum of 0.36 % (33 storms) was found in the direction between 0 and 10∘.
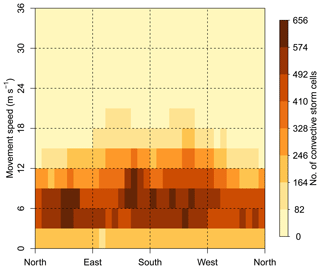
Figure 10The 2-D histogram of the number of convective storm cells as a function of movement direction and storm speed, for the Prut River basin, between 2003 and 2017 (May–August).
The 2-D histogram comparing the radar-derived direction of travel of storm cells and the average storm cells speed (Fig. 10) is consistent with the histogram in Fig. 9 in terms of storm cells' movement direction, revealing that the lowest number of storm cells had a movement direction generally from the north-west. From all the convective storm cells detected within the Prut River basin, between May and August (2003–2017), 80.4 % had a movement speed less than or equal to 12 m s−1. Most of the convective storm cells moving in a general direction from the north-east and south-west had a movement speed between 3 and 9 m s−1, while those moving in a general direction from the south-east had a movement speed between 6 and 12 m s−1. The largest number of convective storm cells with a moving speed between 12 and 18 m s−1 were travelling from southerly directions.
4.4 Large-scale drivers of convective storms in the Prut River basin
Gridded daily and monthly precipitation at 10 and 1 km spatial resolution, developed for the Prut River basin (http://imdroflood.meteoromania.ro/geoportal/, last access: 12 May 2019), were used to test the relation between the radar-derived data and observations. The correlation coefficients between the basin-averaged number of convection cells and observed precipitation amounts are positive and statistically significant for both gridded datasets reaching a magnitude of around 0.6 (Fig. 11).
Even though the time interval is limited to 60 convective months (15 seasons), it is interesting to note the upward trend in the number of convective cells associated with a downward trend in precipitation amounts (Fig. 11). Several physical mechanisms shape the extreme precipitation response to thermodynamic and dynamic factors. The thermodynamic contribution is relatively well understood, but theoretical understanding of the microphysical and convective-related dynamical contributions is still under development (O'Gorman, 2015). It is not the purpose of our study to analyse such issues in depth. However, we want to highlight here the importance of integrating radar measurements with other types of meteorological data to better document the risks related to extreme precipitation drivers in the context of climate variability and change.
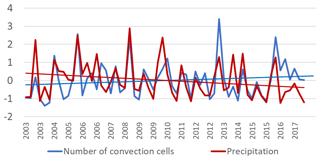
Figure 11Basin-averaged standardized anomalies of the number of storm cells and precipitation amounts for the months of the active season (May to August) in the period 2003–2017. The precipitation amounts averaged over the Prut basin are computed from the gridded dataset with 10 km resolution in latitude and longitude. The correlation coefficient is 0.60.
In this context, monthly values of sea level pressure (SLP), convective available potential energy (CAPE), and total column water were extracted from ERA 5 reanalysis to identify the large-scale drivers of local convective storms from the Prut River basin. The reanalysis data span the common active seasons.
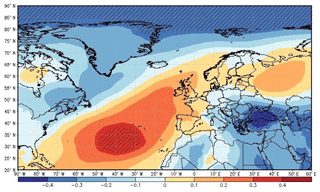
Figure 12Correlation coefficients between the monthly number of storms in the Prut River basin and monthly means of sea level pressure (SLP) for the active season (May to August) in the period 2003–2017. The SLP data are from the ERA 5 reanalysis (the deterministic dataset). Hatched areas illustrate correlation coefficients statistically significant at confidence levels higher than 99 %.
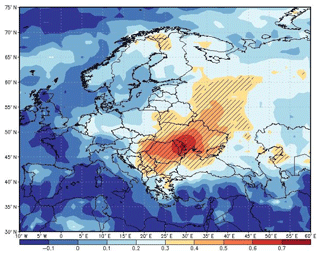
Figure 13Correlation coefficients between the monthly number of convective storm in the Prut River basin and monthly means of convective available potential energy (CAPE) for the active season (May to August) in the period 2003–2017. The CAPE data are from the ERA 5 reanalysis (the deterministic dataset). Hatched areas illustrate correlation coefficients statistically significant at confidence levels higher than 99 %.
We performed a correlation analysis using the monthly number of convective systems in the Prut basin and SLP over the Atlantic–European region. The correlation map (Fig. 12) suggests that low atmospheric pressure over the Black Sea region can be a dynamical driver of convective storms in the analysed river basin. Furthermore, the statistical signature of the high convective activity appears in a larger-scale context by mapping the correlation of monthly number of convective systems in the Prut basin and the CAPE (Fig. 13). The correlation map between the monthly number of convective systems in the Prut basin and total column water in the European regions is also illustrated in Fig. 14. The area of high positive correlations shown in Figs. 13 and 14 supports the hypothesis that extratropical cyclones passing the Black Sea are the main large-scale dynamical drivers of convection in the Prut River basin. The patterns of atmospheric circulations which can lead to the presence of low SLP over the Black Sea are those identified by Surkova et al. (2013) using clustering analysis. The first SLP pattern in the paper of Surkova et al. (2013) shows an atmospheric trough extending from Scandinavia and the Baltic Sea to the Black Sea. In this case, high atmospheric pressure prevails to the west of the Black Sea region. The second SLP pattern shown by Surkova et al. (2013) reveals an atmospheric trough spreading from the eastern Mediterranean towards the Black Sea, which is blocked to the north by a high-pressure field. Our correlation map linking the monthly number of convective systems in the Prut basin with the SLP (Fig. 12) can be interpreted as a linear superposition of the above-mentioned patterns. Furthermore, the maps of monthly number of convective systems in the Prut basin correlated to CAPE (Fig. 13) and total column water (Fig. 14) show a consistent picture with the patterns identified by Surkova et al. (2013).
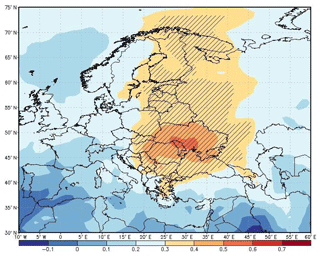
Figure 14Correlation coefficients between the monthly number of storms in the Prut River basin and monthly total column water for the active season (May to August) in the period 2003–2017. The SLP data are from the ERA 5 reanalysis (the deterministic dataset). Hatched areas illustrate correlation coefficients statistically significant at confidence levels higher than 99 %.
The characteristics of the convective storms within the transboundary Prut River basin have been analysed over a 15-year period (2003–2017). The analysis is based on radar data, and the SCIT algorithm has been used to detect and track convective storms in successive 6 min volumetric scans. For the whole study period, more than 2.2 million storm cells were detected in the coverage area limited to between 20 and 300 km from the radar. A simple selection criterion, based on the average VIL values, has been used to separate convective from stratiform storm cells. A number of 9507 convective storms with a duration of at least approximately 20 min have been identified in a study area. These were associated with just over 84 000 storm cells.
From the analysis of the results in this study, a few matters of the climatology of convective storms in the Prut River basin have become clear. Interannual variation of the convective activity was observed, with the largest number of convective cells detected in 2016. The monthly variation revealed that the convection prevails during May–August, when more than 90 % of the convective cells were identified. According to the same analysis, the peak was registered in the month of July, when close to one-third of the total number of convective cells were detected. The majority of convective storm cells occurred in the afternoon and evening hours (between 10:00 and 18:00 UTC), while during the night, convection occurred more frequently than in the morning. These characteristics are associated with the seasonal and diurnal cycles of temperature in the analysed area.
Although the spatial distribution of the convective cells shows convection developing throughout the whole basin area, interannual spatial variations in finer-scale patterns are also observed. The analysis revealed convection hotspots in the northern, central, and southern parts of the basin. The north-western part of the basin has been identified as the region where the smallest number of convective cells were detected, but this could be explained by the limitations of radar measurements at long distances. Nevertheless, despite the inherent limitations of radar measurements, this type of analysis offers one of the most complete sources of information on the convective storms' characteristics in the coverage area.
Considering the convective storms that occurred between May and August (2003–2017), their radar-derived characteristics have been analysed. The median duration of a convective storm was found to be 42 min, while the median distance travelled was found to be 23 km. The median speed of a storm was 7.7 m s−1, increasing to 12.9 m s−1 for the convective storms that lasted more than 60 min and travelled more than 100 km. It was also found that the longer-lasting convective storms were more intense, and their frequency of occurrence approximately follows a log-linear decrease with increasing intensity.
The convective storms' movement direction and speed analysis highlighted the fact that there are intervals within a given month when a great number of storm cells travel in preferential directions. An important number of convective storms had a southerly track (12.2 % from the south-west and 10.8 % from the south–south-east). Also, the largest number of convective storms moving with higher speeds (between 12 and 18 m s−1) came from the southerly directions. The above-mentioned statistics are consistent with the correlation analysis performed using the monthly number of convective systems in the Prut basin and large-scale SLP. The monthly number of convective systems in the Prut basin is best correlated with low values of SLP over the Black Sea, which is situated southward of the area of interest. The Black Sea seems to act as an additional source of atmospheric humidity feeding in the atmospheric low systems, with trajectories passing over it and enhancing atmospheric instability in the area of interest.
This study, based mainly on radar data, offers perspective for the better understanding of convective storm activity, which is of paramount importance in assessing the risks associated with severe weather episodes in the context of climate variability and change. Given the study region and the density of radar sites, additional observations from neighbouring radars should be included to improve the quality of the observations in the overlapping areas. Supplemental storm properties could be analysed by including other datasets like lightning, satellite measurements, reanalysis, and ground observations. The latter could be also used to assess the performance of the convective storm identification and tracking algorithm, and, through this, to derive comprehensive and high-resolution climatology of weather events such as extreme precipitation and hailfall. Our study reveals the feasibility of integrating radar measurements with other types of meteorological observations and reanalysis to better document the risks related to extreme meteorological events in the context of climate variability and change.
Because of the data policy, raw radar data and radar products are not publicly available.
All the authors contributed equally to the manuscript.
The authors declare that they have no conflict of interest.
This article is part of the special issue “Hydroclimatic extremes and impacts at catchment to regional scales”. It is not associated with a conference.
The study was financially supported by the IMDROFLOOD project under the ERA-NET Cofund WaterWorks2014. We thank our colleagues Alexandru Dumitrescu and Sorin Dascălu, from the National Meteorological Administration (Bucharest, Romania), for their help in processing gridded precipitation datasets for the Prut River basin.
This research has been supported by the Romanian National Authority for Scientific Research and Innovation (UEFISCDI) and ERA-NET Cofund WaterWorks2014 (grant no. 81/2016).
This paper was edited by Chris Reason and reviewed by two anonymous referees.
American Meteorological Society: Cell, Glossary of Meteorology, available at: http://glossary.ametsoc.org/wiki/Cell (last access: 12 May 2019), 2018.
Brooks, H. E. and Doswell, C. A.: Some aspects of the international climatology of tornadoes by damage classification, Atmos. Res., 56, 191–201, https://doi.org/10.1016/S0169-8095(00)00098-3, 2001.
Brooks, H. E. and Stensrud, D. J.: Climatology of heavy rain events in the United States from hourly precipitation observations, Mon. Weather Rev., 128, 1194–1201, https://doi.org/10.1175/1520-0493(2000)128<1194:COHREI>2.0.CO;2, 2000.
Cărbunaru, D., Stefan, S., Sasu, M., and Stefănescu, V.: Analysis of Convective Thunderstorm Split Cells in South-Eastern Romania, International Journal of Atmospheric Sciences, 2013, 162541, https://doi.org/10.1155/2013/162541, 2013.
Cică, R. , Burcea, S., and Bojariu, R.: Assessment of severe hailstorms and hail risk using weather radar data, Met. Apps., 22, 746–753, https://doi.org/10.1002/met.1512, 2015.
Copernicus Climate Change Service (C3S): ERA5: Fifth generation of ECMWF atmospheric reanalyses of the global climate, Copernicus Climate Change Service Climate Data Store (CDS), available at: https://cds.climate.copernicus.eu/cdsapp\#!/home (last access: 10 May 2019), 2017.
Davini, P., Bechini, R., Cremonini, R., and Cassardo, C.: Radar-based analysis of convective storms in Nortwestern Italy, Atmosphere, 3, 33–58, https://doi.org/10.3390/atmos3010033, 2012.
Goudenhoofdt, E. and Delobbe, L.: Statistical Characteristics of Convective Storms in Belgium Derived from Volumetric Weather Radar Observations, J. Appl. Meteor. Climatol., 52, 918–934, https://doi.org/10.1175/JAMC-D-12-079.1, 2013.
Greene, D. R. and Clark, R. A.: Vertically Integrated Liquid Water – A New Analysis Tool, Mon. Weather Rev., 100, 548–552, https://doi.org/10.1175/1520-0493(1972)100<0548:VILWNA>2.3.CO;2, 1972.
Johnson, J. T., MacKeen, P. L., Witt, A., Mitchell, E. D., Stumpf, G. J., Eilts, M. D., and Thomas, K. W.: The Storm Cell Identification and Tracking Algorithm: An Enhanced WSR-88D Algorithm, Weather Forecast., 13, 263–276, https://doi.org/10.1175/1520-0434(1998)013<0263:TSCIAT>2.0.CO;2, 1998.
Lemon, L. R., Stan-Sion, A., Soci, C., and Cordoneanu, E.: A strong, long-track, Romanian tornado, Atmos. Res., 67–68, 391–416, https://doi.org/10.1016/S0169-8095(03)00063-2, 2003.
May, P. T. and Ballinger, A.: The Statistical Characteristics of Convective Cells in a Monsoon Regime (Darwin, Northern Australia), Mon. Weather Rev., 135, 82–92, https://doi.org/10.1175/MWR3273.1, 2007.
Mohee, F. M. and Miller, C.: Climatology of Thunderstorms for North Dakota, 2002–06, J. Appl. Meteor. Climatol., 49, 1881–1890, https://doi.org/10.1175/2010JAMC2400.1, 2010.
O'Gorman, P. A.: Precipitation Extremes Under Climate Change, Curr. Clim. Change Rep., 1, 49–59, https://doi.org/10.1007/s40641-015-0009-3, 2015.
Peter, J. R., Manton, M. J., Potts, R. J., May, P. T., Collis, S. M., and Wilson, L.: Radar-Derived Statistics of Convective Storms in Southeast Queensland, J. Appl. Meteor. Climatol., 54, 1985–2008, https://doi.org/10.1175/JAMC-D-13-0347.1, 2015.
Qi, Y., Zhang, J., and Zhang, P.: A real-time automated convective and stratiform precipitation segregation algorithm in native radar coordinates, Q. J. Roy. Meteor. Soc., 139, 2233–2240, https://doi.org/10.1002/qj.2095, 2013.
Romanescu, G. and Stoleriu, C. C.: Exceptional floods in the Prut basin, Romania, in the context of heavy rains in the summer of 2010, Nat. Hazards Earth Syst. Sci., 17, 381–396, https://doi.org/10.5194/nhess-17-381-2017, 2017.
Romanescu, G., Stoleriu, C. C., and Romanescu, A. M.: Water reservoirs and the risk of accidental flood occurrence. Case study: Stanca–Costeşti reservoir and the historical floods of the Prut river in the period July–August 2008, Romania, Hydrol. Process., 25, 2056–2070, https://doi.org/10.1002/hyp.7957, 2011.
Seres, A. T. and Horváth, Á.: Thunderstorm climatology in Hungary using Doppler radar data, Quarterly Journal of the Hungarian Meteorological Service, 119, 185–196, 2015.
Surkova, G. V., Arkhipkin, V. S., and Kislov, A. V.: Atmospheric circulation and storm events in the Black Sea and Caspian Sea, Centr. Eur. J. Geosci., 5, 548–559, https://doi.org/10.2478/s13533-012-0150-7, 2013.
Wang, Y., Han, L., and Wang, H.: Statistical characteristics of convective initiation in the Beijing-Tianjin region revealed by six-year radar data, J. Meteorol. Res., 28, 1127–1136, https://doi.org/10.1007/s13351-014-3061-3, 2014.
Wilks, D. S.: Statistical Methods in the Atmospheric Sciences, Second Edition, Academic Press, San Diego, USA, 627 pp., 2006.
Zhang, J. and Qi, Y.: A Real-Time Algorithm for the Correction of Brightband Effects in Radar-Derived QPE, J. Hydrometeor., 11, 1157–1171, https://doi.org/10.1175/2010JHM1201.1, 2010.