the Creative Commons Attribution 4.0 License.
the Creative Commons Attribution 4.0 License.
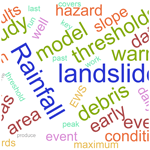
Preface: Landslide early warning systems: monitoring systems, rainfall thresholds, warning models, performance evaluation and risk perception
Luca Piciullo
Stefano Luigi Gariano
- Article
(872 KB) - Full-text XML
- BibTeX
- EndNote
Among the diverse mitigation measures available for reducing the risk to life related to landslides, early warning systems certainly constitute a significant option available to the authorities in charge of risk management and governance. Landslide early warning systems (LEWSs) are nonstructural risk mitigation measures usable at different scales of analysis. Basically, they are used to monitor one or more variables responsible for triggering landslides and to generate and disseminate timely and meaningful warning information to enable individuals, communities and organizations threatened by a hazard to act appropriately and in sufficient time to reduce the possibility of harm or loss (UNISDR, 2009). The installation of a LEWS is often a cost-effective risk mitigation measure and, in some instances, the only suitable option for sustainable management of disaster risks (Glade and Nadim, 2014). The increasing trend shown in the last decade in the employment of LEWSs, in particular at a regional scale, in developing countries confirms the previous statement. Several general schemes of LEWS were proposed in the literature, among which are those recently presented by Intrieri (2013), Fathani et al. (2016), Sättele et al. (2016), Calvello (2017) and Piciullo et al. (2018). Even if a general scheme to describe the structure of a LEWS can be provided, the choice of variables to be measured and monitored varies with the type of landslide that is being forecast and the system's objectives (Lacasse and Nadim, 2009).
The Natural Hazards Division of the European Geosciences Union (EGU) has always paid great attention to LEWSs. In the past, several sessions focused on landslide warning systems have been held during EGU general assemblies, starting at the EGU 2007 with the sessions “Multidisciplinary monitoring, characterization and early warning projects on large landslides” (convened by Lars Harald Bilkra) and “Early warning systems and multidisciplinary approaches in natural hazards and risk assessments” (convened by Thomas Glade). The first was focused on a particular type of landslide, while other sessions were focused specifically on methods used in the early warning procedure (e.g., monitoring or forecasting). The latter was more generally focused on all natural hazards, as was the interdisciplinary PICO session entitled “Operational forecasting and warning systems for natural hazards: challenges and innovation”, organized in 2015 by Femke Davids and Michael Cranston. However, a session entirely focused on the early warning of landslides, considering different methods and phenomena, as well as operational and prototype systems at both regional and local scales, and performance evaluation of the warnings issued, was missing.
In addition, no special issue has been entirely devoted to this topic in the Natural Hazards and Earth System Sciences (NHESS) journal. Diverse NHESS special issues were focused either on different topics of risk assessment and management or on monitoring, as, for example, among the most recent, “Approaches to hazard evaluation, mapping, and mitigation” by Iovine et al. (2011), “New developments and applications in early warning, monitoring and remote sensing of landslides” by Catani et al. (2012), “Landslide hazard and risk assessment at different scales” by Reichenbach et al. (2013) and “Landslide Prediction and Forecasting” by Catani and Guzzetti (2014). However, the topic is widely studied in NHESS articles. In fact, a search of the keywords “landslide”, “warning” and “system” in the abstracts of all articles that have ever been published in the Division's NHESS journal produced 698 results.
Within this framework, this special issue was initially conceived to collect the most relevant works presented to the session SSS9.5/NH3.13 on “Landslide early warning systems: monitoring systems, rainfall thresholds, warning models, performance evaluation and risk perception” within the 2017 General Assembly of the European Geosciences Union. However, several external contributions were included to better encompass the complex and multidisciplinary aspects characterizing LEWSs. The special issue includes original papers conveying recent scientific advances as well as useful case studies, with the aim of providing details on both the research state of the art and the operational use of such systems.
The special issue focuses on LEWSs at both regional (e.g., Krøgli et al., 2018; Wei et al., 2018) and local scales (e.g., Frodella et al., 2017; Reder et al., 2018). The scale of analysis influences several aspects connected to the design and employment of the system, such as the type of investigated landslide phenomena, the variables to be considered for correlations, the monitoring system and the actors involved. Systems addressing single landslides at slope scale can be named as local LEWSs (Lo-LEWSs), and systems operating over wide areas at regional scale are referred to as territorial LEWSs (Te-LEWSs). An initial key difference between Lo-LEWSs and Te-LEWSs is the a priori knowledge of the areas affected by future landsliding. When the exact location of future landslides is unknown and the area of interest extends beyond a single slope, only a Te-LEWS can be employed (e.g., national, regional, provincial and municipal systems). Conversely, Lo-LEWSs are typically adopted to cope with the risk related to one or more known well-identified landslides (Piciullo et al., 2018).
A broad range of settings are accounted for in the special issue. For instance, different types of landslide, ranging from specific classes to wider groups of landslide are considered in the submitted papers: debris flows (Melo et al., 2018; Pan et al., 2018), lahars (Capra et al., 2018), shallow landslides (Canli et al., 2018), deep-seated landslides (Intrieri et al., 2017; Liu et al., 2018), rockfalls and rock avalanches (Frodella et al., 2017) and landslides of mixed types (Krøgli et al., 2018; Segoni et al., 2018a). The approaches used span from the physically based models (Reder et al., 2018; Salvatici et al., 2018) to the statistical correlations (Wei et al., 2018). Concerning the triggering conditions, the special issue is particularly focused on rainfall-induced landslides (e.g., Peres et al., 2018; Reder et al., 2018); however it also accounts for snowmelt-induced landslides (Krøgli et al., 2018) and for specific predisposing factors such as earthquakes (Shi et al., 2018) and wrong human management of the territory (Mendes et al., 2018). Another key issue in defining reliable LEWSs (Piciullo et al., 2018) is the quality and quantity of data available to properly calibrate the forecasting models. A broad range of conditions is covered, ranging from case studies characterized by scarcity (Shi et al., 2018) to the abundance of data (Devoli et al., 2018; Vaz et al., 2018) and, in some cases, also the measurement of hydrologic (Segoni et al., 2018a) or geotechnical variables (Canli et al., 2018; Salvatici et al., 2018) to strengthen the forecasting models.
Moreover, the works included in the special issue describe early warning systems at very different stages of employment. Some of them are well established and have been operated for years (Devoli et al., 2018); others could be more properly defined as preliminary studies aimed at addressing landslide hazard and establishing a scientific and technical basis for the buildup of a LEWS (Uzielli et al., 2018). It should be remarked that the establishment of a LEWS is a complex task, and before operating a warning system, several preliminary steps are usually required. This is reflected by the content of this special issue, in which different steps of this process are accounted for: the establishment of landslides–rainfall correlations (Liu et al., 2018), the definition of a runout simulation model (Melo et al., 2018), the definition of predictive models (Greco and Pagano, 2017), the setting up of prototypes (Intrieri et al., 2017; Canli et al., 2018), the validation and the performance evaluation of the predictive early warning model (Peres et al., 2018; Segoni et al., 2018a), the performance of operational LEWSs through case studies (Devoli et al., 2018) and the evaluation of risk perception (Chaturvedi et al., 2018). A relevant subject covered by many articles included in the special issue is the definition of rainfall thresholds for landslide prediction (e.g., Pan et al., 2018; Peres et al., 2018; Vaz et al., 2018), a highly debated topic among the landslide community that often overlaps to LEWSs (Segoni et al., 2018b). The most debated unresolved issues in rainfall threshold research include the following
-
the definition of objective and automatic procedures to define the thresholds (Staley et al., 2013; Segoni et al., 2014; Iadanza et al., 2016; Vessia et al., 2016; Rossi et al., 2017; Melillo et al., 2018);
-
the need for taking into account the hydrological conditions of the hillslope system with more complex approaches (Posner et al., 2015; Bogaard and Greco, 2018);
-
the evaluation and quantification of diverse uncertainties (Nikolopoulos et al., 2015; Destro et al., 2017; Marra et al., 2017; Rossi et al., 2017; Marra, 2018; Peres et al., 2018);
-
the importance of validation procedures (Staley et al., 2013; Gariano et al., 2015; Lagomarsino et al., 2015);
-
the use of rainfall data gathered from ground-based radars or satellites (Robbins, 2016; Destro et al., 2017; Rossi et al., 2017; Brunetti et al., 2018);
-
the implementation of the thresholds into hazard management procedures and early warning systems (Kirshbaum et al. 2015; Rosi et al., 2015; Piciullo et al., 2017, 2018; Krøgli et al., 2018; Wei et al., 2018; Pecoraro et al., 2018).
Figure 1 shows the word cloud of the abstracts of the papers published in this special issue. It can be seen that rainfall and thresholds are the most assessed topic, after landslides, of course. Moreover, also “warning” and “model” are among the most used words in the abstracts of the submitted papers, outlining the essential importance of defining a warning model to operate a LEWS.
Overall, the 20 works collected in this special issue contain experiences of LEWSs spread all over the world including Europe (Italy, Portugal, Norway and Austria), the Americas (Brazil and Mexico) and Asia (China, Taiwan, and India).
The paper by Intrieri et al. (2017) deals with the management of logistic issues in LEWSs linked to advanced monitoring techniques, such as big data collection, transfer and storing. They consider data coming from a ground-based interferometric synthetic aperture radar (GB-InSAR) located along a highway section in southern Italy. Such systems have the main drawback of managing large data flow (high-quality displacement time series with a high acquisition frequency) derived from continuous real-time monitoring. The issue is assessed by means of in situ pre-elaboration and data management procedures to produce standardized files, carrying only necessary information. The pre-elaboration is based on data correction, filtering and reduction, and also on the elimination of the areas not relevant for the monitoring. Data management is based on the transmission of data averaged over determined time frames, proportionate with the kinematics of the monitored phenomenon. After this, data can be more easily transferred to the data collecting and processing center of the LEWS, to be compared to fixed threshold values for the issuing of warning messages.
Frodella et al. (2017), describe in detail the outcomes of a 2-year GB-InSAR monitoring campaign (December 2010–December 2012) in the upper Agno River valley. As a consequence of diffuse and severe slope instabilities that affected the whole Veneto region (northeastern Italy) between 31 October and 2 November 2010, a local-scale monitoring system, based on a GBInSAR, is implemented. The paper describes how the monitoring strategy has been implemented for mapping and monitoring slope landslide residual deformations and early warning purposes in case of landslide reactivation. Given the recorded residual deformations, four critical sectors are identified in the monitored scenario on the basis of the measured cumulated displacements, frequency of activation and geomorphological features.
Greco and Pagano (2017) propose a general frame for LEWSs, applicable to all types of water-related natural hazards. The work addresses the importance of identifying the evolutionary stage of the catastrophic event at which the prediction should be applied, to maximize its effectiveness. The evolutionary stages of the phenomenon, based on its physical characteristics, are considered a relevant feature of the LEWS, as is the accuracy of the predictions, more than the spatial scale (two examples of the application for rapid shallow landslides in southern Italy are discussed). Further classification criteria proposed are the (well-known) distinction between empirical and physically based models and the (new) distinction between online and offline prediction. The implementation of an effective LEWS, based on the selection of the predictive model and prediction time, the definition of warning thresholds and the adoption of mitigation measures, might benefit from the proposed framework, at all scales and in all landscapes.
Mendes et al. (2018) analyze a case study in Brazil to show that landslides cannot be attributed solely and exclusively to rainfall events. A detailed geotechnical survey is conducted for three representative slopes to obtain geotechnical parameters needed for slope stability analysis. Then, a set of numerical experiments is designed to assess the contribution of natural and anthropic factors to slope instability separately. Results show that natural factors (intense rainfall and geotechnical conditions) are not severe enough to trigger landslides in the study area and that human disturbance is entirely responsible for the studied landslide events.
The research paper proposed by Melo et al. (2018) presents a model for the simulation of debris flow runout, which might be useful for landslide risk management and early warning. Considering as a case study a basin in central Portugal, a dynamic model is calculated and validated. The model integrates surface runoff, erosion along the channels, flow propagation and deposition of material. Three scenarios are elaborated using different excess rain values and, for each scenario, the buildings at risk in the investigated area are accounted for. However, the model is not dependent on local conditions. The model adopts a physically based approach, so allowing – unlike the data-driven models – for the estimation of flow velocities, thickness of the deposits and impact force against obstacles. Such parameters are very relevant for the development of a LEWS.
Reder et al. (2018) investigate the effect of evapotranspiration on physically based models for slope-scale rainfall-induced landslides. The study evaluates the performance of three approaches that can be used to convert precipitation and evaporative fluxes into hydrological variables to be used in slope stability assessment and early warning. Two of the approaches incorporate evaporation, with one representing evaporation as both a boundary and internal phenomenon, and the other only a boundary phenomenon. The third approach totally disregards evaporation. Model performances are assessed by analyzing a well-documented case study involving a sloping volcanic cover 2 m thick (Nocera Inferiore landslide, in Italy). The comparison of the results indicates that the greater the complexity and completeness of the model, the lower the number of false alarms, thus providing a valuable help to early warning systems.
Concerning uncertainty in rainfall and landslide datasets, Peres et al. (2018) perform a quantitative analysis of the impacts of uncertain landslide initiation instants on the assessment of rainfall intensity–duration thresholds. These thresholds are often used to separate warning levels in LEWSs. The analysis is based on the definition of a synthetic dataset of rainfall events triggering landslides. The authors introduce errors into the synthetic dataset by hypothesizing the way such information may be retrieved from newspapers and similar resources (blogs and fire brigade reports), which are the main primary sources available to build landslide historical inventories. The analysis shows that the impacts of the above uncertainty sources can be significant, especially when errors exceed 1 day or when the estimated triggering time is precedent to the actual one. Errors generally lead to underestimated thresholds, i.e., lower than those that would be obtained from an error-free dataset. Consequently, these thresholds employed in a landslide early warning system would lead to a high number of false alerts, reducing the reliability of the system.
The paper by Shi et al. (2018) deals with debris flow occurrence thresholds, expressed in terms of rainfall intensity and duration, by using radar data in an earthquake-affected area (Sichuan, China). The case study is challenging, as the dataset is limited (six events in the period 2012–2014) and the rain gauge network is very sparse; however, a better understanding of the relationship between rainfall and debris flow initiation could be obtained by means of radars with highly spatiotemporal resolution. The results highlight the significance of using remote sensing observations for the estimation of debris-flow-triggering rainfall, within the perspective of establishing a dedicated early warning system.
The paper of Capra et al. (2018) provides a valuable contribution to the analysis of the relationships between flood runoff formation and lahar occurrence during hurricanes. They analyze the correlations among multiple streams of data (rainfall intensity, cumulative rain, geophone records) and perform rainfall–runoff simulations to examine the relationship between rainfall and lahar pulses. The results are compared with the arrival time of the main lahar fronts, showing that flow pulses can be correlated with rainfall peak intensity and watershed discharge, depending on the watershed area and shape. This outcome can be considered a preliminary study, which is essential to establish a lahar early warning system based on the monitoring of hydro-meteorological events.
Segoni et al. (2018a) perform an experiment on an existing and operating Te-LEWS, trying to increase its forecasting effectiveness by using soil moisture as an input variable together with (or instead of) rainfall. They test two approaches: the first one is based on a simple soil moisture threshold value under which rainfall thresholds are not used because landslides are not expected to occur. The second approach substitutes the rainfall thresholds based on antecedent precipitation (used by the original version of the early warning system) with purposely defined soil moisture thresholds. The first approach has the advantages of being very easy and straightforward to implement, but it could only be used to reduce false alarms, while the second approach requires a more thorough calibration but could reduce both false alarms and missed alarms.
The work presented in Vaz et al. (2018) proposes a comprehensive method to assess rainfall thresholds for landslide initiation using a centenary landslide database associated with a single centenary daily rainfall dataset. The landslide database is used for defining landslide events that occurred between 1865 and 2010. The method is applied to the Lisbon region and includes the rainfall return period analysis that is used to identify the critical rainfall combination (cumulated rainfall duration) related to each landslide event. Results show that landslide events located up to 10 km from the rain gauge can be used to calculate the rainfall thresholds in the study area; however, these thresholds may be used with acceptable confidence up to 50 km from the rain gauge.
Liu et al. (2018) propose a state fusion entropy method to derive landslide instability through an entropy analysis of deformation states. The method is based on the relationship between displacement monitoring data, deformation states and landslide stability, and might be useful to study continuous landslide stability, also considering a historical maximum index to identify key time nodes of stability changes. Both cumulative state fusion entropy and the historical maximum index can be used to judge deformation stages of landslides and thus to determine early warning levels. The proposed method is tested considering the Xintan landslide as a detailed case study, as well as other landslides in the Three Gorges Reservoir, in China.
Devoli et al. (2018) present a description and a comparison of two LEWSs currently operating, since the late 2000s, in Italy (Piedmont region) and Norway, designed to predict rainfall- and snowmelt-induced landslides. Both systems provide landslide predictions, in four warning levels disseminated through the internet, based on a comparison of statistical thresholds, daily rainfall forecasts and real-time observation, together with expert analysis. Rainfall thresholds for the different types of landslides are used in Piedmont, while a unique threshold based on water supply and soil moisture is used for all type of landslides in Norway. The analyzed case study is a large low-pressure system that struck Europe in May 2013, producing several geo-hydrological effects in both regions, successfully forecasted and communicated to the public by the two systems. This collaboration of two technical and scientific institutions is quite promising for sharing techniques and best practices (e.g., for reducing the lead time of the LEWSs).
Pan et al. (2018) present a challenging case study, trying to devise rainfall thresholds for post-seismic debris flows' early warning when the scarcity of input data (both rainfall and debris flows) prevents a proper calibration of statistical rainfall thresholds. After a geomorphological and hydrological characterization of the debris flows monitored in the study area, they define a process-based rainfall threshold based on an antecedent precipitation index and on the peak 1 h rainfall. The comparison with other threshold configurations and with different threshold models shows that the proposed approach is a promising starting point for further studies on debris flow early warning systems in case studies characterized by similar constraints.
Krøgli et al. (2018) describe in detail the “Landslide Forecasting and Warning Service”, which operates in Norway at national scale since 2013. The main points of strengths of the system are automatic hydrological and meteorological stations, the landslide and flood historical database, hydro-meteorological forecasting models, thresholds or return periods and a trained group of forecasters. The paper also provides an evaluation of the model, by means of a validation performed against data collected through 4 years of operation (rate of over 95 % correct daily assessments), by positive feedbacks received from users through a questionnaire and by a case study from autumn 2017.
The work presented by Chaturvedi et al. (2018) concerns the influence of differing strengths of experiential feedback on people's decisions relating to landslides. The authors present a tool that allows for the determination of the effect of feedback and its availability, under different conditions, in the landslide risk decision-making process, in particular regarding investments. The tool is tested in a study area in Mandi, Himachal Pradesh, India. They find that investments are greater in conditions where experiential feedback is present and damages are high. Such simulation is very useful for landslide risk communication and perception.
Wei et al. (2018) propose a hazard prediction model that combines landslide susceptibility and rainfall thresholds. First, they divide slope units into three susceptibility levels using a logistic regression analysis of preparatory factors. Then, for each susceptibility level, a rainfall threshold based on 3 h mean intensity and 24 h accumulated rainfall is calculated separately. It is found that the threshold values gradually increase as the susceptibility decreases for the same alert level, thus potentially providing a spatial refinement for Te-LEWSs based on empirical rainfall thresholds.
Salvatici et al. (2018) apply a physically based model to forecast shallow landslides at regional scale. The model is a physically based distributed slope stability simulator for analyzing shallow-landslide-triggering conditions during a rainfall event, namely HIRESSS (HIgh REsolution Slope Stability Simulator). This model is applied to a portion of the Aosta Valley region, located in the northwest of the Italian Alpine mountain chain. The model is tested in the back analysis of two past rainfall events that triggered several shallow landslides in the study area between 2008 and 2009. In order to run the model and to increase its reliability, an in-depth study of the geotechnical and hydrological properties of hillslopes controlling shallow landslides formation is conducted. This method can be useful to identify warning areas with different probabilities of landslide occurrence.
Canli et al. (2018) provide a summary of the main and most recent advances obtained in hydrology for flood forecasting and they apply the lesson learnt to a distributed physically based landslide model in a probabilistic framework, obtaining a prototype landslide ensemble prediction system. The paper also discusses additional details that are of key importance to implement a physically based model in a Te-LEWS, such as computational resources needed, parameter variability and uncertainty, calibration and validation.
The paper by Uzielli et al. (2018) provides a risk framework for infrastructures. It assesses the temporal variation in landslide hazard, specifically for a section of the Autostrada A3 Salerno–Napoli highway, which runs across the toe of the Monte Albino relief in the municipality of Nocera Inferiore, Campania, Italy. The reach probability, the probability that a given spatial location is affected by debris flows, is calculated spatially through numerical simulation of landslide runout. Landslide probability is computed spatially using Flow-R, a distributed empirical model developed in Matlab. The results displayed temporal and spatial variability of hazard in the study area.
The contributions of the special issue “Landslide early warning systems: monitoring systems, rainfall thresholds, warning models, performance evaluation and risk perception” provide interesting understanding and new perspectives on the very wide world of the early warning systems for landslide monitoring and predictions. The different aspects covered in this special issue demonstrate that the establishment of a LEWS is a complex task, and that before operating a warning system, several preliminary steps are usually required. The research contributions deal with both scientific and technical aspects, demonstrating the importance of the collaboration among scientists and technical system managers, as well as the importance of defining the right variables to be measured and monitored as a function of the type of landslide and scale of analysis. At the same time, the contributions provide an additional step forward for the definition of reliable LEWSs as risk mitigation measures. However, not all important aspects have been covered in this special issue. Further efforts are also needed in the performance evaluation of the warnings issued, as well as in the analysis of how the warnings are communicated and perceived by the elements at risk. The main insights that can be derived from this special issue for designing and employing reliable LEWSs can be summarized as follows:
-
the relevance of joining scientific, technical and social components in the structure of a LEWS;
-
the significance of the quality and quantity of input data and calibration parameters, in order to obtain reliable forecasts and to adequately assess, handle and communicate their degree of uncertainty;
-
the importance of defining and carrying out validation and performance evaluation procedures of the LEWSs;
-
the need for the adoption of multi-source and multi-parameter warning systems, e.g., by coupling rainfall data from rain gauges and from radar, or by coupling rainfall measurements and forecasting with the monitoring of other hydrogeological and geotechnical parameters;
-
the importance of the implementation of adequate communication strategies and emergency plans, and full integration between model outputs, issued warnings and countermeasures to be undertaken in response to a forecasted landslide hazard;
-
the need to share experiences and data and to provide open access to local and governmental databases.
The community dealing with LEWSs is very broad and pertains to different scientific backgrounds and technical sectors; thus multi-thematic meetings and workshops are really important in this field of research. The aim of this special issue and of the EGU session goes in this direction, and this hopefully poses a step toward reliable landslide early warning systems.
We acknowledge Thom Bogaard (Delft University of Technology) and Filippo Catani (University of Florence) for supporting us in organizing and carrying out the special issue. In addition, Thom Bogaard provided useful comments and suggestions to improve this preface. We are grateful to Søren Boje (Norwegian Water Resources and Energy Directorate), who contributed to the organization of the thematic session within the EGU 2017 General Assembly. We thank all the authors of the 20 papers of this special issue for their interesting contributions.
Bogaard, T. and Greco, R.: Invited perspectives: Hydrological perspectives on precipitation intensity-duration thresholds for landslide initiation: proposing hydro-meteorological thresholds, Nat. Hazards Earth Syst. Sci., 18, 31–39, https://doi.org/10.5194/nhess-18-31-2018, 2018.
Brunetti, M. T., Melillo, M., Peruccacci, S., Ciabatta, L., and Brocca, L.: How far are we from the use of satellite rainfall products in landslide forecasting?, Remote Sens. Environ., 210, 65–75, https://doi.org/10.1016/j.rse.2018.03.016, 2018.
Calvello, M.: Early warning strategies to cope with landslide risk, Rivista Italiana di Geotecnica, 2/2017, 63–91, https://doi.org/10.19199/2017.2.0557-1405.063, 2017.
Canli, E., Mergili, M., Thiebes, B., and Glade, T.: Probabilistic landslide ensemble prediction systems: lessons to be learned from hydrology, Nat. Hazards Earth Syst. Sci., 18, 2183–2202, https://doi.org/10.5194/nhess-18-2183-2018, 2018.
Capra, L., Coviello, V., Borselli, L., Márquez-Ramírez, V.-H., and Arámbula-Mendoza, R.: Hydrological control of large hurricane-induced lahars: evidence from rainfall-runoff modeling, seismic and video monitoring, Nat. Hazards Earth Syst. Sci., 18, 781–794, https://doi.org/10.5194/nhess-18-781-2018, 2018.
Catani, F. and Guzzetti, F. (Eds.): Landslide Prediction & Forecasting, Nat. Nat. Hazards Earth Syst. Sci., https://www.nat-hazards-earth-syst-sci.net/special_issue206.html, 2014.
Catani, F., Casagli, N., Segoni, S., Tofani, V., Jaboyedoff, M., and Günther, A. (Eds.): New developments and applications in early warning, monitoring and remote sensing of landslides, Nat. Hazards Earth Syst. Sci., https://www.nat-hazards-earth-syst-sci.net/special_issue168.html, 2012.
Chaturvedi, P., Arora, A., and Dutt, V.: Learning in an interactive simulation tool against landslide risks: the role of strength and availability of experiential feedback, Nat. Hazards Earth Syst. Sci., 18, 1599–1616, https://doi.org/10.5194/nhess-18-1599-2018, 2018.
Destro, E., Marra, F., Nikolopoulos, E. I., Zoccatelli, D., Creutin, J. D., and Borga, M.: Spatial estimation of debris flows-triggering rainfall and its dependence on rainfall return period, Geomorphology, 278, 269–279, https://doi.org/10.1016/j.geomorph.2016.11.019, 2017.
Devoli, G., Tiranti, D., Cremonini, R., Sund, M., and Boje, S.: Comparison of landslide forecasting services in Piedmont (Italy) and Norway, illustrated by events in late spring 2013, Nat. Hazards Earth Syst. Sci., 18, 1351–1372, https://doi.org/10.5194/nhess-18-1351-2018, 2018.
Frodella, W., Salvatici, T., Pazzi, V., Morelli, S., and Fanti, R.: GB-InSAR monitoring of slope deformations in a mountainous area affected by debris flow events, Nat. Hazards Earth Syst. Sci., 17, 1779–1793, https://doi.org/10.5194/nhess-17-1779-2017, 2017.
Gariano, S. L., Brunetti, M. T., Iovine, G., Melillo, M., Peruccacci, S., Terranova, O., Vennari, C., and Guzzetti, F.: Calibration and validation of rainfall thresholds for shallow landslide forecasting in Sicily, southern Italy, Geomorphology, 228, 653–665, https://doi.org/10.1016/j.geomorph.2014.10.019, 2015.
Glade, T. and Nadim, F.: Early warning systems for natural hazards and risks, Nat. Hazards, 70, 1669–1671, https://doi.org/10.1007/s11069-013-1000-8, 2014.
Greco, R. and Pagano, L.: Basic features of the predictive tools of early warning systems for water-related natural hazards: examples for shallow landslides, Nat. Hazards Earth Syst. Sci., 17, 2213–2227, https://doi.org/10.5194/nhess-17-2213-2017, 2017.
Iadanza, C., Trigila, A., and Napolitano, F.: Identification and characterization of rainfall events responsible for triggering of debris flows and shallow landslides, J. Hydrol., 541, 230–245, https://doi.org/10.1016/j.jhydrol.2016.01.018, 2016.
Intrieri, E., Gigli, G., Casagli, N., and Nadim, F.: Brief communication “Landslide Early Warning System: toolbox and general concepts”, Nat. Hazards Earth Syst. Sci., 13, 85–90, https://doi.org/10.5194/nhess-13-85-2013, 2013.
Intrieri, E., Bardi, F., Fanti, R., Gigli, G., Fidolini, F., Casagli, N., Costanzo, S., Raffo, A., Di Massa, G., Capparelli, G., and Versace, P.: Big data managing in a landslide early warning system: experience from a ground-based interferometric radar application, Nat. Hazards Earth Syst. Sci., 17, 1713–1723, https://doi.org/10.5194/nhess-17-1713-2017, 2017.
Iovine, G. G. R., Huebl, J., Pastor, M., and Sheridan, M. F.: Outcomes of the Special Issue on Approaches to hazard evaluation, mapping, and mitigation, Nat. Hazards Earth Syst. Sci., 11, 2433–2436, https://doi.org/10.5194/nhess-11-2433-2011, 2011.
Kirschbaum, D. B., Stanley, T., and Simmons, J.: A dynamic landslide hazard assessment system for Central America and Hispaniola, Nat. Hazards Earth Syst. Sci., 15, 2257–2272,https://doi.org/10.5194/nhess-15-2257-2015, 2015.
Krøgli, I. K., Devoli, G., Colleuille, H., Boje, S., Sund, M., and Engen, I. K.: The Norwegian forecasting and warning service for rainfall- and snowmelt-induced landslides, Nat. Hazards Earth Syst. Sci., 18, 1427–1450, https://doi.org/10.5194/nhess-18-1427-2018, 2018.
Lacasse, S. and Nadim, F.: Learning to live with geohazards: from research to practice, in: GeoRisk 2011: Geotechnical Risk Assessment and Management, 26–28 June 2011, Atlanta, Georgia, 64–116, https://doi.org/10.1061/41183(418)4, 2011.
Lagomarsino, D., Segoni, S., Rosi, A., Rossi, G., Battistini, A., Catani, F., and Casagli, N.: Quantitative comparison between two different methodologies to define rainfall thresholds for landslide forecasting, Nat. Hazards Earth Syst. Sci., 15, 2413–2423, https://doi.org/10.5194/nhess-15-2413-2015, 2015.
Liu, Y., Qin, Z., Hu, B., and Feng, S.: State fusion entropy for continuous and site-specific analysis of landslide stability changing regularities, Nat. Hazards Earth Syst. Sci., 18, 1187–1199, https://doi.org/10.5194/nhess-18-1187-2018, 2018.
Marra, F.: Rainfall thresholds for landslide occurrence: systematic underestimation using coarse temporal resolution data, Nat. Hazards, 1–8, https://doi.org/10.1007/s11069-018-3508-4, online first, 2018.
Marra, F., Destro, E., Nikolopoulos, E. I., Zoccatelli, D., Creutin, J. D., Guzzetti, F., and Borga, M.: Impact of rainfall spatial aggregation on the identification of debris flow occurrence thresholds, Hydrol. Earth Syst. Sci., 21, 4525–4532, https://doi.org/10.5194/hess-21-4525-2017, 2017.
Melillo, M., Brunetti, M. T., Peruccacci, S., Gariano, S. L., Roccati, A., and Guzzetti, F.: A tool for the automatic calculation of rainfall thresholds for landslide occurrence, Environ. Modell. Softw., 105, 230–243, https://doi.org/10.1016/j.envsoft.2018.03.024, 2018.
Melo, R., van Asch, T., and Zêzere, J. L.: Debris flow run-out simulation and analysis using a dynamic model, Nat. Hazards Earth Syst. Sci., 18, 555–570, https://doi.org/10.5194/nhess-18-555-2018, 2018.
Mendes, R. M., de Andrade, M. R. M., Tomasella, J., de Moraes, M. A. E., and Scofield, G. B.: Understanding shallow landslides in Campos do Jordão municipality – Brazil: disentangling the anthropic effects from natural causes in the disaster of 2000, Nat. Hazards Earth Syst. Sci., 18, 15–30, https://doi.org/10.5194/nhess-18-15-2018, 2018.
Nikolopoulos, E. I., Borga, M., Creutin, J. D., and Marra, F.: Estimation of debris flow triggering rainfall: influence of rain gauge density and interpolation methods, Geomorphology, 243, 40–50, https://doi.org/10.1016/j.geomorph.2015.04.028, 2015.
Pan, H.-L., Jiang, Y.-J., Wang, J., and Ou, G.-Q.: Rainfall threshold calculation for debris flow early warning in areas with scarcity of data, Nat. Hazards Earth Syst. Sci., 18, 1395–1409, https://doi.org/10.5194/nhess-18-1395-2018, 2018.
Pecoraro, G., Calvello, M., and Piciullo, L.: Monitoring strategies for local landslide early warning systems, Landslides, 1–19, https://doi.org/10.1007/s10346-018-1068-z, online first, 2018.
Peres, D. J., Cancelliere, A., Greco, R., and Bogaard, T. A.: Influence of uncertain identification of triggering rainfall on the assessment of landslide early warning thresholds, Nat. Hazards Earth Syst. Sci., 18, 633–646, https://doi.org/10.5194/nhess-18-633-2018, 2018.
Piciullo, L., Gariano, S. L., Melillo, M., Brunetti, M .T., Peruccacci, S., Guzzetti, F., and Calvello, M.: Definition and performance of a threshold-based regional early warning model for rainfall-induced landslides, Landslides, 14, 995–1008, https://doi.org/10.1007/s10346-016-0750-2, 2017.
Piciullo, L., Calvello, M., and Cepeda, J. M.: Territorial early warning systems for rainfall-induced landslides, Earth-Sci. Rev., 179, 228–247, https://doi.org/10.1016/j.earscirev.2018.02.013, 2018.
Posner, A. J. and Georgakakos, K. P.: Soil moisture and precipitation thresholds for realtime landslide prediction in El Salvador, Landslides, 12, 1179–1196, https://doi.org/10.1007/s10346-015-0618-x, 2015.
Reder, A., Rianna, G., and Pagano, L.: Physically based approaches incorporating evaporation for early warning predictions of rainfall-induced landslides, Nat. Hazards Earth Syst. Sci., 18, 613–631, https://doi.org/10.5194/nhess-18-613-2018, 2018.
Reichenbach, P., Günther, A., and Glade, T.: Preface “Landslide hazard and risk assessment at different scales”, Nat. Hazards Earth Syst. Sci., 13, 2169–2171, https://doi.org/10.5194/nhess-13-2169-2013, 2013.
Robbins, J. C.: A probabilistic approach for assessing landslide-triggering event rainfall in Papua New Guinea, using TRMM satellite precipitation estimates, J. Hydrol., 541, 296–309, https://doi.org/10.1016/j.jhydrol.2016.06.052, 2016.
Rosi, A., Lagomarsino, D., Rossi, G., Segoni, S., Battistini, A., and Casagli., N.: Updating EWS rainfall thresholds for the triggering of landslides, Nat. Hazards, 78, 297–308, https://doi.org/10.1007/s11069-015-1717-7, 2015.
Rossi, M., Luciani, S., Valigi, D., Kirschbaum, D., Brunetti, M. T., Peruccacci, S., and Guzzetti, F.: Statistical approaches for the definition of landslide rainfall thresholds and their uncertainty using rain gauge and satellite data, Geomorphology, 285, 16–27, https://doi.org/10.1016/j.geomorph.2017.02.001, 2017.
Salvatici, T., Tofani, V., Rossi, G., D'Ambrosio, M., Tacconi Stefanelli, C., Masi, E. B., Rosi, A., Pazzi, V., Vannocci, P., Petrolo, M., Catani, F., Ratto, S., Stevenin, H., and Casagli, N.: Application of a physically based model to forecast shallow landslides at a regional scale, Nat. Hazards Earth Syst. Sci., 18, 1919–1935, https://doi.org/10.5194/nhess-18-1919-2018, 2018.
Sättele, M., Bründl, M., and Straub, D.: Quantifying the effectiveness of early warning systems for natural hazards, Nat. Hazards Earth Syst. Sci., 16, 149–166, https://doi.org/10.5194/nhess-16-149-2016, 2016.
Segoni, S., Rossi, G., Rosi, A., and Catani, F.: Landslides triggered by rainfall: a semiautomated procedure to define consistent intensity-duration thresholds, Comput. Geosci., 3063, 123–131, https://doi.org/10.1016/j.cageo.2013.10.009, 2014.
Segoni, S., Rosi, A., Lagomarsino, D., Fanti, R., and Casagli, N.: Brief communication: Using averaged soil moisture estimates to improve the performances of a regional-scale landslide early warning system, Nat. Hazards Earth Syst. Sci., 18, 807–812, https://doi.org/10.5194/nhess-18-807-2018, 2018a.
Segoni, S., Piciullo, L., and Gariano, S. L.: A review of the recent literature on rainfall thresholds for landslide occurrence, Landslides, 15, 1483–1501, https://doi.org/10.1007/s10346-018-0966-4, 2018b.
Shi, Z., Wei, F., and Chandrasekar, V.: Radar-based quantitative precipitation estimation for the identification of debris flow occurrence over earthquake-affected regions in Sichuan, China, Nat. Hazards Earth Syst. Sci., 18, 765–780, https://doi.org/10.5194/nhess-18-765-2018, 2018.
Staley, D. M., Kean, J. W., Cannon, S. H., Schmidt, K. M., and Laber, J. L.: Objective definition of rainfall intensity-duration thresholds for the initiation of post-fire debris flows in southern California, Landslides, 10, 547–562, https://doi.org/10.1007/s10346-012-0341-9, 2013.
United Nations International Strategy for Disaster Reduction (UNISDR): Developing Early Warning Systems: A Checklist, available at: http://www.unisdr.org/2006/ppew/info-resources/ewc3/checklist/English.pdf (last access: 16 November 2018), 2006.
Uzielli, M., Rianna, G., Ciervo, F., Mercogliano, P., and Eidsvig, U. K.: Temporal evolution of flow-like landslide hazard for a road infrastructure in the municipality of Nocera Inferiore (southern Italy) under the effect of climate change, Nat. Hazards Earth Syst. Sci., 18, 3019–3035, https://doi.org/10.5194/nhess-18-3019-2018, 2018.
Vaz, T., Zêzere, J. L., Pereira, S., Oliveira, S. C., Garcia, R. A. C., and Quaresma, I.: Regional rainfall thresholds for landslide occurrence using a centenary database, Nat. Hazards Earth Syst. Sci., 18, 1037–1054, https://doi.org/10.5194/nhess-18-1037-2018, 2018.
Vessia, G., Pisano, L., Vennari, C., Rossi, M., and Parise, M.: Mimic expert judgement through automated procedure for selecting rainfall events responsible for shallow landslide: a statistical approach to validation, Comput. Geosci., 86, 146–153, https://doi.org/10.1016/j.cageo.2015.10.015, 2016.
Wei, L.-W., Huang, C.-M., Chen, H., Lee, C.-T., Chi, C.-C., and Chiu, C.-L.: Adopting the I3–R24 rainfall index and landslide susceptibility for the establishment of an early warning model for rainfall-induced shallow landslides, Nat. Hazards Earth Syst. Sci., 18, 1717–1733, https://doi.org/10.5194/nhess-18-1717-2018, 2018.